Construction of a two-gene prognostic model related to ferroptosis in renal cell carcinoma
Highlight box
Key findings
• A two-gene prognostic model associated with ferroptosis was constructed in this study, which has a promising ability to predict the prognosis of RCC in specific cohorts.
What is known and what is new?
• FRGs are effective diagnostic markers and even drug targets for numerous cancer types.
• The present study focuses on the less-investigated relationship between FRGs and RCC progression, identifying signature genes (NCOA4 and CDKN1A) as potential prognostic biomarkers and even therapeutic targets for RCC patients.
What are the implications, and what should change now?
• This prognostic model may provide clinicians with a reliable predictive assessment tool and offer new perspectives for the future clinical management of RCC.
Introduction
Renal cell carcinoma (RCC) is a highly malignant tumor of the urinary system (1), accounting for 90% of renal cancers, and it also contributes to cancer-specific mortality (2,3). Kidney cancer is responsible for at least 175,000 cancer-related deaths worldwide, with approximately 403,262 new cases reported in 2018, according to Global Cancer Statistics (4). The incidence of RCC is increasing globally, causing nearly 200,000 deaths annually and accounting for 1.8% of all cancer-related deaths (5). RCC is the second leading cause of death among urological tumor patients (6). Radical or partial nephrectomy is the first-line treatment for early-stage RCC; however, 30–40% of patients experience disease recurrence (7). Unfortunately, RCC is either confined to the kidney (65% of cases) or has already metastasized (16% of cases) at initial diagnosis, with associated 5-year survival rates of 93% and 12%, respectively (8). Although the advent of targeted therapies has revolutionized the treatment of RCC and improved the survival time of patients with metastases, the median survival time is still less than 3 years (9). Therefore, to improve the survival rate of patients, it is clinically significant to find reliable markers to closely monitor their prognosis and adjust the treatment plan.
As a genetically encoded cell death program, ferroptosis differs from classical types of programmed cell death, such as apoptosis, necrosis, senescence, and pyroptosis (10-12). Ferroptosis is an iron-dependent cell death process driven by lipid peroxidation (13,14). Previous research has shown that ferroptosis-related genes (FRGs) can be used as diagnostic markers and may even function as drug targets, especially when in terms of developing and pharmacologically designing anticancer drugs and cancer treatments (15). For example, the study has discussed the sensitivity of different subtypes of breast cancer to iron death, suggesting that iron death related genes may provide a new direction for the development of biomarkers and treatment strategies for breast cancer (16). The induction of ferroptosis is considered a promising cancer treatment, especially in ferroptosis-sensitive cancer cells (13). However, the potential of ferroptosis in cancer treatment needs to be further explored (17), and the exact role of ferroptosis in the development of kidney cancer remains unclear.
Numerous studies have also demonstrated the critical role of ferroptosis in tumorigenesis and progression (18-20). For example, it has been shown that the FRG, FDFT1, is essential for predicting the prognosis of patients with colorectal cancer and clear cell RCC (ccRCC) (21,22). Significant overexpression of GPX4 in hepatocellular carcinoma is correlated with increased malignancy (23). There is also evidence that various primary tumors and metastases have varying degrees of DPP4 expression (24). In addition, other genes regulated by ferroptosis, such as ACSL4 (25), TFRC (26), and GLS2 (27), have also been shown to be closely related to the occurrence and development of tumors. However, there are few studies on the relationship between FRGs and the prognosis of RCC patients.
Therefore, the present study aimed to investigate ferroptosis-related prognostic markers, which could improve the current treatment, diagnosis, and prevention strategies for RCC patients. We also constructed a two-gene signature that could predict the outcome of RCC patients after screening for 23 FRGs. Our findings suggest that some FRGs play a crucial role in RCC progression and may serve as potential prognostic biomarkers and therapeutic targets for RCC patients. We present this article in accordance with the TRIPOD reporting checklist (available at https://tau.amegroups.com/article/view/10.21037/tau-23-346/rc).
Methods
Firstly, we screened differential FRGs through The Cancer Genome Atlas (TCGA)-RCC data set, then narrowed the range of differential genes by prognostic analysis, and finally constructed a prognostic risk model based on iron death related genes by COX regression analysis. At the same time, we analyzed the differences in clinical characteristics, immune infiltration and drug sensitivity between high-risk group and low-risk group. The study was conducted in accordance with the Declaration of Helsinki (as revised in 2013). The specific analyses were as follows.
Screening for differentially expressed genes (DEGs)
TCGA dataset (https://portal.gdc.cancer.gov) was used to obtain the RNA-sequencing expression (level 3) profiles and clinical information for RCC. The limma R software package (version 4.2.0, W. N. Venables, D. M. Smith and the R Core Team) was used to study the differentially expressed messenger RNAs (mRNAs). The following threshold was set for the differential gene expression of mRNA: adjusted P<0.05 and log2(fold change) >1 or <−1. Functional enrichment analysis was conducted on the data to confirm the underlying function of potential targets. Gene Ontology (GO) is an open-source tool that is widely used to annotate genes with their functions, such as molecular functions, biological pathways, and cellular components. An enrichment analysis based on the Kyoto Encyclopedia of Genes and Genomes (KEGG) is another important tool for analyzing gene functions as well as associated information about high-level genome functions. A KEGG pathway enrichment analysis was carried out using the ClusterProfiler package in R to better understand the carcinogenesis of mRNA. A boxplot was drawn using the ggplot2 R software package.
Differential iron death-associated genes in kidney cancer
The RNA-sequencing expression profiles and corresponding clinical information for RCC were downloaded from TCGA dataset (https://portal.gdc.cancer.gov), and the acquisition method and application complied with the relevant guidelines and policies. The FRGs reported by Liu et al. were applied to systematically analyze the aberrances and functional implications of ferroptosis in cancer (28). The survival difference between the two groups was also compared using Kaplan-Meier survival analysis with a log-rank test. Kaplan-Meier curves were analyzed using log-rank tests and univariate Cox proportional hazards regressions to generate P values and hazard ratios (HRs) with 95% confidence intervals (CIs). The above analysis methods were conducted using R software (version 4.2.0), and P<0.05 was considered statistically significant. A Venn diagram Wenny was drawn using online sites (/tools/venny/index.html).
Construction of the iron death-associated model
The Caret R package was used to randomly divide all of the RCC samples into a training set and a test set, and differences in the clinical parameters between the training and test groups were assessed using Pearson’s χ test (or Fisher’s exact test). Univariate Cox regression and multivariate stepwise Cox regression of proportional hazards analyses were performed using the survival and survminer packages in R. We calculated the risk score as follows: risk score = λ1 × Exp1 + λ2 × Exp2 + … + λi × Expi, where λ represented the coefficient value.
Next, we built a predictive model according to the risk score. The optimum cutoff evaluation was determined using the survival and survminer R packages, and the samples were divided into high- and low-risk groups according to the cutoff point. Then, overall survival (OS) and progression-free survival (PFS) curves for risk assessment were constructed using the survival and survminer R packages. Three-dimensional (3D) principal component analysis (PCA) plots were visualized using the scatterplot3d R package. Receiver operating characteristic (ROC) curves were generated using the Survival ROC R package, and heatmaps were drawn using the pheatmap function in the pheatmap package. We then tested the model’s estimation on the test and overall sets.
Establishment and validation of a predictive nomogram
To determine whether the model was independent of age, gender, tumor-node-metastasis (TNM) stage, and tumor stage, we performed univariate and multivariate analyses using the Cox regression modeling procedure with forward stepwise selection, and the critical value was set as P<0.05. We used the survival R package to plot each variable’s P value, HR, and 95% CI in a forest plot. Nomogram prediction plots were used to predict cancer prognosis. Factors including age, gender, tumor stage, T stage, N stage, and M stage were utilized to produce nomograms that predicted the OS of RCC patients at 1, 3, and 5 years. We also tested the validity of the nomogram using the consistency index (C-index) and calibration. In addition, 1,000 replicate samples were measured using the bootstrap method to assess the nomograms’ recognition ability. We then generated calibration curves for the nomograms and monitored the predicted trends based on the observed results. The ggpurb R package was used to demonstrate the differences in risk scores between different subgroups, such as females and males and M0 and M1.
Tumor mutational burden (TMB) analysis
TMB reflects the number of cancer mutations. The PEARL programming language was used to extract the mutation data from TCGA website. We then used the “maftools” R package to examine and integrate the TCGA data and analyzed the TMB differences between the high- and low-risk groups. Waterfall plots were used to illustrate the relationship between the risk score and TMB in RCC patients.
Immune cell infiltration analysis
CIBERSORT analysis was performed to assess the correlation between the expression of 22 tumor-infiltrating immune cells in RCC tumor tissues and the risk score. Immune cells were visualized using the “corrplot” R package, and the Wilcoxon rank-sum test, and P<0.05 was used to compare the immune cell infiltration levels between groups. The single-sample gene set enrichment analysis (ssGSEA) R package was used to explore the relationship between risk scores and immune cell infiltration, which was drawn by using a related heatmap. In addition, the differential expression of immune checkpoints in the high- and low-risk populations was analyzed by using “limma”, “reshape2”, “ggplot2”, and “ggpubr” R packages and presented as boxplots.
Assessment of drug sensitivity
To assess the sensitivity of the high- and low-risk groups in the ferroptosis-related prediction model to drugs in the clinical treatment of RCC, we used the “pRRophetic” R package and its dependencies, including “car, ridge, genefilter, preprocessCore, and sva”. The Wilcoxon signed-rank test was used to compare the half maximal inhibitory concentration (IC50) differences of standard anticancer drugs between the high- and low-risk groups. In addition, the “limma”, “ggpubr”, and “ggplot2” R packages were also utilized.
Statistical analysis
The statistical analysis was performed on R studio using the R programming language (version 4.2.0). The R package “limma” was used to combine RNA-sequencing transcriptome data and TCGA somatic mutation data. Survival analysis was performed with the “survival” and “survminer” packages in R. For comparing categorical data between groups, chi-square tests were used. It was considered statistically significant when the P<0.05.
Results
Screening for FRGs in RCC
First, we compared the expression of 23 FRGs in kidney cancer and normal renal tissues, among which 18 FRGs were differentially expressed (P<0.05). Most differentially expressed FRGs were highly expressed in tumor tissues (Figure 1A). Subsequently, we identified 3,035 DEGs (2,185 upregulated genes and 850 downregulated genes) by comparing normal kidney tissue and tumor samples of RCC (Figure 1B). Table S1 shows the clinical information of 883 patients with kidney cancer. The enriched KEGG signaling pathways and GO analysis were selected to demonstrate the primary biological actions of DEGs (Figure S1). The correlation circle plot confirmed the positive and negative correlations among FRGs; the circle of CDKN1A was the largest and its log-rank P value was minimal (Figure 1C). The heatmap shows the expression trends of FRGs between tumor and normal tissues (Figure S2). Furthermore, we performed Kaplan-Meier survival analysis on 18 FRGs and found that 12 genes were associated with prognosis (Figure S3, Table S2). From the intersection of the screening above, we finally obtained four candidate FRGs, as shown in the Venn diagram (Figure 1D). The survival curves of these four genes were shown in the Figure 2A-2D.
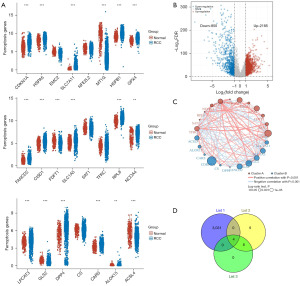

Building a prognostic model based on two genes
We identified three genes that were significantly associated with OS using univariate Cox regression (NCOA4, CDKN1A, and DPP4, P<0.05). We divided the patients into two groups: a training group (n=440) and a testing group (n=439). As shown in clinical data in Table S3, the results showed no differences in clinical characteristics between the training and testing groups. Subsequently, we performed stepwise multivariate Cox regression analyses in the training set (n=440) to further narrow the genes. We then constructed an FRG-related prognostic model using two genes, NCOA4 and CDKN1A. The risk score was calculated as follows: 0.20534 × expressionCDKN1A − 0.12909 × expressionNCOA4. We performed a similar process for the test and entire sets. High-risk patients had shorter OS than low-risk patients in the training (P<0.001, Figure 3A), testing (P=0.015, Figure 3B), and entire (P<0.001, Figure 3C) sets. Likewise, PFS in the high-risk group was significantly lower than that in the low-risk group (Figure 3D-3F). PCA showed marked differences between the high- and low-risk groups (Figure 3G-3I). The expression heatmap, risk score, and survival status of the two subgroups demonstrated that individual patient survival was closely related to the risk assessment (Figure 4A).
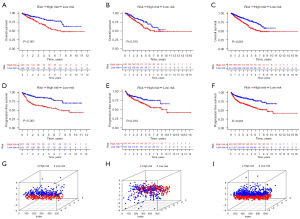
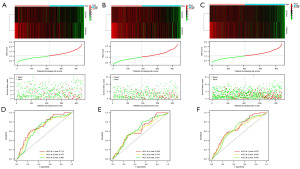
To confirm the accuracy of the risk score model, we performed the same analysis on the test and entire groups, with similar results (Figure 4B,4C). In the training set, the areas under the ROC curves (AUCs) for 1-, 3-, and 5-year OS were 0.714, 0.673, and 0.668, respectively (Figure 4D). Similarly, the AUC for the test and whole set indicated that the model had moderate clinical prognostic significance (Figure 4E,4F). To validate the expression of NCOA4 and CDKN1A in RCC patients, we performed bioinformatics analysis using data from TCGA dataset. The analysis showed that the expression of NCOA4 mRNA was reduced, and CDKN1A expression was increased in 893 RCC samples compared to 128 normal samples. Reverse transcriptase-quantitative polymerase chain reaction (RT-qPCR) validated the results. The mRNA levels of NCOA4 and CDKN1A were significantly downregulated and upregulated in 12 RCC samples compared to paired normal samples, respectively (Figure 5).

Clinical value of the prognostic risk model
Univariate and multivariate Cox regression analyses were further used to investigate the risk score as an independent prognostic indicator for RCC. The univariate Cox analysis showed statistically significant differences among age (HR =1.027, P<0.001), T (HR =2.094, P<0.001), M (HR =1.975, P<0.001), N (HR =0.865, P=0.043), stage (HR =2.143, P<0.001), and risk score (HR =2.072, P<0.001). Multivariate Cox analysis revealed that age (HR =1.031, P<0.001), T stage (HR =0.730, P=0.038), stage (HR =2.583, P<0.001), and risk score (HR =1.757, P=0.002) were significantly associated with OS (Figure 6A).
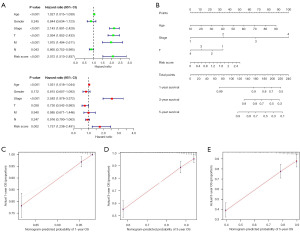
Next, based on the risk score and clinicopathological characteristics, an accurate nomogram was developed to predict the 1-, 3-, and 5-year survival probability of RCC patients (Figure 6B). The C-index of the nomogram was 0.731 (95% CI: 0.672–0.790). It appeared that the model was stable over time, as the AUCs were 0.728, 0.704, and 0.898 at 1-, 3-, and 5-year, respectively. The calibration plots were in good agreement with the nomogram prediction (Figure 6C-6E). Finally, an analysis of the risk score’s relationship with clinical characteristics was conducted. We found that patients with higher TNM stage, T stage, and M stage had higher risk scores than those with lower TNM stage, T stage, and M stage (P<0.05). Also, males had higher risk scores than females. However, there were no significant differences between dissimilar ages and N stages (P>0.05) (Figure 7A-7D).
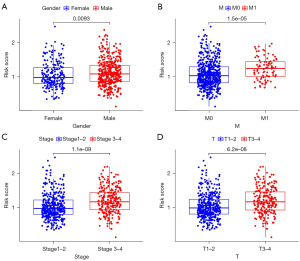
Exploration of the immune profile of the high- and low-risk groups
To further explore the correlation between the risk score and the immune microenvironment, the CIBERSORT algorithm was used to analyze the proportion of tumor-infiltrating immune subpopulations and construct 22 immune cell profiles in the RCC samples (Figure 8A). Correlation analysis of the immune cell populations and related functions revealed that functions (such as costimulation and coinhibition) and antigen-presenting cell (APC)-related functions [including costimulation and coinhibition, human leukocyte antigen (HLA), checkpoint, and parainflammation] were significantly different between the high- and low-risk groups based on the ssGSEA algorithm in TCGA-RCC data (Figure 8B). Based on these results, it was determined that FRGs were associated with immune cell infiltration in RCC.
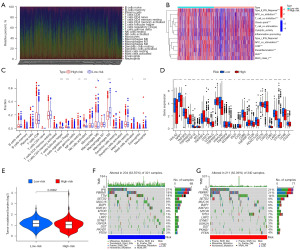
We also investigated the immune infiltration of patients in the low- and high-risk groups using the CIBERSORT algorithm (Figure 8C), which indicated a significant correlation between risk class and level of immune infiltration. A comparison of the low- and high-risk groups was also performed in terms of immune checkpoints (Figure 8D). Most immune checkpoints differed significantly between them. Interestingly, the tumor necrosis factor receptor superfamily, including TNFRSF25, TNFRSF4, and TNFRSF18, was highly expressed in the high-risk group.
TMB and therapeutic drug sensitivity
To investigate the possibility that tumor mutational load may play a role in RCC, we measured mutations in RCC and calculated the corresponding TMB scores. As shown in Figure 8E, the mutational burden in the high-risk RCC group was lower than that in the low-risk group. Mutation status was also compared between the high- and low-risk groups. The waterfall plot showed the 15 genes with the highest mutation frequency in the two groups (Figure 8F,8G). We found significant differences in the IC50 values between the low- and high-risk groups for various drugs when we compared drug susceptibility. Table S4 shows the sensitive drugs in the high- and low-risk groups. Among these drugs, high-risk patients responded better to crizotinib, etoposide, and sorafenib (Figure 9A-9C). In contrast, gemcitabine, pazopanib, and sunitinib were more effective in low-risk patients (Figure 9D-9F).
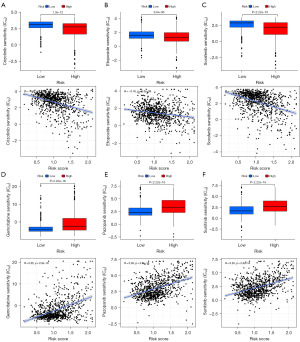
Discussion
RCC is not a single entity but encompasses a variety of different tumor subtypes, which are defined by unique pathological features or molecular alterations (29,30). RCC is predominantly resistant to standard systemic chemotherapy and radiation therapy (31). Activating regulated cell death is considered an ideal cancer treatment strategy, contributing to the development of resistance to useful drugs (32). Several recent studies have confirmed that ferroptosis is a newly discovered form of regulated cell death caused by the accumulation of lethal lipid peroxidation (11,33). Dysfunctional ferroptosis is associated with a variety of diseases, including cancer, and inhibition or upregulation of ferroptosis modulates metabolic reprogramming in cancer cells (34,35). Given the critical role of ferroptosis in regulating cell death and the lack of studies on ferroptosis in RCC, it is important to investigate the expression pattern of FRGs to understand the role of ferroptosis in RCC patients.
In this study, we performed a systematic analysis of 23 FRGs. Eighteen genes were differentially expressed between RCC tumors and paracancerous tissues. We performed a correlation analysis of these genes to gain more insight into the functional role of differentially expressed FRGs in RCC. The results showed either positive or negative correlations between them, suggesting that FRGs do not act independently but through a complex series of processes working together to drive or inhibit lipid peroxidation (36). Subsequently, we selected 12 FRGs associated with the prognosis of RCC, intersected them with DEGs of kidney cancer, and finally obtained four candidate FRGs: CDKN1A, DPP4, MT1G, and NCOA4. Specifically, the four genes involved in ferroptosis perform distinct functions. For example, CDKN1A is a direct transcriptional target of p53, and CDKN1A ablation could phenocopy some aspects of p53 loss-of-function (37). In addition, DPP4 inhibits erastin-induced ferroptosis in colorectal cancer when its activity is blocked by p53. Nevertheless, when p53 is not present, DPP4 binds to NOX1, forming the NOX-DPP4 complex, which results in lipid peroxidation in the plasma membrane and iron toxicity (38,39). MT1G hypermethylation significantly increases the risk of lymph node metastasis in patients with thyroid cancer. In addition, MT1G can also mediate tumor cell growth through PI3K/AKT signaling pathway (40). Protein imbalance of NCOA4 plays an important role in the pathogenesis of ovarian cancer (41). However, the mechanism of action of these genes in RCC needs to be further explored. We are also continuing to study the specific molecular mechanism of FRGs in the occurrence and development of RCC.
Next, using Cox regression analyses, we constructed a risk signature based on two prognostic FRGs (CDKN1A and NCOA4). CDKN1A was highly expressed in kidney cancer, while NCOA4 was expressed at low levels in kidney cancer, and this result was verified by RT-qPCR. The RCC patients were divided into high- and low-risk groups according to their median risk scores. We noted that OS and PFS were shorter in high-risk patients than in low-risk patients. The ROC curve showed that the signature worked well and had an excellent prognostic prediction ability. Additionally, multivariate Cox analysis indicated that the risk score was an independent prognostic indicator. We also developed an alignment diagram that combined the risk score with other clinical parameters, which was simplified to a single numerical estimate of event probability, to predict each patient’s prognosis. The calibration curves showed excellent agreement between actual and expected results. We also observed a higher risk score for individuals with more advanced disease, which showed that the signature-based risk score was highly correlated with RCC progression. An increasing number of studies have reported that abnormal expression of FRGs is associated with human cancer (42,43). The above results also indicate a potential role of iron death in RCC. However, the specific mechanisms of CDKN1A and NCOA4 in RCC needs to be further investigated.
In general, TMB is a valid marker for immunotherapy (44,45), with higher TMBs signifying a greater likelihood that the immune system will recognize the tumor and a stronger possibility that immunotherapy will be effective. Our results showed that the high- and low-risk groups were significantly different in terms of TMB. Among the top 15 mutated genes, VHL had the highest mutation frequency in RCC patients, followed by PBRM1. There is an interaction between FRGs and tumor immune microenvironment (TIME). It has been shown that TIME is associated with iron metabolism and homeostasis, while ferroptosis is essential for tumor immunity. Besides, the immunosuppressive molecules released by iron-death cells can inhibit the role of T cells and APCs in the immune microenvironment, thus promoting tumor immune escape (46). Typically, as the tumor progresses, immune cells infiltrate into the tumor microenvironment. A previous study showed that regulatory T-cells, follicular helper T-cells, and memory B-cells were associated with poor ccRCC outcomes (47). Moreover, the infiltration of natural killer (NK) cells can impair the body’s immune regulation (48). According to the studies described above, the high-risk group we identified exhibited characteristics consistent with a poor prognosis. In addition, the ssGSEA results pointed to immune features such as type II interferon (IFN) response inactivation and T-cell costimulation activation in high-risk populations. Based on these results, it appears that our features may act to block the immune response in RCC’s tumor microenvironment and contribute to its progression. Moreover, the tumor necrosis factor receptor superfamily can initiate numerous immune and inflammatory processes (49). We found that the family members, TNFRSF25, TNFRSF4, and TNFRSF18, had elevated expression in the high-risk group. Therefore, the evaluation of FRGs in RCC will enhance the understanding of immune infiltration in the immune microenvironment, thus helping oncologists to develop personalized immunotherapy strategies. However, the correlation with ferroptosis needs to be further explored. Finally, we analyzed the drug sensitivity of these FRGs to guide clinical treatment. There are significant differences in IC50 between high-risk and low-risk patients to dozens of drugs, suggesting that some drugs are more sensitive to high-risk patients than low-risk patients. Sorafenib and sunitinib are both approved for treating advanced RCC (50,51). Our study found that sorafenib was sensitive in the high-risk group, while sunitinib was sensitive in the low-risk group. The role of crizotinib and pazopanib in treating kidney cancer needs to be further defined.
Our study developed a prognostic risk model based on the genetic association of ferroptosis and identified low- and high-risk RCC groups. In our study, a significant correlation was identified between this model and prognosis. In addition, immune cell infiltration and drug sensitivity analyses were performed, and the relevance of the FRG model to RCC was initially demonstrated. However, despite achieving some encouraging results, this study has some limitations. First, we were not able to obtain validation from the Gene Expression Omnibus (GEO) and International Cancer Genome Consortium (ICGC) databases. The bias and limitations of commercial microarray data compared to TCGA data prevented us from obtaining the correct information on FRGs despite using the GEO and ICGC databases. Second, additional experiments are needed to determine specific mechanisms of action of critical genes for clinical application.
Conclusions
In summary, we provided new insights into the role of FRGs in RCC and constructed a promising risk-prognostic model that has potential as a biomarker of OS in RCC patients. Then, we established a new favorable prognostic column line graph for personalized survival prediction. Our constructed ferroptosis-associated signature has important clinical implications and may offer a new therapeutic strategy for individualized treatment and immunotherapeutic response in RCC patients. These two ferroptosis-associated genes may be therapeutic targets for RCC.
Acknowledgments
Funding: This work was supported by the National High Level Hospital Clinical Research Funding (High Quality Clinical Research Project of Peking University First Hospital, No. 2022CR75), the National Natural Science Foundation of China (Nos. 82141103, 82172617, 82172665, 81872081), the Scientific Research Seed Fund of Peking University First Hospital (No. 2021SF01), the Capital’s Funds for Health Improvement and Research (No. 2022-2-4074), and the Sino-Russian Mathematics Center.
Footnote
Reporting Checklist: The authors have completed the TRIPOD reporting checklist. Available at https://tau.amegroups.com/article/view/10.21037/tau-23-346/rc
Peer Review File: Available at https://tau.amegroups.com/article/view/10.21037/tau-23-346/prf
Conflicts of Interest: All authors have completed the ICMJE uniform disclosure form (available at https://tau.amegroups.com/article/view/10.21037/tau-23-346/coif). The authors have no conflicts of interest to declare.
Ethical Statement: The authors are accountable for all aspects of the work in ensuring that questions related to the accuracy or integrity of any part of the work are appropriately investigated and resolved. The study was conducted in accordance with the Declaration of Helsinki (as revised in 2013).
Open Access Statement: This is an Open Access article distributed in accordance with the Creative Commons Attribution-NonCommercial-NoDerivs 4.0 International License (CC BY-NC-ND 4.0), which permits the non-commercial replication and distribution of the article with the strict proviso that no changes or edits are made and the original work is properly cited (including links to both the formal publication through the relevant DOI and the license). See: https://creativecommons.org/licenses/by-nc-nd/4.0/.
References
- Wang G, Zhang ZJ, Jian WG, et al. Novel long noncoding RNA OTUD6B-AS1 indicates poor prognosis and inhibits clear cell renal cell carcinoma proliferation via the Wnt/β-catenin signaling pathway. Mol Cancer 2019;18:15. [Crossref] [PubMed]
- Wang Z, Kim TB, Peng B, et al. Sarcomatoid Renal Cell Carcinoma Has a Distinct Molecular Pathogenesis, Driver Mutation Profile, and Transcriptional Landscape. Clin Cancer Res 2017;23:6686-96. [Crossref] [PubMed]
- Yun EJ, Lin CJ, Dang A, et al. Downregulation of Human DAB2IP Gene Expression in Renal Cell Carcinoma Results in Resistance to Ionizing Radiation. Clin Cancer Res 2019;25:4542-51. [Crossref] [PubMed]
- Cheng WK, Kaur G, Sjöberg E, et al. Nuclear and stromal expression of Manic fringe in renal cell carcinoma. Exp Mol Pathol 2021;122:104667. [Crossref] [PubMed]
- Khazaei Z, Sohrabivafa M, Momenabadi V, et al. Global cancer statistics 2018: Globocan estimates of incidence and mortality worldwide prostate cancers and their relationship with the human development index. Advances in Human Biology 2019;9:245. [Crossref]
- Li J, Huang C, Zou Y, et al. CircTLK1 promotes the proliferation and metastasis of renal cell carcinoma by sponging miR-136-5p. Mol Cancer 2020;19:103. [Crossref] [PubMed]
- Buti S, Puligandla M, Bersanelli M, et al. Validation of a new prognostic model to easily predict outcome in renal cell carcinoma: the GRANT score applied to the ASSURE trial population. Ann Oncol 2017;28:2747-53. [Crossref] [PubMed]
- Jilaveanu LB, Puligandla M, Weiss SA, et al. Tumor Microvessel Density as a Prognostic Marker in High-Risk Renal Cell Carcinoma Patients Treated on ECOG-ACRIN E2805. Clin Cancer Res 2018;24:217-23. [Crossref] [PubMed]
- Greef B, Eisen T. Medical treatment of renal cancer: new horizons. Br J Cancer 2016;115:505-16. [Crossref] [PubMed]
- Zhang Z, Guo M, Li Y, et al. RNA-binding protein ZFP36/TTP protects against ferroptosis by regulating autophagy signaling pathway in hepatic stellate cells. Autophagy 2020;16:1482-505. [Crossref] [PubMed]
- Zhang Z, Yao Z, Wang L, et al. Activation of ferritinophagy is required for the RNA-binding protein ELAVL1/HuR to regulate ferroptosis in hepatic stellate cells. Autophagy 2018;14:2083-103. [Crossref] [PubMed]
- Luo M, Wu L, Zhang K, et al. miR-137 regulates ferroptosis by targeting glutamine transporter SLC1A5 in melanoma. Cell Death Differ 2018;25:1457-72. [Crossref] [PubMed]
- Chen GQ, Benthani FA, Wu J, et al. Artemisinin compounds sensitize cancer cells to ferroptosis by regulating iron homeostasis. Cell Death Differ 2020;27:242-54. [Crossref] [PubMed]
- Verma N, Vinik Y, Saroha A, et al. Synthetic lethal combination targeting BET uncovered intrinsic susceptibility of TNBC to ferroptosis. Sci Adv 2020;6:eaba8968. [Crossref] [PubMed]
- Ji X, Qian J, Rahman SMJ, et al. xCT (SLC7A11)-mediated metabolic reprogramming promotes non-small cell lung cancer progression. Oncogene 2018;37:5007-19. [Crossref] [PubMed]
- Li Z, Chen L, Chen C, et al. Targeting ferroptosis in breast cancer. Biomark Res 2020;8:58. [Crossref] [PubMed]
- Gao M, Monian P, Quadri N, et al. Glutaminolysis and Transferrin Regulate Ferroptosis. Mol Cell 2015;59:298-308. [Crossref] [PubMed]
- Zhang H, Deng T, Liu R, et al. CAF secreted miR-522 suppresses ferroptosis and promotes acquired chemo-resistance in gastric cancer. Mol Cancer 2020;19:43. [Crossref] [PubMed]
- Fu J, Li T, Yang Y, et al. Activatable nanomedicine for overcoming hypoxia-induced resistance to chemotherapy and inhibiting tumor growth by inducing collaborative apoptosis and ferroptosis in solid tumors. Biomaterials 2021;268:120537. [Crossref] [PubMed]
- Liang JY, Wang DS, Lin HC, et al. A Novel Ferroptosis-related Gene Signature for Overall Survival Prediction in Patients with Hepatocellular Carcinoma. Int J Biol Sci 2020;16:2430-41. [Crossref] [PubMed]
- Nie J, Shan D, Li S, et al. A Novel Ferroptosis Related Gene Signature for Prognosis Prediction in Patients With Colon Cancer. Front Oncol 2021;11:654076. [Crossref] [PubMed]
- Huang R, Zhang C, Wang X, et al. Identification of FDFT1 as a potential biomarker associated with ferroptosis in ccRCC. Cancer Med 2022;11:3993-4004. [Crossref] [PubMed]
- Guerriero E, Capone F, Accardo M, et al. GPX4 and GPX7 over-expression in human hepatocellular carcinoma tissues. Eur J Histochem 2015;59:2540. [Crossref] [PubMed]
- Enz N, Vliegen G, De Meester I, et al. CD26/DPP4 - a potential biomarker and target for cancer therapy. Pharmacol Ther 2019;198:135-59. [Crossref] [PubMed]
- Li Y, Feng D, Wang Z, et al. Ischemia-induced ACSL4 activation contributes to ferroptosis-mediated tissue injury in intestinal ischemia/reperfusion. Cell Death Differ 2019;26:2284-99. [Crossref] [PubMed]
- Su H, Tao T, Yang Z, et al. Circular RNA cTFRC acts as the sponge of MicroRNA-107 to promote bladder carcinoma progression. Mol Cancer 2019;18:27. [Crossref] [PubMed]
- Jennis M, Kung CP, Basu S, et al. An African-specific polymorphism in the TP53 gene impairs p53 tumor suppressor function in a mouse model. Genes Dev 2016;30:918-30. [Crossref] [PubMed]
- Liu Z, Zhao Q, Zuo ZX, et al. Systematic Analysis of the Aberrances and Functional Implications of Ferroptosis in Cancer. iScience 2020;23:101302. [Crossref] [PubMed]
- Signoretti S, Flaifel A, Chen YB, et al. Renal Cell Carcinoma in the Era of Precision Medicine: From Molecular Pathology to Tissue-Based Biomarkers. J Clin Oncol 2018;36:JCO2018792259. [Crossref] [PubMed]
- Tang S, Xiao X. Ferroptosis and kidney diseases. Int Urol Nephrol 2020;52:497-503. [Crossref] [PubMed]
- Chan DA, Sutphin PD, Nguyen P, et al. Targeting GLUT1 and the Warburg effect in renal cell carcinoma by chemical synthetic lethality. Sci Transl Med 2011;3:94ra70. [Crossref] [PubMed]
- Yang WS. Regulation of ferroptotic cancer cell death by GPX4. Cell 2014;156:317-31. [Crossref] [PubMed]
- Xue CC, Li MH, Zhao Y, et al. Tumor microenvironment-activatable Fe-doxorubicin preloaded amorphous CaCO(3) nanoformulation triggers ferroptosis in target tumor cells. Sci Adv 2020;6:eaax1346. [Crossref] [PubMed]
- Wu Y, Zhang S, Gong X, et al. The epigenetic regulators and metabolic changes in ferroptosis-associated cancer progression. Mol Cancer 2020;19:39. [Crossref] [PubMed]
- Matsushita M, Freigang S, Schneider C, et al. T cell lipid peroxidation induces ferroptosis and prevents immunity to infection. J Exp Med 2015;212:555-68. [Crossref] [PubMed]
- Stockwell BR, Friedmann Angeli JP, Bayir H, et al. Ferroptosis: A Regulated Cell Death Nexus Linking Metabolism, Redox Biology, and Disease. Cell 2017;171:273-85. [Crossref] [PubMed]
- Mehta S, Huillard E, Kesari S, et al. The central nervous system-restricted transcription factor Olig2 opposes p53 responses to genotoxic damage in neural progenitors and malignant glioma. Cancer Cell 2011;19:359-71. [Crossref] [PubMed]
- Hassannia B, Vandenabeele P, Vanden Berghe T. Targeting Ferroptosis to Iron Out Cancer. Cancer Cell 2019;35:830-49. [Crossref] [PubMed]
- Tarangelo A, Magtanong L, Bieging-Rolett KT, et al. p53 Suppresses Metabolic Stress-Induced Ferroptosis in Cancer Cells. Cell Rep 2018;22:569-75. [Crossref] [PubMed]
- Fu J, Lv H, Guan H, et al. Metallothionein 1G functions as a tumor suppressor in thyroid cancer through modulating the PI3K/Akt signaling pathway. BMC Cancer 2013;13:462. [Crossref] [PubMed]
- Rockfield S, Flores I, Nanjundan M. Expression and function of nuclear receptor coactivator 4 isoforms in transformed endometriotic and malignant ovarian cells. Oncotarget 2017;9:5344-67. [Crossref] [PubMed]
- Xie Y, Hou W, Song X, et al. Ferroptosis: process and function. Cell Death Differ 2016;23:369-79. [Crossref] [PubMed]
- Lu B, Chen XB, Ying MD, et al. The Role of Ferroptosis in Cancer Development and Treatment Response. Front Pharmacol 2018;8:992. [Crossref] [PubMed]
- Cui Y, Chen H, Xi R, et al. Whole-genome sequencing of 508 patients identifies key molecular features associated with poor prognosis in esophageal squamous cell carcinoma. Cell Res 2020;30:902-13. [Crossref] [PubMed]
- Khagi Y, Goodman AM, Daniels GA, et al. Hypermutated Circulating Tumor DNA: Correlation with Response to Checkpoint Inhibitor-Based Immunotherapy. Clin Cancer Res 2017;23:5729-36. [Crossref] [PubMed]
- Lu L, Liu LP, Zhao QQ, et al. Identification of a Ferroptosis-Related LncRNA Signature as a Novel Prognosis Model for Lung Adenocarcinoma. Front Oncol 2021;11:675545. [Crossref] [PubMed]
- Yu Y, Chang Z, Han C, et al. Long non-coding RNA MINCR aggravates colon cancer via regulating miR-708-5p-mediated Wnt/β-catenin pathway. Biomed Pharmacother 2020;129:110292. [Crossref] [PubMed]
- Sierra JM, Secchiari F, Nuñez SY, et al. Tumor-Experienced Human NK Cells Express High Levels of PD-L1 and Inhibit CD8(+) T Cell Proliferation. Front Immunol 2021;12:745939. [Crossref] [PubMed]
- Shin W, Lee HT, Lim H, et al. BAFF-neutralizing interaction of belimumab related to its therapeutic efficacy for treating systemic lupus erythematosus. Nat Commun 2018;9:1200. [Crossref] [PubMed]
- Castellsagué J, Gel B, Fernández-Rodríguez J, et al. Comprehensive establishment and characterization of orthoxenograft mouse models of malignant peripheral nerve sheath tumors for personalized medicine. EMBO Mol Med 2015;7:608-27. [Crossref] [PubMed]
- Singhal SS, Singhal J, Yadav S, et al. RLIP76: a target for kidney cancer therapy. Cancer Res 2009;69:4244-51. [Crossref] [PubMed]
(English Language Editor: A. Kassem)