Development and validation of a novel defined mutation classifier based on Lasso logistic regression for predicting the overall survival of immune checkpoint inhibitor therapy in renal cell carcinoma
Highlight box
Key findings
• Depicting the mutation profile landscape of patients with advanced renal cell carcinoma. A novel mutation classifier was constructed for predicting the overall survival of advanced renal cell carcinoma patients to immune checkpoint inhibitor therapy.
What is known and what is new?
• Immune checkpoint inhibitor therapy has been recommended as the first-line treatment for advanced renal cancer, but the response rates are varying.
• Our study provided evidence support for screening advanced renal cell carcinoma patients who may benefit from Immune checkpoint inhibitor therapy.
What is the implication, and what should change now?
• Further validation in prospective studies with larger cohorts from multiple centers, and further combination with clinical information can provide ideas for the selection of patients receiving immunotherapy.
Introduction
Advanced tumor treatment has entered the era of immunotherapy, and immune checkpoint inhibitors (ICIs) represented by anti-programmed cell death protein 1/programmed cell death 1 ligand 1 (PD-1/PD-L1) therapy have been widely used in various solid tumors (1-3). According to the 2020 European Association of Urology (EAU) guidelines and the 2021 National Comprehensive Cancer Network (NCCN) guidelines (4,5), ICI therapy for advanced renal cancer has been recommended as the first-line treatment. In the process of advanced renal cancer therapy, ICI drugs have demonstrated their significant survival benefits, whether used alone or in combination with targeted drugs (6-8). However, the application of ICIs yields varying degrees of response rates in different solid tumors, and there is still a subset of patients with advanced renal cancer that cannot benefits from ICIs. In CheckMate 025, a phase 3 trial of nivolumab (a PD-1 inhibitor), the objective response rate (ORR) was 25% (6). Similar results were observed in CheckMate 214, a phase 3 trial with patients receiving nivolumab plus ipilimumab (a CTLA-4 inhibitor), with ORR confirmed as 42% (7). In another multicenter phase 3 study, pembrolizumab (a PD-1 inhibitor) in combination with axitinib [a unique vascular endothelial growth factor receptor (VEGFR) tyrosine kinase inhibitor] reached an ORR of 60% (8). Therefore, how to screen out patients with advanced renal cell carcinoma (RCC) who may benefit from ICIs has become a top priority in clinical research. Tumoral mutation burden (TMB) and microsatellite instability (MSI) emerged as positive predictive biomarkers for ICI therapy (9,10). Higher levels of TMB are derived from a higher frequency of gene mutations, and MSI associated with mismatch repair (MMR) machinery mutations may lead to the accumulation of neoantigens and stimulate antitumor immunity, thus predicting a favorable response to ICI therapy. However, controversy remains concerning the ability of TMB and MSI to predict ICI therapy efficacy in RCC (11-13). The expression of immune checkpoints such as PD-1 and PD-L1, have not been convincingly proved to predict response to ICIs in RCC (14,15). Meanwhile, clinicopathological characteristics have been demonstrated as potential indicators that enable the prediction of clinical response to ICI, such as human endogenous lentivirus virus expression and defective antigen presentation, may indicate poor response to ICI in RCC patients (15). However, there are still few prognostic factors available.
Gene mutation signatures have gradually been confirmed to predict the outcome of ICI therapy across multiple cancer types (16). And nonsynonymous gene mutation results in more neo-antigens, thus increasing chances for T cell recognition, and indicating better ICI outcomes (17). Unfortunately, few biomarkers or mutation signatures have been identified to predict the response to ICI therapy in patients RCC. Sun et al. refined a subgroup of TFE3-translocation RCC (TFE3-tRCC) patients who may benefit from ICI therapy (18). Moreover, Miao et al. reported that patients with clear cell renal cell carcinoma (ccRCC) harboring PBRM1 loss-of-function mutation seemed to be more responsive to immunotherapy with nivolumab (3). Hagiwara et al. also found that lower expression of PARP1 in patients with ccRCC and the PBRM1 mutation enjoyed a better prognosis after nivolumab treatment (19). Nevertheless, large-scale validation in multiple centers still needs to be performed to further verify these findings. Meanwhile, prognostic models established by multiple mutations remain lacking in RCC.
In this study, we determined the mutation profile landscape of patients with advanced RCC and subsequently developed a polygenic mutation classifier that could precisely predict the efficacy of ICI therapy. Furthermore, the mutation classifier was verified in independent validation sets. Ultimately, we conducted comprehensive bioinformatics analyses to evaluate the effect of the mutation classifier on the predictive value of ICI treatment response. We present the following article in accordance with the TRIPOD reporting checklist (available at https://tau.amegroups.com/article/view/10.21037/tau-23-21/rc).
Methods
Patients and samples
The somatic mutation data and clinical data of patients from the Memorial Sloan Kettering Cancer Center (MSKCC) pan-cancer ICI therapy cohort [n=1,610 (1,003 (62.3%) men; mean (SD) age, 61.6 (13.6) years)], with patients with advanced RCC included [n=143; 104 (72.7%) men; mean (SD) age, 60.2 (10.2) years] (9), were downloaded from cBioPortal (20). The data from the CheckMate ICI therapy cohort [n=261; 185 (70.9%) men; mean (SD) age, 60.5 (10.6) years; nivolumab treatment] and CheckMate non-ICI therapy cohort [n=193; 136 (70.5%) men, mean (SD) age, 61.1 (9.4) years; everolimus treatment] were obtained from the work of Braun et al. (21), with The Cancer Genome Atlas (TCGA) cohort (n=451; 293 (65.0%) men; mean (SD) age, 60.5 (12.1) years] downloaded from TCGA data portal (https://portal.gdc.cancer.gov/repository). Patients from the above cohorts were distinguished by the patient ID and other clinical characteristics to avoid any overlap of cases. Gene expression data in log2-transformed upper quartile-normalized transcripts per kilobase of exon model per million mapped reads (TPM) for the CheckMate ICI and non-ICI therapy cohort were also obtained from Braun et al. (21). TPM and high-throughput sequence counts data (HTSeq-Counts) for TCGA cohort were available and also downloaded from the TCGA data portal. All the patients from the MSKCC ICI therapy cohort and the CheckMate ICI therapy cohort received at least 1 dose of ICI therapy. The study was conducted in accordance with the Declaration of Helsinki (as revised in 2013).
TMB assessment
We collected and analyzed the TMB data from the MSKCC ICI therapy cohort and the CheckMate ICI therapy cohort. TMB data from the MSKCC ICI therapy cohort were generated from the Memorial Sloan Kettering Integrated Mutation Profiling of Actionable Cancer Targets (MSK-IMPACT). TMB data from the CheckMate ICI therapy cohort were calculated as the sum of all nonsynonymous mutations in a sample according to Braun et al. (21). TMB in the 2 cohorts was further stratified by the R package “survminer” (version 0.4.8; The R Foundation for Statistical Computing) as low-TMB and high-TMB.
Oncoplot and summarized information of gene mutation
Publicly available Mutation Annotation Format (MAF) files of the MSKCC advanced RCC ICI therapy cohort and the CheckMate ICI therapy cohort were used for further visualization.
R package “maftools” (version 2.12.0) was then used to graph the oncoplot and summarized information (22).
Protein–protein interaction network construction
A protein–protein interaction (PPI) network functional enrichment analysis was conducted on the Search Tool for the Retrieval of Interacting Genes/Proteins (STRING) website (https://string-db.org) and reconstructed using Cytoscape software version 3.9.1 (23).
Construction of the mutation classifier
A total of 261 patients with advanced RCC in the CheckMate ICI therapy cohort were randomly assigned to either a training set or a validation set in a nearly 3:2 ratio through “createDataPartition” function in R package “caret” (version 6.0). To conduct further analysis, 41 frequently mutated genes in the CheckMate and MSKCC advanced RCC cohorts were identified. Then, in the training set, the least absolute shrinkage and selection operator (Lasso) logistic regression model (R package “glmnet”, version 4.1) was used to narrow down the candidate genes and develop the mutation classifier. Ultimately, 10 genes and their coefficients were retained, with the penalty parameter (λ) decided by the minimum criteria. The risk score was calculated using the following formula: risk score = (beta1× mutation status of Gene1) + (beta2×mutation status of Gene2) + … + (betan× mutation status of Gene10). A gene with mutated status was coded as 1, while a wild-type gene was coded as 0. The Lasso logistic regression analysis generated beta as the regression coefficient. Patients in the training set were divided into a low-risk group and a high-risk group based on the optimal cutoff point of the risk score as calculated with the “surv_cutpoint” function in “survminer” package in R. The overall survival (OS) between the low- and high-risk groups was compared by Kaplan-Meier analysis. The receiver operating characteristic (ROC) curve analysis was conducted using R package “pROC” (version 1.18.0) to test the prognostic role of the risk score based on OS. After taking adjustment of other clinical covariates (gender, age, metastasis status, sarcoma-like status, rhabdomyoma-like status, TMB) using multivariate Cox regression, further evaluation of the prediction effect of the risk score was conducted by Harrell’s concordance index (C-index). The multivariate Cox regression was implemented using R package “rms” (version 6.2).
Internal and external validation of the mutation classifier
The risk score of patients in the CheckMate ICI validation set and the whole CheckMate ICI therapy cohort was calculated according to the same formula as that of the training set. Then, patients were divided into the low- and high-risk groups based on the “survminer” package in R. Kaplan-Meier analysis was performed to compare the OS between the low- and high-risk groups in the CheckMate ICI validation set and the whole CheckMate ICI therapy cohort for internal validation of the mutation classifier. We subsequently extracted the clinical information (gender, age, metastasis status, sarcoma-like property, rhabdomyoma-like property) of patients in the CheckMate ICI therapy cohort. These variables were analyzed in combination with TMB and the risk score in our Lasso regression model. Univariate and multivariate Cox regression models were employed for the independent prognostic analysis. ROC curve analysis and C-index calculation were implemented the same way in the training set.
Patients from each of the MSKCC advanced RCC ICI therapy cohort, MSKCC pan-cancer ICI therapy cohort, CheckMate non-ICI therapy cohort, and TCGA cohort were stratified into low- and high-risk groups using the same strategy as that used for CheckMate ICI therapy cohort in external validation. Kaplan-Meier analysis in the MSKCC advanced RCC and pan-cancer ICI therapy cohorts was undertaken to verify the genericity of the classifier, while it was also used on the CheckMate non-ICI therapy cohort and TCGA cohort to demonstrate the specificity of the mutation classifier in the prediction of ICI-treatment outcome. ROC curve analysis and C-index calculation were also conducted in 2 MSKCC ICI therapy cohorts with clinical information that we could collect (gender, age, TMB) for further testing the prognostic role of the classifier based on OS.
Single-sample gene set enrichment analysis (ssGSEA)
We conducted the ssGSEA to determine the activation levels of various pathways using the transcriptome data in the CheckMate ICI therapy cohort (n=123 in common with mutation data). The implementation of ssGSEA was performed with the R package “GSVA” (version 1.44.2) (24).
Gene set and functional enrichment analysis
The differentially expressed genes (DEGs) between the low- and high-risk groups in TCGA cohort (n=448 in common with mutation data) were screened with the |log2 fold change (FC)| ≥1 and false discovery rate (FDR) <0.05 using the R package “edgeR” (version 3.38.1) (25). Gene Ontology (GO) analysis and GSEA analysis based on the DEG analysis were conducted to investigate the differentially enriched pathways between the patients in the 2 risk groups with the R package “clusterProfiler” (version 4.4.4) (26).
Tumor-infiltrating immune cells analysis
The CIBERSORT algorithm was applied to calculate the immune cell composition of RCC tissues via an LM22 gene signature matrix (containing 547 genes that distinguish 22 human hematopoietic cell phenotypes) (27). The transcriptome profiles from the CheckMate ICI therapy cohort (n=123) and the TCGA cohort (n=448) were uploaded to the CIBERSORT website (http://cibersort.stanford.edu) as mixture files. The LM22 signature matrix file was used to run CIBERSORTx, with 1000 permutations and quantile normalization disabled on RNA sequencing (RNA-seq) data. CIBERSORT completed deconvolution with Monte Carlo sampling and derived an empirical P value. In the CheckMate ICI therapy cohort, 109 out of 123 samples had P values <0.05, while in the TCGA cohort, 258 out of 448 samples had P values <0.05. Only samples with a P value less than 0.05 were included in the subsequent analysis.
Statistical analysis
R software (version 4.2.0) was used to conduct statistical analyses in this study. Fisher exact test was performed to compare the differences in clinical features. Kaplan-Meier survival curves were generated and compared using the log-rank test. ROC curve analysis and C-index were used to test the prognostic role of the classifier. Univariate and multivariate Cox regression analyses were conducted to identify prognostic indicators of OS. A 2-tailed unpaired t-test and Wilcoxon rank-sum test were used to determine the differences between low- and high-risk groups with or without normal distribution, respectively. The Spearman correlation coefficient was applied to estimate the correlations among risk scores and expression levels of immune checkpoint molecules. Statistical significance indicated with a P value <0.05 in a 2-tailed test.
Results
Landscape and clinical significance of the mutation profile in advanced RCC patients
Somatic mutation data of 597 patients with advanced RCC were first collected, including 143 patients from the MSKCC ICI therapy cohort [104 (72.7%) men; mean (SD) age, 60.2 (10.2) years], 261 patients from the CheckMate ICI therapy cohort [185 (70.9%) men; mean (SD) age, 60.5 (10.6) years], and 193 patients from the CheckMate non-ICI therapy cohort [136 (70.5%) men; mean (SD) age, 61.1 (9.4) years]. We also collected somatic mutation data of 451 patients with RCC from TCGA cohort [293 (65.0%) men; mean (SD) age, 60.5 (12.1) years] and 1,610 pan-cancer patients from the MSKCC ICI therapy cohort [1,003 (62.3%) men; mean (SD) age, 61.6 (13.6) years] (Figure S1). The most predominant variant classification in advanced RCC was found to be missense mutation, while the most common variant type was single-nucleotide polymorphism (SNP) in advanced RCC (Figure S2A,S2B). The oncoplot of the MSKCC advanced RCC ICI therapy cohort revealed the top 45 frequently mutated genes, in which the mutation frequency of 42 genes was more than 2% (mutated in at least 2 patients) (Figure S3). When we constructed the mutation profile of these 42 genes in the CheckMate (ICI and non-ICI therapy) cohort, only 41 genes were mutated in patients with advanced RCC (Figure 1A).
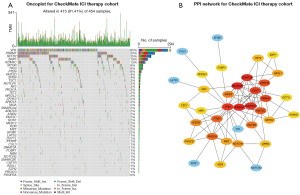
To better elucidate the functional relationship between these mutated genes, we used the 41 genes to construct a PPI network via the STRING database, and subsequently searched for hub genes using Cytoscape based on the PPI network (Figure 1B). ARID1A (NCBI Entrez 8289), TP53 (NCBI Entrez 7157), SMARCA4 (NCBI Entrez 6597), and SMARCB1 (NCBI Entrez 6598) were found to have more complex interactions (brighter colors) and were more centrally located in the PPI network, indicating the important role of these genes in regulating the progression of advanced RCC.
Construction of a prognostic mutation classifier in patients from the CheckMate ICI therapy cohort
To investigate the mutation-derived signatures that can predict the prognosis of ICI treatment, a total of 261 patients from the CheckMate ICI therapy cohort were randomly assigned to either a training set (n=157) or a validation set (n=104) in a nearly 3:2 ratio, with each patient having undergone PD-1 inhibitor therapy. The clinical features of all patients are shown in detail in Table 1. No statistically significant differences in clinical features were found between patients in the training set and validation set. We subsequently conducted Lasso logistic regression analysis on the mutation status of the 41 mutated genes in the training set and chose the model with the optimal area under the ROC curve (AUC) for predicting the OS status of patients receiving ICI therapy (Figure 2A,2B). Ultimately, 10 genes, CUL3, SF3B1, TET2, ARID1B, PBRM1, NOTCH2, PIK3CA, VHL, EZH2, and KMT2C, were identified to construct the mutation classifier. The prognostic mutation classifier was established based on the following formula:
Table 1
Variable | Total (N=261) | Data sets | ||
---|---|---|---|---|
Training (N=157) | Validation (N=104) | P values | ||
Gender | 0.890 | |||
Female | 76 (29.1%) | 45 (28.7%) | 31 (29.8%) | |
Male | 185 (70.9%) | 112 (71.3%) | 73 (70.2%) | |
Age (years) | 0.409 | |||
≤60 | 122 (46.7%) | 75 (47.8%) | 47 (45.2%) | |
>60 | 133 (51.0%) | 80 (51.0%) | 53 (51.0%) | |
Unknown | 6 (2.3%) | 2 (1.3%) | 4 (3.8%) | |
Metastasis | 0.587 | |||
No | 191 (73.2%) | 112 (71.3%) | 79 (76.0%) | |
Yes | 68 (26.1%) | 44 (28.0%) | 24 (23.1%) | |
Unknown | 2 (0.8%) | 1 (0.6%) | 1 (1.0%) | |
Sarcoma-like | 0.230 | |||
No | 195 (74.7%) | 118 (75.2%) | 77 (74.0%) | |
Yes | 26 (10.0%) | 12 (7.6%) | 14 (13.5%) | |
Unknown | 40 (15.3%) | 27 (17.2%) | 13 (12.5%) | |
Rhabdomyoma-like | 0.558 | |||
No | 205 (78.5%) | 120 (76.4%) | 85 (81.7%) | |
Yes | 16 (6.1%) | 10 (6.4%) | 6 (5.8%) | |
Unknown | 40 (15.3%) | 27 (17.2%) | 13 (12.5%) | |
OS status | 0.785 | |||
Living | 80 (30.7%) | 47 (29.9%) | 33 (31.7%) | |
Dead | 181 (69.3%) | 110 (70.1%) | 71 (68.3%) |
OS, overall survival; ICI, immune checkpoint inhibitor.
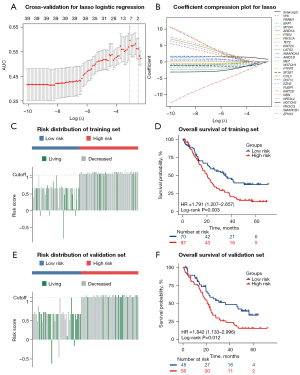
In the formula, a mutated gene was regarded as 1, and a wild-type gene was regarded as 0. The risk scores of the 157 patients in the training set ranged from –0.8485 to 1.2120, with –0.6206 to 1.1617 in the validation set of the CheckMate ICI therapy cohort.
We used the “survminer” package in R software to determine the optimal cutoff point and classify patients into low- and high-risk groups in the training and validation set, respectively. We then evaluated the risk score distributions and OS status of patients receiving ICI therapy in the training set, and patients with higher risk scores generally showed worse OS to ICI therapy compared with those with lower risk scores (Figure 2C). The result of the Kaplan-Meier analysis in the training set suggested that patients in the high-risk group had shorter median OS (low-risk group: median OS 35.6 months; high-risk group: median OS 20.3 months; P<0.01) (Figure 2D). Consistent with the training set, advanced RCC patients receiving ICI therapy with higher risk scores in the validation set tended to die earlier (Figure 2E). The result of the Kaplan-Meier analysis showed that patients in the high-risk group had a significantly poorer prognosis (low-risk group: median OS 36.4 months; high-risk group: median OS 20.9 months; P<0.05) (Figure 2F). The ROC curve analysis was used to test the prognostic role of the risk score based on OS. After taking adjustment of other clinical covariates (gender, age, metastasis status, sarcoma-like status, rhabdomyoma-like status, TMB) using multivariate Cox regression, further evaluation of the OS prediction effect of the risk score was conducted by Harrell’s concordance index (C-index). the C-index and AUC were 0.589 (95% CI: 0.522–0.656; P=0.009) and 0.711 (P<0.001) for OS prediction in the training set, respectively (Figure S4A). In the validation set, the C-index and AUC were 0.622 (95% CI: 0.551–0.693; P<0.001) and 0.642 (P=0.015), respectively (Figure S4B).
The mutation classifier vs. TMB as an independent prognostic factor for ICI therapy in advanced RCC patients
Univariate and multivariate Cox regression analysis were conducted to investigate the independent prognostic value of the mutation classifier for patients with advanced RCC from the entire CheckMate ICI therapy cohort. As shown in Figure 3A, the risk score calculated by our mutation classifier was a prognostic indicator for OS in univariate Cox regression analysis [hazard ratio (HR): 2.89; 95% CI: 1.69–4.96; P<0.001]. After adjustment for potential confounders, including TMB, in multivariate Cox regression analysis, the risk score remained an independent predictor of OS (HR: 2.20; 95% CI: 1.25–3.86; P<0.01) (Figure 3B). RCC with a sarcoma-like appearance was another variable that showed significance in the univariate and multivariate Cox regression analysis, while TMB showed no prognostic value for predicting OS of patients with advanced RCC receiving ICI therapy (Figure 3A,3B). The C-index and AUC were 0.577 (95% CI: 0.527–0.627; P=0.003) and 0.683 (P<0.001) for OS prediction respectively with the same clinical factors as in the training and validation sets (Figure S4C).
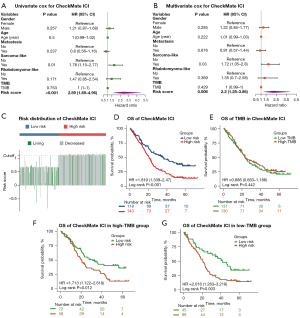
Subsequently, the R package “survminer” was used to examine the applicability of our mutation classifier in the entire CheckMate ICI therapy cohort: patients receiving ICI therapy with higher risk scores still received less benefit and shorter OS according Kaplan-Meier analysis (low-risk group: median OS 35.6 months; high-risk group: median OS 20.6 months; P<0.001) (Figure 3C,3D), which was consistent with the results of training or validation set alone. Patients were further divided into low-TMB and high-TMB groups based on the same method, and there was no difference in OS between the 2 groups (P=0.422) (Figure 3E). Meanwhile, when stratified by the TMB status, our mutation classifier remained a steady prognostic factor, with the low-risk group demonstrating longer OS than the high-risk group in low-TMB (low-risk group: median OS 41.5 months; high-risk group: median OS 20.6 months, P<0.05) and high-TMB (low-risk group: median OS 31.3 months; high-risk group: 20.3 months; P<0.05) (Figure 3F,3G). Notably, when the patients were classified by ORR into a complete response (CR)/partial response (PR) group, a stable disease (SD) group, and progressive disease (PD) group, the CR/PR group contained a higher proportion of patients in the low-risk group, although these differences did not reach statistical significance (Figure S4D).
External validation of the mutation classifier in the ICI therapy and non-ICI therapy cohorts
To explore the generalizability of our model across different ICI-treated populations and simultaneously validate its specificity in predicting the prognosis of ICI-treated as opposed to non–ICI-treated patients, we tested the classifier in the MSKCC advanced RCC ICI therapy cohort (n=143), MSKCC pan-cancer ICI therapy cohort (n=1,610), as well as in the CheckMate non-ICI therapy cohort (n=193) and TCGA cohort (n=451). As expected, patients in the low-risk group showed better OS both in the MSKCC advanced RCC ICI therapy cohort (low-risk group: median OS 50.0 months; high-risk group: median OS 11.0 months; P<0.001) and MSKCC pan-cancer ICI therapy cohort (low-risk group: median OS 30.0 months; high-risk group: median OS 15.0 months; P<0.001) (Figure 4A,4B). When utilizing the same ROC curve and C-index analysis method as in the ChecakMate cohort with available clinical factors (gender, age, TMB), the C-index and AUC were 0.568 (95% CI: 0.484–0.651; P=0.112) and 0.556 (P=0.296) for OS prediction in the MSKCC advanced RCC ICI therapy cohort, respectively (Figure S4E). In the MSKCC pan-cancer ICI therapy cohort, the C-index and AUC were 0.579 (95% CI: 0.558–0.601; P<0.001) and 0.584 (P<0.001) respectively (Figure S4F), indicating that our classifier also had a certain value in predicting the prognosis of ICI-treatments on the pan-cancer level. When extending our classifier to patients with RCC from the CheckMate non-ICI therapy cohort, we did not find any significant differences in OS between the 2 risk groups (P=0.535) (Figure 4C). Similar results were identified in patients with RCC from TCGA cohort (P=0.144) (Figure 4D). These results demonstrated the generalizability and specificity of the predictive value of our mutation classifier in patients with RCC responding to ICI therapy.
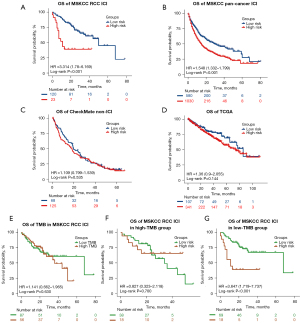
In accordance with the result of the CheckMate ICI therapy cohort, TMB had little value in predicting the OS in the MSKCC advanced RCC ICI therapy cohort (P=0.630) (Figure 4E). After TMB stratification, although no significant differences were observed between the 2 risk groups in patients with high TMB (P=0.700) (Figure 4F), patients with lower risk scores still obtained greater therapeutic benefit from ICI therapy than did patients with higher risk scores in the low-TMB group (low-risk group: median OS 68.0 months; high-risk group: median OS 11.0 months; P<0.001) (Figure 4G).
Pathway enrichment and gene function analyses based on the mutation classifier
To further elucidate the differences in the pathway enrichment and gene function between the subgroups categorized by the mutation classifier and to understand the mechanism of response to ICI therapy underlying the risk scores, we used the RNA-seq data from the CheckMate ICI therapy cohort and TCGA cohort. Based on the ssGSEA algorithm, pathway enrichment scores of different gene sets [hallmark gene sets, Kyoto Encyclopedia of Genes and Genomes (KEGG) gene sets, Reactome gene sets] were quantified in the CheckMate ICI therapy cohort. A heatmap was graphed to better demonstrate the function enrichment landscape between the 2 risk groups according to the mutation classifier (Figure 5A). By showing some of the pathways that were up- or downregulated between the 2 risk groups (Wilcoxon rank-sum P<0.05), we found that cell cycle-related pathways, such as the cell cycle pathway (P=0.005) from KEGG, the E2F targets pathway (P=0.014), and the G2M checkpoint pathway (P=0.047) from hallmark, were enriched in the high-risk group. The same situation was revealed for DNA repair–related pathways, including the DNA repair pathway (P=0.018) from hallmark and the homologous recombination pathway (P=0.021) from KEGG. Variation in these pathways may disturb the genomic stability status, thus leading to higher MSI, which may sensitize patients with advanced RCC to ICI therapy.
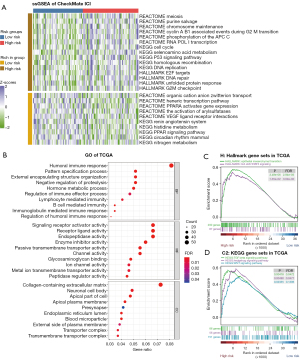
GO enrichment analysis and GSEA were then performed based on the DEG analysis between the 2 risk groups in TCGA cohort. Intriguingly, the GO results came to the conclusion that the DEGs were strongly correlated with the immune response (Figure 5B). The GSEA was performed to appraise the H sets (hallmark gene sets) and C2_KEGG sets (KEGG gene sets). In the hallmark gene sets, 17 pathways were significantly enriched in the high-risk group (FDR <0.25), including epithelial–mesenchymal transition (FDR <0.001) and Tyrosine-protein kinase-Signal transducer and activator of transcription 3 (JAK-STAT3) (FDR =0.030), with no pathway enrichment in the low-risk group (Figure 5C). Altered pathways from the KEGG gene sets included 42 enriched in the high-risk group and 2 in the low-risk group (FDR <0.25). Remarkably, the transforming growth factor beta (TGF-β) (FDR =0.047), Hedgehog (FDR =0.048), and WNT (FDR =0.140) signaling pathways were found to be significant in the high-risk group (Figure 5D). As previously reported, TGF-β plays a vital role in tumor immune evasion and is associated with poor responses to cancer immunotherapy (28), Hedgehog and WNT signaling cascades are crucial for cancer stem cell homeostasis and function (29). Patients with higher risk scores are more likely to be less responsive to immunotherapy.
RCC immune microenvironment analysis with the mutation classifier
According to the results shown above, RNA-seq data from the CheckMate ICI therapy cohort and TCGA cohort were used for further analysis to assess the relationship between immune status and the risk score as calculated by our mutation classifier. First, we used the CIBERSORT algorithm to estimate the fraction of 22 tumor-infiltrating immune cells (LM22) in the low- and high-risk groups. In the CheckMate ICI therapy cohort, the fractions of CD8+ T cells, M1 macrophages, and eosinophils were higher in the high-risk group, whereas the low-risk group had higher infiltration levels of M2 macrophages and neutrophils (Figure 6A,6B). Higher infiltration of regulatory T cells (Tregs), lower infiltration of resting natural killer (NK) cells and monocytes were also observed in the high-risk group in TCGA cohort (Figure S5A,S5B). We were surprised to find that patients in the high-risk group with a poorer OS on ICI therapy had a greater infiltration of CD8+ T cells within the tumor, in which situation the immune response of activated CD8+ T cells was thought to contribute to the therapeutic effect of ICI therapy. Kaplan-Meier analysis in the CheckMate ICI therapy cohort confirmed our conclusion that patients with advanced RCC with greater CD8+ T cell infiltration in the tumor have worse OS (low-infiltration group: median OS 25.9 months; high- infiltration group: median OS 16.7 months; P<0.01) (Figure 6C), which is also consistent with previous studies (30-32). Recent studies using single-cell RNA-seq technology have attempted to partly explain this phenomenon (15,33-35), suggesting that cases with a diversity of pre-existing CD8+ T cell clones undergo expansion and reprogramming during ICI therapy. Some of the CD8+ T cells subgroups like 4-1BBlow or CXCL13+ populations are critical for eliciting the favorable response within patients with ICI-treated advanced RCC. Other populations such as those with a higher expression level of immune checkpoints (PD-1, CTLA4, TIGIT, TIM-3, LAG3) and with a lower production of effective molecules (IFN-γ, TNF-α), referred to as highly exhausted CD8+ T cells, make no contribution or even play a counterproductive role to ICI therapy. To verify these suppositions, we subsequently investigated the correlation between the expressions of 16 previously reported common immune checkpoint genes and the risk score in the CheckMate ICI therapy cohort (Figure 6D). Interestingly, the risk score was positively correlated with LAG3 (R2=0.172; P=0.037) and was negatively correlated with CD40 (R2=–0.216; P=0.016) and CD70 (R2=–0.194; P=0.032). LAG3 participates in the inhibition of T-cell function, while CD40 and CD70 exert an opposite effect. Additionally, in TCGA cohort, the higher expression of PD-1 (P=0.045) and LAG3 (P=0.022), along with the lower expression of CD40 (P=0.014), was observed in the high-risk group compared with the low-risk group (Figure 6E). The expression levels of other immune checkpoint genes between the two risk groups can be seen in the supplementary materials (Figure S6).
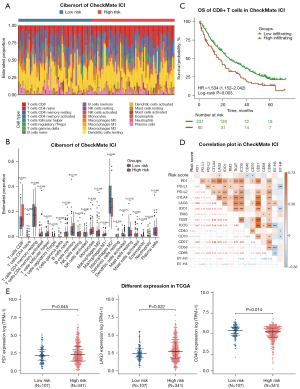
Discussion
ICI therapy has thus far been shown to have a powerful therapeutic efficacy in patients with advanced cancers. However, there is still a subset of patients who do not respond, with the innate and adaptive resistance to ICI therapy undetermined. Thus, novel biomarkers for predicting the ICI therapeutic response and the application of better-individualized treatments are urgently needed. In this study, we sought to explore the mutation patterns in advanced RCC and established a 10-gene mutation classifier using the CheckMate ICI therapy cohort. The efficacy of the classifier was then confirmed in the MSKCC advanced RCC ICI therapy cohort and additionally in the MSKCC pan-cancer ICI therapy cohort to further extend the applicability of the classifier. The risk scoring system based on the mutation classifier could effectively stratify patients into low- and high-risk groups, and it could be therefore concluded that patients with advanced RCC with a lower risk score had a longer OS. Moreover, functional enrichment analysis and immune infiltration analysis in the CheckMate ICI therapy cohort and TCGA cohort were conducted to clarify the potential biological mechanisms underlying the mutation classifier. Findings obtained from this study suggested that the 10-gene mutation classifier might be a potential indicator for assessing ICI efficacy in patients with advanced RCC.
Accumulation of somatic mutations throughout life can alter key cellular functions and lead to cancer (36). Additionally, the expression of nonsynonymous mutations can activate immune response by producing tumor-specific antigens called neoantigens (37). TMB is defined as the total number of somatic mutations per megabase, including single-nucleotide variants as well as frameshift mutations generated by insertion and deletions (indels). TMB mainly involves nonsynonymous mutations in the coding region, and the more mutations a tumor has, the higher probability that the amino acids, polypeptides, and proteins the mutated genes produce become neoantigens (38); this is the theoretical basis for explaining how TMB can predict the efficacy of ICI therapy. Since its conceptual establishment, TMB has become a useful biomarker for the identification of patients that would most benefit from ICIs across many cancer types (9). However, TMB is not a perfect biomarker. First, the levels of TMB vary in different types of tumors, and tumors originating from the kidney possess much lower levels of TMB (39). The thresholds for stratifying patients into low- and high-TMB groups differ and have not been thoroughly investigated across different tumors. Second, only a minority of mutations generate neoantigens that are properly processed and loaded onto Major histocompatibility complex (MHC), and even fewer can be recognized by T cells (40). Considering the high sensitivity of RCC to ICI therapy even with low levels of TMB, biomarkers derived from mutation information for predicting ICI therapy efficacy in RCC require further investigation.
In our study, TMB had little value in predicting the OS of ICI treatment in patients with advanced RCC. Nevertheless, some genetic mutations have been shown to affect the function of tumor immune-related pathways and reshape the tumor immune microenvironment, thus affecting ICI response. As reported previously, JAK1- and JAK2-truncating mutations led to a lack of response to interferon-γ, and the B2M-truncating mutation resulted in MHC-I expression loss in melanoma (41). In another study, the STAT5A mutation was found to elevate the sensitivity of PD-L1 expression and promoted the immune evasion of lymphoma (42). Moreover, different somatic alteration profiles have been shown capable of stratify patients with advanced RCC into different subtypes with distinctive transcriptomic signatures associated with ICI-treatment outcomes (43), indicating that mutations in RCC do have the potential to predict the efficacy of ICI therapy. Of the 10 genes included in our mutation classifier, VHL is the most commonly mutated gene, followed by PBRM1 in ccRCC, which accounts for approximately 70% of the pathological types of RCC (44). In addition to the critical role in promoting the initiation of ccRCC, VHL loss-of-function mutations were reported to upregulate the expression of PD-L1 through hypoxia-inducible factor-2 alpha (HIF-2α) in ccRCC (45).
The prognostic value of PBRM1 mutation is controversial. Miao et al. reported that patients with RCC and PBRM1 loss had amplified transcriptional outputs of HIF1 and STAT3, which are involved in hypoxia response and JAK-STAT signaling respectively. Furthermore, patients with PBRM1 loss experienced prolonged OS compared to patients without PBRM1 loss of function (3). However, Liu et al. reported that PBRM1 deficiency led to the inactivation of IFNγ-STAT1 signaling, resulting in a nonimmunogenic tumor microenvironment (TME) associated with reduced benefit from ICIs (46). In our classifier, however, the PBRM1 mutation was found to be a protective factor. PIK3CA is the third most frequently mutated gene in the classifier, with a mutation rate of 3% in the CheckMate cohort and 5% in the MSKCC advanced RCC cohort. Ugai et al. reported that PIK3CA mutation promoted PD-L1 expression through the PI3K signaling pathway in colorectal carcinoma (47), indicating that PIK3CA mutations may augment response to ICI therapy.
The biological mechanisms of the 10-gene mutation classifier relating to the predictive value for ICI therapy response were revealed by functional analysis. According to GSEA, we found enriched signaling pathways closely related to tumor immunity in the high-risk group. The IL-6–JAK–STAT3 signaling pathway plays a critical role in the formation of a immune-suppressive microenvironment, and coinhibition of PD-L1 with IL-6 was proven to be more effective in murine models of pancreatic cancer (48). Consistent with what we have discussed above, the TGF-β signaling pathway may mediate immune tolerance by reducing the activity of tumor-specific cytotoxic T lymphocytes (CTLs) in RCC (49). In ssGSEA analysis, given that the high-risk group was significantly enriched in DNA repair–related pathways, the innate immune-related pathways including cyclic GMP–AMP synthase (cGAS)-stimulator of interferon genes (STING) pathway would be less activated due to lower DNA replication stress in the high-risk group. Whereas, the innate immune system has been indicated recently to take part in recruiting immune cells to tumors and in arousing T-cell responses (50).
In immune infiltration analysis, we found greater infiltration of CD8+ T cells in the high-risk group and worse OS. Except for some subgroups of the CD8+ T cells with highly exhausted phenotypes we have discussed previously, we observed a higher expression of Tregs that may contribute to the immunosuppressive TME in the high-risk group. In particular, LAG3, a molecule that is expressed on multiple cell types (CD4+ T cells, CD8+ T cells, Tregs) to regulate T cells homeostasis, was identified to have a higher expression in the high-risk group. Furthermore, in cancer including RCC with persistent antigen-stimulation, elevated levels of chronic LAG3 expression can lead to T-cell exhaustion and subsequent impairment of T-cell function (51), thus providing further explanation for the immunosuppressive status in the high-risk group. Recently, the US Food and Drug Administration (FDA) approved the fixed-dose combination of relatlimab, a LAG3 inhibitor, plus nivolumab (Opdualag) in the treatment of melanoma. LAG3 is the third immune checkpoint molecule that has been approved for clinical application after CTLA-4 and PD-1/PD-L1. Our mutation classifier might provide some predictive information for determining when Opdualag therapy can be applied to the treatment of RCC.
Despite the consistent performance observed for predicting the OS of patients with advanced RCC receiving ICIs, our study had several limitations. First, our findings were based on a retrospective analysis using publicly available online data. Restriction in the number of available cases examined from a limited number of centers might have resulted in outcome bias during data analysis. The mutation classifier should be validated in prospective studies with larger cohorts from multiple centers in the future. Second, immune infiltration analysis was conducted through CIBERSORT, a bioinformatics algorithm using the bulk RNA-seq data of signature genes to estimate the relative infiltration levels of certain types of immune cells in samples. The results did not take the heterogeneity in tumor samples into consideration and ignored the phenotypic plasticity of immune cells in different tumors with different pathological states. More accurate methods, such as spatial transcriptome sequencing, can be used to further confirm the immune infiltration status in RCC. Third, clinicopathological characteristics such as exacerbation in proteinuria after ICI therapy (52), may also influence the prognosis of RCC patients, more adverse events post ICI treatment should be considered in future studies. Fourth, the mechanisms to stratify advanced patients with RCC into groups with different OS times underlying the 10-gene mutation classifier remain unclear. Further investigation through in vivo and in vitro experiments should be conducted to fully elucidate the impact of the 10-gene mutation classifier.
Conclusions
We constructed a mutation classifier with the ability to predict the OS of patients with advanced RCC to ICI therapy. This mutation classifier is expected to replace TMB in guiding clinical decision-making.
Acknowledgments
We would like to thank the cBioPortal for Cancer Genomics, TCGA, and David A. Braun (Department of Medical Oncology, Dana-Farber Cancer Institute, Boston, MA, 02215, USA) for their efforts and for providing valuable data.
Funding: This work was supported by grants from the National Natural Science Foundation of China (Nos. 81725016, 81872094, 81772718, 81602219, 81972376, and 81902576), the Guangdong Provincial Science and Technology Foundation of China (Nos. 2017B020227004 and 2017A030313538), and the Key Project of Basic and Applied Basic Research of Jiangmen, Guangdong (Nos. 2021030103460007434).
Footnote
Reporting Checklist: The authors have completed the TRIPOD reporting checklist. Available at https://tau.amegroups.com/article/view/10.21037/tau-23-21/rc
Peer Review File: Available at https://tau.amegroups.com/article/view/10.21037/tau-23-21/prf
Conflicts of Interest: All authors have completed the ICMJE uniform disclosure form (available at https://tau.amegroups.com/article/view/10.21037/tau-23-21/coif). The authors have no conflicts of interest to declare.
Ethical Statement: The authors are accountable for all aspects of the work in ensuring that questions related to the accuracy or integrity of any part of the work are appropriately investigated and resolved. The study was conducted in accordance with the Declaration of Helsinki (as revised in 2013).
Open Access Statement: This is an Open Access article distributed in accordance with the Creative Commons Attribution-NonCommercial-NoDerivs 4.0 International License (CC BY-NC-ND 4.0), which permits the non-commercial replication and distribution of the article with the strict proviso that no changes or edits are made and the original work is properly cited (including links to both the formal publication through the relevant DOI and the license). See: https://creativecommons.org/licenses/by-nc-nd/4.0/.
References
- Topalian SL, Drake CG, Pardoll DM. Immune checkpoint blockade: a common denominator approach to cancer therapy. Cancer Cell 2015;27:450-61. [Crossref] [PubMed]
- Gide TN, Quek C, Menzies AM, et al. Distinct Immune Cell Populations Define Response to Anti-PD-1 Monotherapy and Anti-PD-1/Anti-CTLA-4 Combined Therapy. Cancer Cell 2019;35:238-255.e6. [Crossref] [PubMed]
- Miao D, Margolis CA, Gao W, et al. Genomic correlates of response to immune checkpoint therapies in clear cell renal cell carcinoma. Science 2018;359:801-6. [Crossref] [PubMed]
- Rouprêt M, Babjuk M, Burger M, et al. European Association of Urology Guidelines on Upper Urinary Tract Urothelial Carcinoma: 2020 Update. Eur Urol 2021;79:62-79. [Crossref] [PubMed]
- Motzer RJ, Jonasch E, Boyle S, et al. NCCN Guidelines Insights: Kidney Cancer, Version 1.2021. J Natl Compr Canc Netw 2020;18:1160-70. [Crossref] [PubMed]
- Motzer RJ, Escudier B, McDermott DF, et al. Nivolumab versus Everolimus in Advanced Renal-Cell Carcinoma. N Engl J Med 2015;373:1803-13. [Crossref] [PubMed]
- Motzer RJ, Tannir NM, McDermott DF, et al. Nivolumab plus Ipilimumab versus Sunitinib in Advanced Renal-Cell Carcinoma. N Engl J Med 2018;378:1277-90. [Crossref] [PubMed]
- Powles T, Plimack ER, Soulières D, et al. Pembrolizumab plus axitinib versus sunitinib monotherapy as first-line treatment of advanced renal cell carcinoma (KEYNOTE-426): extended follow-up from a randomised, open-label, phase 3 trial. Lancet Oncol 2020;21:1563-73. [Crossref] [PubMed]
- Samstein RM, Lee CH, Shoushtari AN, et al. Tumor mutational load predicts survival after immunotherapy across multiple cancer types. Nat Genet 2019;51:202-6. [Crossref] [PubMed]
- Le DT, Uram JN, Wang H, et al. PD-1 Blockade in Tumors with Mismatch-Repair Deficiency. N Engl J Med 2015;372:2509-20. [Crossref] [PubMed]
- McGrail DJ, Pilié PG, Rashid NU, et al. High tumor mutation burden fails to predict immune checkpoint blockade response across all cancer types. Ann Oncol 2021;32:661-72. [Crossref] [PubMed]
- Hause RJ, Pritchard CC, Shendure J, et al. Classification and characterization of microsatellite instability across 18 cancer types. Nat Med 2016;22:1342-50. [Crossref] [PubMed]
- Yakirevich E, Patel NR. Tumor mutational burden and immune signatures interplay in renal cell carcinoma. Ann Transl Med 2020;8:269. [Crossref] [PubMed]
- Erlmeier F, Weichert W, Schrader AJ, et al. Prognostic impact of PD-1 and its ligands in renal cell carcinoma. Med Oncol 2017;34:99. [Crossref] [PubMed]
- Au L, Hatipoglu E, Robert de Massy M, et al. Determinants of anti-PD-1 response and resistance in clear cell renal cell carcinoma. Cancer Cell 2021;39:1497-1518.e11. [Crossref] [PubMed]
- Wang F, Zhao Q, Wang YN, et al. Evaluation of POLE and POLD1 Mutations as Biomarkers for Immunotherapy Outcomes Across Multiple Cancer Types. JAMA Oncol 2019;5:1504-6. [Crossref] [PubMed]
- Jardim DL, Goodman A, de Melo Gagliato D, et al. The Challenges of Tumor Mutational Burden as an Immunotherapy Biomarker. Cancer Cell 2021;39:154-73. [Crossref] [PubMed]
- Sun G, Chen J, Liang J, et al. Integrated exome and RNA sequencing of TFE3-translocation renal cell carcinoma. Nat Commun 2021;12:5262. [Crossref] [PubMed]
- Hagiwara M, Fushimi A, Matsumoto K, et al. The Significance of PARP1 as a biomarker for Predicting the Response to PD-L1 Blockade in Patients with PBRM1-mutated Clear Cell Renal Cell Carcinoma. Eur Urol 2022;81:145-8. [Crossref] [PubMed]
- Gao J, Aksoy BA, Dogrusoz U, et al. Integrative analysis of complex cancer genomics and clinical profiles using the cBioPortal. Sci Signal 2013;6:pl1. [Crossref] [PubMed]
- Braun DA, Hou Y, Bakouny Z, et al. Interplay of somatic alterations and immune infiltration modulates response to PD-1 blockade in advanced clear cell renal cell carcinoma. Nat Med 2020;26:909-18. [Crossref] [PubMed]
- Mayakonda A, Lin DC, Assenov Y, et al. Maftools: efficient and comprehensive analysis of somatic variants in cancer. Genome Res 2018;28:1747-56. [Crossref] [PubMed]
- Shannon P, Markiel A, Ozier O, et al. Cytoscape: a software environment for integrated models of biomolecular interaction networks. Genome Res 2003;13:2498-504. [Crossref] [PubMed]
- Hänzelmann S, Castelo R, Guinney J. GSVA: gene set variation analysis for microarray and RNA-seq data. BMC Bioinformatics 2013;14:7. [Crossref] [PubMed]
- Robinson MD, McCarthy DJ, Smyth GK. edgeR: a Bioconductor package for differential expression analysis of digital gene expression data. Bioinformatics 2010;26:139-40. [Crossref] [PubMed]
- Wu T, Hu E, Xu S, et al. clusterProfiler 4.0: A universal enrichment tool for interpreting omics data. Innovation (Camb) 2021;2:100141. [Crossref] [PubMed]
- Newman AM, Liu CL, Green MR, et al. Robust enumeration of cell subsets from tissue expression profiles. Nat Methods 2015;12:453-7. [Crossref] [PubMed]
- Batlle E, Massague J. Transforming Growth Factor-beta Signaling in Immunity and Cancer. Immunity 2019;50:924-40. [Crossref] [PubMed]
- Clara JA, Monge C, Yang Y, et al. Targeting signalling pathways and the immune microenvironment of cancer stem cells - a clinical update. Nat Rev Clin Oncol 2020;17:204-32. [Crossref] [PubMed]
- Fridman WH, Zitvogel L, Sautès-Fridman C, et al. The immune contexture in cancer prognosis and treatment. Nat Rev Clin Oncol 2017;14:717-34. [Crossref] [PubMed]
- Drake CG, Stein MN. The Immunobiology of Kidney Cancer. J Clin Oncol 2018; Epub ahead of print. [Crossref] [PubMed]
- Galon J, Bruni D. Tumor Immunology and Tumor Evolution: Intertwined Histories. Immunity 2020;52:55-81. [Crossref] [PubMed]
- Bi K, He MX, Bakouny Z, et al. Tumor and immune reprogramming during immunotherapy in advanced renal cell carcinoma. Cancer Cell 2021;39:649-661.e5. [Crossref] [PubMed]
- Dai S, Zeng H, Liu Z, et al. Intratumoral CXCL13(+)CD8(+)T cell infiltration determines poor clinical outcomes and immunoevasive contexture in patients with clear cell renal cell carcinoma. J Immunother Cancer 2021;9:e001823. [Crossref] [PubMed]
- Krishna C, DiNatale RG, Kuo F, et al. Single-cell sequencing links multiregional immune landscapes and tissue-resident T cells in ccRCC to tumor topology and therapy efficacy. Cancer Cell 2021;39:662-677.e6. [Crossref] [PubMed]
- Martincorena I, Campbell PJ. Somatic mutation in cancer and normal cells. Science 2015;349:1483-9. [Crossref] [PubMed]
- Peng M, Mo Y, Wang Y, et al. Neoantigen vaccine: an emerging tumor immunotherapy. Mol Cancer 2019;18:128. [Crossref] [PubMed]
- Yarchoan M, Johnson BA 3rd, Lutz ER, et al. Targeting neoantigens to augment antitumour immunity. Nat Rev Cancer 2017;17:209-22. [Crossref] [PubMed]
- Valero C, Lee M, Hoen D, et al. Response Rates to Anti-PD-1 Immunotherapy in Microsatellite-Stable Solid Tumors With 10 or More Mutations per Megabase. JAMA Oncol 2021;7:739-43. [Crossref] [PubMed]
- Coulie PG, Van den Eynde BJ, van der Bruggen P, et al. Tumour antigens recognized by T lymphocytes: at the core of cancer immunotherapy. Nat Rev Cancer 2014;14:135-46. [Crossref] [PubMed]
- Zaretsky JM, Garcia-Diaz A, Shin DS, et al. Mutations Associated with Acquired Resistance to PD-1 Blockade in Melanoma. N Engl J Med 2016;375:819-29. [Crossref] [PubMed]
- Rong QX, Wang F, Guo ZX, et al. GM-CSF mediates immune evasion via upregulation of PD-L1 expression in extranodal natural killer/T cell lymphoma. Mol Cancer 2021;20:80. [Crossref] [PubMed]
- Motzer RJ, Banchereau R, Hamidi H, et al. Molecular Subsets in Renal Cancer Determine Outcome to Checkpoint and Angiogenesis Blockade. Cancer Cell 2020;38:803-817.e4. [Crossref] [PubMed]
- Jonasch E, Walker CL, Rathmell WK. Clear cell renal cell carcinoma ontogeny and mechanisms of lethality. Nat Rev Nephrol 2021;17:245-61. [Crossref] [PubMed]
- Messai Y, Gad S, Noman MZ, et al. Renal Cell Carcinoma Programmed Death-ligand 1, a New Direct Target of Hypoxia-inducible Factor-2 Alpha, is Regulated by von Hippel-Lindau Gene Mutation Status. Eur Urol 2016;70:623-32. [Crossref] [PubMed]
- Liu XD, Kong W, Peterson CB, et al. PBRM1 loss defines a nonimmunogenic tumor phenotype associated with checkpoint inhibitor resistance in renal carcinoma. Nat Commun 2020;11:2135. [Crossref] [PubMed]
- Ugai T, Zhao M, Shimizu T, et al. Association of PIK3CA mutation and PTEN loss with expression of CD274 (PD-L1) in colorectal carcinoma. Oncoimmunology 2021;10:1956173. [Crossref] [PubMed]
- Mace TA, Shakya R, Pitarresi JR, et al. IL-6 and PD-L1 antibody blockade combination therapy reduces tumour progression in murine models of pancreatic cancer. Gut 2018;67:320-32. [Crossref] [PubMed]
- Wang L, Wen W, Yuan J, et al. Immunotherapy for human renal cell carcinoma by adoptive transfer of autologous transforming growth factor beta-insensitive CD8+ T cells. Clin Cancer Res 2010;16:164-73. [Crossref] [PubMed]
- Jiang M, Jia K, Wang L, et al. Alterations of DNA damage response pathway: Biomarker and therapeutic strategy for cancer immunotherapy. Acta Pharm Sin B 2021;11:2983-94. [Crossref] [PubMed]
- Wherry EJ. T cell exhaustion. Nat Immunol 2011;12:492-9. [Crossref] [PubMed]
- Ning K, Wu Z, Zou X, et al. Immune checkpoint inhibitors further aggravate proteinuria in patients with metastatic renal cell carcinoma after long-term targeted therapy. Transl Androl Urol 2022;11:386-96. [Crossref] [PubMed]