Comprehensive analysis of alternative polyadenylation regulators of CTLA4 and immune infiltration in clear cell renal cell carcinoma
Highlight box
Key findings
• APA regulators play a key role in immune infiltration in ccRCC and SNRNP70 is a promising prognostic biomarker and a potential target for ccRCC’s immunotherapy.
What is known and what is new?
• New evidence has uncovered a vital role of APA in many human cancers. Several APA-related genes have been identified as important regulators and prognostic indicators for a variety of cancers.
• The expression of 36 key APA regulators in ccRCC was under researched and two groups with different characteristics were identified by using consensus clustering methods.
What is the implication, and what should change now?
• These findings will aid in risk classification and the precise treatment of ccRCC patients. However, these results require further reliable validation analyses with additional samples in the future. Additionally, more fundamental experiments are required to investigate the potential link between APA and the TIME.
Introduction
Renal cell carcinoma is a common malignancy (1), of which clear cell renal cell carcinoma (ccRCC) accounts for approximately 70% of the cases (2). Systemic treatment of ccRCC includes chemotherapy, targeted therapy, and immunotherapy. However, chemotherapy has a limited therapeutic effect on ccRCC and is mainly combined with experimental immune drugs as treatment. Numerous clinical studies have confirmed that monotherapy or combined therapy with immune checkpoint inhibitors can provide a significant survival benefit for ccRCC patients. As a result, immune checkpoint inhibitors have been included in various guidelines for first- and second-line therapeutic use since 2015 (3-5). Despite the increasing use of immune checkpoint inhibitors, most ccRCC patients do not derive lasting clinical benefits from mainstream immunotherapies like co-therapy with anti-Cytotoxic T-Lymphocyte Associated Protein 4 (CTLA4) and/or anti-programmed cell death 1 (PD-1) antibodies (5,6). Therefore, a biological understanding of the regulatory mechanism of the tumor immune microenvironment (TIME) and the tumor response to immunotherapy in ccRCC may help improve treatment efficacy.
Alternative polyadenylation (APA) is a critical RNA-processing mechanism that produces different 3' termini on mRNAs derived from different poly(A) sites (PAS). At least 70% of human genes have more than two transcript isoforms whose 3' untranslated regions (3'UTRs) are available (7,8). APA has been shown to play a role in critical biological processes (e.g., cell growth, proliferation, and differentiation) and various human diseases (9). Recently, several researchers have reported that APA plays an important role in tumor progression (10). For example, NUDT21 inhibits bladder cancer progression through ANXA2 and LIMK2 via APA (11). One study identified APA dysregulation as a contributor to the differentiation block of acute myeloid leukemia (12). Moreover, Wang et al. reported that upregulation of CPSF1 or PABPN1 was associated with tumorigenesis, proliferation, metastasis, and chemotherapy sensitivity in breast cancer (13). In addition, Zhong et al. explored the potential of APA and alternative splicing (AS) interaction in immunotherapy and targeted therapy of ccRCC (14). However, the mechanism by which APA affects tumors via the TIME remains poorly understood.
In this study, a systematic analysis of the expression profiles of 36 APA regulatory factors and their role in ccRCC was carried out. In addition, two different ccRCC clusters were identified based on the level of APA factor expression and the tumor heterogeneity and compositional diversity of the TIME between the two subtypes were compared. SNRNP70 was identified as a promising APA regulator associated with immune infiltration, and its elevated expression was associated with poor prognosis in ccRCC. Finally, this study also investigated the expression levels, prognostic value, and correlation of SNRNP70 with immune checkpoint-related genes in 33 types of cancer. Thus, the findings from this study shed new light on APA’s role in the TIME and immunotherapy of ccRCC. We present the following article in accordance with the REMARK reporting checklist (available at https://tau.amegroups.com/article/view/10.21037/tau-22-565/rc).
Methods
Data acquisition
The expression profiles of genes and clinical information about ccRCCs were downloaded from The Cancer Genome Atlas (TCGA; https://portal.gdc.cancer.gov/) (15). The dataset contained 539 ccRCC tissues and 72 normal kidneys. The SNRNP70, RNA-seq, and corresponding clinical characteristics for the pan-cancer analysis were obtained from the Genomic Data Commons (GDC) Data Portal. In addition, healthy tissue samples were downloaded from the Genotype Tissue Expression dataset (GTEx; http://commonfund.nih.gov/GTEx/) (16). To further validate the level of SNRNP70 expression, the GSE15641 dataset from the Gene Expression Omnibus database available at https://www.ncbi.nlm.nih.gov/geo/ was downloaded and used (17). Table S1 provides abbreviations for 33 types of cancer. The study was conducted in accordance with the Declaration of Helsinki (as revised in 2013).
Bioinformatics analysis
Based on the expression profiles of 36 APA regulatory factors in patients with ccRCC, the clustering analysis (80% of all samples were drawn 1,000 times, and the maximum number of clusters was six) was performed using the Consensus Cluster Plus package (version 1.56.0) (18). A principal component analysis (PCA) and visualization were conducted with the R package ggplot2 (version 3.3.5) (19). Gene Ontology (GO) and Kyoto Encyclopedia of Genes and Genomes (KEGG) analyses were performed using the clusterProfiler package (version 4.0.5) (20). The P values obtained from the permutation test were adjusted for multiple testing using the Benjamini-Hochberg correction method. A pathway that had a P value or false discovery rate (FDR) <0.05 was significantly enriched. The level of immune cell infiltration in ccRCC was assessed using the ssGSEA function of the GSVA package (version 1.40.1) (21). Corresponding immune cell marker genes were obtained from Bindea et al. (22). R packages ggplot2 (version 3.3.5) and ComplexHeatmap (version 2.8.0) were used to visualize the relative fractions (23). To conduct survival analyses, the survival package (version 3.2-13) and survminer package (version 0.4.9) were used (24,25). Cox proportional hazards regression models were used to determine the prognostic value of SNRNP70 in a variety of cancers. Clinical factors (age; T, N, and M stages; pathologic stage; histologic grade) and the level of SNRNP70 expression were used to construct prognostic nomograms using R package rms (version 6.3-0) to assess the rate of the 1-, 3-, and 5-year overall survival (OS) in patients with ccRCC (26).
Statistical analysis
RStudio (version 4.1.3) was used to perform the statistical tests. For comparisons between groups, a Wilcoxon rank sum test or Kruskal-Wallis test was applied. The correlation between APA regulators and immune checkpoint-related genes was evaluated using a Spearman correlation analysis. Log-rank tests and a univariate Cox proportional hazard regression analysis were used to compute the P values and 95% confidence intervals (CI) for the Kaplan-Meier (KM) curves. The independent prognostic value of SNRNP70 in combination with other clinical features was determined using univariate and multivariate analyses based on Cox regression models. Using the receiver operating characteristic (ROC) curves of the R package timeROC (version 0.4), 1-, 3-, and 5-year OS prediction efficiency of the genes were estimated (27). Statistical significance was determined as *, P<0.05; **, P<0.01; and ***, P<0.001.
Results
Differences in APA regulator factor expression between ccRCC and normal samples
To investigate the biological impact of APA regulators in ccRCC, the expression profiles of 36 APA regulators in TCGA were systematically explored. APA regulators were differentially expressed in ccRCC and normal tissues (Figure 1), including the upregulated APA regulators, CLP1, CSTF1, PCF11, WDR33, RRAGA, RBBP6, SYMPK, NXF1, PTBP1, CPSF7, CPSF4, PABPN1, CPSF1, PABPC4, HNRNPH1, CPSF4L, SNRPA, CELF2, and SNRNP70, in addition to the downregulated genes, NUDT21, HNRNPH2, CPSF2, CSTF2, PPP1CB, FIP1L1, HNRNPF, SRSF3, HNRNPK, and HNRNPC (P<0.05). Moreover, the prognosis and correlation between gene expression indicated that the expression of most APA regulators played a significant prognostic role and was positively correlated in ccRCC (Figure 2). These results demonstrated that APA regulators may contribute to the development and progression of ccRCC.
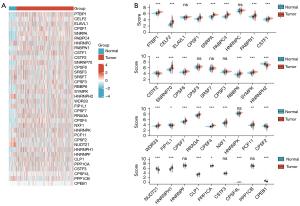
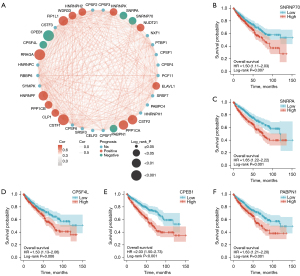
Consensus clustering analysis of APA regulators
Gene expression profiles and ambiguous clustering measures were used to perform a consensus clustering analysis. K-means clustering (K=2 clusters) was adopted as a suitable parameter from 2 to 6 (Figure 3A,3B; Figure S1A-S1F). A total of 539 ccRCC patients were segregated into Cluster 1, which contained 219 patients, and Cluster 2, which contained 320 patients. A differential expression analysis of APA regulators was performed between the two subtypes. Compared with Cluster 2, 12 of the 36 factors exhibited statistically high expression, whereas 14 factors showed low expression levels. Of the remaining 10 factors, no significant difference was observed between the two clusters (Figure 3C). Next, the clinicopathological characters and prognosis from both clusters were grouped for a differentiation analysis (Table 1). A preferential correlation was observed between Cluster 1 and a higher tumor stage (P<0.01) and grade (P<0.001). Moreover, the survival curve showing overall survival (OS), disease-specific survival (DSS), and progress free interval (PFI) were significantly favorable for Cluster 2 compared to that of Cluster 1 (OS Log-rank P<0.05, DSS Log-rank P<0.01, PFI Log-rank P<0.01) (Figure 3D-3F). PCA was used to further confirm the difference in the expression level between the two subtypes (Figure S1G). These results showed that significant differences exist in the expression profiles of the two clusters.
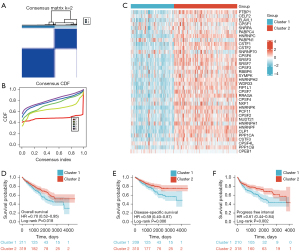
Table 1
Characteristic | Cluster 1 | Cluster 2 | P |
---|---|---|---|
n | 219 | 320 | |
Gender, n (%) | <0.001 | ||
Female | 53 (9.8) | 133 (24.7) | |
Male | 166 (30.8) | 187 (34.7) | |
Race, n (%) | 0.665 | ||
Asian | 2 (0.4) | 6 (1.1) | |
Black or African American | 20 (3.7) | 37 (6.9) | |
Not available | 3 (0.6) | 4 (0.7) | |
White | 194 (36.0) | 273 (50.6) | |
M, n (%) | 0.427 | ||
M0 | 173 (32.1) | 255 (47.3) | |
M1 | 36 (6.7) | 42 (7.8) | |
MX | 10 (1.9) | 21 (3.9) | |
Not available | 0 (0) | 2 (0.4) | |
N, n (%) | 0.413 | ||
N0 | 95 (17.6) | 146 (27.1) | |
N1 | 9 (1.7) | 7 (1.3) | |
NX | 115 (21.3) | 167 (31.0) | |
pTNM_stage, n (%) | 0.006 | ||
Discrepancy | 2 (0.4) | 1 (0.2) | |
Stage I | 91 (16.9) | 181 (33.6) | |
Stage II | 24 (4.5) | 35 (6.5) | |
Stage III | 64 (11.9) | 59 (10.9) | |
Stage IV | 38 (7.1) | 44 (8.2) | |
Grade, n (%) | <0.001 | ||
G1 | 3 (0.6) | 11 (2.0) | |
G2 | 79 (14.7) | 156 (28.9) | |
G3 | 90 (16.7) | 117 (21.7) | |
G4 | 41 (7.6) | 34 (6.3) | |
GX | 5 (0.9) | 0 (0) | |
Not available | 1 (0.2) | 2 (0.4) | |
Age, median (IQR), years | 60 (53.5, 70) | 61 (51, 70) | 0.371 |
ccRCC, clear cell renal cell carcinoma; TCGA, The Cancer Genome Atlas; pTNM, pathologic tumor node metastasis; IQR, interquartile range.
Association between APA regulators with levels of CD274, CTLA4, and PDCD1 expression and tumor-infiltrating immune cells
To research on the association between CD274, CTLA4, and PDCD1 and APA factors, this study investigated the differences in the levels of CD274, CTLA4, and PDCD1 expression in normal tissues and tumor samples and its correlation with APA regulators. Compared with normal adjacent tissues, CD274, CTLA4, and PDCD1 were remarkably overexpressed in the ccRCC tissues (P<0.001; Figure 4A-4C). An analysis of 539 ccRCC patients revealed that CD274, CTLA4, and PDCD1 were positively associated with the expression of the majority of APA regulatory factors, including CTLA4, CELF2, CPSF1 (Figure 4D). To further explore the role of APA regulatory factors in the ccRCC immune microenvironment, tumor-infiltrating immune cell densities were calculated based on the two clustered subtypes (Figure 4E). Cluster 1 was characterized by a lower percentage of the majority of immune cell types compared to that of Cluster 2. Specifically, Cluster 1 showed higher proportions of NK CD56 bright cells, cytotoxic cells, and Tregs (Figure 4F). Through the use of the GO and KEGG methods, the underlying regulatory mechanisms responsible for the differences in the TIME between the two clusters were clarified. Subsequently, the GO annotation and KEGG pathway enrichment analyses of upregulated differential expression genes identified major biological processes, including the humoral immune response, hormone metabolic process, B cell-mediated immunity, and leukocyte migration (Figure S1H-S1I).
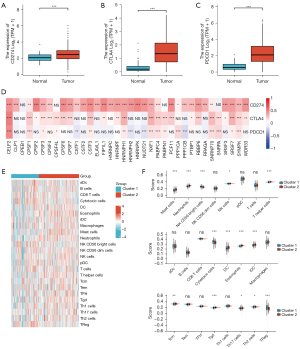
Key APA regulator SNRNP70 is upregulated in ccRCC and represents an independent prognostic marker in ccRCC
Notable tumor heterogeneity and differences in the TIME were observed between the two ccRCC clusters, as previously described. These results suggest that APA regulatory factors play a critical role in tumor progression and immune cell infiltration. Key APA regulatory factors were identified among the 36 APA regulators according to the elevated expression of APA regulators in ccRCC, the association with poor prognosis, and the correlation with CD274, CTLA4, and PDCD1 expression. The Venn diagram and the heat map showed that SNRNP70 appeared to represent an important APA regulator of poor prognosis and was positively correlated with the level of CD274, CTLA4, and PDCD1 expression (Figure 5A,5B). Analysis of the TCGA and GEO databases from this study further depicted the expression of SNRNP70 in ccRCC. SNRNP70 exhibited a significantly higher level of expression in the TCGA and GSE15641 datasets compared to the normal tissues in ccRCC (P<0.001; Figure 5C,5D). In addition, the upregulated SNRNP70 group exhibited worse overall survival and disease-specific survival compared to that of the low-expression group (P<0.05; Figure 2B, Figure 6A). Moreover, ccRCC patients with higher SNRNP70 expression were associated with a lower ratio of the number of survivors to the number of deaths and shorter survival times (Figure 6B,6C). Next, the ROC curve was used to estimate the prognostic significance of SNRNP70 with the 1-, 3-, and 5-year survival in ccRCC (Figure 6D). The univariate and multivariate analyses indicated that SNRNP70 was a promising prognostic factor in ccRCC (Figure 6E,6F). According to the nomogram, the level of SNRNP70 expression contributed multiple points in comparison with other clinical factors, such as the T, N, and M stages; pathologic stage; and histologic grade (Figure 6G). According to the calibration plot, the predicted and observed lines were in good agreement (Figure 6H).
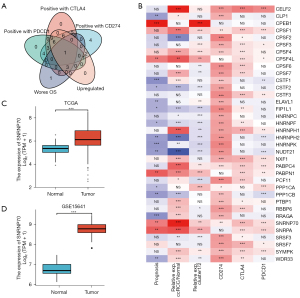
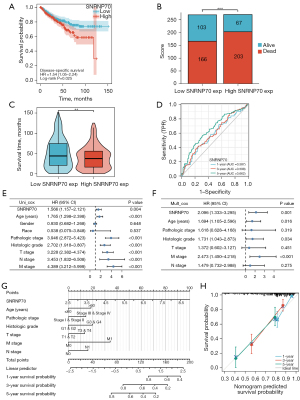
Correlation analysis of SNRNP70 with CTLA4 and infiltrating immune cells
According to the correlation analysis, SNRNP70 expression was positively correlated with CTLA4 expression in high histologic grades (Figure 7A-7C). The median level of SNRNP70 expression was used to divide patients into two groups. GO and KEGG pathway enrichment analyses were performed to further investigate the biological functions of SNRNP70 in ccRCC. The GO annotation identified major biological processes, such as the humoral immune response, chemical carcinogenesis, lymphocyte-mediated immunity, B cell-mediated immunity, and regulation of the humoral immune response (Figure 7D). The KEGG analysis showed that these pathways were included in the PPAR signaling pathway (Figure 7D). SNRNP70 appears to participate in crucial signaling pathways in the TIME in ccRCC. The GSVA package was used to calculate the percentage of immune cell subpopulations (Figure 7E). As shown in the boxplot of Figure 7F, the high SNRNP70 expression group had a higher proportion of CD8 T cells, T helper cells, and T effector memory (Tem), whereas the low expression group displayed a higher proportion of T cells, dendritic cells (DCs), activated DCs (aDCs), B cells, eosinophils, and immature DCs (iDCs).
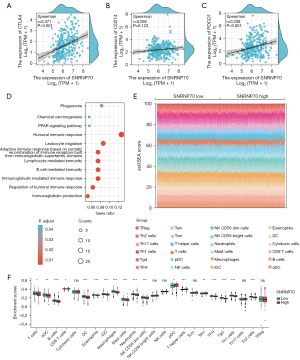
Comprehensive analysis of pan-cancer SNRNP70 expression
Since APA and immune-cell infiltration can lead to the development and progression of a variety of cancers, a correlation analysis on whether SNRNP70 expression can predict a poor prognosis and affects the TIME in other types of cancers was conducted. Therefore, the level of SNRNP70 expression was compared between tumor samples and normal samples from the TCGA as well as GTEx databases. According to the results, SNRNP70 was significantly upregulated in 5 (KIRC, HNSC, DLBC, THYM, and CHOL) and downregulated in 22 (OV, THCA, UCS, ESCA, ACC, UCEC, READ, STAD, LUAD, KICH, COAD, PRAD, CESC, LUSC, SKCM, BRCA, GBM, TGCT, PAAD, LIHC, LGG, and LAML) cancer types (Figure 8A). The prognostic value of SNRNP70 in 32 other cancers was further confirmed. A Univariate Cox regression analysis of 33 cancers showed high expression of SNRNP70 was associated with a worse prognosis in six cancer types (ACC, COAD, KIRC, LIHC, PAAD, and SARC) (Figure 8B). Additionally, the correlation analysis between SNRNP70 and the 10 most frequent immune checkpoint-related genes was also analyzed. These 10 immune checkpoint-associated genes included CTLA4, CD274, CD24, CD47, HAVCR2, LAG3, PD-1, PD-L2, SIGLEC15, and TIGIT. The results revealed that SNRNP70 was closely associated with the expression of immune checkpoint-associated genes in the majority of the 33 cancers (Figure 8C). Therefore, the results of the pan-cancer analysis suggest that SNRNP70 may also play a role in other cancer types by affecting the TIME.
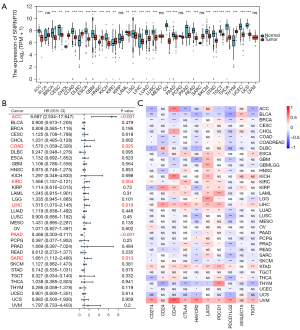
Discussion
APA is an RNA-processing mechanism that allows a protein-coding gene with more than one PAS to encode multiple mRNAs with different 3' ends (28-30). Recently, new evidence has uncovered a vital role of APA in many human cancers. Several APA-related genes have been identified as important regulators and prognostic indicators for a variety of cancers (11,31); however, few studies have assessed the role of APA regulators in the TIME of ccRCC. In this study, the expression of 36 key APA regulators in ccRCC was under researched and two groups with different characteristics were identified by using consensus clustering methods. Furthermore, SNRNP70 has been identified as a potential immune infiltration regulator.
Immune cell infiltration and angiogenesis are crucial characteristics of TIME in ccRCC. According to pan-cancer analyses, a prominent inflammatory feature of ccRCC is its high degree of T-cell infiltration (32-34). In most ccRCCs, CD8+ tumor-infiltrating T lymphocytes (TILs) are highly infiltrated, which increases the effectiveness of ICI (35). M2-like TAMs, Tregs, MDSCs, and NTLS-DCs in TIME make the TIME immunosuppressive in a proportion of ccRCC patients, and may therefore lead to the insensitivity of ccRCC to immunotherapy. Furthermore, ccRCC has abnormal neovascularization permeability, which limits Teff cell infiltration, resulting in an immunosuppressive microenvironment (36-38). Therefore, the discovery of predictive biomarkers and of new tools for monitoring immunotherapies are imperatively needed in all phases of ccRCC patients. In the era of innovative technologies and intelligence, immunotherapy drugs, and personalized therapy is at the horizon.
The heterogeneity of tumor cells has been a challenge in cancer treatment. Relevant attempts have been made to individualize patient treatment by subdividing cancers with heterogeneity into distinct subtypes. For example, in the study by Wang et al. patients with lung adenocarcinoma were classified into two subtypes with different prognoses based on immune-related gene expression characteristics. Patients with high-risk immune subtypes were found to have greater sensitivity to treatment with immune checkpoint inhibitors (39). In this study, a comprehensive analysis of immune infiltration showed that Cluster 2 possessed a mostly higher proportion of immune cells. In contrast, Cluster 1 exhibited a higher infiltration of NK CD56 bright cells, cytotoxic cells, and Tregs. The results herein suggest that ccRCC subtypes with generally higher immune cell infiltration have better overall and disease-specific survival. Moreover, APA regulators may represent an important genetic feature of ccRCC.
Based on the important role of APA in the TIME of ccRCC, unfavorable prognostic factors that were expressed at elevated levels in ccRCC and a positive correlation with CTLA4 expression (r>0.3) were identified. SNRNP70 is the only APA regulator that meets all the three conditions (worse OS; upregulated; positive with CTLA4) simultaneously. SNRNP70, a member of the spliceosome U1 snRNP, has been shown to play a key role in pre-mRNA splice site recognition and subsequent spliceosomal assembly (40,41). The study by Azam et al. developed a model in which the U1 snRNP component suppressed spliced lncRNAs in the nucleus by interacting with nuclear retention elements (42). Related studies have also demonstrated that SNRNP70 is associated with a variety of immune system diseases, including systemic lupus erythematosus, connective tissue diseases, and rheumatoid arthritis (43-45). In addition, patients with higher SNRNP70 expression had a distinctive composition of immune infiltrating cells. Additional pan-cancer analysis indicated a close relationship between SNRNP70 expression and immune checkpoint genes. The above results indicate that the SNRNP70 gene may represent a promising target for ccRCC immunotherapy and a potential prognostic marker.
However, few limitations are noted in this study. Firstly, 36 key APA regulators were selected for the present study based on previous studies. Therefore, some other APA regulators, may have been missed. Secondly, the current clustering type and ssGSEA analysis were based on TCGA data. These results require further reliable validation analyses with additional samples in the future. Finally, more fundamental experiments are required to investigate the potential link between APA and the TIME.
Conclusions
In summary, this study systematically analyzed the prognostic value of APA regulators in ccRCC and the correlation with CTLA4 and TIME. Consensus clustering of the expression profiles of APA regulatory factors identified two distinct clusters. These findings will aid in risk classification and the precise treatment of ccRCC patients. Among the 36 APA regulators, SNRNP70 was finally selected as an independent prognostic indicator for ccRCC patients. An additional pan-cancer analysis validated the close association between SNRNP70 expression and immune checkpoint genes. A series of clinical and basic experiments may help to improve the efficacy of cancer immunotherapy.
Acknowledgments
Thanks to all the researchers who participated.
Funding: The study was supported by Science Foundation of Wuhan Union Hospital (No. 900000332).
Footnote
Reporting Checklist: The authors have completed the REMARK reporting checklist. Available at https://tau.amegroups.com/article/view/10.21037/tau-22-565/rc
Conflicts of Interest: All authors have completed the ICMJE uniform disclosure form (available at https://tau.amegroups.com/article/view/10.21037/tau-22-565/coif). The authors have no conflicts of interest to declare.
Ethical Statement: The authors are accountable for all aspects of the work in ensuring that questions related to the accuracy or integrity of any part of the work are appropriately investigated and resolved. The study was conducted in accordance with the Declaration of Helsinki (as revised in 2013). Ethical approval was not required because the data used in the present study were obtained from public databases.
Open Access Statement: This is an Open Access article distributed in accordance with the Creative Commons Attribution-NonCommercial-NoDerivs 4.0 International License (CC BY-NC-ND 4.0), which permits the non-commercial replication and distribution of the article with the strict proviso that no changes or edits are made and the original work is properly cited (including links to both the formal publication through the relevant DOI and the license). See: https://creativecommons.org/licenses/by-nc-nd/4.0/.
References
- Sung H, Ferlay J, Siegel RL, et al. Global Cancer Statistics 2020: GLOBOCAN Estimates of Incidence and Mortality Worldwide for 36 Cancers in 185 Countries. CA Cancer J Clin 2021;71:209-49. [Crossref] [PubMed]
- Capitanio U, Bensalah K, Bex A, et al. Epidemiology of Renal Cell Carcinoma. Eur Urol 2019;75:74-84. [Crossref] [PubMed]
- Motzer RJ, Tannir NM, McDermott DF, et al. Nivolumab plus Ipilimumab versus Sunitinib in Advanced Renal-Cell Carcinoma. N Engl J Med 2018;378:1277-90. [Crossref] [PubMed]
- Hammers HJ, Plimack ER, Infante JR, et al. Safety and Efficacy of Nivolumab in Combination With Ipilimumab in Metastatic Renal Cell Carcinoma: The CheckMate 016 Study. J Clin Oncol 2017;35:3851-8. [Crossref] [PubMed]
- Motzer RJ, Escudier B, McDermott DF, et al. Nivolumab versus Everolimus in Advanced Renal-Cell Carcinoma. N Engl J Med 2015;373:1803-13. [Crossref] [PubMed]
- Choueiri TK, Larkin J, Oya M, et al. Preliminary results for avelumab plus axitinib as first-line therapy in patients with advanced clear-cell renal-cell carcinoma (JAVELIN Renal 100): an open-label, dose-finding and dose-expansion, phase 1b trial. Lancet Oncol 2018;19:451-60. [Crossref] [PubMed]
- Hoque M, Ji Z, Zheng D, et al. Analysis of alternative cleavage and polyadenylation by 3' region extraction and deep sequencing. Nat Methods 2013;10:133-9. [Crossref] [PubMed]
- Tian B, Manley JL. Alternative polyadenylation of mRNA precursors. Nat Rev Mol Cell Biol 2017;18:18-30. [Crossref] [PubMed]
- Chang JW, Yeh HS, Yong J. Alternative Polyadenylation in Human Diseases. Endocrinol Metab (Seoul) 2017;32:413-21. [Crossref] [PubMed]
- Zhang J, Sun W, Ren C, et al. A PolH Transcript with a Short 3'UTR Enhances PolH Expression and Mediates Cisplatin Resistance. Cancer Res 2019;79:3714-24. [Crossref] [PubMed]
- Xiong M, Chen L, Zhou L, et al. NUDT21 inhibits bladder cancer progression through ANXA2 and LIMK2 by alternative polyadenylation. Theranostics 2019;9:7156-67. [Crossref] [PubMed]
- Davis AG, Johnson DT, Zheng D, et al. Alternative polyadenylation dysregulation contributes to the differentiation block of acute myeloid leukemia. Blood 2022;139:424-38. [Crossref] [PubMed]
- Wang L, Lang GT, Xue MZ, et al. Dissecting the heterogeneity of the alternative polyadenylation profiles in triple-negative breast cancers. Theranostics 2020;10:10531-47. [Crossref] [PubMed]
- Zhong W, Wu Y, Zhu M, et al. Alternative splicing and alternative polyadenylation define tumor immune microenvironment and pharmacogenomic landscape in clear cell renal carcinoma. Mol Ther Nucleic Acids 2022;27:927-46. [Crossref] [PubMed]
- Tomczak K, Czerwińska P, Wiznerowicz M. The Cancer Genome Atlas (TCGA): an immeasurable source of knowledge. Contemp Oncol (Pozn) 2015;19:A68-77. [Crossref] [PubMed]
- The Genotype-Tissue Expression (GTEx) project. Nat Genet 2013;45:580-5. [Crossref] [PubMed]
- Jones J, Otu H, Spentzos D, et al. Gene signatures of progression and metastasis in renal cell cancer. Clin Cancer Res 2005;11:5730-9. [Crossref] [PubMed]
- Wilkerson MD, Hayes DN. ConsensusClusterPlus: a class discovery tool with confidence assessments and item tracking. Bioinformatics 2010;26:1572-3. [Crossref] [PubMed]
- Ginestet C. ggplot2: Elegant Graphics for Data Analysis. Journal of the Royal Statistical Society Series a-Statistics in Society 2011;174:245-.
- Wu T, Hu E, Xu S, et al. clusterProfiler 4.0: A universal enrichment tool for interpreting omics data. Innovation (Camb) 2021;2:100141. [Crossref] [PubMed]
- Hänzelmann S, Castelo R, Guinney J. GSVA: gene set variation analysis for microarray and RNA-seq data. BMC Bioinformatics 2013;14:7. [Crossref] [PubMed]
- Bindea G, Mlecnik B, Tosolini M, et al. Spatiotemporal dynamics of intratumoral immune cells reveal the immune landscape in human cancer. Immunity 2013;39:782-95. [Crossref] [PubMed]
- Gu Z, Eils R, Schlesner M. Complex heatmaps reveal patterns and correlations in multidimensional genomic data. Bioinformatics 2016;32:2847-9. [Crossref] [PubMed]
- Li JCA. Modeling survival data: Extending the Cox model. Sociological Methods & Research 2003;32:117-20. [Crossref]
- Biecek AKMKP. survminer: Drawing Survival Curves using 'ggplot2'. 0.4.9 ed2021.
- Jr FEH. rms: Regression Modeling Strategies. 2022.
- Blanche P, Dartigues JF, Jacqmin-Gadda H. Estimating and comparing time-dependent areas under receiver operating characteristic curves for censored event times with competing risks. Stat Med 2013;32:5381-97. [Crossref] [PubMed]
- Shen Y, Ji G, Haas BJ, et al. Genome level analysis of rice mRNA 3'-end processing signals and alternative polyadenylation. Nucleic Acids Res 2008;36:3150-61. [Crossref] [PubMed]
- Tian B, Hu J, Zhang H, et al. A large-scale analysis of mRNA polyadenylation of human and mouse genes. Nucleic Acids Res 2005;33:201-12. [Crossref] [PubMed]
- Danckwardt S, Hentze MW, Kulozik AE. 3' end mRNA processing: molecular mechanisms and implications for health and disease. EMBO J 2008;27:482-98. [Crossref] [PubMed]
- Xiang Y, Ye Y, Lou Y, et al. Comprehensive Characterization of Alternative Polyadenylation in Human Cancer. J Natl Cancer Inst 2018;110:379-89. [Crossref] [PubMed]
- Ock CY, Keam B, Kim S, et al. Pan-Cancer Immunogenomic Perspective on the Tumor Microenvironment Based on PD-L1 and CD8 T-Cell Infiltration. Clin Cancer Res 2016;22:2261-70. [Crossref] [PubMed]
- Şenbabaoğlu Y, Gejman RS, Winer AG, et al. Tumor immune microenvironment characterization in clear cell renal cell carcinoma identifies prognostic and immunotherapeutically relevant messenger RNA signatures. Genome Biol 2016;17:231. [Crossref] [PubMed]
- Yoshihara K, Shahmoradgoli M, Martínez E, et al. Inferring tumour purity and stromal and immune cell admixture from expression data. Nat Commun 2013;4:2612. [Crossref] [PubMed]
- Tang H, Wang Y, Chlewicki LK, et al. Facilitating T Cell Infiltration in Tumor Microenvironment Overcomes Resistance to PD-L1 Blockade. Cancer Cell 2016;29:285-96. [Crossref] [PubMed]
- Fukumura D, Kloepper J, Amoozgar Z, et al. Enhancing cancer immunotherapy using antiangiogenics: opportunities and challenges. Nat Rev Clin Oncol 2018;15:325-40. [Crossref] [PubMed]
- Georganaki M, van Hooren L, Dimberg A. Vascular Targeting to Increase the Efficiency of Immune Checkpoint Blockade in Cancer. Front Immunol 2018;9:3081. [Crossref] [PubMed]
- Hegde PS, Wallin JJ, Mancao C. Predictive markers of anti-VEGF and emerging role of angiogenesis inhibitors as immunotherapeutics. Semin Cancer Biol 2018;52:117-24. [Crossref] [PubMed]
- Wang Q, Li M, Yang M, et al. Analysis of immune-related signatures of lung adenocarcinoma identified two distinct subtypes: implications for immune checkpoint blockade therapy. Aging (Albany NY) 2020;12:3312-39. [Crossref] [PubMed]
- Pomeranz Krummel DA, Oubridge C, Leung AK, et al. Crystal structure of human spliceosomal U1 snRNP at 5.5 A resolution. Nature 2009;458:475-80. [Crossref] [PubMed]
- Kondo Y, Oubridge C, van Roon AM, et al. Crystal structure of human U1 snRNP, a small nuclear ribonucleoprotein particle, reveals the mechanism of 5' splice site recognition. Elife 2015;4:e04986. [Crossref] [PubMed]
- Azam S, Hou S, Zhu B, et al. Nuclear retention element recruits U1 snRNP components to restrain spliced lncRNAs in the nucleus. RNA Biol 2019;16:1001-9. [Crossref] [PubMed]
- Ibáñez-Costa A, Perez-Sanchez C, Patiño-Trives AM, et al. Splicing machinery is impaired in rheumatoid arthritis, associated with disease activity and modulated by anti-TNF therapy. Ann Rheum Dis 2022;81:56-67. [Crossref] [PubMed]
- Macri C, Wang F, Tasset I, et al. Modulation of deregulated chaperone-mediated autophagy by a phosphopeptide. Autophagy 2015;11:472-86. [Crossref] [PubMed]
- Paradowska-Gorycka A, Wajda A, Stolarek I, et al. Is the T-G-CT-G SNRNP70 haplotype another proof that mixed connective tissue disease is distinct from systemic lupus erythematosus and systemic sclerosis? A novel gene variant in SNRNP70 gene. Clin Exp Rheumatol 2019;37:32-40. [PubMed]