EZH2-regulated immune risk score prognostic model predicts outcome of clear cell renal cell carcinoma
Highlight box
Key findings
• EZH2 regulated immune-risk score prognostic model was an independent prognostic factor, accuracy and good predictability in ccRCC. Our research also indicated immune-risk score prognostic model may guide EZH2-targeted therapies for ccRCC patients.
What is known and what is new?
• High EZH2 expression always leads to less CD8+ T cell infiltration. However, ccRCC were “hot” tumor as reported, it is contradictory with high EZH2 expression in ccRCC;
• EZH2 regulated-immune risk score prognostic model to predict ccRCC patient outcome.
What is the implication, and what should change now?
• Assessment of the EZH2 effects on TEM will help inform the best utilization of EZH2 inhibitors in the clinic.
Introduction
According to the 2022 cancer statistics, there were ≈431,288 new cases of kidney and renal pelvis cancer in 2020, and 179,368 estimated deaths (The Global Cancer Observatory). Approximately 85–90% of renal cell carcinoma (RCC) cases are the clear cell type (ccRCC) (1), and the prognosis of metastatic ccRCC is poor, with a 5-year survival rate <10% after diagnosis (2).
Enhancer of zeste homolog 2 (EZH2) has pleiotropic functions in both tumor and tumor microenvironment (TME) immune cells (3). In most cancer types, EZH2 has an important immunoediting function, leading to an immunosuppressive TME through a variety of mechanisms: (I) tumor-associated antigens or neoantigens loss of expression (4); (II) immunosuppressive molecules and changing the expression of proinflammatory cytokines (5); (III) aberrant expression of checkpoint pathway proteins [e.g., programmed cell death 1 ligand 1 (PD-L1)] (6). EZH2 is overexpressed in endometrial cancer, small-cell lung cancer, prostate cancer, breast cancer and melanoma. To date, EZH2 inhibitors have been evaluated in clinical trials targeting EZH2-driven epigenetic alterations alone or in combination with an immune checkpoint antibody, such as CPI-1205, CPI-0209, and DS-3201 (3,4,7). It is important to know if EZH2 inhibition will overcome its potential immunosuppressing effect in most cancers.
Sun et al. reported that EZH2 is highly expressed in BAP1-mutant ccRCC and is related to poor prognosis (8). Intrinsic or acquired resistance to receptor tyrosine kinase inhibitors is a major problem in ccRCC clinical treatment (9). Adelaiye-Ogala et al. reported that EZH2 is a rational target for therapeutic intervention in sunitinib-resistant ccRCC (10). Furthermore, Eichenauer et al. (11) investigated the relationship between EZH2 expression and the density of CD8+ T cells in 1,800 RCC patients’ tumor tissues. They found that the density of CD8+ T cells continuously increased with raising EZH2 levels. EZH2 was reported to decrease CD8+ T cells infiltration though secretion of CXCL9/CXCL10 (6). There are contradictory phenomena (high EZH2 expression and “hot” TME) in ccRCC.
In this study based on the multiple immune effects of EZH2 on TME-forming immune cells, we aimed to establish a EZH2-regulated immunomodulators risk score prognostic model that could be applied to ccRCC diagnosis and treatment. In addition, this study demonstrated that the prognostic signature might show indispensable implications on modulating the TME and directing immunotherapy intervention in ccRCC. Pertaining to the limited data obtained from the TCGA database, these findings need to be further corroborated in a larger cohort or in cytological experiments. We present the following article in accordance with the TRIPOD reporting checklist (available at https://tau.amegroups.com/article/view/10.21037/tau-22-817/rc).
Methods
Datasets and samples
We downloaded data for 611 ccRCC patients from The Cancer Genome Atlas (TCGA), including transcriptome [count and fragments per kilobase of exon model per million mapped fragments (FPKM) value] and clinical information, and the 611 ccRCC patients’ clinical information including: survival time, TNM, stage, age, and gender. This study was conducted in accordance with the Declaration of Helsinki (as revised in 2013).
Tumor-infiltrating lymphocytes (TILs)
The immune-related signatures of 28 TILs [activated B cell; activated CD4+ T cell; activated CD8+ T cell; central memory CD4+ T cell; central memory CD8+ T cell; effector memory CD4+ T cell; effector memory CD8+ T cell; gamma delta T cell; immature B cell; memory B cell; regulatory T cell; T follicular helper cell; type 1 T helper cell; type 17 T helper cell; type 2 T helper cell; activated dendritic cell; CD56bright natural killer (NK) cell; CD56dim NK cell; eosinophil; immature dendritic cell; macrophage; mast cell; monocyte; myeloid-derived suppressor cell; NK cell; NK T cell; neutrophil; plasmacytoid dendritic cell] were analyzed in TISIDB website (12). The 28 TILs, gene expression, copy number, methylation, and mutation were viewed and downloaded. For each cancer type, the relative abundance of TILs was inferred from gene set variation analysis based on the gene expression profile.
Identification of EZH2-related immunomodulators
The relationship between two types of immunomodulator (immunoinhibitors and immunostimulators) and EZH2 expression was viewed and downloaded in TISIDB web site (12). The co-expression of immunoinhibitors and immunostimulators was analyzed with EZH2, and only immunoinhibitor or immunostimulator genes with a P value <±0.05 were regarded as EZH2-related immunogenes.
Enrichment analysis and protein-protein interaction (PPI) network of EZH2-related immune genes
WebGestalt (WEB-based Gene SeT AnaLysis Toolkit, http://www.webgestalt.org/) was utilized to generate a Kyoto Encyclopedia of Genes and Genomes (KEGG) pathway of EZH2-related immune genes. PPI networks were generated on the STRING database (online), and the interactive source of minimum interaction score was set as 0.4.
Heatmap
We installed R packages: “e1071”, “limma”, “BiocManager”, and “preprocessCore” to prepare CIBERSORT files. We used the R packages “pheatmap” and “vioplot”, with P value <±0.05, to construct an immune cells heatmap in ccRCC.
Overall survival (OS)
Variance stabilizing transformation was used to normalize the 611 ccRCC patients, and then low-value genes were removed using heterogeneity analysis. According to EZH2 gene expression, Low and High groups were set up. Next, “BiocManager”, “survival”, and “survminer” packages were installed in R and used to calculate gene survival analysis.
Prognostic gene among EZH2-related immunogenes
EZH2-related immunogenes, and TCGA ccRCC clinical information were matched and merged using R package “limma”. P value <±0.05 was considered as a prognostic immune-related gene.
Establishment of risk score prognostic model
A total of 26 related genes were analyzed to construct a predictive risk score prognostic model, using “glmnet”, “survival”, and “survminer” in a multivariable Cox model. According to the risk score prognostic model, ccRCC patients get a risk score, and divided into a high-risk group (risk score above the median value) and low-risk group (risk score below the median value) in R software. Furthermore, both groups were tested for OS, and prognostic model gene expression. Software package “survival ROC” was applied and the risk model prognostic ability was calculated by receiver operating characteristic (ROC) and area under the curve (AUC) analysis in R studio.
Statistical analysis
In this study, R software (version 4.0.3) was used for statistical analysis and visualization of results. Univariate and multivariate Cox regression analyses were applied for variable survival and OS. P<0.05 was considered as statistically significant.
Results
High-expression EZH2 not related to “Cool” TME in ccRCC
To understand the complexity and diversity of the role of EZH2 in the TME, we analyzed the relationships between the abundance of 28 TILs and EZH2 expression, copy number, and promoter methylation. As shown in Figure 1A, high mRNA EZH2 expression was negatively associated with almost all 28 TILs in various cancers (green arrowheads, blue small square in Figure 1A represents negative relationship), but not in thyroid carcinoma or ccRCC (indicated by arrowheads in Figure 1A,1B). In this study, we focused on ccRCC. We observed that immunostimulatory TILs (active CD8+ T cells, P<0.05; effector CD8+ T cells, P<0.05; active CD4+ T cells, P<0.05) were all significantly positively correlated with high EZH2 expression. The increased number of immunostimulatory TILs indicated that EZH2 is a positive prognostic immunotherapy biomarker in ccRCC. We also observed immunosuppressive TILs: T regulatory cell (Treg) infiltration increased with high EZH2 expression, which indicated that EZH2 is also a negative immunotherapy biomarker in ccRCC. As can be seen in Figure 1B,1C, contradictory immunostimulatory and immunosuppressive TILs are observed with EZH2 copy number and promoter methylation alteration in ccRCC (green arrowheads, blue small square in Figure 1C represents negative relationship).
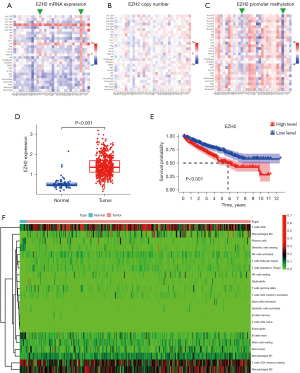
We further explored EZH2 expression in tumor tissue and the effect on ccRCC OS. EZH2 mRNA expression was significantly increased in tumor tissues compared with normal tissues (Figure 1D), and was positively correlated with survival outcomes (Figure 1E, P<0.05). Using the CIBERSOFT algorithm, we estimated the infiltration proportion of 22 immune cell types from TCGA kidney renal clear cell carcinoma (KIRC) samples, divided into normal and tumor groups according to the clinical information. As shown in Figure 1F, CD8+ T cells exhibited significantly higher infiltration levels in the tumor groups. In addition, we also observed that “T cells CD4 memory resting” were highly infiltrated in ccRCC tumors, which indicated ccRCC is not a “cool” tumor, but rather a “hot” tumor as reported.
Figure 1 shows that EZH2 was highly expressed and related to poor outcome. However, high-expression EZH2 is not related to a “cool” TME in ccRCC. It is urgent to investigate the complex effect of EZH2 on immunostimulatory and immunosuppressive TILs in ccRCC.
Immunostimulatory and immunosuppressive genes regulated by EZH2
To verify the complex effect of EZH2 on the immune TME of ccRCC, we further investigated the relationship between immunomodulators and EZH2 expression. As shown in Figure 2A, unlike other tumors, high EZH2 expression was positively correlated with most immunomodulators in ccRCC and thyroid carcinoma (purple arrowheads, red small square in Figure 2A represents positive relationship). We found immunostimulators such as lymphocyte activation gene 3 (LAG3), cytotoxic lymphocyte antigen 4 (CTLA4), CD96 (T-cell surface protein tactile, CD96 molecule), and T cell immunoreceptor with Ig and ITIM domains (TIGIT) were positively correlated with EZH2 expression. In Figure 2B, high EZH2 expression was also positively correlated with most immunosuppressors in ccRCC (purple arrowheads, red small square in Figure 2A represents positive relationship), such as: CD80, inducible T cell costimulator (ICOS), and TNF receptor superfamily member 9 (TNFRSF9). In Figure 2A,2B, it can be seen that 49 immunomodulator genes were significantly regulated by EZH2 (P>0.05 or P<0.05), 49 immunomodulator genes: BTLA, CD96, CD160, CD244, CD274, CSF1R, CTLA4, HAVCR2, IL10, IL10RB, KDR, LAG3, LGALS9, PDCD1, PDCD1LG2, TIGIT, VTCN1, C10orf54, CD27, CD28, CD40LG, CD48, CD70, CD80, CD86, CXCR4, PVRL2, ICOS, IL2RA, IL6, KLRC1, KLRK1, LTA, MICB, RAET1E, TNFRSF4, TNFRSF8, TNFRSF9, TNFRSF17, TNFRSF14, TNFRSF18, TNFRSF25, TNFSF4, TNFSF9, TNFSF13, TNFSF14, TNFSF13B, TNFSF15, ULBP1. We submitted these 49 immunomodulators to WebGestalt for KEGG pathway enrichment and as shown in Figure 2C, intestinal immune network for IgA, T-cell receptor signaling pathway, and cytokine-cytokine receptor interaction were all influenced by these immunomodulators. Figure 2C shows the complex system immune effect, underlining the urgent need to construct a precise predictive immune risk score prognostic model for ccRCC.
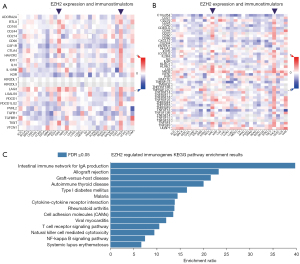
Establishment of EZH2 immune risk score prognostic model
To explore the prognostic ability of EZH2-regulated immunomodulators, we analyzed the expression of the 49 immunomodulators and clinical information in TCGA ccRCC datasets. A forest plot showed 26 immunomodulator signatures independently associated with OS (Figure 3A): 5 low-risk genes and 21 high-risk genes (CTLA4, HAVCR2, IL10RB, KDR, LAG3, LGALS9, PDCD1, TIGIT, CD80, IL2RA, IL6, KLRK1, LTA, MICB, RAET1E, TNFRSF8, TNFRSF9, TNFRSF17, TNFRSF18, TNFRSF25, TNFSF4, TNFSF13, TNFSF14, TNFSF13B, TNFSF15, ULBP1). Next, based on the expression levels of the immunomodulators and their coefficients derived from the multivariable Cox model, we established an immune risk score prognostic model (Figure 3B), The computer risk score model = (HAVCR2 × −0.1057) + (IL10RB ×0.4372) + (KDR × −0.2385) + (TNFRSF25 ×0.2402) + (TNFSF13 × −0.1671) + (TNFSF14 ×0.1777) + (ULBP1 ×0.6268) + (TNFSF4 ×0.3271).
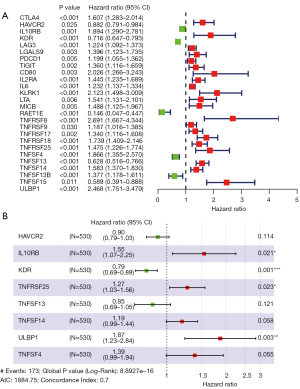
Next, to validate the immune risk score prognostic model, the high- and low-risk subgroups of ccRCC patients were evaluated by the risk score model. The OS results showed the low-risk group Kaplan-Meier OA curve was significantly higher than for the high-risk group (Figure 4A, P<0.001). According to the immune risk score, most of the deceased patients had been in the high immune risk score area (Figure 4B). The high-risk group patients had increasing risk score and death rate (Figure 4C). A heatmap showed the expression of 8 genes in both risk group patients (Figure 4D).
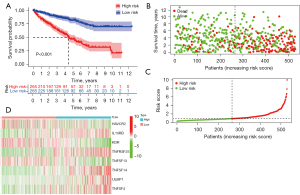
Based on the findings shown in Figures 3,4, the EZH2-regulated immune-risk score prognostic model was able to predict the OS of ccRCC patients. However, the question is whether the EZH2-regulated immune-risk score prognostic model is an independent predictor of ccRCC OS?
Assessment of the independence of the EZH2-regulated immune-risk score prognostic model
We conducted univariate and multivariate Cox regression analyses to evaluate whether our model can be used as an independent prognostic factor. As shown in Figure 5A,5B, immune risk score, age, grade, and stage were all significantly associated with OS in ccRCC. In both analyses the EZH2-regulated immune-risk score prognostic model correlated significantly with poor OS [hazard ratio (HR) =1.268, P<0.001; HR =1.142, P<0.001, respectively]. Furthermore, multivariate Cox regression analysis showed that age and clinicopathologic stage correlated with worse OS (HR =1.031, P<0.001; HR =1.608, P<0.001, respectively). Therefore, the EZH2-regulated immune-risk score prognostic model could be an independent prognostic factor of OS when adjusted by these variables.
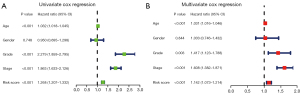
Immune risk score prognostic model with clinicopathologic parameters to predict survival rate in ccRCC
To validate clinical diagnostic value of the immune risk score prognostic model with/without clinicopathologic parameters, ROC curves were obtained. As showed in Figure 6A, immune risk score prognostic model, grade, and stage were all had a ROC area. The bigger of the value of ROC area, the better of the clinical diagnostic value. The AUC of our immune risk score prognostic model was 0.708, especially, the immune risk score prognostic model plus clinicopathologic parameters (AUC =0.816). Next, the immune risk score prognostic model prediction and actual outcome in ccRCC were calculated. Figure 6B shows that the 5-year OS prediction fitted well in TCGA cohort validation, indicating the immune risk score prognostic model provided accuracy and good predictability. Figure 6C shows the nomogram combining meaningful clinical characteristics and immune risk score prognostic model. Figure 6 indicates more reliable predictive ability of our immune risk score prognostic model.
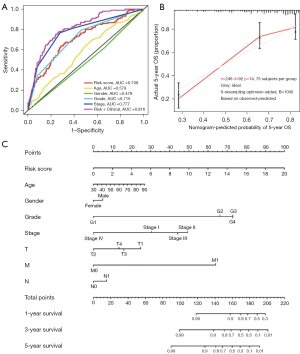
Discussion
In 2019, the US Food and Drug Administration (FDA) approved programmed cell death 1 (PD-1)/PD-L1 inhibitors, or PD-1/PD-L1 inhibitors combined with targeted drug therapy, to replace targeted drugs as first-line treatment of intermediate- and high-risk metastatic renal cancer (9,13-15). In nearly 2,000 kidney cancer patients in 151 countries, the objective response rate and progression-free survival was significantly better in the PD-1/PD-L1 inhibitor combined targeted drug therapy patient group, with respect to the targeted drug therapy patient group (16-20). However, up to 70% of renal cancer patient still do not respond to PD-1/PD-L1 inhibitors (21,22).
Substantial data have confirmed that EZH2 is involved in promoting metastasis, invasiveness (23,24), and maintenance of malignant phenotype (3,23,25). It influences key aspects of the TME, protects tumor cells from identification and elimination, and leads to tumor escape and immune resistance (3). For example, CD8+ T cells play a key role in antitumor immunity, and EZH2 downregulates the chemokines (CCL28, CCL3L1, CXCL16, CXCL9, CXCL10) that inhibit CD8+ T cell infiltration (6). EZH2 inhibitors could lead to increased Treg trafficking and impaired Treg capacity (4,5). It has been demonstrated that EZH2 stabilizes the functional phenotype of activated Tregs through CD28 co-stimulatory receptor (4). In mouse MC38 tumor models, breast cancer, and human colorectal cancer, EZH2 expression and associated H3K27me3 marking of tumor-infiltrating Tregs has been confirmed (4,7). NK cells are core cells of the natural immune system (4,7). EZH2 depresses NK cell development and cytolytic activity by regulating NKG2D and GzmB expression (4,7). In view of these findings, studies suggest that targeting EZH2 expression could enhance antitumor immunity (3,6,11).
In ccRCC, the positive expression rate of EZH2 is as high as 80%, and the expression level of EZH2 in metastatic renal cancer is higher than in localized renal cancer (8,26). Furthermore, EZH2 positively correlates with ccRCC stage, grade and lymph node metastasis, and negatively correlates with patient prognosis (24,27). In our study, contradictory immunostimulatory and immunosuppressive TILs were observed in EZH2 copy number and promoter methylation alteration in ccRCC. We also observed that CD8+ T cells and “T cells CD4 memory resting” exhibited significantly higher infiltration levels in tumor groups compared with normal groups, and high levels of infiltration of NK cells and Tregs were observed in ccRCC tumor groups, confirming that ccRCC is a “hot” tumor.
We found 26 EZH2 significantly regulated immune genes (CTLA4, HAVCR2, IL10RB, KDR, LAG3, LGALS9, PDCD1, TIGIT, CD80, IL2RA, IL6, KLRK1, LTA, MICB, RAET1E, TNFRSF8, TNFRSF9, TNFRSF17, TNFRSF18, TNFRSF25, TNFSF4, TNFSF13, TNFSF14, TNFSF13B, TNFSF15, ULBP1) closely related to ccRCC prognosis. Based on this, we established a EZH2-regulated immune-risk score prognostic model considered and filtered by TCGA database. Compared with ingle pathological features, our EZH2-regulated immune-risk score prognostic model combines pathological features showing profound ability to predict patients’ prognosis (AUC =0.816). The calibration curve confirmed that the nomogram was more reliable for predicting the survival rate of patients at 3 years.
There are several advantages of our model Firstly, it can accurately predict prognostic survival status in ccRCC. Secondly, it evaluates the ccRCC TME immune status, and could be a model for evaluating EZH2 inhibitor sensitivity in ccRCC patients. However, our model does have some limitations. Firstly, the potent mechanisms among activated NK cells, more CD8+ T cells, more Tregs and high expression of EZH2 are not yet clear in ccRCC and need further exploration. Secondly, whether the model is suitable for screening out ccRCC patients unsuitable for PD-1/PD-L1 inhibitor combined targeted drugs therapy still needs to explored and validated in the future.
Conclusions
We developed an immune risk score prognostic model based on EZH2-regulated immunogenes in ccRCC. This model can serve as an independent prognostic factor for ccRCC patients. The immune risk score prognostic model was based on all the immunostimulatory and immunosuppressive TILs within cancerous tissue. Our research also indicated model may be used to guide EZH2-targeted therapies for ccRCC patients. Further assessment of the effects of EZH2 on the TME will help inform the best utilization of EZH2 inhibitors in the clinic.
Acknowledgments
Funding: This work was supported by the National Natural Science Foundation of China (No. 82072829), and the Natural Science Basic Research Plan in Shaanxi Province of China (No. 2021JM-265).
Footnote
Reporting Checklist: The authors have completed the TRIPOD reporting checklist. Available at https://tau.amegroups.com/article/view/10.21037/tau-22-817/rc
Conflicts of Interest: All authors have completed the ICMJE uniform disclosure form (available at https://tau.amegroups.com/article/view/10.21037/tau-22-817/coif). The authors have no conflicts of interest to declare.
Ethical Statement: The authors are accountable for all aspects of the work in ensuring that questions related to the accuracy or integrity of any part of the work are appropriately investigated and resolved. The study was conducted in accordance with the Declaration of Helsinki (as revised in 2013).
Open Access Statement: This is an Open Access article distributed in accordance with the Creative Commons Attribution-NonCommercial-NoDerivs 4.0 International License (CC BY-NC-ND 4.0), which permits the non-commercial replication and distribution of the article with the strict proviso that no changes or edits are made and the original work is properly cited (including links to both the formal publication through the relevant DOI and the license). See: https://creativecommons.org/licenses/by-nc-nd/4.0/.
References
- Stone L. Control of oncogenic signalling in ccRCC. Nat Rev Urol 2022;19:453. [Crossref] [PubMed]
- Hsieh JJ, Purdue MP, Signoretti S, et al. Renal cell carcinoma. Nat Rev Dis Primers 2017;3:17009. [Crossref] [PubMed]
- Qiu J, Sharma S, Rollins RA, et al. The complex role of EZH2 in the tumor microenvironment: opportunities and challenges for immunotherapy combinations. Future Med Chem 2020;12:1415-30. [Crossref] [PubMed]
- Kang N, Eccleston M, Clermont PL, et al. EZH2 inhibition: a promising strategy to prevent cancer immune editing. Epigenomics 2020;12:1457-76. [Crossref] [PubMed]
- Shao FF, Chen BJ, Wu GQ. The functions of EZH2 in immune cells: Principles for novel immunotherapies. J Leukoc Biol 2021;110:77-87. [Crossref] [PubMed]
- Stairiker CJ, Thomas GD, Salek-Ardakani S. EZH2 as a Regulator of CD8+ T Cell Fate and Function. Front Immunol 2020;11:593203. [Crossref] [PubMed]
- Kim HJ, Cantor H, Cosmopoulos K. Overcoming Immune Checkpoint Blockade Resistance via EZH2 Inhibition. Trends Immunol 2020;41:948-63. [Crossref] [PubMed]
- Sun C, Zhao C, Li S, et al. EZH2 Expression is increased in BAP1-mutant renal clear cell carcinoma and is related to poor prognosis. J Cancer 2018;9:3787-96. [Crossref] [PubMed]
- Thoma C. Kidney cancer: Combining targeted and immunotherapy. Nat Rev Urol 2018;15:263. [PubMed]
- Adelaiye-Ogala R, Budka J, Damayanti NP, et al. EZH2 Modifies Sunitinib Resistance in Renal Cell Carcinoma by Kinome Reprogramming. Cancer Res 2017;77:6651-66. [Crossref] [PubMed]
- Eichenauer T, Simmendinger L, Fraune C, et al. High level of EZH2 expression is linked to high density of CD8-positive T-lymphocytes and an aggressive phenotype in renal cell carcinoma. World J Urol 2021;39:481-90. [Crossref] [PubMed]
- Ru B, Wong CN, Tong Y, et al. TISIDB: an integrated repository portal for tumor-immune system interactions. Bioinformatics 2019;35:4200-2. [Crossref] [PubMed]
- Highlights in kidney cancer. Clin Adv Hematol Oncol 2019;17:336-7. [PubMed]
- Turajlic S, Swanton C, Boshoff C. Kidney cancer: The next decade. J Exp Med 2018;215:2477-9. [Crossref] [PubMed]
- Hsieh JJ. The 2020 Kidney Cancer Treatment Sequence Issue. Clin Genitourin Cancer 2020;18:241-3. [Crossref] [PubMed]
- Albiges L, Tannir NM, Burotto M, et al. Nivolumab plus ipilimumab versus sunitinib for first-line treatment of advanced renal cell carcinoma: extended 4-year follow-up of the phase III CheckMate 214 trial. ESMO Open 2020;5:e001079. [Crossref] [PubMed]
- Escudier B, Motzer RJ, Tannir NM, et al. Efficacy of Nivolumab plus Ipilimumab According to Number of IMDC Risk Factors in CheckMate 214. Eur Urol 2020;77:449-53. [Crossref] [PubMed]
- Taylor MH, Lee CH, Makker V, et al. Phase IB/II Trial of Lenvatinib Plus Pembrolizumab in Patients With Advanced Renal Cell Carcinoma, Endometrial Cancer, and Other Selected Advanced Solid Tumors. J Clin Oncol 2020;38:1154-63. [Crossref] [PubMed]
- Motzer RJ, Jonasch E, Boyle S, et al. NCCN Guidelines Insights: Kidney Cancer, Version 1.2021. J Natl Compr Canc Netw 2020;18:1160-70. [Crossref] [PubMed]
- Motzer RJ, Robbins PB, Powles T, et al. Avelumab plus axitinib versus sunitinib in advanced renal cell carcinoma: biomarker analysis of the phase 3 JAVELIN Renal 101 trial. Nat Med 2020;26:1733-41. [Crossref] [PubMed]
- Highlights in Kidney Cancer. Clin Adv Hematol Oncol 2020;18:423-4. [PubMed]
- Xu W, Atkins MB, McDermott DF. Checkpoint inhibitor immunotherapy in kidney cancer. Nat Rev Urol 2020;17:137-50. [Crossref] [PubMed]
- Chen YN, Hou SQ, Jiang R, et al. EZH2 is a potential prognostic predictor of glioma. J Cell Mol Med 2021;25:925-36. [Crossref] [PubMed]
- Wagener N, Macher-Goeppinger S, Pritsch M, et al. Enhancer of zeste homolog 2 (EZH2) expression is an independent prognostic factor in renal cell carcinoma. BMC Cancer 2010;10:524. [Crossref] [PubMed]
- Wagener N, Holland D, Bulkescher J, et al. The enhancer of zeste homolog 2 gene contributes to cell proliferation and apoptosis resistance in renal cell carcinoma cells. Int J Cancer 2008;123:1545-50. [Crossref] [PubMed]
- Shen Y, Guo X, Wang Y, et al. Expression and significance of histone H3K27 demethylases in renal cell carcinoma. BMC Cancer 2012;12:470. [Crossref] [PubMed]
- Lee HW, Choe M. Expression of EZH2 in renal cell carcinoma as a novel prognostic marker. Pathol Int 2012;62:735-41. [Crossref] [PubMed]
(English Language Editor: K. Brown)