Bioinformatics analysis of prognosis and immune microenvironment of immunological cell death-related gemcitabine-resistance genes in bladder cancer
Highlight box
Key findings
• The present study constructed a prognostic model containing 5 gemcitabine resistance-related ICD-associated genes.
• The prognosis of the ICD-high group was significantly better than that those in the ICD-low group in bladder cancer patients.
What is known and what is new?
• ICD-associated genes play an important role in the development of BC, and gemcitabine resistance also affects the prognosis of patients with BC.
• The present study highlighted the functional role of gemcitabine resistance-related ICD-associated genes in bladder cancer outcome prediction.
What is the implication, and what should change now?
• The present study constructed a prognostic score for the outcome evaluation and searched for potential targets to overcome gemcitabine chemoresistance in bladder cancer.
• The pathways and mechanisms involved in immunological cell death and chemoresistance deserve more in–depth researches to improve the outcome of bladder cancer.
Introduction
Bladder cancer (BC) is the most common malignant tumor of the urinary system and is estimated to account for 80,000 new cases and 170,000 deaths worldwide each year (1-3). The latest cancer statistics report that BC is the 6th and 7th most common cause of cancer-related deaths in males and in females, respectively, in China (4). Nearly 70% of cases have non-muscle-invasive bladder cancer (NMIBC), and 30% have muscle-invasive bladder cancer (MIBC) (5). The typical treatment for NMIBC is the transurethral resection of the bladder tumor, while that for MIBC is the radical removal of the entire bladder. However, the 5-year survival rate of BC patients with recurrence and metastasis is <5% (6).
Despite remarkable breakthroughs in tumor treatments over the past decade, chemotherapy is still the 1st-line treatment for BC. Gemcitabine is widely applied in adjuvant and palliative chemotherapy to treat recurrence and metastasis in BC (7,8). As the 1st-line therapy, chemotherapy is effective in 70% of metastatic BC cases. The lack of any 2nd-line chemotherapeutics contributes to the poor outcomes of BC patients. TNM staging system, as well as Spanish Urological Club for Oncological Treatment (CUETO) scoring system, are widely used for outcome prediction in resectable BC. Nevertheless, the prognosis of patients with similar stage still varies in response to chemotherapy, recurrence, and disease progression (9). Thus, potential therapeutic targets urgently need to be developed and chemoresistance against gemcitabine needs to be overcome to improve the outcomes of BC patients.
As a type of programmed cell death, immunological cell death (ICD) is widely accepted as a unique model of cell death triggered by a series of anti-tumor therapies (10). ICD in tumor cells is accompanied by damage-associated molecular patterns (DAMPs), which can be recognized by antigen presenting cells, and further induce anti-tumor immunity (11). Further, ICD induced by anti-tumor therapeutics may enhance patients’ sensitivity to chemotherapy and radiotherapy by immunoregulation (12,13). Thus, ICD is closely correlated to chemosensitivity, chemoresistance, and the tumor immune microenvironment. The present study sought to identify gemcitabine resistance-related ICD-associated genes and explore potential treatment targets for BC. We present the following article in accordance with the TRIPOD reporting checklist (available at https://tau.amegroups.com/article/view/10.21037/tau-22-736/rc).
Methods
Data acquisition and processing
Transcriptome RNA-sequencing and clinical data for BC patients were obtained from The Cancer Genome Atlas (TCGA) data portal (https://portal.gdc.cancer.gov/). A total of 412 BC patients were included in the present study. The ICD-related gene list was obtained from Garg et al. (14). The transcriptome profiling data of 92 patients with BC in the GSE24450 data set from the Gene Expression Omnibus (GEO) database were used for validation. The study was conducted in accordance with the Declaration of Helsinki (as revised in 2013). Patient characteristics are detailed in Table 1.
Table 1
Clinical features | Numbers of patients (n=398) |
---|---|
Age (years), median [range] | 68.5 [34–89] |
Gender, n (%) | |
Female | 102 (25.63) |
Male | 296 (74.37) |
T stage, n (%) | |
T1 | 4 (1.01) |
T2 | 116 (29.15) |
T3 | 190 (47.74) |
T4 | 56 (14.07) |
Unknown | 32 (8.04) |
N stage, n (%) | |
0 | 230 (57.79) |
1 | 44 (11.06) |
2 | 75 (18.84) |
3 | 6 (1.51) |
Unknown | 43 (10.80) |
Grade, n (%) | |
High | 375 (94.22) |
Low | 20 (5.03) |
Unknown | 3 (0.75) |
AJCC stage, n (%) | |
I | 2 (0.50) |
II | 126 (31.66) |
III | 138 (34.67) |
IV | 130 (32.67) |
Unknown | 2 (0.50) |
BC, bladder cancer; TCGA, The Cancer Genome Atlas; T stage, tumor stage; N stage, lymph node stage; AJCC, American Joint Committee on Cancer.
Consensus clustering
The ICD-associated molecular subtypes were identified by ConsensusClusterPlus tool in R. Subsequently, we evaluated the ideal cluster numbers between k=2–9, and repeated the process 1,000 times to ensure stable results.
Identification of differentially expressed genes (DEGs), differentially expressed gemcitabine resistance-related genes (DEGRRGs), and survival-associated DEGRRGs
The DEGs were identified using the edgeR package in R language (http://bioconductor.org/packages/edgeR/) to further analyze the data. A |log2 fold change (FC)| >1 and false discovery rate (FDR) adjusted to a P value <0.05 were set as the thresholds (15). In addition, volcano maps and heat maps of the DEGs were produced using the gplots and heatmap packages in the edgeR package. By comparing the gemcitabine resistance-related gene lists, we obtained the DEGRRGs. The survival-associated DEGRRGs were screened out by a univariate Cox analysis, which was conducted using the R software survival package. The results were externally validated using the GSE24450 data set from the GEO database.
Development of the gemcitabine resistance-related ICD-associated prognostic index
A prognostic risk score was obtained for each patient by the univariate Cox regression analysis and least absolute shrinkage and selection operator (LASSO)-penalized Cox regression. To verify the feasibility of the prognostic model, we also divided the GSE58812 patients into 2 groups according to the median risk score. The outcome of the 2 groups was analyzed by the Kaplan-Meier curve. The risk score calculation formula for all patients was as follows:
where k represents the number of messenger RNA (mRNA), Ci represents the coefficient of mRNA in the multivariate Cox regression analysis, and Vi represents the expression level of mRNA.
Relationship between prognostic index and immune cell infiltration
The TIMER online database analyses and visualizes the abundances of tumor-infiltrating immune cells (16). TIMER was used to reanalyze gene expression data from TCGA, which included 10,897 samples across 15 cancer types, to estimate the abundance of multiple subtypes of tumor-infiltrating immune cells, including CD4+ T cells, regulatory T cells (Tregs), macrophages, and dendritic cells (DCs). TIMER can be used to determine the relationship between immune cell infiltration and other parameters. We downloaded the immune infiltrate levels of BC patients and calculated the associations between the prognostic index and immune cell infiltration.
Relationship between the prognostic model and clinical characteristics
To further understand the relationship between the immune prognostic model and other clinical data, such as age, gender, grade, American Joint Committee on Cancer (AJCC) stage, tumor (T) stage and lymph nodes (N) stage, we performed univariate and multivariate Cox analyses, which revealed that the immune prognostic model serves as an independent factor for predicting the progneosis of BC patients.
Statistical analysis
We performed a survival analysis for patients with the prognostic model using the “survival” package in R. Survival curves were generated using the Kaplan-Meier method and the log-rank test to compare the difference between the 2 groups. The area under the curve of the survival receiver operating characteristic (ROC) curve was calculated via the survival ROC R software package to validate the performance of the prognostic signature (17). P values <0.05 were considered statistically significant.
Results
The clinical features of BC patients in TCGA
The median age of the patients was 68.5 years (range, 34–89 years). In total, 398 patients were included in TCGA data set, of whom 296 were male and 102 were female. The patients were further categorized into subgroups on the basis of T stage, N stage, cancer grade, and AJCC stage. The clinical characteristics of patients involved in the present study are shown in Table 1.
The expression of ICD-related genes in BC patients
The ICD-related genes involved in our study were identified by Garg et al. (14). We analyzed the expression profiles of the BC and adjacent tissues in TCGA database, in which the expression levels of IL6, ENTPD1, P2RX7, NLRP3, TLR4, CD4, IL10, IL1R1, LY96, and NT5E were downregulated, and the expression levels of HMGB1, FOXP3, CASP8, IL17RA, PDIA3, BAX, CALR, and IFNB1 were upregulated (Figure 1A). Protein-protein interactions were used to analyze the connections between ICD-related genes in the search tool for the retrieval of interacting genes/proteins (STRING) database (Figure 1B).
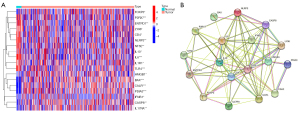
Consensus clustering was used to identify 3 ICD-associated subtypes
We determined the ICD-associated clusters of BC using consensus clustering. In TCGA cohort, 3 clusters were identified using distinct ICD genes expression patterns after k-means clustering (Figure 2A). Among them, the C1 group, which we called the ICD-high group, showed a high gene expression level, while the C2 and C3 groups, which we called the ICD-low group, showed low gene expression levels. The survival analysis showed that the prognosis of patients in the ICD-high group was significantly better than that of patients in the ICD-low group (Figure 2B).
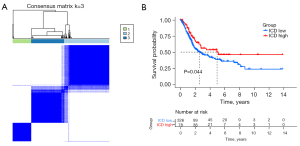
Tumor immune microenvironment evaluation of the ICD subgroups
The ICD-high group had a higher estimation of stromal and immune cells in malignant tumours using expression data (ESTIMATE) score than the ICD-low group (Figure 3A) (P<0.001). The ICD-high group had a higher immune score than the ICD-low group (Figure 3B) (P<0.001). The ICD-high group had a higher stromal score than the ICD-low group (Figure 3C) (P<0.001). The ICD-high group had lower tumor purity than the ICD-low group (Figure 3D) (P<0.001).
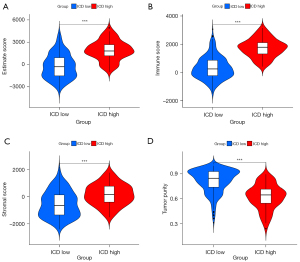
Identification of DEGRRGs
Using edgeR, we identified 2,372 DEGRRGs in the GSE80617 data set. Among these DEGRRGs, 1,784 genes were upregulated and 588 genes were downregulated with thresholds of |log2 FC| >1.0 and P<0.01 (https://cdn.amegroups.cn/static/public/tau-22-736-1.xlsx).
Identification of ICD-related DEGRRGs
We identified 3536 DEGs within the different ICD groups (i.e., the ICD-high group and the ICD-low group) using the data of BC patients obtained from TCGA database via edgeR. Among them, 2,054 genes were downregulated and 1,442 genes were upregulated with thresholds of |log2FC| >1.0 and an adjusted P<0.05. Intersection was performed in ICD-relate genes and DEGRRGs, and 131 ICD-related DEGRRGs were identified (Figure S1). The heatmap and volcano map are shown in Figure 4A and Figure 4B, respectively.
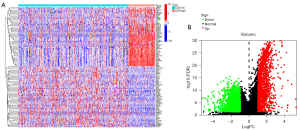
Construction of the gemcitabine-resistance prognostic model
We conducted a univariate Cox regression analysis to identify the survival-associated genes in both TCGA and GEO validation data (Table 2). A LASSO-penalized Cox regression analysis was performed to identify the genes in the prognostic model. The prognostic model was verified by a validation prognostic model based on the GSE31684 data set obtained from the GEO database. The risk score for the prognostic gene signature was calculated as follows:
Table 2
Gene | OS | ||||
---|---|---|---|---|---|
TCGA | GEO | ||||
OR (95% CI) | P value | OR (95% CI) | P value | ||
MFAP3L | 0.855 (0.758–0.965) | 0.011* | – | – | |
TBL1Y | 0.736 (0.573–0.949) | 0.018* | – | – | |
APOL6 | 0.801 (0.687–0.934) | 0.005** | – | – | |
SLAMF7 | 0.868 (0.770–0.979) | 0.021* | – | – | |
PTPRR | 0.831 (0.752–0.919) | 0.000** | 0.458 (0.249–0.842) | 0.012* | |
IL21 | 0.323 (0.135–0.769) | 0.011* | – | – | |
APOL3 | 0.859 (0.753–0.979) | 0.023* | – | – | |
HOXB3 | 0.795 (0.695–0.910) | 0.001** | 0.456 (0.246–0.846) | 0.013* | |
CAPS | 0.917 (0.848–0.992) | 0.032* | – | – | |
DHRS2 | 0.942 (0.901–0.985) | 0.009** | 0.548 (0.300–0.998) | 0.049* | |
SIGLEC15 | 0.843 (0.748–0.949) | 0.005** | 0.489 (0.265–0.901) | 0.020* | |
NNMT | 1.083 (1.005–1.172) | 0.045* | – | – | |
UNC5CL | 0.694 (0.560–0.859) | 0.001** | 0.541 (0.297–0.985) | 0.042* | |
CASQ1 | 0.864 (0.790–0.944) | 0.001** | 0.500 (0.274–0.911) | 0.022* | |
S100A7 | 1.040 (1.001–1.080) | 0.041* | |||
TNC | 1.140 (1.057–1.229) | 0.001** | |||
BTBD16 | 0.934 (0.881–0.989) | 0.020* | |||
SPHK1 | 1.164 (1.053–1.286) | 0.003** | 2.014 (1.107–3.665) | 0.022* | |
PYHIN1 | 0.793 (0.644–0.975) | 0.028* | |||
TLL1 | 1.253 (1.081–1.451) | 0.003** | |||
PCSK9 | 1.155 (1.051–1.270) | 0.003** | |||
CALB2 | 1.108 (1.019–1.205) | 0.016* |
*, P<0.05; **, P<0.01. CI, confidence interval; GEO, Gene Expression Omnibus; OR, odds ratio; OS, overall survival; TCGA, The Cancer Genome Atlas.
risk score = (expression level of PTPRR × −0.01623) + (expression level of HOXB3 × −0.00335) + (expression level of SIGLEC15 × 0.05481) + (expression level of UNC5CL × −0.04691) + (expression level of CASQ1 × 0.096733).
The survival of the 2 groups was analyzed by a Kaplan-Meier curve. In the prognostic model, the low-risk group had better overall survival (OS) than the high-risk group (P<0.001) (Figure 5A). In the GEO validation model, the low-risk group had better OS than the high-risk group (P<0.001) (Figure 5B). In relation to the prognostic model, the expression of genes for each patient is shown in Figure 5C. In relation to the GEO validation model, the expression of genes for each patient is shown in Figure 5D. The distribution of different survival statuses (in terms of years) of the prognostic model is shown in Figure 5E. The distribution of different survival statuses (in terms of years) of the GEO validation model is shown in Figure 5F. The distribution of different risk scores of the prognostic model is shown in Figure 5G. In the GEO validation model, the distribution of different risk scores is shown in Figure 5H.
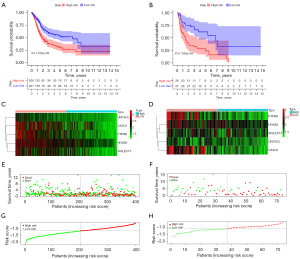
Verify the accuracy of the prognostic model
Prediction accuracy was compared among clinical features and riskscore. The ROC curve analysis of the prognostic model and validation model were showed in Figure 6. According to the ROC curves, the risk score, age, gender, AJCC stage, T stage and N stage AUC of the TCGA prognostic model were calculated to be 0.705, 0.661, 0.465, 0.605, 0.611 and 0.597, respectively (Figure 6A). According to the ROC curves, the risk score, age, T stage and N stage AUC of the GEO validation model were calculated to be 0.716, 0.640, 0.597 and 0.639, respectively (Figure 6B).
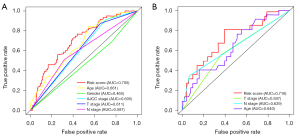
The clinical features of the prognostic model
In TCGA prognostic model, the univariate Cox regression analyses (Table 3) showed that an older age (>58 years) [hazard ratio (HR) 1.555; 95% confidence interval (CI): 1.125–2.148; P=0.007], a high AJCC stage (III–IV) (HR: 2.349; 95% CI: 1.480–3.729; P<0.001), a high T stage (3 to 4) (HR: 2.019; 95% CI: 1.344–3.032; P=0.001), lymph node metastasis (positive) (HR: 1.543; 95% CI: 0.767–2.794; P=0.089), high tumor differentiation (HR: 0.227; 95% CI: 0.032–1.630; P=0.140), and a high risk score (HR: 1.824; 95% CI: 1.306–2.548; P<0.001) were significant risk factors for a poor prognosis. The multivariate Cox regression analysis (Table 3) showed that a high-risk score (HR: 1.679; 95% CI: 1.198–2.354; P=0.003) was an independent risk factor for a poor prognosis. In the prognostic model, a high T stage, the AJCC stage, higher grade, and being female were correlated with higher risk scores (Figure 7).
Table 3
Clinical feature | OS | ||||
---|---|---|---|---|---|
Univariate | Multivariate | ||||
OR (95% CI) | P value | OR (95% CI) | P value | ||
Age | 1.555 (1.125–2.148) | 0.007** | 1.533 (1.109–2.121) | 0.010* | |
Grade | 0.227 (0.032–1.630) | 0.140 | – | – | |
T stage | 2.019 (1.344–3.032) | 0.001** | 1.232 (0.634–2.395) | 0.538 | |
N stage | 1.543 (0.767–2.794) | 0.089 | – | – | |
AJCC stage | 2.349 (1.480–3.729) | <0.001** | 1.791 (0.838–3.826) | 0.132 | |
Risk score | 1.824 (1.306–2.548) | <0.001** | 1.679 (1.198–2.354) | 0.003** |
*, P<0.05; **, P<0.01. AJCC, American Joint Committee on Cancer; CI, confidence interval; N stage, lymph nodes stage; OR, odds ratio; OS, overall survival; T, tumor stage; TCGA, The Cancer Genome Atlas.
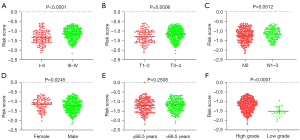
Immune cell infiltration correlation analysis of risk score
Patients in the high-risk group had a significantly higher immune score, stromal score, and infiltration of M0 macrophages, M1 macrophages, M2 macrophages, and activated CD4+ T cells than those in the low-risk group (Figure 8). Patients in the high-risk group had significantly lower infiltration of Tregs, resting DCs, and activated DCs than those in the low-risk group (Figure 8).
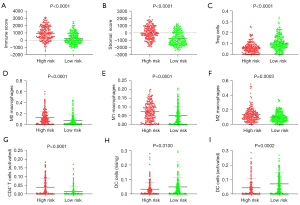
Discussion
Tumor cell death can be categorized into immunogenic or non-immunogenic death according to external stimuli. ICD is generally referred to as cell death triggered by anti-tumor therapeutics, such as chemotherapy and radiotherapy (18,19), and is recognized as a potential predictor of anti-tumor immunity (20,21). Recent studies have conducted in-depth investigations to explore the mechanism and find solutions for overcoming the chemoresistance of gemcitabine in BC. The present study established a prognostic model based on ICD-related genes for outcome prediction, and expanded the approaches for overcoming gemcitabine resistance in BC.
ICD is characterized by the release of a series of DAMPs, such as HMGB1, IFN-1, ANXA1 and surface-exposed CALR (22). The present study identified a series of survival-associated ICD-related genes, including HMGB1. A previous meta-analysis revealed that the upregulation of HMGB1 was unfavorably correlated with the outcomes of various types of malignant tumors, including BC (23). Patients were further classified into 3 clusters according to the expression level of the ICD-related genes by consensus clustering. The survival analysis indicated that patients in the ICD-high group had better outcomes than those in the ICD-low group, which is consistent with the conclusions drawn in previous research.
Gemcitabine is a classic anti-tumor drug for adjuvant chemotherapy after radical surgery and palliative chemotherapy in patients with recurrent and metastatic BC. However, in the treatment of early and late stage patients, the therapeutic effect decreases sharply, and patients gradually develop chemoresistance to gemcitabine, which leads to treatment failure. Previous research has shown that ICD plays an important role in the anti-tumor activity of oncolytic parvovirus H-1 in combination with gemcitabine in pancreatic cancer (24). Additionally, Zhao et al. reported that S-2-amino-3-[4V-N,N,-bis(2-chloroethyl) amino]-phenyl propionic acid N-oxide dihydrochloride) (PX-478), a HIF-α inhibitor, could enhance the anti-tumor effect of gemcitabine by inducing ICD in pancreatic cancer cells (25).
To the best of our knowledge, no previous research has focused on the correlation between gemcitabine-resistance and ICD in BC. Given the correlation between the anti-tumor properties of gemcitabine and ICD, an intersection analysis of the gemcitabine-related genes and the above-mentioned ICD-related DEGs was performed and 131 gemcitabine resistance-related ICD-associated DEGs were identified in the present study.
PTPRR is an inhibitor of the mitogen-activated protein kinase signaling pathway (26). Previous research has revealed a correlation between the expression level of PTPRR and multiple malignant tumors, such as pancreatic cancer (27) and ovarian cancer (28). Previous research has also reported that silencing of PTPRR facilitates metastasis in cervical cancer (29).
HOXB3, which is a member of the HOXB family, plays a crucial role in the invasion and recurrence in various malignant tumors (30-32). For example, a lower expression level of HOXB3 is correlated with worse outcomes in breast cancer patients, and further pathway enrichment analysis revealed that HOXB3 is negatively correlated with malignant proliferation, invasion and metastasis (33). Similarly, Yan et al. showed that the upregulation of HOXB3 is independently correlated with unsatisfactory outcomes in lung cancer. Additionally, a functional analysis revealed that HOXB3 is positively correlated with the alleviation of apoptosis, and the proliferation and migration of lung cancer cells (34).
SIGLEC15, which is a member of the SIGLEC family, plays a crucial role in the regulation of immune cell function and attenuates the innate immune response against malignant cells, and is upregulated in various cancers (35,36). A meta-analysis reported positive or negative correlations between the expression level of SIGLEC15 and tumor outcomes depending on the cancer subtypes (37). Additionally, SIGLEC15 is complementary to programmed cell death ligand 1 and recognized as a promising target for cancer immunotherapy (38). A recent study showed that the upregulation of SIGLEC15 was linked to the lower density of tumor infiltration lymphocytes, and a worse response to chemotherapy and immunotherapy in BC (36). Thus, SIGLEC15 may be a prospective therapeutic target for immunotherapy and outcome prediction in BC.
CASQ1, which is a type of Ca2+ binding protein in the skeletal muscle (39), has rarely been studied in malignant tumors. However, the overexpression of CASQ2 was shown to increase tumorigenesis and metastasis in breast cancer (40).
UNC5CL promotes peptidase activity via the positive regulation of the I-κB/NF-κB signaling pathway (41). To the best of our knowledge, the present study was the first to recognize UNC5CL as a prognostic indicator in BC.
ICD is usually accompanied by anti-tumor immunity, which is induced by the release of a series of DAMPs from dying malignant cells (42). The relationship between the survival-associated gemcitabine resistance-related ICD DEGs and immune cell infiltration was examined by the TIMER online database. A high-risk score was correlated with a higher immune score and stromal score, and the infiltration of activated CD4+ T cells, M0 macrophages, M1 macrophages, and M2macrophages. Conversely, a high risk score was correlated with a lower infiltration of Tregs, resting DCs, and activated DCs.
Conclusions
To the best of our knowledge, this study was the first to identify a series of gemcitabine resistance-related ICD-associated DEGs, establish a prognostic model for prognostic assessment, and explore promising targets for overcome gemcitabine-resistance in BC. The specific mechanisms of gemcitabine-resistance will be further explored in future research.
Limitations
The present study had several limitations. It was based on data obtained from TCGA and GEO database, and more data sets from multi-centers are required for further analyses. Additionally, given the lack of research focused on the key ICD-related gemcitabine-resistance genes identified in the present study, further integrated analyses need to be conducted to reveal their specific biological mechanisms in BC.
Acknowledgments
We thank TCGA database (https://portal.gdc.cancer.gov/) and the GEO database (www.ncbi.nlm.nih.gov/geo/) for data acquisition.
Funding: The study was supported by the Science and Education for Health Foundation of Suzhou for Youth (grant No. KJXW2021033), the Foundation of Six one-good's Project for High-level talents (grant No. LGY2020044), and the Foundation of Gusu institute of Nanjing Medical University (grant No. GSKY20210222).
Footnote
Reporting Checklist: The authors have completed the TRIPOD reporting checklist Available at https://tau.amegroups.com/article/view/10.21037/tau-22-736/rc
Conflicts of Interest: All authors have completed the ICMJE uniform disclosure form (available at https://tau.amegroups.com/article/view/10.21037/tau-22-736/coif). All the authors report funding from the Science and Education for Health Foundation of Suzhou for Youth (grant No. KJXW2021033), the Foundation of Six one-good's Project for High-level talents (grant No. LGY2020044), and the Foundation of Gusu institute of Nanjing Medical University (grant No. GSKY20210222). The authors have no other conflicts of interest to declare.
Ethical Statement:
Open Access Statement: This is an Open Access article distributed in accordance with the Creative Commons Attribution-NonCommercial-NoDerivs 4.0 International License (CC BY-NC-ND 4.0), which permits the non-commercial replication and distribution of the article with the strict proviso that no changes or edits are made and the original work is properly cited (including links to both the formal publication through the relevant DOI and the license). See: https://creativecommons.org/licenses/by-nc-nd/4.0/.
References
- Dobruch J, Oszczudłowski M. Bladder Cancer: Current Challenges and Future Directions. Medicina (Kaunas) 2021;57:749. [Crossref] [PubMed]
- Patel VG, Oh WK, Galsky MD. Treatment of muscle-invasive and advanced bladder cancer in 2020. CA Cancer J Clin 2020;70:404-23. [Crossref] [PubMed]
- Richters A, Aben KKH, Kiemeney LALM. The global burden of urinary bladder cancer: an update. World J Urol 2020;38:1895-904. [Crossref] [PubMed]
- Li HZ, Zheng RS, Du LB, et al. Bladder cancer incidence, mortality and temporal trends in China. Chinese Journal of Oncology 2021;43:293-8. [PubMed]
- Ro JY, Staerkel GA, Ayala AG. Cytologic and histologic features of superficial bladder cancer. Urol Clin North Am 1992;19:435-53. [Crossref] [PubMed]
- Saxman SB, Propert KJ, Einhorn LH, et al. Long-term follow-up of a phase III intergroup study of cisplatin alone or in combination with methotrexate, vinblastine, and doxorubicin in patients with metastatic urothelial carcinoma: a cooperative group study. J Clin Oncol 1997;15:2564-9. [Crossref] [PubMed]
- Hurle R, Contieri R, Casale P, et al. Midterm follow-up (3 years) confirms and extends short-term results of intravesical gemcitabine as bladder-preserving treatment for non-muscle-invasive bladder cancer after BCG failure. Urol Oncol 2021;39:195.e7-195.e13. [Crossref] [PubMed]
- Lu JL, Xia QD, Lu YH, et al. Efficacy of intravesical therapies on the prevention of recurrence and progression of non-muscle-invasive bladder cancer: A systematic review and network meta-analysis. Cancer Med 2020;9:7800-9. [Crossref] [PubMed]
- Vedder MM, Márquez M, de Bekker-Grob EW, et al. Risk prediction scores for recurrence and progression of non-muscle invasive bladder cancer: an international validation in primary tumours. PLoS One 2014;9:e96849. [Crossref] [PubMed]
- Legrand AJ, Konstantinou M, Goode EF, et al. The Diversification of Cell Death and Immunity: Memento Mori. Mol Cell 2019;76:232-42. [Crossref] [PubMed]
- Kono K, Mimura K, Kiessling R. Immunogenic tumor cell death induced by chemoradiotherapy: molecular mechanisms and a clinical translation. Cell Death Dis 2013;4:e688. [Crossref] [PubMed]
- Ye W, Gunti S, Allen CT, et al. ASTX660, an antagonist of cIAP1/2 and XIAP, increases antigen processing machinery and can enhance radiation-induced immunogenic cell death in preclinical models of head and neck cancer. Oncoimmunology 2020;9:1710398. [Crossref] [PubMed]
- Galluzzi L, Humeau J, Buqué A, et al. Immunostimulation with chemotherapy in the era of immune checkpoint inhibitors. Nat Rev Clin Oncol 2020;17:725-41. [Crossref] [PubMed]
- Garg AD, De Ruysscher D, Agostinis P. Immunological metagene signatures derived from immunogenic cancer cell death associate with improved survival of patients with lung, breast or ovarian malignancies: A large-scale meta-analysis. Oncoimmunology 2015;5:e1069938. [Crossref] [PubMed]
- Robinson MD, McCarthy DJ, Smyth GK. edgeR: a Bioconductor package for differential expression analysis of digital gene expression data. Bioinformatics 2010;26:139-40. [Crossref] [PubMed]
- Li T, Fan J, Wang B, et al. TIMER: A Web Server for Comprehensive Analysis of Tumor-Infiltrating Immune Cells. Cancer Res 2017;77:e108-10. [Crossref] [PubMed]
- Heagerty PJ, Lumley T, Pepe MS. Time-dependent ROC curves for censored survival data and a diagnostic marker. Biometrics 2000;56:337-44. [Crossref] [PubMed]
- Kroemer G, Galluzzi L, Kepp O, et al. Immunogenic cell death in cancer therapy. Annu Rev Immunol 2013;31:51-72. [Crossref] [PubMed]
- Ahmed A, Tait SWG. Targeting immunogenic cell death in cancer. Mol Oncol 2020;14:2994-3006. [Crossref] [PubMed]
- Garg AD, Martin S, Golab J, et al. Danger signalling during cancer cell death: origins, plasticity and regulation. Cell Death Differ 2014;21:26-38. [Crossref] [PubMed]
- Krysko DV, Garg AD, Kaczmarek A, et al. Immunogenic cell death and DAMPs in cancer therapy. Nat Rev Cancer 2012;12:860-75. [Crossref] [PubMed]
- Fucikova J, Kepp O, Kasikova L, et al. Detection of immunogenic cell death and its relevance for cancer therapy. Cell Death Dis 2020;11:1013. [Crossref] [PubMed]
- Wu T, Zhang W, Yang G, et al. HMGB1 overexpression as a prognostic factor for survival in cancer: a meta-analysis and systematic review. Oncotarget 2016;7:50417-27. [Crossref] [PubMed]
- Angelova AL, Grekova SP, Heller A, et al. Complementary induction of immunogenic cell death by oncolytic parvovirus H-1PV and gemcitabine in pancreatic cancer. J Virol 2014;88:5263-76. [Crossref] [PubMed]
- Zhao T, Ren H, Jia L, et al. Inhibition of HIF-1α by PX-478 enhances the anti-tumor effect of gemcitabine by inducing immunogenic cell death in pancreatic ductal adenocarcinoma. Oncotarget 2015;6:2250-62. [Crossref] [PubMed]
- Lin G, Aranda V, Muthuswamy SK, et al. Identification of PTPN23 as a novel regulator of cell invasion in mammary epithelial cells from a loss-of-function screen of the 'PTP-ome'. Genes Dev 2011;25:1412-25. [Crossref] [PubMed]
- Luo L, Li Y, Huang C, et al. A new 7-gene survival score assay for pancreatic cancer patient prognosis prediction. Am J Cancer Res 2021;11:495-512. [PubMed]
- Wang Y, Cao J, Liu W, et al. Protein tyrosine phosphatase receptor type R (PTPRR) antagonizes the Wnt signaling pathway in ovarian cancer by dephosphorylating and inactivating β-catenin. J Biol Chem 2019;294:18306-23. [Crossref] [PubMed]
- Su PH, Lin YW, Huang RL, et al. Epigenetic silencing of PTPRR activates MAPK signaling, promotes metastasis and serves as a biomarker of invasive cervical cancer. Oncogene 2013;32:15-26. [Crossref] [PubMed]
- Yang D, Yan R, Zhang X, et al. Deregulation of MicroRNA-375 inhibits cancer proliferation migration and chemosensitivity in pancreatic cancer through the association of HOXB3. Am J Transl Res 2016;8:1551-9. [PubMed]
- Bi L, Zhou B, Li H, et al. A novel miR-375-HOXB3-CDCA3/DNMT3B regulatory circuitry contributes to leukemogenesis in acute myeloid leukemia. BMC Cancer 2018;18:182. [Crossref] [PubMed]
- Miller KR, Patel JN, Zhang Q, et al. HOXA4/HOXB3 gene expression signature as a biomarker of recurrence in patients with high-grade serous ovarian cancer following primary cytoreductive surgery and first-line adjuvant chemotherapy. Gynecol Oncol 2018;149:155-62. [Crossref] [PubMed]
- Zhu L, Yu S, Jiang S, et al. Loss of HOXB3 correlates with the development of hormone receptor negative breast cancer. PeerJ 2020;8:e10421. [Crossref] [PubMed]
- Yan M, Yin X, Zhang L, et al. High expression of HOXB3 predicts poor prognosis and correlates with tumor immunity in lung adenocarcinoma. Mol Biol Rep 2022;49:2607-18. [Crossref] [PubMed]
- Macauley MS, Crocker PR, Paulson JC. Siglec-mediated regulation of immune cell function in disease. Nat Rev Immunol 2014;14:653-66. [Crossref] [PubMed]
- Hu J, Yu A, Othmane B, et al. Siglec15 shapes a non-inflamed tumor microenvironment and predicts the molecular subtype in bladder cancer. Theranostics 2021;11:3089-108. [Crossref] [PubMed]
- Li B, Zhang B, Wang X, et al. Expression signature, prognosis value, and immune characteristics of Siglec-15 identified by pan-cancer analysis. Oncoimmunology 2020;9:1807291. [Crossref] [PubMed]
- Wang J, Sun J, Liu LN, et al. Siglec-15 as an immune suppressor and potential target for normalization cancer immunotherapy. Nat Med 2019;25:656-66. [Crossref] [PubMed]
- Dainese M, Quarta M, Lyfenko AD, et al. Anesthetic- and heat-induced sudden death in calsequestrin-1-knockout mice. FASEB J 2009;23:1710-20. [Crossref] [PubMed]
- Kim JH, Lee ES, Yun J, et al. Calsequestrin 2 overexpression in breast cancer increases tumorigenesis and metastasis by modulating the tumor microenvironment. Mol Oncol 2022;16:466-84. [Crossref] [PubMed]
- Harmonizing model organism data in the Alliance of Genome Resources. Genetics 2022; [PubMed]
- Scarpitta A, Hacker UT, Büning H, et al. Pyroptotic and Necroptotic Cell Death in the Tumor Microenvironment and Their Potential to Stimulate Anti-Tumor Immune Responses. Front Oncol 2021;11:731598. [Crossref] [PubMed]