A lncRNA-immune checkpoint-related gene signature predicts metastasis-free survival in prostate adenocarcinoma
Highlight box
Key findings
• The mRNA expressions of two immune checkpoint genes,
What is known and what is new?
• The growth and development of tumors are associated with immunosuppression;
• The prognostic value of immune checkpoint genes in predicting metastasis-free survival in prostate adenocarcinoma patients.
What is the implication, and what should change now?
• Our study may provide additional evidence for prognostic biomarkers and therapeutic targets for predicting and treating metastatic prostate adenocarcinoma. In addition,
Introduction
Prostate adenocarcinoma (PRAD) is one of the most common cancers in men (1). It is estimated that 98% of patients with metastatic PRAD will have an overall survival of less than 5 years (2,3). Therefore, the development of a model to predict the metastasis-free survival (MFS) of PRAD patients is conducive to timely treatment.
Although many biomarkers or genetic markers have been identified that have the potential to predict MFS in PRAD patients, they have not yet been applied in clinical settings and are still in the molecular research stage. Therefore, it is important to continue investigating the genetic characteristics that can predict MFS in PRAD patients.
The growth and development of tumors are associated with immunosuppression and the ability to activate different immune checkpoint pathways that have immunosuppressive functions (4). Over the past decade, immunotherapy has made great strides in cancer treatment (5). However, the association between immune checkpoint genes (ICGs) and cancer is extremely complicated. The prognostic value of ICGs in predicting MFS in PRAD patients has not yet been elucidated.
In this study, a bioinformatics analysis was performed to investigate ICGs’ expression pattern, prognostic utility, and associated mechanisms in PRAD patients. Our data may provide additional evidence for prognostic biomarkers and therapeutic targets for predicting and treating metastatic PRAD. We present the following article in accordance with the TRIPOD reporting checklist (available at https://tau.amegroups.com/article/view/10.21037/tau-22-711/rc).
Methods
Patients and datasets
We obtained the expression profiles of PRAD tumor samples and adjacent normal tissues from The Cancer Genome Atlas (TCGA) database (https://portal.gdc.cancer.gov/), as well as the MFS duration and status of patients with PRAD. The study was conducted in accordance with the Declaration of Helsinki (as revised in 2013).
Acquisition of ICGs
A hub of 60 ICGs was extracted from a previously reported article (6). The expression profiles of these 60 genes in PRAD tumor samples and adjacent normal tissue samples were obtained from the TCGA-PRAD dataset.
Univariate analysis
We used the “survival” R software version 4.1.3 package to integrate the MFS duration, MFS status, and individual gene expression data and evaluated the prognostic significance of each gene using Cox regression. R is a free programming language application.
Stepwise regression, Cox regression, and nomogram analysis
We used the “survival” R software package to integrate the MFS duration, MFS status, and expression of multiple genes and evaluated the prognostic significance of multiple genes using Cox regression. The risk score was also calculated.
Kaplan-Meier (KM) survival
The samples were divided into low- and high-expression groups according to the median gene expression or risk-score value. KM-survival analysis was used to evaluate the gene expression or risk score for MFS in PRAD patients.
In-house patients and tissues
A total of 100 tumor tissues and 50 adjacent normal tissues from PRAD patients (55±11.6 years) who underwent surgical treatment (without chemotherapy) were obtained from January 2020 to January 2021 in Shanghai Changhai Hospital. Patient outcomes were assessed by MFS survival, defined as the time from diagnosis to the occurrence of the first tumor metastasis. Written informed consent was obtained from all participants. The research protocol was approved by the Committee on Ethics of Medicine, Navy Medical University.
Immunohistochemistry (IHC)
Rabbit monoclonal antibodies for ADORA2A (ab260032) and TNFRSF18 (ab223841) were purchased from Abcam (USA). After slicing into 4-µm sections, all tissues were deparaffinized and treated with ethylenediamine tetraacetic acid (EDTA) (pH 9.0) for antigen retrieval in a microwave for 20 min. We used an Autostainer Link 48 machine (Dako, Denmark A/S, Denmark) for the staining. Subsequently, primary antibodies for ADORA2A (rabbit monoclonal, 1:100 dilution) and TNFRSF18 (rabbit monoclonal, 1:200 dilution) were added to the sections, while phosphate buffered saline (PBS) buffer was used as a blank control instead of the antibody. An EnVision Flex Kit (Dako, Denmark A/S, Denmark) was used as the second antibody. Two senior pathologists examined all cases to validate the initial scores. The percentage of positively stained cells and staining scores were used to assess the IHC results according to the methodology described in previous articles (7,8). The Gene-Score = (percentage of cells of weak intensity ×1) + (percentage of cells of moderate intensity ×2) + percentage of cells of strong intensity ×3).
Real-time fluorescence quantitative polymerase chain reaction (RT-PCR)
Trizol was used to extract total RNA from the tissues. Total RNA was reverse transcribed into cDNA using a RevertAidTM First Strand cDNA Synthesis Kit for qPCR (K1622, Genecopeia, China). A Power SYBR Green PCR Master Mix (4368708, Applied Biosystems, USA) was used for the PCR detection. All primers used in this study are shown in Table S1. The relative expression was calculated using the 2−ΔΔCt method.
Mutation analysis
The single nucleotide variant (SNV) data from PRAD patients was obtained from the TCGA dataset. We divided the samples into low- and high-expression groups according to the gene expression level and then conducted an SNV analysis.
Drug sensitivity
According to the gene expression level, we divided the samples into low- and high-expression groups, and used the “pRRophetic” R package to predict the clinical chemotherapy response from the tumor gene expression levels (9).
Statistical analysis
The data analysis was performed using IBM®SPSS®, version 24.0 (IBM Corp., Armonk, NY, USA). The data are expressed as mean ± standard deviation. The Kruskal-Wallis test was used to compare data among multiple groups, and the Wilcoxon test was used to compare data between two groups. The Pearson correlation coefficient was used for the correlation analysis. A P value <0.05 was considered statistically significant.
Results
Landscape of the expression variation and prognostic value of ICGs in PRAD
Compared with adjacent normal tissues, the mNRA expression of CD40, CX3CL1, VTCN1, EDNRB, CD274, IL1A, ENTPD1, TLR4, IL12A, BTN3A1, BTN3A2, IFNA1, LAG3, VEGFA, and TGFB1 were downregulated, while the mNRA expression of TNFRSF14, TNFRSF18, CTLA4, CD276, IL2RA, ADORA2A, CXCL10, CXCL9, CD80, TNFRSF9, TIGIT, CD28, ARG1, KIR2DL3, PDCD1, TNFRSF4, and ICAM1 were upregulated in PRAD tumor tissues (Figure 1A, Table S2). A KM-survival analysis was used to determine the effect of ICGs on MFS in PRAD patients, and the results showed that a high expression of TGFB1, ADORA2A, IL2RA, TNFRSF18, TNFRSF4, or CD80 was significantly positively associated with poor MFS in PRAD patients (Figure 1B, Table S3).
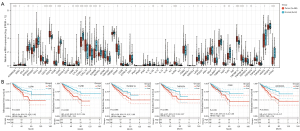
Construction of the ADORA2A/TNFRSF18 prognostic gene model and the predictive nomogram
In the TCGA dataset, univariate analysis showed that the mRNA expression of a 6-gene cluster (TGFB1, ADORA2A, IL2RA, TNFRSF18, TNFRSF4, and CD80) could be used as a clinical indicator to predict MFS in PRAD patients (Figure 2A). Further stepwise regression analysis showed that the mRNA expression of ADORA2A and TNFRSF18 were independent predictive factors for MFS in PRAD patients in this 6-gene cluster (Figure 2B). A Cox regression analysis was performed to construct a prognostic gene model based on the mRNA expression of ADORA2A and TNFRSF18, and the risk score was calculated for the TCGA dataset (Figure 2C). Based on the risk score, PRAD patients were separated into two groups. The KM-survival result showed that MFS rates were worse in the high-risk-score group compared with the low-risk-score group (Figure 2D). We also constructed a nomogram to predict the MFS probability. The predictive nomogram suggested that 12-, 36-, and 60-month MFS rates could be predicted relatively well based on the mRNA expression of ADORA2A and TNFRSF18 (Figure 2E,2F).
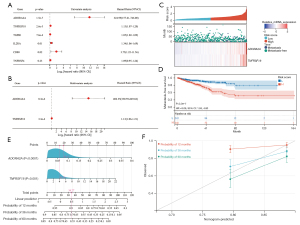
In our in-house cohort, the IHC results showed that the protein expression of ADORA2A and TNFRSF18 was increased in PRAD tumor tissues compared with adjacent normal tissues (Figure 3A). A high protein expression of ADORA2A or TNFRSF18 was also significantly positively correlated with poorer MFS in PRAD patients (Figure 3B). A Cox regression analysis was performed to construct a prognostic gene model based on the protein expression of ADORA2A and TNFRSF18, and the risk score was calculated (Figure 3C). Based on the risk score, PRAD patients were separated into two groups. The KM-survival result showed that the MFS rates were worse for the high-risk-score PRAD patients compared with the low-risk-score PRAD patients (Figure 3C). We also constructed a nomogram to predict the MFS probability. The predictive nomogram suggested that the 36-month MFS rates could be predicted relatively well based on the protein expression of ADORA2A and TNFRSF18 (Figure 3D).
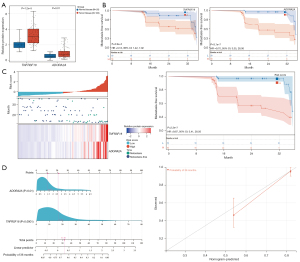
Prediction of the long non-coding RNAs (lncRNAs) related to ADORA2A/TNFRSF18 genes and the estimation of their prognostic utility
According to the median ADORA2A or TNFRSF18 mRNA expression, PRAD patients in the TCGA dataset were divided into a low-ADORA2A group, a high-ADORA2A group, a low-TNFRSF18 group, and a high-TNFRSF18 group. The differentially expressed (DE)-lncRNAs were screened between the low- and high-ADORA2A groups and between the low- and high-TNFRSF18 groups (Figure 4A). A hub of 44 DE-lncRNAs was found to be related to ADORA2A and TNFRSF18 (Figure 4B). Univariate analysis showed that the mRNA expression of a 17-gene cluster (SLC9A3-AS1, AL139287.1, AC110285.2, AC087741.1, SNHG1, MELTF-AS1, RP11-258C19.7, LINC01089, AHSA2P, ASMTL-AS1, SNHG12, AC073869.1, NSUN5P1, AL390728.6, NAPSB, SNHG3, and AC132872.1) could be used as a clinical indicator to predict MFS in PRAD patients (Figure 4C). Further stepwise regression analysis of the 17-gene cluster showed that the mRNA expressions of AL139287.1, SLC9A3-AS1, NAPSB, SNHG12, and AC110285.2 could be used as independent predictors of MFS in PRAD patients (Figure 4D). A Cox regression analysis was performed to construct a prognostic gene model based on the mRNA expression of AL139287.1, SLC9A3-AS1, NAPSB, SNHG12, and AC110285.2, and the risk score was calculated (Figure 4E). Based on the risk score, PRAD patients were separated into two groups. The KM-survival analysis showed that the MFS rates were worse in the high-risk-score group compared with the low-risk-score group (Figure 4E). We also constructed a nomogram to predict the MFS probability. The predictive nomogram suggested that 12-, 36-, and 60-month MFS rates could be predicted relatively well based on the mRNA expression of AL139287.1, SLC9A3-AS1, NAPSB, and SNHG12 (Figure 4F).
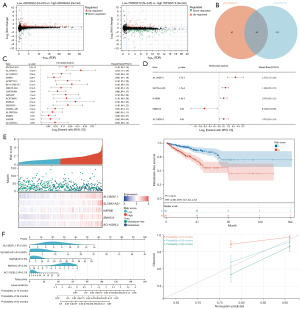
A Pearson correlational analysis was conducted for the 7-gene cluster (ADORA2A, TNFRSF18, AL139287.1, SLC9A3-AS1, NAPSB, SNHG12, and AC110285.2) (Figure 5A). Further stepwise regression analysis showed that the mRNA expressions of AL139287.1, SLC9A3-AS1, SNHG12, ADORA2A, and TNFRSF18 were independent predictive factors of MFS in PRAD patients (Figure 5B). A Cox regression analysis was performed to construct a prognostic gene model based on the mRNA expression of AL139287.1, SLC9A3-AS1, SNHG12, ADORA2A, and TNFRSF18, and the risk score was calculated (Figure 5C). Based on the risk score, PRAD patients were separated into two groups. The KM-survival analysis showed that the MFS rates were worse in the high-risk-score group compared with the low-risk-score group (Figure 5D). We also constructed a nomogram to predict the MFS probability. The predictive nomogram suggested that 12-, 36-, and 60-month MFS rates could be predicted relatively well based on the mRNA expressions of AL139287.1, SLC9A3-AS1, SNHG12, ADORA2A, and TNFRSF18 (Figure 5E,5F).
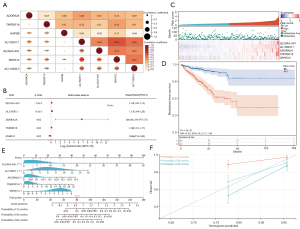
In our in-house cohort, the mRNA expressions of AL139287.1, SLC9A3-AS1, SNHG12, ADORA2A, and TNFRSF18 were detected by RT-PCR assay. The KM-survival analysis showed that a high expression of AL139287.1, SLC9A3-AS1, SNHG12, ADORA2A, or TNFRSF18 was significantly positively associated with poor MFS rates in PRAD patients (Figure 6A). A Cox regression analysis was performed to construct a prognostic gene model based on the mRNA expression of AL139287.1, SLC9A3-AS1, SNHG12, ADORA2A, and TNFRSF18, and the risk score was calculated (Figure 6B). Based on the risk score, PRAD patients were separated into two groups. The KM-survival analysis showed that the MFS rates were worse in the high-risk-score PRAD patients than in the low-risk-score PRAD patients (Figure 6B). We also constructed a nomogram to predict the MFS probability. The predictive nomogram suggested that 36-month MFS rates could be predicted relatively well based on the mRNA expression of AL139287.1, SLC9A3-AS1, and TNFRSF18 (Figure 6C).
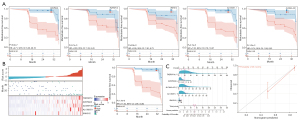
Mutation analysis of ICGs
In the TCGA dataset, the SNV mutation of USH2A, ABCA13, and PCDH15 was increased in the high-AL139287.1 group compared with the low-AL139287.1 group (Figure 7A). The SNV mutation of RYR1, APC, RNF213, and SORCS1 was increased, while the SNV mutation of PTEN was reduced in the high-SHNG12 group compared with the low-SHNG12 group (Figure 7B). The SNV mutation of LRP1B, ALMS1, CNTN6, SRCAP, LYST, UBR4, ZC3H13, and ERF was increased in the high-SLC9A3-AS1 group compared with the low-SLC9A3-AS1 group (Figure 7C). The SNV mutation of RYR1, MXRA5, SACS, DNHD1, SRCAP, LYST, and ZC3H13 was increased in the high-ADORA2A group compared with the low-ADORA2A group (Figure 7D). The SNV mutation of TP53, LRP1B, CUBN, MYO3A, RNF43, MYH10, HTR1E, and PCDH10 was increased in the high-TNFRSF18 group compared with the low-TNFRSF18 group (Figure 7E). The SNV mutation of KMT2C, SACS, ALMS1, SRCAP, NEB, ZC3H13, and ERF was increased in the high-TNFRSF18 group compared with the low-TNFRSF18 group (Figure 7F).
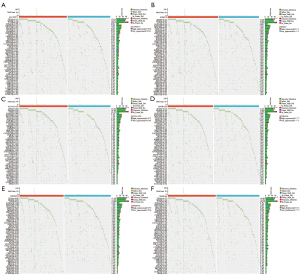
Prediction of AL139287.1, SLC9A3-AS1, SNHG12, ADORA2A, and TNFRSF18 genes on drug sensitivity
Paclitaxel sensitivity (IC50) demonstrated a significant negative correlation with the mRNA expression of ADORA2A (Figure 8A). Erlotinib sensitivity (IC50) had a significant negative correlation with the mRNA expression of TNFRSF18 (Figure 8B) and AL139287.1 (Figure 8C). Erlotinib and vinorelbine sensitivity (IC50) showed a significant negative correlation with the mRNA expression of SLC9A3-AS1 (Figure 8D). Doxorubicin, erlotinib, gemcitabine, and vinorelbine sensitivity (IC50) demonstrated a significant negative correlation with the mRNA expression of SNHG12 (Figure 8E).
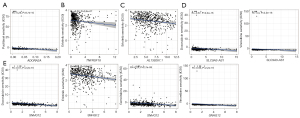
Discussion
Cancer immunotherapy targeting adaptive ICGs has significantly improved patient outcomes in multiple metastatic cancer types (10). However, the role of ICGs in predicting the MFS of PRAD patients and treating metastatic PRAD patients has not yet been elucidated. Therefore, we performed the current study to clarify this role.
We first clarified the expression and prognostic value of ICGs in PRAD. The mNRA expression of TNFRSF14, TNFRSF18, CTLA4, CD276, IL2RA, ADORA2A, CXCL10, CXCL9, CD80, TNFRSF9, TIGIT, CD28, ARG1, KIR2DL3, PDCD1, TNFRSF4, and ICAM1 were upregulated, while the mNRA expression of CD40, CX3CL1, VTCN1, EDNRB, CD274, IL1A, ENTPD1, TLR4, IL12A, BTN3A1, BTN3A2, IFNA1, LAG3, VEGFA, and TGFB1 were downregulated in tumor tissues compared with adjacent normal tissues. The prognostic analysis suggested poorer MFS rates in PRAD patients with a high expression of TGFB1, ADORA2A, IL2RA, TNFRSF18, TNFRSF4, or CD80. These data were consistent with prior results. A high level of TGFB1 has been found to enhance PRAD metastasis by inhibiting the immune response to tumor cells and stimulating angiogenesis (11). ADORA2A has been shown to promote lymph node metastasis and lymphangiogenesis (12). Elevated IL2RA may also play an important role in promoting melanoma metastasis (13), and CD80 was highly expressed in non-small cell lung carcinomas and positively correlated with distant metastasis (14).
Univariate analysis and stepwise regression were performed to estimate the prognostic utility of TGFB1, ADORA2A, IL2RA, TNFRSF18, TNFRSF4, or CD80 in PRAD. ADORA2A and TNFRSF18 were able to predict MFS in PRAD patients with medium-high accuracy. A Cox regression analysis was performed to construct a prognostic gene model based on the mRNA expression of ADORA2A and TNFRSF18, which significantly predicted the MFS of PRAD patients. The nomogram survival diagram showed that the mRNA expression of ADORA2A and TNFRSF18 had a good ability to predict 12-, 36- and 60-month MFS compared with an ideal model of the whole cohort. A Cox regression analysis and a nomogram survival diagram were also conducted based on the protein expression of ADORA2A and TNFRSF18 in our in-house cohort, and ADORA2A and TNFRSF18 protein expression showed a good clinical ability to predict MFS. Previous studies have developed and validated an immune-related prognostic signature for predicting the recurrence of PRAD (15,16). Our study is the first to identify an ICG prognostic signature for predicting the MFS of PRAD patients, which provides more choices for prognostic prediction in PRAD.
Immuno-related lncRNA prognostic markers of PRAD have been previously reported (17). In this study, a hub of 44 DE-lncRNAs was related to ADORA2A and TNFRSF18. Univariate analysis and stepwise regression analysis showed that the mRNA expression of AL139287.1, SLC9A3-AS1, NAPSB, SNHG12, and AC110285.2 could be used as independent factors to predict MFS in PRAD patients. A Cox regression analysis was performed to construct a prognostic gene model based on the mRNA expression of AL139287.1, SLC9A3-AS1, NAPSB, SNHG12, and AC110285.2, which significantly predicted the MFS of PRAD patients. The nomogram survival diagram showed that the mRNA expression of AL139287.1, SLC9A3-AS1, NAPSB, SNHG12, and AC110285.2 predicted 12-, 36-, and 60-month MFS better than an ideal model of the whole cohort. A high expression of SNHG12 has been found to promote tumor metastasis in various tumor types (18-20). Our study identified an ICG-related lncRNA prognostic signature for predicting the MFS of PRAD patients, which provides more choices for prognostic prediction in PRAD.
We conducted a correlation analysis of ADORA2A, TNFRSF18, AL139287.1, SLC9A3-AS1, NAPSB, SNHG12, and AC110285.2. A further stepwise regression analysis showed that the mRNA expressions of AL139287.1, SLC9A3-AS1, SNHG12, ADORA2A, and TNFRSF18 could be used as independent factors to predict MFS in PRAD patients. A Cox regression analysis was performed to construct a prognostic gene model based on the mRNA expression of AL139287.1, SLC9A3-AS1, SNHG12, ADORA2A, and TNFRSF18, which significantly predicted the MFS of PRAD patients in both the TCGA dataset and our in-house cohort. We also built a nomogram to predict MFS based on the mRNA expression of AL139287.1, SLC9A3-AS1, SNHG12, ADORA2A, and TNFRSF18 in both the TCGA dataset and our in-house cohort. The nomogram survival diagram showed that the mRNA expression of AL139287.1, SLC9A3-AS1, SNHG12, ADORA2A, and TNFRSF18 was able to provide a good prediction of 12-, 36-, and 60-month MFS in the TCGA cohort, and the mRNA expression of AL139287.1, SLC9A3-AS1 and TNFRSF18 provided a good prediction of 36-month MFS in our in-house cohort compared with an ideal model of the entire cohort.
Large-scale sequencing studies have shown that mutational events occur at various stages of PRAD progression (21). Compared with the low-AL139287.1 group, the SNV mutation of USH2A, ABCA13, and PCDH15 was increased in the high-AL139287.1 group in the TCGA dataset. USH2A mutations have been found to be associated with tumor mutation burden and anti-tumor immunity in colorectal adenocarcinoma patients (22). Whole exome sequencing has suggested that the PCDH15 mutation is associated with metastasis of ocular adnexal sebaceous gland carcinoma (23). Compared with the low-SHNG12 group, the SNV mutation of RYR1, APC, RNF213, and SORCS1 was increased, while the SNV mutation of PTEN was reduced, in the high-SHNG12 group in the TCGA dataset. PTEN is one of the most common mutated genes in malignant tumors, and a mutated PTEN has been reported to inhibit tumor metastasis (24). Compared with the low-SLC9A3-AS1 group, the SNV mutation of LRP1B, ALMS1, CNTN6, SRCAP, LYST, UBR4, ZC3H13, and ERF was increased in the high-SLC9A3-AS1 group in the TCGA dataset. It has been reported that lymph node metastasis of esophagogastric junction adenocarcinoma is associated with LRP1B mutation (25). The SNV mutation of RYR1, MXRA5, SACS, DNHD1, SRCAP, LYST, and ZC3H13 was increased in the high-ADORA2A group compared with the low-ADORA2A group, and the SNV mutation of TP53, LRP1B, CUBN, MYO3A, RNF43, MYH10, HTR1E, and PCDH10 was increased in the high-TNFRSF18 group compared with the low-TNFRSF18 group in the TCGA dataset. TP53 mutations are known to act as a driver of metastatic signaling in patients with advanced cancer (26), and RNF43 mutations are associated with overall survival in colorectal cancer (27). The SNV mutation of KMT2C, SACS, ALMS1, SRCAP, NEB, ZC3H13, and ERF was increased in the high-risk-score group compared with the low-risk-score group in the TCGA dataset. KMT2C mutations have been associated with poorer survival in adult medulloblastomas (28), and whole-exome analysis in osteosarcoma has shown that metastasis is associated with NEB mutations (29).
Erlotinib (30), paclitaxel (31), vinorelbine (32), gemcitabine (33), and doxorubicin (34) are commonly used to treat PRAD. However, tumors usually overcome the cytotoxic effects of chemotherapy through acquired or environment-mediated drug resistance (35). In this study, the IC50 of paclitaxel was found to be significantly negatively correlated with the mRNA expression of ADORA2A, the IC50 of erlotinib was significantly negatively correlated with the mRNA expression of TNFRSF18 and AL139287.1, the IC50 of erlotinib and vinorelbine was significantly negatively correlated with the mRNA expression of SLC9A3-AS1, and the IC50s of doxorubicin, erlotinib, gemcitabine, and vinorelbine were significantly negatively correlated with the mRNA expression of SNHG12. These results suggest that PRAD patients with a high expression of these genes may be more sensitive to treatment with these five chemotherapy drugs.
Our study has some limitations. TCGA PRAD queues were used for most of the analysis, requiring validation with more queues. In addition, in vivo and in vitro experiments are needed to further confirm our results.
Conclusions
In conclusion, we performed a comprehensive and systematic bioinformatics analysis and identified the ICG-related prognostic genes and lncRNA signatures containing five genes (AL139287.1, SLC9A3-AS1, SNHG12, ADORA2A, and TNFRSF18) for predicting the MFS of PRAD patients. Further studies should be conducted to verify this result.
Acknowledgments
Funding: None.
Footnote
Reporting Checklist: The authors have completed the TRIPOD reporting checklist. Available at https://tau.amegroups.com/article/view/10.21037/tau-22-711/rc
Data Sharing Statement: Available at https://tau.amegroups.com/article/view/10.21037/tau-22-711/dss
Conflicts of Interest: All authors have completed the ICMJE uniform disclosure form (available at https://tau.amegroups.com/article/view/10.21037/tau-22-711/coif). The authors have no conflicts of interest to declare.
Ethical Statement: The authors are accountable for all aspects of the work in ensuring that questions related to the accuracy or integrity of any part of the work are appropriately investigated and resolved. This study obtained written informed consent from all patients. This study received approval from the Committee on Ethics of Medicine, Navy Medical University. The study was conducted in accordance with the Declaration of Helsinki (as revised in 2013).
Open Access Statement: This is an Open Access article distributed in accordance with the Creative Commons Attribution-NonCommercial-NoDerivs 4.0 International License (CC BY-NC-ND 4.0), which permits the non-commercial replication and distribution of the article with the strict proviso that no changes or edits are made and the original work is properly cited (including links to both the formal publication through the relevant DOI and the license). See: https://creativecommons.org/licenses/by-nc-nd/4.0/.
References
- Litwin MS, Tan HJ. The Diagnosis and Treatment of Prostate Cancer: A Review. JAMA 2017;317:2532-42. [Crossref] [PubMed]
- Wang G, Zhao D, Spring DJ, et al. Genetics and biology of prostate cancer. Genes Dev 2018;32:1105-40. [Crossref] [PubMed]
- Tangen CM, Faulkner JR, Crawford ED, et al. Ten-year survival in patients with metastatic prostate cancer. Clin Prostate Cancer 2003;2:41-5. [Crossref] [PubMed]
- Darvin P, Toor SM, Sasidharan Nair V, et al. Immune checkpoint inhibitors: recent progress and potential biomarkers. Exp Mol Med 2018;50:1-11. [Crossref] [PubMed]
- Li B, Chan HL, Chen P. Immune Checkpoint Inhibitors: Basics and Challenges. Curr Med Chem 2019;26:3009-25. [Crossref] [PubMed]
- Thorsson V, Gibbs DL, Brown SD, et al. The Immune Landscape of Cancer. Immunity 2018;48:812-830.e14. [Crossref] [PubMed]
- Azim HA Jr, Peccatori FA, Brohée S, et al. RANK-ligand (RANKL) expression in young breast cancer patients and during pregnancy. Breast Cancer Res 2015;17:24. [Crossref] [PubMed]
- Fehrenbacher L, Spira A, Ballinger M, et al. Atezolizumab versus docetaxel for patients with previously treated non-small-cell lung cancer (POPLAR): a multicentre, open-label, phase 2 randomised controlled trial. Lancet 2016;387:1837-46. [Crossref] [PubMed]
- Geeleher P, Cox N, Huang RS. pRRophetic: an R package for prediction of clinical chemotherapeutic response from tumor gene expression levels. PLoS One 2014;9:e107468. [Crossref] [PubMed]
- Feng M, Jiang W, Kim BYS, et al. Phagocytosis checkpoints as new targets for cancer immunotherapy. Nat Rev Cancer 2019;19:568-86. [Crossref] [PubMed]
- Wikström P, Damber J, Bergh A. Role of transforming growth factor-beta1 in prostate cancer. Microsc Res Tech 2001;52:411-9. [Crossref] [PubMed]
- Allard B, Cousineau I, Allard D, et al. Adenosine A2a receptor promotes lymphangiogenesis and lymph node metastasis. Oncoimmunology 2019;8:1601481. [Crossref] [PubMed]
- Zhang C, Dang D, Cong L, et al. Pivotal factors associated with the immunosuppressive tumor microenvironment and melanoma metastasis. Cancer Med 2021;10:4710-20. [Crossref] [PubMed]
- Sato T, Takagi K, Higuchi M, et al. Immunolocalization of CD80 and CD86 in Non-Small Cell Lung Carcinoma: CD80 as a Potent Prognostic Factor. Acta Histochem Cytochem 2022;55:25-35. [Crossref] [PubMed]
- Fu M, Wang Q, Wang H, et al. Immune-Related Genes Are Prognostic Markers for Prostate Cancer Recurrence. Front Genet 2021;12:639642. [Crossref] [PubMed]
- Lv D, Wu X, Chen X, et al. A novel immune-related gene-based prognostic signature to predict biochemical recurrence in patients with prostate cancer after radical prostatectomy. Cancer Immunol Immunother 2021;70:3587-602. [Crossref] [PubMed]
- Liang L, Xia W, Yao L, et al. Long non-coding RNA profile study identifies an immune-related lncRNA prognostic signature for prostate adenocarcinoma. Int Immunopharmacol 2021;101:108267. [Crossref] [PubMed]
- Zhang T, Beeharry MK, Wang Z, et al. YY1-modulated long non-coding RNA SNHG12 promotes gastric cancer metastasis by activating the miR-218-5p/YWHAZ axis. Int J Biol Sci 2021;17:1629-43. [Crossref] [PubMed]
- Yang HG, Wang TP, Hu SA, et al. Long Non-coding RNA SNHG12, a New Therapeutic Target, Regulates miR-199a-5p/Klotho to Promote the Growth and Metastasis of Intrahepatic Cholangiocarcinoma Cells. Front Med (Lausanne) 2021;8:680378. [Crossref] [PubMed]
- Ding S, Qu W, Jiao Y, et al. LncRNA SNHG12 promotes the proliferation and metastasis of papillary thyroid carcinoma cells through regulating wnt/β-catenin signaling pathway. Cancer Biomark 2018;22:217-26. [Crossref] [PubMed]
- Van Etten JL, Dehm SM. Clonal origin and spread of metastatic prostate cancer. Endocr Relat Cancer 2016;23:R207-17. [Crossref] [PubMed]
- Sun Y, Li L, Yao W, et al. USH2A Mutation is Associated With Tumor Mutation Burden and Antitumor Immunity in Patients With Colon Adenocarcinoma. Front Genet 2021;12:762160. [Crossref] [PubMed]
- Xu S, Moss TJ, Laura Rubin M, et al. Whole-exome sequencing for ocular adnexal sebaceous carcinoma suggests PCDH15 as a novel mutation associated with metastasis. Mod Pathol 2020;33:1256-63. [Crossref] [PubMed]
- Zhang Q, Liang H, Zhao X, et al. PTENε suppresses tumor metastasis through regulation of filopodia formation. EMBO J 2021;40:e105806. [Crossref] [PubMed]
- Han R, Chen G, Li M, et al. Screening and clinical significance of lymph node metastasis-related genes within esophagogastric junction adenocarcinoma. Cancer Med 2021;10:5088-100. [Crossref] [PubMed]
- Pandey R, Johnson N, Cooke L, et al. TP53 Mutations as a Driver of Metastasis Signaling in Advanced Cancer Patients. Cancers (Basel) 2021;13:597. [Crossref] [PubMed]
- Wen D, Wang G, Huang Z, et al. Reduced Frequency and Prognostic Significance of Ring Finger Protein 43 Nucleotide Polymorphisms in a Chinese Colorectal Cancer Cohort. DNA Cell Biol 2019;38:541-8. [Crossref] [PubMed]
- Wong GC, Li KK, Wang WW, et al. Clinical and mutational profiles of adult medulloblastoma groups. Acta Neuropathol Commun 2020;8:191. [Crossref] [PubMed]
- Chiappetta C, Mancini M, Lessi F, et al. Whole-exome analysis in osteosarcoma to identify a personalized therapy. Oncotarget 2017;8:80416-28. [Crossref] [PubMed]
- Gross M, Higano C, Pantuck A, et al. A phase II trial of docetaxel and erlotinib as first-line therapy for elderly patients with androgen-independent prostate cancer. BMC Cancer 2007;7:142. [Crossref] [PubMed]
- Yang YH, Mao JW, Tan XL. Research progress on the source, production, and anti-cancer mechanisms of paclitaxel. Chin J Nat Med 2020;18:890-7. [Crossref] [PubMed]
- Roviello G, Corona SP, Conca R, et al. Is there still a place for vinorelbine in advanced metastatic castration resistant prostate cancer? Medicine (Baltimore) 2019;98:e16249. [Crossref] [PubMed]
- Correia C, Xavier CPR, Duarte D, et al. Development of potent CPP6-gemcitabine conjugates against human prostate cancer cell line (PC-3). RSC Med Chem 2020;11:268-73. [Crossref] [PubMed]
- Sun G, Sun K, Sun J. Combination prostate cancer therapy: Prostate-specific membranes antigen targeted, pH-sensitive nanoparticles loaded with doxorubicin and tanshinone. Drug Deliv 2021;28:1132-40. [Crossref] [PubMed]
- Karagiannis GS, Condeelis JS, Oktay MH. Chemotherapy-induced metastasis: mechanisms and translational opportunities. Clin Exp Metastasis 2018;35:269-84. [Crossref] [PubMed]
(English Language Editor: D. Fitzgerald)