Prediction of clinically significant prostate cancer using a novel 68Ga-PSMA PET-CT and multiparametric MRI-based model
Highlight box
Key findings
• This study developed a novel predictive nomogram incorporating the maximum standardized uptake value (SUVmax), Prostate Imaging Reporting and Data System (PI-RADS) categories, and prostate-specific antigen density (PSAd) that was capable of accurately detecting clinically significant prostate cancer (csPCa) prior to biopsy in a manner likely to offer clinical benefit.
What is known and what is new?
• To detect csPCa more reliably, several recent studies have explored the combined applications of Multiparametric magnetic resonance imaging (mpMRI) and 68Ga- prostate-specific membrane antigen (PSMA) positron emission tomography-computed tomography (PET-CT).
• Nomograms predicting csPCa based on the combination of mpMRI and 68Ga-PSMA PET-CT was constructed herein.
What is the implication, and what should change now?
• The newly constructed nomograms can be used by clinicians to gauge csPCa risk prior to unnecessary biopsies.
Introduction
There are many diagnostic technologies which have been developed to aid in the detection of prostate cancer (PCa). Serum prostate-specific antigen (PSA) level is the most utilized biomarker in screening for PCa, whereas the drawback is the high rate of false-positive (1). Multiparametric magnetic resonance imaging (mpMRI) of the prostate and its Prostate Imaging Reporting and Data System (PI-RADS) (2) have been widely accepted as tools for the routine management of individuals with suspected or confirmed diagnoses of PCa (3). It is an effective image examination method with relatively high accuracy in tumor detection and stage determination (4,5). However, up to 16% of clinically significant prostate cancer (csPCa) cases may be overlooked by preoperative mpMRI, owing to the lack of visible suspect MRI target lesions prior to systematic biopsy (6). Moreover, debate remains pertaining to PI-RADS category 3 lesions.
68Ga-prostate-specific membrane antigen (PSMA) positron emission tomography-computed tomography (PET-CT) imaging, a recently developed molecular imaging approach, is of great value in detecting biochemical recurrence (7), lymph node metastases (8), and the first diagnosis of primary PCa (9). Compared with mpMRI, 68Ga-PSMA PET-CT offers greater sensitivity and comparable specificity in detecting primary PCa (9), while achieving higher PCa detection rate (69.77% vs. 36.14%) (10). However, not all PCa cells exhibit PSMA upregulation (11), which would make 68Ga-PSMA PET-CT fail to detect csPCa before the biopsy. In addition, PSMA uptake has been reported in cases of granulomatous disease, prostatitis, and benign prostatic hyperplasia (BPH), thus limiting the specificity of this imaging modality (12). In order to detect PCa more reliably, several recent studies have explored the combined use of mpMRI and 68Ga-PSMA PET-CT (13). However, there is still a lack of predictive model to predicting csPCa based on a combination of mpMRI and 68Ga-PSMA PET-CT. Here, we explored the maximum standardized uptake value (SUVmax) as a quantitative 68Ga-PSMA PET-CT parameter, together with mpMRI, to develop and validate a novel nomogram capable of readily and reliably predicting csPCa. We present this article in accordance with the TRIPOD Checklist (available at https://tau.amegroups.com/article/view/10.21037/tau-22-832/rc).
Methods
Patient selection
From September 2020 to June 2021, patients with suspected PCa consecutively enrolled in our outpatient clinics, and any participants who met at least one inclusion criteria were considered to be included in the study: (I) serum PSA level >4 ng/mL; (II) palpable nodules, induration or asymmetry on digital rectal examination; (III) abnormal prostate imaging findings. On the other hand, patients will be excluded if they meet any of the following criteria: (I) exhibited pathologically diagnosed with PCa and had undergone corresponding treatment; (II) had a history of drug use or procedures with the potential to impact serum PSA levels; (III) presented with MRI contraindications such as claustrophobia or the use of electronic, magnetic, or mechanical implants; (IV) were diagnosed via imaging with extracapsular PCa (T stage > T3a). An event per independent variable value of 10 was used as a rule of thumb for study size estimation. This study was registered at ClinicalTrials.gov with identifier: NCT05073653. The study was conducted in accordance with the Declaration of Helsinki (as revised in 2013). The Medical Ethics Committee of Xiangya Hospital Central South University approved the present prospective study (No. 201909253). Patients were informed of all study procedures and written informed consent was collected from all the included subjects prior to study participation.
Imaging and prostate biopsy
All patients enrolled in the present study underwent mpMRI followed by 68Ga-PSMA PET-CT within a 15-day interval. Detailed imaging protocols were detailed in our prior study (10). Two experienced genitourinary radiologists (X Yi and J Zhang, average 10 years of experience) independently reviewed all mpMRI images while blinded to patient clinical characteristics, and these mpMRI results were interpreted by PI-RADS guidelines. Discrepancies were resolved by consensus of the two radiologists. PET-CT results were interpreted based on the consensus of two experienced nuclear medicine physicians (YX Tang and SH Zhang, average 10 years of experience) blinded to both mpMRI results and patient clinical characteristics.
All systematic biopsies (SB) were conducted by a urologist blinded to imaging results [with experience of performing over 1,000 transperineal SB and 300 prostate targeted biopsy (TB) procedures] within 4 weeks following mpMRI and 68Ga-PSMA PET-CT imaging. All patients underwent a 12-core transperineal SB performed using a BK Fusion Biopsy System. Additionally, for patients with visible 68Ga-PSMA PET-CT lesions further underwent 68Ga-PSMA PET-CT-guided targeted biopsy (68Ga-PSMA PET-CT-TB). For patients who were considered with exhibition of suspicious lesions with a PI-RADS ≥3, they additionally underwent mpMRI guided targeted biopsy (mpMRI-TB). Before performing prostate biopsies, radiologists used the BK Fusion software (MIM 6.9, MIM Software Inc.) to label a maximum of 2 highest scoring lesions per patient. The biopsy sequence was as follows: 68Ga-PSMA PET-CT-TB, mpMRI-TB, and SB.
External validation cohort
We retrospectively collected data from patients who had undergone both 68Ga-PSMA PET-CT, mpMRI and 12-core transperineal SB in our Electronic Medical Records System. Inclusion criteria included: (I) serum PSA level or digital rectal examination abnormalities; (II) abnormal prostate imaging findings. Patients were excluded if they: (I) were pathologically confirmed PCa and had undergone corresponding treatment; (II) had a history of drug use or procedures with the potential to impact serum PSA levels; (III) presented with MRI contraindications; and (IV) and with imaging indicated PCa with stage > T4. Totally, 61 patients were enrolled to validate the model. Of these patients, 45 individuals were pathologically diagnosed with csPCa. Among them, 19 patients received mpMRI, and 68Ga-PSMA PET-CT before biopsy. Unlike the prospective cohort exhibited above, 42 patients in the external cohort underwent the procedures with sequence as follows: mpMRI, SB, and 68Ga-PSMA PET-CT. To avoid potential lesions affecting 68Ga-PSMA PET-CT, the interval between SB and subsequent 68Ga-PSMA PET-CT was beyond 2 weeks.
Data collection and definitions of terms
Demographic, clinical, and imaging characteristics were collected by Electronic Medical Record. The screening of patients and the collection of clinical data were managed using the Chestnut electronic data capture system. According to EAU guidelines, csPCa was defined as a Gleason score ≥3+4 (14), and non-csPCa was defined as PCa with a Gleason score of 3+3. Cases of prostatitis, high-grade prostatic intraepithelial neoplasia, atypical small acinar proliferation, and BPH were considered as non-tumor cases. Pathological classification was dependent on biopsy specimens.
Statistical analyses
Categorical variables were displayed as frequencies (percentages) which were analyzed using the Chi-squared test or Fisher’s exact test. Continuous variables were given as the medians with interquartile ranges and compared using the Mann-Whitney U test. Significant independent predictors of csPCa status were identified through univariate and multivariate logistic regression analyses, with those predictors that were significant (P<0.05) in univariate analyses being included in multivariate analyses. We used logical regression to develop a prediction model with the selected predictors and draw a nomogram. The diagnostic performance of the developed nomogram and associated variables was assessed using a receiver operating characteristic (ROC) curve approach with the R pROC package. Calibration curves were then used with 1,000 bootstrap iterations to assess rate of csPCa prediction. Decision curve analysis (DCA) was additionally conducted using the R ggDCA package to gauge the value of this model in routine clinical practice. For model validation, predictions were calculated by the formula of the evaluated prediction model. The ROC curve and calibration curves were used to evaluate the predictive model in the external validation cohort. A two-sided P<0.05 was the threshold of significance, and all figure generation and analyses were performed using R version 4.0.4.
Results
Patient selection
In total, 112 patients who underwent mpMRI and 68Ga-PSMA PET-CT at our Hospital between September 2020 and June 2021 were recruited. The flowchart of the research procedure was displayed in Figure S1. Of these patients, 83 underwent mpMRI-TB+SB, and 43 underwent 68Ga-PSMA PET-CT-TB+SB.
Based on the pathological result of biopsy, 34 patients were diagnosed with csPCa. The detailed baseline characteristics of enrolled patients are summarized in Table 1. No significant differences in baseline body mass index (BMI), comorbidities, smoking status, or history of alcohol intake were observed between csPCa and the other patient cohorts (P>0.05). On the other hand, patients with csPCa had higher tPSA and prostate-specific antigen density (PSAd), SUVmax relative to non-csPCa or non-tumor patients (15.4 vs. 8.4, 0.5 vs. 0.2, and 11.8 vs. 0.0, respectively, all P<0.05).
Table 1
Characteristics | Overall (N=112) | Non-csPCa or non-tumor (N=78) | csPCa (N=34) | P value |
---|---|---|---|---|
Age (years) | 64.0 [59.0, 70.0] | 64.0 [58.0, 69.0] | 66.0 [64.0, 70.8] | 0.007 |
BMI (kg/m2) | 24.1 [21.7, 25.8] | 24.1 [22.0, 25.6] | 24.1 [21.7, 26.6] | 0.769 |
tPSA (ng/mL) | 11.1 [6.2, 15.7] | 8.4 [5.5, 14.2] | 15.4 [11.5, 32.3] | <0.001 |
Comorbidity | ||||
Hypertension | 39 (35.1) | 25 (32.1) | 14 (42.4) | 0.385 |
Diabetes | 12 (10.8) | 8 (10.3) | 4 (12.1) | 0.748 |
CHD | 8 (7.2) | 7 (9.0) | 1 (3.0) | 0.432 |
Smoking | 57 (51.4) | 39 (50.0) | 18 (54.5) | 0.683 |
Drinking | 47 (42.3) | 30 (38.5) | 17 (51.5) | 0.215 |
PSAd (ng/mL2) | 0.2 [0.1, 0.4] | 0.2 [0.1, 0.3] | 0.5 [0.3, 0.8] | <0.001 |
Prostate volume (mL) | 43.0 [29.5, 68.5] | 48.6 [31.4, 74.0] | 35.5 [24.1, 48.6] | 0.015 |
PI-RADS | <0.001 | |||
1 | 18 (16.1) | 14 (17.9) | 4 (11.8) | |
2 | 11 (9.8) | 11 (14.1) | 0 (0.0) | |
3 | 42 (37.5) | 39 (50.0) | 3 (8.8) | |
4 | 15 (13.4) | 8 (10.3) | 7 (20.6) | |
5 | 26 (23.2) | 6 (7.7) | 20 (58.8) | |
SUVmax | 6.0 [0.0, 9.4] | 0.0 [0.0, 6.2] | 11.8 [8.2, 18.5] | <0.001 |
Data are shown as n (%) or median [IQR]. Non-csPCa, non-clinically significant prostate cancer; csPCa, clinically significant prostate cancer; IQR, interquartile range; BMI, body mass index; tPSA, total prostate-specific antigen; CHD, coronary heart disease; PSAd, prostate-specific antigen density; PI-RADS, Prostate Imaging Reporting and Data System; SUVmax, maximum standard uptake value.
Determination of csPCa predictors
In the univariate analyses, age, tPSA, PSAd, PI-RADS category, and SUVmax were all significantly associated with csPCa status (P<0.05, Table 2). Subsequent multivariate analyses indicated that PI-RADS (PI-RADS 4 OR: 6.02, 95% CI: 1.11–32.49, P=0.037; PI-RADS 5 OR: 8.34, 95% CI: 1.84–37.90, P=0.006; with PI-RADS 3 as a reference), and SUVmax (OR: 1.28, 95% CI: 1.11–1.47, P<0.001) were predictors of csPCa in Table 2.
Table 2
Characteristics | Univariate regression analysis | Multivariate regression analysis | |||||
---|---|---|---|---|---|---|---|
OR | 95% CI | P value | OR | 95% CI | P value | ||
Age (years) | 1.08 | 1.02–1.15 | 0.008 | 0.99 | 0.90–1.10 | 0.917 | |
BMI (kg/m2) | 1.04 | 0.90–1.21 | 0.609 | ||||
tPSA (ng/mL) | 1.12 | 1.06–1.17 | <0.001 | 1.06 | 0.94–1.19 | 0.330 | |
Hypertension | 1.56 | 0.68–3.61 | 0.297 | ||||
Diabetes | 1.21 | 0.34–4.32 | 0.773 | ||||
CHD | 0.32 | 0.04–2.68 | 0.292 | ||||
Smoking | 1.20 | 0.53–2.71 | 0.662 | ||||
Drinking | 1.70 | 0.75–3.86 | 0.205 | ||||
Prostate volume (mL) | 0.99 | 0.97–1.00 | 0.117 | ||||
PSAd (ng/mL2) | 118.14 | 15.06–926.73 | <0.001 | 1.40 | 0.03–57.55 | 0.860 | |
PI-RADS 4 vs. PI-RADS≤3 | 8.00 | 2.22–28.77 | 0.001 | 6.02 | 1.11–32.49 | 0.037 | |
PI-RADS 5 vs. PI-RADS≤3 | 30.48 | 9.18–101.23 | <0.001 | 8.34 | 1.84–37.90 | 0.006 | |
SUVmax | 1.34 | 1.18–1.51 | <0.001 | 1.28 | 1.11–1.47 | <0.001 |
OR, odds ratio; CI, confidence interval; BMI, Body mass index; tPSA, total prostate-specific antigen; CHD, coronary heart disease; PSAd, prostate-specific antigen density; PI-RADS, Prostate Imaging Reporting and Data System; SUVmax, maximum standard uptake value.
Diagnostic efficacy of clinical indicators
Then, we compared the diagnostic ability of PSAd, PI-RADS category and SUVmax to detect csPCa under their optimal cutoff values (Table S1). Remarkably, SUVmax exhibited the strongest ability to screen csPCa, with the highest area under the curve (AUC) (0.903; 95% CI: 0.846–0.960). Besides, the sensitivity of SUVmax (0.912; 95% CI: 0.763–0.981) was significantly higher than that of PSAd (0.676; 95% CI: 0.495–0.826) and PI-RADS (0.794; 95% CI: 0.621–0.913). In contrast, SUVmax had a moderate specificity of 0.795 (95% CI: 0.688–0.878) which was similar with PSAd (0.859; 95% CI: 0.762–0.927) and PI-RADS (0.821; 95% CI: 0.717–0.898). Thus, the positive predictive value of SUVmax was also relatively moderate (0.660; 95% CI: 0.507–0.791), which may lead to a higher false positive rate of csPCa and unnecessary prostate biopsies.
Predictive model construction
Although SUVmax generally showed excellent diagnostic performance for identifying csPCa with optimal AUC, sensitivity and negative predictive values, the specificity and positive predictive values were still not satisfactory. In order to more accurately identify patients with csPCa and avoid false positive cases, we determined to construct a clinical prediction model, with an attempt to combine the diagnostic advantages of mpMRI and 68Ga-PSMA PET-CT.
Based on the results of multivariate logistic analyses above, PI-RADS and SUVmax were determined as important predictive indicators for csPCa. According to recent literatures, PSAd is an important predictive indicator for csPCa compared to traditional tPSA, and has a broader prospect. The strategy of mpMRI combined with PSAd can effectively detect PI-RADS 1–3 cases and tumors in peripheral zone lesions with extremely high negative predictive values (15-17). Therefore, we subsequently compared two schemes of the prediction model, PI-RADS + SUVmax + PSAd and PI-RADS + SUVmax. As shown in Figure S2, the prediction models have similar AUC areas (P=0.4303). Despite PSAd not demonstrating statistical significance in our multivariate logistic regression analysis, PSAd was also incorporated in the predictive model as an important clinical indicator.
In summary, in this study we utilized the clinical information of 112 participants as a training cohort to construct a predictive model including PI-RADS, SUVmax and PSAd, which can predict csPCa before biopsies. The formula for the predictive model is as follows:
Additionally, we have created a nomogram based on the predictive model, which is presented in Figure 1.
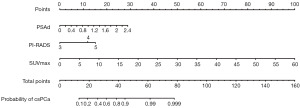
Performance of the predictive model
ROC curve analyses indicated that the predictive model exhibited a higher AUC (0.936, 95% CI: 0.888–0.984) when used to diagnose csPCa, relative to corresponding AUC for PI-RADS (0.806, 95% CI: 0.703–0.909) and PSAd (0.812, 95% CI: 0.719–0.905) alone (Figure 2A,2B). However, the AUC of predictive model was only slightly higher than that of SUVmax (0.903, 95% CI: 0.846–0.960) with no significant statistical difference (P=0.1541). In addition, the sensitivity and negative predictive values of the predictive model were 0.882 (95% CI: 0.725–0.967) and 0.910 (95% CI: 0.824–0.963), respectively. It is worth noting that the predictive model resulted in a good specificity (0.910, 95% CI: 0.824–0.963) and positive predictive values (0.811, 95% CI: 0.648–0.920). Although the predictive model only slightly increased the AUC for diagnosing csPCa compared with SUVmax alone, it significantly improved the relatively moderate specificity and positive predictive values of SUVmax, which may reduce unnecessary prostate biopsy in clinical practice.
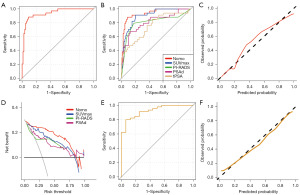
The model exhibited good calibration with a high C-index (0.947), R2 (0.714), D-index (0.722), and low Brier (0.087) values, and the mean absolute error was 0.028 (Figure 2C). As such, good agreement was observed between csPCa status as predicted by the model and from actual observations.
Next, a DCA approach was used to gauge the clinical net benefit of this predictive model (Figure 2D). The model exhibited the high net benefit for patients at threshold probabilities of 0–75%, suggesting that this model was of clinical capability on most occasions.
Model validation
To validate the developed predictive model, internal validation with 400 repetitions and five-fold cross-validation was performed. The model exhibited excellent performance under these conditions with respect to the mean AUC (0.940), R2 (0.577), D-index (0.551), and Brier (0.097) values. External validation was also conducted in a retrospective cohort including 61 patients from our center who underwent mpMRI, 68Ga-PSMA PET-CT, and SB. Baseline characteristics for patients in the external cohort are listed in Table S2. The model achieved good discrimination (Figure 2E) and calibration (Figure 2F) in the external validation cohort, with an AUC of 0.924 (95% CI: 0.857–0.990).
Subgroup analyses
To determine the populations that would be able to attain the greatest benefit from the nomogram, subgroup analyses were conducted to compare the predictive ability of this model and 68Ga-PSMA PET-CT, mpMRI, tPSA, or PSAd alone.
Compared with tPSA (AUC 0.611, 95% CI: 0.445–0.776) and PSAd (AUC 0.713, 95% CI: 0.558–0.868), the predictive model (AUC 0.889, 95% CI: 0.810–0.967) and SUVmax (AUC 0.875, 95% CI: 0.795–0.953) significantly improved csPCa diagnosis in PI-RADS category 3 subgroup (Figure 3A). The superiority of predictive model (AUC 0.855, 95% CI: 0.756–0.955) and SUVmax (AUC 0.874, 95% CI: 0.795–0.953) was also observed (Figure 3B) in individuals with tPSA levels ≤10 ng/mL.
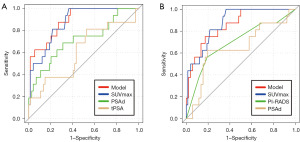
Discussion
68Ga-PSMA PET-CT has recently emerged as an important imaging approach to the first diagnosis of primary PCa. In this study, we compared the diagnostic performance of 68Ga-PSMA PET-CT and mpMRI, and combined the advantages of SUVmax, PSAd, and PI-RADS category to develop a novel predictive model to predict csPCa before prostate biopsy. Compared with SUVmax alone, the novel predictive model demonstrated excellent diagnostic performance with significantly improved specificity (0.910, 95% CI: 0.824–0.963) and positive predictive values (0.811, 95% CI: 0.648–0.920) at a slight sacrifice of sensitivity. Furthermore, the model showed good calibration and clinical benefit, and was successfully validated in an independent external cohort.
PI-RADS category and SUVmax have frequently been reported as predictors used to assess csPCa in prior reports. PI-RADS category is the most widely accepted noninvasive imaging-based approach to csPCa detection, exhibiting excellent sensitivity. A recent meta-analysis showed that PI-RADS v2.1 had excellent performance in the diagnosis of primary PCa with the combined sensitivity and specificity of 0.89 and 0.7314, respectively (18). Currently, multiple predictive models based on mpMRI have been developed and validated, some of which have been used in clinical diagnosis and risk stratification of primary PCa (19-21). As a quantitative parameter, SUVmax is considered to be an important predictor of csPCa, and offers values in diagnosing csPCa and predicting Gleason score (22). Jiao et al. showed that SUVmax could effectively detect csPCa, and its sensitivity and specificity were 85.85% and 86.21%, respectively (23). The results of our study are consistent with this. The optimal threshold of SUVmax is 6.4, and its sensitivity and specificity were 91.2% and 79.5% and specificity. In addition, recent studies have tried to incorporate SUVmax into the predictive model. Hu et al. combined SUVmax with other clinical indicators to effectively improve the performance of predicting the pathological upgrading of PCa (24).
Clinical use of PSAd can effectively reduce unnecessary biopsies. Görtz et al. showed that PSAd was a significant predictor of csPCa in PI-RADS 3 cases (16). Hansen et al. analyzed 236 patients with PI-RADS 1–2 and found that mpMRI combined with PSAd <0.1 ng/mL2 had a very high negative predictive value (0.91) for PCa with Gleason score 7–10 (25). It suggested that PSAd can effectively improve the diagnostic efficacy of the PI-RADS for distinguishing PCa risk in patients with PI-RADS 1–3. Falagario et al. confirmed that PSAd was associated with the risk of csPCa by biopsy, and found that PSAd improved the performance of csPCa discrimination when combined with other clinical information in predictive models (26). Considering the complementary effect of PSAd and mpMRI, Wei et al. incorporated PSAd into the predictive model based on mpMRI, which significantly improved the performance in diagnosing csPCa in the transitional zone (27). Similarly, Wen et al. constructed a clinical predictive model based on PSAd and mpMRI, and the results showed that it could significantly improve the diagnostic performance of tumors in peripheral zone (15).
Our multivariate analysis did not indicate PSAd as an independent predictor of csPCa, which is contrary to previous research. Nevertheless, since PSAd is a recognized factor for risk stratification of PI-RADS 1–3 patients, it was also incorporated into our predictive model. Additionally, we compared the diagnostic performance of two prediction models, one including PSAd and the other one not including PSAd, and found no significant difference in diagnosing PCa. Considering the relatively small sample size of our study, this difference may be confirmed in subsequent research.
Multiple prior MRI-based models have been developed to gauge the risk of csPCa at biopsy (19-21,28). However, relative to 68Ga-PSMA PET-CT, these models were less able to predict PCa and reduce unnecessary biopsy (29). Jiao et al. (23) indicated that SUVmax derived from 68Ga-PSMA PET-CT were able to effectively detect csPCa with high sensitivity (85.85%), and specificity (86.21%) at a 5.30 cut-off value. The results of the present study similarly yielded high sensitivity (91.2%) and specificity (79.5%) values at a cut-off value of 6.4. These findings are in contrast to those from a recent meta-analysis indicating that, when used to detect csPCa, 68Ga-PSMA PET-CT yielded high sensitivity (0.9, 95% CI: 0.90–0.99) but relatively low specificity (0.66, 95% CI: 0.52–0.78) (30). This may be attributable to the moderately increased uptake of PSMA in cases of granulomatous disease, BPH, and prostatitis (12).
According to PI-RADS guidelines, PI-RADS category 3 lesions represent an equivocal risk of csPCa (31). Chen et al. previously demonstrated that the combination of mpMRI and 68Ga-PSMA PET-CT was associated with higher sensitivity than mpMRI alone without sacrificing the specificity, and these improvements may be most evident in the PI-RADS category 3 lesions (32). In our subgroup analyses, we also observed the model and SUVmax exhibited higher diagnostic accuracy in cases with PI-RADS category 3 lesions or tPSA levels ≤10 ng/mL. It indicated that 68Ga-PSMA PET-CT would benefit this population. It is worth noting that the patients in the subgroup analysis, who had a lower pre-test probability, did not show considerable difference in the accuracy of the model vs. that of the SUVmax. The possible reason for this is that the training cohort had a higher pre-test probability for having csPCa (median PSA: 11, median PSAd: 0.2). And the external cohort also had a considerably high csPCa rate (73%) and high PI-RADS category 5 rate (60%).
At present, 68Ga-PSMA PET-CT has not been widely used as the clinical routine application in the first diagnosis of PCa mainly as it is expensive and not routinely available in community. On the other hand, emerging evidences have indicated the higher diagnostic ability compared with mpMRI, especially in some specific situations. Yang et al. revealed that 68Ga-PSMA PET-CT may be helpful in risk stratification of men with PI-RADS 3 lesions with better performance than current models based on mpMRI (33). Besides, the sensitivity and specificity of 68Ga-PSMA PET-CT to identify the PI-RADS 3 lesions were 96.2% and 80.8%, respectively (33). In patients with tPSA level of 4–20 ng/mL, PSMA PET-CT was reported to be more accurate than mpMRI. In addition, PSMA PET-CT was more accurate in the diagnosis of bilateral, multifocal prostate lesions (34). 68Ga-PSMA PET-CT could also accurately distinguish between GG2 and GG3 PCa in ISUP grading group (35).
Based on the current evidences, we cannot identify which patients are more likely to benefit from pre-biopsy 68Ga-PSMA PET-CT. However, our study showed that some patients had false-negative results on MRI and true-positive results on 68Ga-PSMA PET-CT. To identify which patients are more likely to benefit from additional 68Ga-PSMA PET-CT, we are conducting further analyses to determine their risk factors. These risk factors may include age, PSA levels, tumor size and location, among others. In future studies, we will further explore these issues to identify which patients are most likely to benefit from 68Ga-PSMA PET-CT and avoid missing csPCa.
One of the primary limitations of the present study is that although all patients in the external cohort eventually received all imaging modalities, some patients received 68Ga-PSMA PET-CT after biopsy. Thus, we refined a 2-week interval between biopsy and subsequent 68Ga-PSMA PET-CT. Unlike the performance of mpMRI, the reading of 68Ga-PSMA PET-CT imaging was not much affected by hemorrhage and the injury of prostate structure. However, it would be also a bias as the loss of tumor by biopsy will potentially impact the SUVmax of suspicious lesions. On the other hand, although the model performed effectively in the external cohort, there were some drawbacks. Because of the limited sample size of the prospective study, we additionally collected 42 patients who underwent PET-CT after biopsy to serve as the validation cohort. Besides, patients in validation cohort only received SB by different urologist who may have erred on diagnosing a lower rate of csPCa. Even though the combination of mpMRI-TB and dual-tracer PET-CT-TB has been reported to outperform SB in detecting csPCa (32), misclassification and lower csPCa detection rate may be evident in comparison with prostatectomy specimens. And the external cohort was collected retrospectively with relatively small sample size and from the same institution, there may be a lack of representativeness. Further large-scale multi-center validations are needed to clarify the performance of this predictive model.
Conclusions
In summary, this study developed a novel predictive nomogram incorporating SUVmax, PI-RADS categories, and PSAd, and that was capable of accurately detecting csPCa prior to biopsy in a manner likely to offer clinical benefit. This tool can thus be used by clinicians to gauge csPCa risk prior to unnecessary biopsies.
Acknowledgments
We thank everyone who provided support for this study.
Funding: This investigation was sponsored by the key Research and Development program of Hunan Province (No. 2021SK2014), the National Natural Science Foundation of China (No. 91859207) and Natural Science Foundation of Hunan Province (No. 2020JJ5882). The funders had no role in the design and conduct of the study; collection, management, analysis, and interpretation of the data; preparation, review, or approval of the manuscript; and decision to submit the manuscript for publication.
Footnote
Reporting Checklist: The authors have completed the TRIPOD reporting checklist. Available at https://tau.amegroups.com/article/view/10.21037/tau-22-832/rc
Data Sharing Statement: Available at https://tau.amegroups.com/article/view/10.21037/tau-22-832/dss
Peer Review File: Available at https://tau.amegroups.com/article/view/10.21037/tau-22-832/prf
Conflicts of Interest: All authors have completed the ICMJE uniform disclosure form (available at https://tau.amegroups.com/article/view/10.21037/tau-22-832/coif). All authors report that this investigation was sponsored by the Key Research and Development program of Hunan Province (No. 2021SK2014), the National Natural Science Foundation of China (No. 91859207) and Natural Science Foundation of Hunan Province (No. 2020JJ5882). The authors have no other conflicts of interest to declare.
Ethical Statement:
Open Access Statement: This is an Open Access article distributed in accordance with the Creative Commons Attribution-NonCommercial-NoDerivs 4.0 International License (CC BY-NC-ND 4.0), which permits the non-commercial replication and distribution of the article with the strict proviso that no changes or edits are made and the original work is properly cited (including links to both the formal publication through the relevant DOI and the license). See: https://creativecommons.org/licenses/by-nc-nd/4.0/.
References
- US Preventive Services Task Force. Screening for Prostate Cancer: US Preventive Services Task Force Recommendation Statement. JAMA 2018;319:1901-13. [Crossref] [PubMed]
- Weinreb JC, Barentsz JO, Choyke PL, et al. PI-RADS Prostate Imaging - Reporting and Data System: 2015, Version 2. Eur Urol 2016;69:16-40. [Crossref] [PubMed]
- Richenberg J, Løgager V, Panebianco V, et al. The primacy of multiparametric MRI in men with suspected prostate cancer. Eur Radiol 2019;29:6940-52. [Crossref] [PubMed]
- Hoeks CM, Barentsz JO, Hambrock T, et al. Prostate cancer: multiparametric MR imaging for detection, localization, and staging. Radiology 2011;261:46-66. [Crossref] [PubMed]
- Le JD, Tan N, Shkolyar E, et al. Multifocality and prostate cancer detection by multiparametric magnetic resonance imaging: correlation with whole-mount histopathology. Eur Urol 2015;67:569-76. [Crossref] [PubMed]
- Filson CP, Natarajan S, Margolis DJ, et al. Prostate cancer detection with magnetic resonance-ultrasound fusion biopsy: The role of systematic and targeted biopsies. Cancer 2016;122:884-92. [Crossref] [PubMed]
- Afshar-Oromieh A, Avtzi E, Giesel FL, et al. The diagnostic value of PET/CT imaging with the (68)Ga-labelled PSMA ligand HBED-CC in the diagnosis of recurrent prostate cancer. Eur J Nucl Med Mol Imaging 2015;42:197-209. [Crossref] [PubMed]
- Budäus L, Leyh-Bannurah SR, Salomon G, et al. Initial Experience of (68)Ga-PSMA PET/CT Imaging in High-risk Prostate Cancer Patients Prior to Radical Prostatectomy. Eur Urol 2016;69:393-6. [Crossref] [PubMed]
- Berger I, Annabattula C, Lewis J, et al. (68)Ga-PSMA PET/CT vs. mpMRI for locoregional prostate cancer staging: correlation with final histopathology. Prostate Cancer Prostatic Dis 2018;21:204-11. [Crossref] [PubMed]
- Qiu DX, Li J, Zhang JW, et al. Dual-tracer PET/CT-targeted, mpMRI-targeted, systematic biopsy, and combined biopsy for the diagnosis of prostate cancer: a pilot study. Eur J Nucl Med Mol Imaging 2022;49:2821-32. [Crossref] [PubMed]
- Paschalis A, Sheehan B, Riisnaes R, et al. Prostate-specific Membrane Antigen Heterogeneity and DNA Repair Defects in Prostate Cancer. Eur Urol 2019;76:469-78. [Crossref] [PubMed]
- Shetty D, Patel D, Le K, et al. Pitfalls in Gallium-68 PSMA PET/CT Interpretation-A Pictorial Review. Tomography 2018;4:182-93. [Crossref] [PubMed]
- Jena A, Taneja R, Taneja S, et al. Improving Diagnosis of Primary Prostate Cancer With Combined (68)Ga-Prostate-Specific Membrane Antigen-HBED-CC Simultaneous PET and Multiparametric MRI and Clinical Parameters. AJR Am J Roentgenol 2018;211:1246-53. [Crossref] [PubMed]
- Cornford P, van den Bergh RCN, Briers E, et al. EAU-EANM-ESTRO-ESUR-SIOG Guidelines on Prostate Cancer. Part II-2020 Update: Treatment of Relapsing and Metastatic Prostate Cancer. Eur Urol 2021;79:263-82. [Crossref] [PubMed]
- Wen J, Tang T, Ji Y, et al. PI-RADS v2.1 Combined With Prostate-Specific Antigen Density for Detection of Prostate Cancer in Peripheral Zone. Front Oncol 2022;12:861928. [Crossref] [PubMed]
- Görtz M, Radtke JP, Hatiboglu G, et al. The Value of Prostate-specific Antigen Density for Prostate Imaging-Reporting and Data System 3 Lesions on Multiparametric Magnetic Resonance Imaging: A Strategy to Avoid Unnecessary Prostate Biopsies. Eur Urol Focus 2021;7:325-31. [Crossref] [PubMed]
- Bryant RJ, Yamamoto H, Eddy B, et al. Protocol for the TRANSLATE prospective, multicentre, randomised clinical trial of prostate biopsy technique. BJU Int 2023;131:694-704. [Crossref] [PubMed]
- Woo S, Suh CH, Kim SY, et al. Diagnostic Performance of Prostate Imaging Reporting and Data System Version 2 for Detection of Prostate Cancer: A Systematic Review and Diagnostic Meta-analysis. Eur Urol 2017;72:177-88. [Crossref] [PubMed]
- van Leeuwen PJ, Hayen A, Thompson JE, et al. A multiparametric magnetic resonance imaging-based risk model to determine the risk of significant prostate cancer prior to biopsy. BJU Int 2017;120:774-81. [Crossref] [PubMed]
- Radtke JP, Wiesenfarth M, Kesch C, et al. Combined Clinical Parameters and Multiparametric Magnetic Resonance Imaging for Advanced Risk Modeling of Prostate Cancer-Patient-tailored Risk Stratification Can Reduce Unnecessary Biopsies. Eur Urol 2017;72:888-96. [Crossref] [PubMed]
- Mehralivand S, Shih JH, Rais-Bahrami S, et al. A Magnetic Resonance Imaging-Based Prediction Model for Prostate Biopsy Risk Stratification. JAMA Oncol 2018;4:678-85. [Crossref] [PubMed]
- Xue AL, Kalapara AA, Ballok ZE, et al. (68)Ga-Prostate-Specific Membrane Antigen Positron Emission Tomography Maximum Standardized Uptake Value as a Predictor of Gleason Pattern 4 and Pathological Upgrading in Intermediate-Risk Prostate Cancer. J Urol 2022;207:341-9. [Crossref] [PubMed]
- Jiao J, Kang F, Zhang J, et al. Establishment and prospective validation of an SUV(max) cutoff value to discriminate clinically significant prostate cancer from benign prostate diseases in patients with suspected prostate cancer by (68)Ga-PSMA PET/CT: a real-world study. Theranostics 2021;11:8396-411. [Crossref] [PubMed]
- Hu Q, Hong X, Xu L, et al. A nomogram for accurately predicting the pathological upgrading of prostate cancer, based on (68) Ga-PSMA PET/CT. Prostate 2022;82:1077-87. [Crossref] [PubMed]
- Hansen NL, Barrett T, Kesch C, et al. Multicentre evaluation of magnetic resonance imaging supported transperineal prostate biopsy in biopsy-naïve men with suspicion of prostate cancer. BJU Int 2018;122:40-9. [Crossref] [PubMed]
- Falagario UG, Jambor I, Lantz A, et al. Combined Use of Prostate-specific Antigen Density and Magnetic Resonance Imaging for Prostate Biopsy Decision Planning: A Retrospective Multi-institutional Study Using the Prostate Magnetic Resonance Imaging Outcome Database (PROMOD). Eur Urol Oncol 2021;4:971-9. [Crossref] [PubMed]
- Wei X, Xu J, Zhong S, et al. Diagnostic value of combining PI-RADS v2.1 with PSAD in clinically significant prostate cancer. Abdom Radiol (NY) 2022;47:3574-82. [Crossref] [PubMed]
- Alberts AR, Roobol MJ, Verbeek JFM, et al. Prediction of High-grade Prostate Cancer Following Multiparametric Magnetic Resonance Imaging: Improving the Rotterdam European Randomized Study of Screening for Prostate Cancer Risk Calculators. Eur Urol 2019;75:310-8. [Crossref] [PubMed]
- Zhang J, Shao S, Wu P, et al. Diagnostic performance of (68)Ga-PSMA PET/CT in the detection of prostate cancer prior to initial biopsy: comparison with cancer-predicting nomograms. Eur J Nucl Med Mol Imaging 2019;46:908-20. [Crossref] [PubMed]
- Satapathy S, Singh H, Kumar R, et al. Diagnostic Accuracy of (68)Ga-PSMA PET/CT for Initial Detection in Patients With Suspected Prostate Cancer: A Systematic Review and Meta-Analysis. AJR Am J Roentgenol 2021;216:599-607. [Crossref] [PubMed]
- Barentsz JO, Richenberg J, Clements R, et al. ESUR prostate MR guidelines 2012. Eur Radiol 2012;22:746-57. [Crossref] [PubMed]
- Chen M, Zhang Q, Zhang C, et al. Combination of (68)Ga-PSMA PET/CT and Multiparametric MRI Improves the Detection of Clinically Significant Prostate Cancer: A Lesion-by-Lesion Analysis. J Nucl Med 2019;60:944-9. [Crossref] [PubMed]
- Yang J, Tang Y, Zhou C, et al. The use of (68) Ga-PSMA PET/CT to stratify patients with PI-RADS 3 lesions according to clinically significant prostate cancer risk. Prostate 2023;83:430-9. [Crossref] [PubMed]
- Li Y, Han D, Wu P, et al. Comparison of (68)Ga-PSMA-617 PET/CT with mpMRI for the detection of PCa in patients with a PSA level of 4-20 ng/ml before the initial biopsy. Sci Rep 2020;10:10963. [Crossref] [PubMed]
- Yi N, Wang Y, Zang S, et al. Ability of (68) Ga-PSMA PET/CT SUVmax to differentiate ISUP GG2 from GG3 in intermediate-risk prostate cancer: A single-center retrospective study of 147 patients. Cancer Med 2023;12:7140-8. [Crossref] [PubMed]