Construction and validation of a prognostic model for predicting clear cell renal cell carcinoma based on complement-related genes
Highlight box
Key findings
• We established and validated a prognostic survival model based on 5 complement-related genes in clear cell renal cell carcinoma (ccRCC) and thus developed a new predictive tool for clinical settings.
What is known and what is new?
• Previous studies have reported that accurate prediction models for patient prognosis that classify patients into high-risk and low-risk groups based on reliable predictive features can improve the ability of clinicians to make personalized treatment decisions.
• We were the first to develop and validate a survival prognostic model based on 5 complement-related genes in ccRCC and elucidate their relationships with tumor immune status. Our model can be used as a new predictive tool for clinical purposes.
What is the implication, and what should change now?
• Our novel, validated predictive model can be used to predict overall survival in ccRCC patients and may provide new therapeutic targets.
Introduction
Kidney cancer is the third most common malignancy of the genitourinary system worldwide (1). In 2020, more than 400,000 new cases of kidney cancer were diagnosed, and there were more than 175,000 kidney cancer-related deaths worldwide (1). Renal cell carcinoma (RCC) accounts for approximately 80% of all kidney cancers, and clear cell renal cell carcinoma (ccRCC) comprises 75% of all diagnosed RCC cases (2). CcRCC is the most common and aggressive subtype of RCC, and easily metastasizes to the lungs, liver, and bones via hematologic transport (2-4). The standard treatment for patients with surgically resectable RCC (i.e., those with stages T1, T2, and locally advanced disease) remains partial or radical nephrectomy, with a curative intention. Conversely, patients with inoperable or metastatic RCC usually receive systemic therapy with targeted agents and immune checkpoint inhibitors (ICIs). The 2 primary targeted therapies employed include vascular endothelial growth factor (VEGF)-targeted agents and mammalian target of rapamycin inhibitors (5-7). Currently, combination therapies based on ICIs (e.g., ICI-ICI, or ICI with VEGF-tyrosine kinase inhibitor) have shown remarkable efficacy as first-line therapies for metastatic RCC; however, some RCC patients develop resistance to such therapies (8-10). Thus, the identification of new prognostic features and additional therapeutic targets has important clinical implications for the prognosis and treatment of RCC patients.
In recent years, an increasing number of studies have confirmed that the tumor microenvironment (TME), which partially consists of complement proteins expressed by the cancer, stromal, and immune cells, affects the fate of cancer cells, thereby playing a central role in the development of cancer (11). Plasma proteins, cell surface receptors, and intracellular proteins, which are a part of the complement system (i.e., the complement cascade), also play key roles in the TME (12,13). In response to various stimuli, these proteins and regulatory factors are synthesized and secreted by a variety of cells, released into circulation, and then act in concert with receptors, which are expressed on different types of cell membranes, to play a key role in the innate immune defense of the body against pathogens and the maintenance of homeostasis in the host (12,14,15). When produced intracellularly, these complement effectors have atypical functions, are independent of the complement cascade and lead to locally occurring complement activation (16). Notably, parts of the complement system interact with the pathways and cells of both the innate and adaptive immune systems. The 3 complement activation pathways (i.e., the classical, lectin, and alternative pathways) respond to different stimuli, produce C3 (Complement C3) and C5 (Complement C5) convertases, resulting in the cleavage of C3 and C5, respectively, and eventually lead to the formation of the membrane attack complex. The cleavage products of complement proteins have regulatory effects, are biologically active, and induce allergic responses, chemotaxis, and phagocytosis (16). Complement system is likely to play opposite roles in various cancers, with both activation and deficiency of the complement system affecting tumor growth (16). According to our analysis, we found that a single clinical factor cannot accurately predict the prognosis of patients with ccRCC. Therefore, the combination of clinical factors and complement-related genes can improve the accuracy of prediction.
In this study, we identified 5 complement-related genes (i.e., A2M, APOBEC3G, COL4A2, DOCK4, and NOTCH4) by conducting a comprehensive analysis of messenger RNA expression data from the International Cancer Genome Consortium (ICGC) database and established a risk-score model and systematically evaluated its predictive ability for ccRCC patients. The predictive accuracy of the model was also externally validated using data from The Cancer Genome Atlas (TCGA). The correlation of the risk-score model with tumor-infiltrating immune cells and the genes in the risk-score model with drug sensitivity may provide new insights for personalized immunotherapy. We present the following article in accordance with the TRIPOD reporting checklist (available at https://tau.amegroups.com/article/view/10.21037/tau-23-187/rc).
Methods
Collection and analysis of publicly available data
Transcriptomic data and the corresponding clinical data were downloaded from the ICGC and TCGA databases. The ICGC data set comprised the data for 91 patients, and TCGA data set comprised the data for 531 patients. Among them, any patients with an overall survival (OS) of <30 days and for whom survival status information was missing were removed. Ultimately, the intact clinical data of 90 patients from the ICGC data set were used as the training group, and the data of 513 patients from TCGA data set were used as the validation group. A total of 317 complement-related genes were identified from the Molecular Signature Database (MSigDB) (https://www.gsea-msigdb.org/gsea/msigdb/index.jsp), and these genes were subsequently verified against the total genes contained in the ICGC and TCGA data sets, which resulted in 309 genes being retained for the downstream analyses. The study was conducted in accordance with the Declaration of Helsinki (as revised in 2013).
Construction of the risk-score model
A univariate Cox regression analysis was used to identify the genes that were significantly associated with OS (P<0.05) in the training and validation groups. After taking the intersection of the 2 genomes, a least absolute shrinkage and selection operator (LASSO)-Cox regression analysis was performed of the eligible complement-related genes in the training set to construct a prognostic risk signature. The risk score was calculated for each patient as follows:
where exprGENE is the expression level of the gene, and λGENE is the corresponding lambda value.
The patients were divided into high-risk and low-risk groups according to the designated median. A Kaplan-Meier (K-M) survival analysis was conducted to analyze the OS rates of the training and validation groups.
Differential expression analysis
The expression of the identified genes was compared between the 91 tumor samples and 45 normal adjacent tissues in the training group and then also compared between the 531 tumor samples and 72 normal adjacent tissues in the validation group. The genes with an adjusted P<0.05 and a |log fold change (FC)| <0.5 were identified as downregulated genes. The differential analysis results were illustrated using the “pheatmap” R package. The volcano maps were constructed using the “ggplot2” R package. The Wilcox test was used to compare the expression of the 5 complement-related genes (i.e., A2M, APOBEC3G, COL4A2, DOCK4, and NOTCH4) between the tumor and normal adjacent tissues.
Enrichment analysis
A Gene Ontology (GO) (17) functional enrichment analysis, which includes the biological processes (BPs), molecular functions (MFs), and cellular components (CCs), is commonly conducted in large-scale functional enrichment analyses. The Kyoto Encyclopedia of Genes and Genomes (KEGG) (18) database stores information about the genomic and biological pathways. We performed a GO functional enrichment analysis and a KEGG pathway enrichment analysis using the DAVID portal (https://david.ncifcrf.gov/summary.jsp) after identifying the genes most associated with the risk scores. A false discovery rate (FDR) <0.05 was considered statistically significant.
Nomogram construction
To facilitate the clinical application of the complement-related prognostic prediction models, we constructed individualized prediction models using data from the training group. The analysis was performed using the “rms package” in R. In the multiple regression model, the nomogram was used to characterize multiple variables, and the probability of an event occurring was predicted by calculating the total score. The region above the line served as the scoring system, while the region below the line served as the prediction system. We incorporated the risk score and tumor stage into the individualized prediction model. The actual results were also evaluated using the actual and predicted probabilities of the model in a calibration graph. The 1-, 2-, 3-, and 5-year survival of the ccRCC patients was able to be accurately predicted by the sum of the factor scores. Calibration curves and concordance index (C-index) values were used to show the accuracy of the survival prediction.
Single-cell data set analysis
Tumor Immune Single-cell Hub 2 (TISCH2) (http://tisch.comp-genomics.org/) is a single-cell RNA-sequencing (scRNA-seq) database that focuses on the TME. We searched the Gene Expression Omnibus (GEO) database (https://www.ncbi.nlm.nih.gov/geo/) using key words, such as “real cell carcinoma” and “Homo sapiens” and found the GSE159115 gene set, which is scRNA-seq analysis of ~30,000 cells dissociated from benign human kidney and renal tumor specimens. We used the GSE159115 gene set from the TISCH2 website (at http://tisch.comp-genomics.org/), a single-cell sequencing data set, to analyze the expression of the 5 different complement-related genes in different cells.
Immune-infiltration analysis
The TME is an integrated system consisting mainly of tumor cells, surrounding immune and inflammatory cells, mesenchymal cells, and various cytokines and chemokines (19). According to previous study, analyses of immune cell infiltration in tumor tissues are important for understanding the pathogenesis of a disease and predicting patient prognosis (20).
In the present study, we predicted the proportion of 22 infiltrating immune cell types from the training and validation groups using CIBERSORT analysis. For each sample, the sum of all the evaluated immune cell type scores was equal to 1. The effect of our model on immune cell infiltration was verified by analyzing the expression of the genes in the immune cells in the immune microenvironment.
Drug sensitivity analysis
Gene Set Cancer Analysis (GSCA) (http://bioinfo.life.hust.edu.cn/GSCA/#/) is a comprehensive platform for genomic, pharmacogenomic and immunogenomic cancer analyses. A genomic drug resistance analysis was carried out using the half maximal inhibitory concentration (IC50) values found in the Genomics of Drug Sensitivity in Cancer (GDSC) database. A Spearman correlation analysis was performed to determine the correlation between gene expression and drug sensitivity. A positive correlation and high gene expression indicated resistance to a drug, and vice versa.
Statistical analysis
The statistical analysis was performed using R (https://www.r-project.org/, v3.5.0) and GraphPad Prism (version 8.0, La Jolla, CA, USA). K-M and Cox analyses were conducted to identify the prognostic-related genes. The enrichment analysis was performed using the DAVID portal. The differential expression analysis was performed using the Wilcox test. C-index >0.71 was considered to be good accuracy and P value <0.05 was considered significant.
Results
Identification of the 5 complement-related genes for predicting the OS of ccRCC patients
We compared 91 samples of tumor tissues and 45 samples of normal tissues in the training group and detected 125 differentially expressed genes, of which 93 were upregulated and 32 were downregulated (Figure 1A). We also compared 531 tumor tissue samples and 72 normal tissue samples in the validation group and detected 113 differentially expressed genes, of which 94 were upregulated and 19 were downregulated. Next, a univariate Cox regression analysis was conducted and 35 genes were screened in the training group, and 92 genes were screened in the validation group (P<0.05). Additionally, 13 genes were obtained by intersecting the results of the 2 cohorts (Figure 1B). Finally, a LASSO-Cox regression analysis was performed of these 13 genes to obtain the optimal results, and 5 complement-related genes were identified; that is, A2M, APOBEC3G, COL4A2, DOCK4, and NOTCH4. Based on their corresponding λ values, the OS risk score was calculated for each ccRCC patient (Figure 1C).

Clinicopathological characteristics of the 5 complement-related genes
By analyzing the expression of the genes in the tumors and adjacent tissues, we found that A2M, APOBEC3G, COL4A2, DOCK4, and NOTCH4 were significantly more highly expressed in the tumor tissues than the normal tissues in both the training and validation groups (Figure 1D,1E). To examine the relationship between gene expression and the prognosis of ccRCC patients, based on the risk score, the median survival time was used to divide the patients into a low-risk group and a high-risk group, and we found that the ccRCC patients in the different risk groups had different clinicopathological characteristics. In both the training and validation groups, as the risk score increased, the OS of the patients decreased, and the expression of the 5 complement-related genes was also downregulated (Figure 1F). To further validate the prognostic significance of the risk score calculated based on the 5 complement-related genes, we performed a K-M analysis. As expected, in the training group, the prognosis of patients in the high-risk group was significantly worse than that of patients in the low-risk group (P<0.05), and similar results were obtained in the validation group (P<0.05) (Figure 1G).
Expression of the 5 complement-related genes
We next performed a single-cell sequencing analysis of the GSE159115 gene set (Figure 2A) on the TISCH website. The results showed that these genes were highly expressed in various types of immune cells. Among them, A2M and COL4A2 were highly expressed in endothelial cells, erythroblasts, and pericytes; APOBEC3G was highly expressed in CD8+T cells; while DOCK4 and NOTCH4 were highly expressed in endothelial cells (Figure 2B). These results suggested that our model was associated with tumor-infiltrating immune cells.
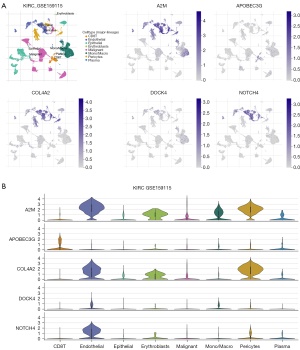
Gene-function enrichment analysis
We investigated the potential mechanism of the risk score in RCC progression. GO and KEGG functional enrichment analyses were performed to explore the biological functions and pathways associated with the risk scores. The GO functional enrichment analysis examined the BPs, MFs, and CCs. After identifying the genes most associated with the risk scores, GO and KEGG analyses were performed on these genes.
In the training group, the BPs most associated with the risk scores included the positive regulation of cell migration, angiogenesis, the regulation of small GTPase-mediated signal transduction, actin cytoskeleton organization, the positive regulation of transcription from RNA polymerase II promoter, and the positive regulation of DNA-templated transcription. In addition, the most relevant CCs associated with the risk scores were the cytosol and cytoplasm. Finally, the most relevant MFs associated with the risk scores were protein binding, guanyl-nucleotide exchange factor activity, and protein-kinase binding (Figure 3A). The BPs, CCs, and MFs associated with the risk scores in the validation group were basically consistent with those in the training group (Figure 3B). The most relevant signaling pathways in the KEGG analysis in the training and validation groups included focal adhesion, pathways in cancer, and the Rap1 signaling pathway (Figure 3C,3D). The results of these enrichment analyses further revealed a strong relationship between the risk scores and tumor progression, such as cell migration, including the positive regulation of cell migration, angiogenesis, and the positive regulation of transcription. The genetic correlation analysis between the training and validation groups revealed positive correlations between the 5 complement-related genes (Figure 3E,3F).
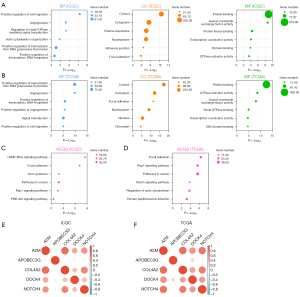
Construction and verification of the nomogram
To facilitate the clinical application of the prognostic and predictive models, we constructed individualized prediction models to predict the 1-, 2-, 3-, and 5-year OS of ccRCC patients (Figure 4A). We incorporated the risk score and tumor stage into the individualized prediction model. To confirm the predictive effect of the nomogram, we plotted a calibration curve. The calibration curve and the actual observations in the nomogram showed satisfactory overlap in both the training and validation group databases (Figure 4B). In addition, the C-index of the prediction mode was 0.795, while the C-index of tumor stage was 0.727, and the risk score was 0.713. The prediction model clearly had a higher prediction accuracy than the other items. Thus, we showed the validity of the prognostic nomogram in several ways.
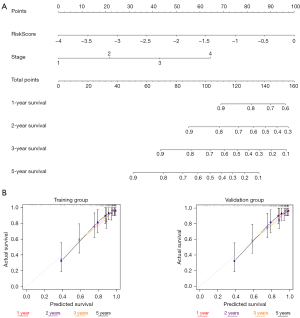
Immune cell infiltration analysis
The results of previous studies suggest that marker genes are closely associated with immune cells. Many studies have shown a close relationship between complement factors and the immune microenvironment (11). To further examine the landscape of the TME, we performed an immune cell infiltration analysis using the training and validation groups and found that M1-type macrophages were more reduced in the high-risk group than the low-risk group in both cohorts (Figure 5A,5B). In addition, the results of the Pearson correlation analysis showed that A2M, APOBEC3G, COL4A2, and NOTCH4 were positively correlated with M1-type macrophages in the training group, and A2M, APOBEC3G, DOCK4, and NOTCH4 were positively correlated with M1-type macrophages in the validation group (Figure 5C,5D).
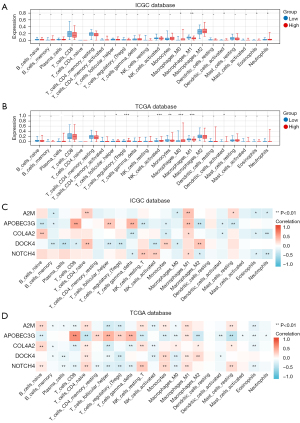
Immune cell infiltration is a common feature of most cancers, and tumor-associated macrophages have been found to be an important element of the TME in RCC (20,21). Macrophages in the TME usually exhibit M2-type features (22). M1-type macrophages are known as tumor cell killing and antigen presenting macrophages, while M2-type macrophages are involved in the promotion of cancer cell growth, invasion, angiogenesis, and the suppression of effective T-cell responses (23,24). TAM infiltration has been identified as a poor prognostic factor in a variety of cancers (25,26). The findings of these previous studies coupled with our analysis suggest that changes in the immune microenvironment of ccRCC patients may be complement factors related.
Drug sensitivity analysis
The drug sensitivity analysis of the GSCA data revealed that the high expression of DOCK4, COL4A2, and A2M simultaneously produced resistance to 10 drugs and small molecules. Among them, EKB-569 (pelitinib) and 5-fluorouraci are common anti-tumor drugs, while others, such as AICAR, CP466722, ispinesib mesylate, methotrexate, and NPK76-II-72, are molecular inhibitors. COL4A2, NOTCH4, A2M, and APOBEC3G were sensitive to some drugs and small molecules. Among them, COL4A2 was sensitive to TGX221, A2M was sensitive to AZ628, SB590885, Dabrafenib, and PLX4720, and APOBEC3G was sensitive to CP466722, ispinesib mesylate, methotrexate, and other molecular inhibitors. These results are consistent with our previous analysis. These genes may serve as potential biomarkers for drug screenings (Figure 6).
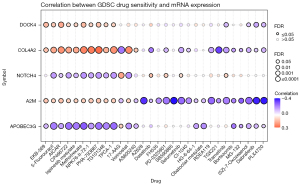
Discussion
The complement system is an important component of the TME and based on its involvement in immune surveillance and microbial defense, the complement system has long been considered to have an active and beneficial role in the fight against malignant cells (13,27). For example, the discovery of regulatory proteins and effectors on the surface of various tumor cells suggests that the complement system is heavily activated in the TME. Complement-dependent cytotoxicity acts synergistically with tumor-targeted antibody therapy (16,27). However, a growing number of studies have shown the contradictory role of the complement system in tumors, with both complement system activation and complement system deficiency promoting tumor growth (13,28). For example, complement system activation has been shown to exert tumor-promoting effects through sustained local T-cell immunosuppression and chronic inflammation, ultimately promoting tumor immune escape, growth, and distant metastasis (29-31); however, in hematologic malignancies, complement-dependent cytotoxicity has been shown to promote tumor cell apoptosis (32). Indeed, the complement system is involved in a variety of processes, such as synaptic maturation, immune complex clearance, angiogenesis, hematopoietic stem/progenitor cell mobilization, tissue regeneration and lipid metabolism, as well as the clearance of microorganisms (27). Most of these studies focused on animal models and in vitro studies, which has led to different and sometimes conflicting conclusions. Various studies have shown that complement system activation in different TME settings has diverse effects on tumor and clinical prognosis, ranging from anti-tumor defense to tumor promotion (33,34). Such findings are important for the design of effective therapeutic strategies that target complement components and their signaling pathways. Thus, we believe that the most appropriate therapeutic approach for activating or neutralizing the complement system will depend on the TME.
RCC includes a heterogeneous group of malignancies with increasingly well-defined genomic and clinical features. The exploration of potential biomarkers for tumors and their microenvironments will be the beginning of a continued push for new diagnostic and therapeutic approaches. Large-scale genome sequencing efforts have also led to advances in the accurate prediction of RCC (35), and there is an urgent need for predictive biomarkers to be identified to guide clinical decision making. Over the past few years, risk models have been continuously developed by integrating genomic data, but such models have not yet been routinely implemented in clinical decision making. Predictive tools that integrate biomarker data may help clinicians to develop personalized treatment plans based on different biological characteristics (36).
Previous studies have found that the accurate prediction of prognosis by classifying patients into high-risk and low-risk groups based on reliable predictive features can improve clinicians’ ability to implement personalized treatment decisions (37,38). In this study, we comprehensively and systematically analyzed patient characteristics based on the 5 complement-related genes, and further examined their practical clinical significance. First, we retrieved a total of 317 complement-related genes and identified the prevalent expression differences among these genes in both cohorts. We then conducted a univariate Cox analysis to identify the genes significantly associated with OS. Next, 13 genes were identified after intersecting the 2 data sets and conducting a LASSO-Cox analysis of the training cohort. Ultimately, 5 complement-related genes (i.e., A2M, APOBEC3G, COL4A2, DOCK4, and NOTCH4) were identified and used to construct a risk-score model. Our K-M survival analysis revealed that the model had good prediction accuracy. The prognosis of patients in the high-risk group was significantly lower than that of patients in the low-risk group, and the model was validated in both the training and validation groups. In addition, the nomogram was validated using the calibration curve and C-index, and satisfactory results were obtained, which also showed that our model could improve the prediction of overall survival time in the clinic. We found that M1-type macrophages were more reduced in the high-risk group than in the low-risk group by immune cell infiltration analysis. We also analyzed the biological functions and drug sensitivity of these 5 genes. Our findings will provide strong support for the exploration of new target genes and drug screenings.
In various tumor tissues, these five complement-related genes were produced by different cells and ultimately affect tumor progression. For example, the overexpression of DOCK4 has been shown to promote migration and invasion in prostate cancer cells (39), APOBEC3G has been shown to promote colorectal liver cancer metastasis by suppressing mir-29-mediated matrix metalloproteinase-2 inhibition (40), ADAMTS1 and A2M downregulation has been shown that activating epithelial-mesenchymal transition and altering the immune microenvironment to promote lung adenocarcinoma metastasis (41), NOTCH4 has been shown to have pro-proliferative, anti-apoptotic, and pro-migratory effects on lung adenocarcinoma cells (42), and COL4A2 has been shown to activate the RhoA/ROCK pathway to promote the invasion and migration of chicken hepatocellular carcinoma cell line (LMH) cells (43). These previous studies validate the reliability of our established model to some extent.
This study is the first to analyze the prognosis of ccRCC by complement-related genes, and to establish a prognosis model associated with OS by fewer genes. However, our findings had some limitations. First, the complex mechanisms of each immune cell and treatment resistance need to be explored further. Second, while the model can be used to predict OS, it needs further validation in clinical patients.
Conclusions
In summary, we developed and validated a prognostic survival model based on 5 complement-related genes in ccRCC and verified the predictive accuracy of the model. We further explored the biological function and the relationship with the TME and performed a drug sensitivity analysis. In brief, our study has developed a new predictive tool for use in clinical settings.
Acknowledgments
Funding: This work was sponsored by the National Natural Science Foundation of China (Nos. 81974391, 82173265, and 82173357), the Clinical Research Plan of SHDC (No. SHDC2020CR4025), the Leading Health Talents of Shanghai Municipal Health Commission (No. 2022LJ002), the Hospital Funded Clinical Research, Xin Hua Hospital Affiliated to Shanghai Jiao Tong University School of Medicine (No. 21XHDB06), and the Shanghai Natural Science Foundation (No. 20ZR1449600).
Footnote
Reporting Checklist: The authors have completed the TRIPOD reporting checklist. Available at https://tau.amegroups.com/article/view/10.21037/tau-23-187/rc
Peer Review File: Available at https://tau.amegroups.com/article/view/10.21037/tau-23-187/prf
Conflicts of Interest: All authors have completed the ICMJE uniform disclosure form (available at https://tau.amegroups.com/article/view/10.21037/tau-23-187/coif). The authors have no conflicts of interest to declare.
Ethical Statement: The authors are accountable for all aspects of the work, including ensuring that any questions related to the accuracy or integrity of any part of the work have been appropriately investigated and resolved. The study was conducted in accordance with the Declaration of Helsinki (as revised in 2013).
Open Access Statement: This is an Open Access article distributed in accordance with the Creative Commons Attribution-NonCommercial-NoDerivs 4.0 International License (CC BY-NC-ND 4.0), which permits the non-commercial replication and distribution of the article with the strict proviso that no changes or edits are made and the original work is properly cited (including links to both the formal publication through the relevant DOI and the license). See: https://creativecommons.org/licenses/by-nc-nd/4.0/.
References
- Sung H, Ferlay J, Siegel RL, et al. Global Cancer Statistics 2020: GLOBOCAN Estimates of Incidence and Mortality Worldwide for 36 Cancers in 185 Countries. CA Cancer J Clin 2021;71:209-49. [Crossref] [PubMed]
- Escudier B, Porta C, Schmidinger M, et al. Renal cell carcinoma: ESMO Clinical Practice Guidelines for diagnosis, treatment and follow-up†. Ann Oncol 2019;30:706-20. [Crossref] [PubMed]
- Chevrier S, Levine JH, Zanotelli VRT, et al. An Immune Atlas of Clear Cell Renal Cell Carcinoma. Cell 2017;169:736-749.e18. [Crossref] [PubMed]
- Lindblad P. Epidemiology of renal cell carcinoma. Scand J Surg 2004;93:88-96. [Crossref] [PubMed]
- Braun DA, Bakouny Z, Hirsch L, et al. Beyond conventional immune-checkpoint inhibition - novel immunotherapies for renal cell carcinoma. Nat Rev Clin Oncol 2021;18:199-214. [Crossref] [PubMed]
- Pal K, Madamsetty VS, Dutta SK, et al. Synchronous inhibition of mTOR and VEGF/NRP1 axis impedes tumor growth and metastasis in renal cancer. NPJ Precis Oncol 2019;3:31. [Crossref] [PubMed]
- Rini BI, Campbell SC, Escudier B. Renal cell carcinoma. Lancet 2009;373:1119-32. [Crossref] [PubMed]
- Motzer RJ, Tannir NM, McDermott DF, et al. Nivolumab plus Ipilimumab versus Sunitinib in Advanced Renal-Cell Carcinoma. N Engl J Med 2018;378:1277-90. [Crossref] [PubMed]
- Rini BI, Plimack ER, Stus V, et al. Pembrolizumab plus Axitinib versus Sunitinib for Advanced Renal-Cell Carcinoma. N Engl J Med 2019;380:1116-27. [Crossref] [PubMed]
- Motzer RJ, Penkov K, Haanen J, et al. Avelumab plus Axitinib versus Sunitinib for Advanced Renal-Cell Carcinoma. N Engl J Med 2019;380:1103-15. [Crossref] [PubMed]
- Pio R, Ajona D, Ortiz-Espinosa S, et al. Complementing the Cancer-Immunity Cycle. Front Immunol 2019;10:774. [Crossref] [PubMed]
- Arbore G, West EE, Spolski R, et al. T helper 1 immunity requires complement-driven NLRP3 inflammasome activity in CD4+ T cells. Science 2016;352:aad1210. [Crossref] [PubMed]
- Markiewski MM, DeAngelis RA, Benencia F, et al. Modulation of the antitumor immune response by complement. Nat Immunol 2008;9:1225-35. [Crossref] [PubMed]
- Bekassy Z, Lopatko Fagerström I, Bader M, et al. Crosstalk between the renin-angiotensin, complement and kallikrein-kinin systems in inflammation. Nat Rev Immunol 2022;22:411-28. [Crossref] [PubMed]
- Noris M, Remuzzi G. Overview of complement activation and regulation. Semin Nephrol 2013;33:479-92. [Crossref] [PubMed]
- Ricklin D, Hajishengallis G, Yang K, et al. Complement: a key system for immune surveillance and homeostasis. Nat Immunol 2010;11:785-97. [Crossref] [PubMed]
- Gene Ontology Consortium. going forward. Nucleic Acids Res 2015;43:D1049-56. [Crossref] [PubMed]
- Kanehisa M, Araki M, Goto S, et al. KEGG for linking genomes to life and the environment. Nucleic Acids Res 2008;36:D480-4. [Crossref] [PubMed]
- Berlato C, Khan MN, Schioppa T, et al. A CCR4 antagonist reverses the tumor-promoting microenvironment of renal cancer. J Clin Invest 2017;127:801-13. [Crossref] [PubMed]
- Sica A, Schioppa T, Mantovani A, et al. Tumour-associated macrophages are a distinct M2 polarised population promoting tumour progression: potential targets of anti-cancer therapy. Eur J Cancer 2006;42:717-27. [Crossref] [PubMed]
- Wang C, Wang Y, Hong T, et al. Targeting a positive regulatory loop in the tumor-macrophage interaction impairs the progression of clear cell renal cell carcinoma. Cell Death Differ 2021;28:932-51. [Crossref] [PubMed]
- Kovaleva OV, Samoilova DV, Shitova MS, et al. Tumor Associated Macrophages in Kidney Cancer. Anal Cell Pathol (Amst) 2016;2016:9307549. [Crossref] [PubMed]
- Biswas SK, Mantovani A. Macrophage plasticity and interaction with lymphocyte subsets: cancer as a paradigm. Nat Immunol 2010;11:889-96. [Crossref] [PubMed]
- Cannarile MA, Ries CH, Hoves S, et al. Targeting tumor-associated macrophages in cancer therapy and understanding their complexity. Oncoimmunology 2014;3:e955356. [Crossref] [PubMed]
- Komohara Y, Hasita H, Ohnishi K, et al. Macrophage infiltration and its prognostic relevance in clear cell renal cell carcinoma. Cancer Sci 2011;102:1424-31. [Crossref] [PubMed]
- Izumi K, Fang LY, Mizokami A, et al. Targeting the androgen receptor with siRNA promotes prostate cancer metastasis through enhanced macrophage recruitment via CCL2/CCR2-induced STAT3 activation. EMBO Mol Med 2013;5:1383-401. [Crossref] [PubMed]
- Markiewski MM, Lambris JD. Is complement good or bad for cancer patients? A new perspective on an old dilemma. Trends Immunol 2009;30:286-92. [Crossref] [PubMed]
- Bonavita E, Gentile S, Rubino M, et al. PTX3 is an extrinsic oncosuppressor regulating complement-dependent inflammation in cancer. Cell 2015;160:700-14. [Crossref] [PubMed]
- Taylor RP, Lindorfer MA. Cytotoxic mechanisms of immunotherapy: Harnessing complement in the action of anti-tumor monoclonal antibodies. Semin Immunol 2016;28:309-16. [Crossref] [PubMed]
- Vadrevu SK, Chintala NK, Sharma SK, et al. Complement c5a receptor facilitates cancer metastasis by altering T-cell responses in the metastatic niche. Cancer Res 2014;74:3454-65. [Crossref] [PubMed]
- Pio R, Ajona D, Lambris JD. Complement inhibition in cancer therapy. Semin Immunol 2013;25:54-64. [Crossref] [PubMed]
- Reis ES, Mastellos DC, Ricklin D, et al. Complement in cancer: untangling an intricate relationship. Nat Rev Immunol 2018;18:5-18. [Crossref] [PubMed]
- Hijaze N, Ledersnaider M, Simanovich E, et al. Inducing regulated necrosis and shifting macrophage polarization with anti-EMMPRIN antibody (161-pAb) and complement factors. J Leukoc Biol 2021;110:343-56. [Crossref] [PubMed]
- Obradovic A, Chowdhury N, Haake SM, et al. Single-cell protein activity analysis identifies recurrence-associated renal tumor macrophages. Cell 2021;184:2988-3005.e16. [Crossref] [PubMed]
- Varela I, Tarpey P, Raine K, et al. Exome sequencing identifies frequent mutation of the SWI/SNF complex gene PBRM1 in renal carcinoma. Nature 2011;469:539-42. [Crossref] [PubMed]
- Liao Z, Yao H, Wei J, et al. Development and validation of the prognostic value of the immune-related genes in clear cell renal cell carcinoma. Transl Androl Urol 2021;10:1607-19. [Crossref] [PubMed]
- Zhong X, Wang L, Shao L, et al. Prognostic Nomogram for Rectal Cancer Patients With Tumor Deposits. Front Oncol 2022;12:808557. [Crossref] [PubMed]
- Zhao J, Wu C, Qiu T, et al. Establishment and validation of a nomogram for predicting the surgical difficulty of lateral retroperitoneal laparoscopic adrenalectomy. Transl Androl Urol 2023;12:9-18. [Crossref] [PubMed]
- Mei Y, Li K, Zhang Z, et al. miR-33b-3p Acts as a Tumor Suppressor by Targeting DOCK4 in Prostate Cancer. Front Oncol 2021;11:740452. [Crossref] [PubMed]
- Ding Q, Chang CJ, Xie X, et al. APOBEC3G promotes liver metastasis in an orthotopic mouse model of colorectal cancer and predicts human hepatic metastasis. J Clin Invest 2011;121:4526-36. [Crossref] [PubMed]
- Lee HC, Chang CY, Huang YC, et al. Downregulated ADAMTS1 Incorporating A2M Contributes to Tumorigenesis and Alters Tumor Immune Microenvironment in Lung Adenocarcinoma. Biology (Basel) 2022;11:760. [Crossref] [PubMed]
- Li X, Cao X, Zhao H, et al. Hypoxia Activates Notch4 via ERK/JNK/P38 MAPK Signaling Pathways to Promote Lung Adenocarcinoma Progression and Metastasis. Front Cell Dev Biol 2021;9:780121. [Crossref] [PubMed]
- Hu X, Tan S, Yin H, et al. Selenium-mediated gga-miR-29a-3p regulates LMH cell proliferation, invasion, and migration by targeting COL4A2. Metallomics 2020;12:449-59. [Crossref] [PubMed]
(English Language Editor: L. Huleatt)