Metabonomic analysis of seminal plasma in necrozoospermia patients based on liquid chromatography-mass spectrometry
Highlight box
Key findings
• The alteration of seminal plasma choline or 2-hydroxyglutarate, and arachidonic acid metabolism pathway are involved in the pathogenesis of necrozoospermia.
What is known and what is new?
• The causes of necrozoospermia are multiple. Choline is needed as an energy source for sperm motility.
• The decrease of seminal plasma choline and the abnormality arachidonic acid metabolism pathway are involved in the pathology of necrozoospermia.
What is the implication, and what should change now?
• Our study provides a basis to further explore the mechanism of necrozoospermia.
• Due to the relatively limited understanding of necrozoospermia, more in-depth and detailed research is needed.
Introduction
Infertility is defined as a couple being unable to conceive despite having regular sexual intercourse and foregoing any contraceptive measures for more than 1 year. The incidence rate of male fertility is in the range of 15% to 20%, with male factors accounting for approximately 50% of cases (1). The cause of male infertility is complex and is caused by many diseases or factors, with the etiology being unclear in more than half of the patients. Asthenozoospermia is a manifestation of male infertility. The incidence rate of necrozoospermia—a special type of asthenozoospermia—is in the range of 0.2% to 0.4% of all male infertility cases (2). The sperm in necrozoospermia are mostly dead and not immotile. To date, there are relatively few studies in this area; in-depth research on the etiology and pathogenesis of necrozoospermia is lacking (routine semen analysis is inadequate). Accordingly, there is a lack of consensus regarding suitable treatments. In the field of metabolomics, differential metabolites linked to a disease or condition are screened, which often facilitates the discovery of new biomarkers associated with the disease and further understanding of the pathogenesis (3). Seminal plasma metabolome alterations in necrozoospermia remain unknown. Seminal plasma is particularly suitable for the detection of biomarkers for both the diagnosis of unexplained male infertility and possible insights into its mechanism (4).
In the present study, the research material consisted of the seminal plasma of patients (adult males) with necrozoospermia compared with samples from adult males with healthy (motile) sperm. The seminal plasma metabolites were analyzed using liquid chromatography-mass spectrometry (LC-MS) based on the principles of nontargeted metabolomics. Principal component analysis (PCA), orthogonal partial least squares-discriminant analysis (OPLS-DA) and other multivariate statistical analyses were conducted to screen out the differential metabolites and to analyze key metabolic pathways as a way of understanding the changes in seminal plasma metabolites of patients with necrozoospermia. Ultimately, the goal is to establish a theoretical basis for research into the cause of necrozoospermia and possible treatments. We present this article in accordance with the MDAR reporting checklist (available at https://tau.amegroups.com/article/view/10.21037/tau-23-14/rc).
Methods
Specimen collection
Human semen samples were donated by volunteers aged 26–56 years from the Affiliated Shenzhen Maternity & Child Healthcare Hospital, Southern Medical University, Shenzhen, China. The study was conducted in accordance with the Declaration of Helsinki (as revised in 2013) and approved by the Ethics Committee of the Shenzhen Maternal and Child Health Care Hospital (No. SFYLS[2021]038). Written informed consent forms were obtained from each participant. A total of 37 patients with normal sperm motility and 28 patients with necrozoospermia were selected for further metabolomic analysis from June 2021 to July 2022. General information was acquired from all subjects, including age, sperm motility and duration of abstinence (Table 1).
Table 1
Parameter | Experimental group (n=28) | Control group (n=37) | P value |
---|---|---|---|
Age (years) | 36.5±7.59 | 36.8±7.04* | 0.845 |
Abstinence days | 4.32±1.19 | 4.36±1.03* | 0.746 |
Semen volume (mL) | 1.91±1.02 | 3.18±1.39** | <0.001 |
pH | 6.98±0.36 | 7.28±0.16** | <0.001 |
Sperm concentration (×106 per mL) | 66.83±101.37 | 80.79±44.56** | <0.001 |
Forward motility percentage (%) | 0. 00 ±0. 00 | 44.24±6.34** | <0.001 |
Sperm viability (%) | 21.71±14.57 | 74.03±8.34** | <0.001 |
Seminal plasma neutral α-glucosidase (µmol per ejaculate) | 30.41±22.52 | 60.1±36.17** | 0.001 |
Seminal plasma zinc (µmol per ejaculate) | 5.72±3.30 | 8.15±4.64** | 0.045 |
*, P>0.05; **, P<0.05. SD, standard deviation.
Inclusion criteria: The normal (control) group consisted of healthy males, without genetic diseases, who had visited our clinic due to infertility from female factors and who had obtained normal results from at least two routine semen examinations, including the following criteria: no agglutination in semen, sperm concentration ≥15×106/mL, percentage of forward motility ≥32%, semen white blood cells ≤1×106/mL. The necrozoospermia study group consisted of males whose sperm evaluation results indicated a percentage of forward motility of 0%, sperm vitality (eosin-aniline black method) of less than 58%, and sperm concentration equal to or above the lower limits of the reference values (sperm concentration ≥15×106/mL).
In the case of both groups, the exclusion criteria were obesity; metabolic diseases (diabetes, abnormal thyroid function, etc.); recent acute urinary tract infection, mycoplasma or chlamydia infection; leukocytospermia; exposure to substances that are toxic to the reproductive system; abstinence of more than 7 days or less than 2 days; and a semen volume of less than 1 mL.
Following 3 to 7 days of abstinence, participants masturbated and collected the semen in a designated dry collection cup, which was then placed in a 37 ℃ water bath to liquefy. The fifth edition of the World Health Organization (WHO) laboratory manual for the examination and processing of human semen (5) was used as a reference. The volume was measured by weighing. The computer-assisted semen analysis (CASA) system (Beijing, China, SAS-II) was used to analyze the semen volume, sperm concentration and sperm motility. The eosin-aniline black method was used to detect sperm viability (Figure S1), mix the semen sample well, remove a 50-µL aliquot of semen, mix with an equal volume of eosin-aniline black suspension, wait for 30 seconds, make a smear on a glass slide and allow it to dry in air, examine the slide with brightfield optics at ×1,000 magnification and oil immersion after drying, and evaluate at least 200 spermatozoa, which were stained or unstained cells with the aid of a laboratory counter, to achieve an acceptably low sampling error. Diff-Quik staining was used to analyze sperm morphology (Figures S2,S3) (Table 1). The semen sample remaining was centrifuged after semen analysis at 3,000 ×g for 15 minutes. The sperm-free seminal plasma samples obtained were all stored frozen at −80 ℃. Seminal plasma samples were collected from patients diagnosed with necrozoospermia (n=28) as well as unaffected males (normozoospermia, n=37). The seminal plasma volume was approximately 1 mL.
Chemicals and reagents
LC-MS grade methanol (MeOH) was purchased from Fisher Scientific (Loughborough, UK, batch No. 215927, item No. A456-4). 2-Amino-3-(2-chloro-phenyl)-propionic acid was obtained from Aladdin (Shanghai, China, batch No. F2104083, item No. C105993-1G). Ultrapure water was generated using a Milli-Q system (Millipore, Bedford, USA).
Equipment
A high-speed centrifuge was obtained from Hunan Xiangyi Experiment Equipment Co., Ltd. (Hunan, China, Product Model: H1850-R). The centrifugal vacuum evaporator was from Eppendorf China Ltd. (Shanghai, China, Product Model: 5305). The vortex mixer was obtained from Haimen Kylin-bell Lab Instruments Co., Ltd. (Haimen, China, Product Model:BE-2600). Microporous membrane filters (0.22 µm) were purchased from Tianjin Jinteng Experiment Equipment Co., Ltd. (Tianjin, China, Product Model: 0.22 µm PTFE).
Reagents
LC-MS grade acetonitrile (ACN) was purchased from Fisher Scientific (Loughborough, UK, batch number: 212213, item No. A955-4). Formic acid was obtained from TCI (Shanghai, China, batch number: SN8GK-Ⅱ, item No. F0654). Ammonium formate was obtained from Sigma-Aldrich (Shanghai, China, batch number: BCCG2341, item No. 70221-25G-F). Ultrapure water was generated using a Milli-Q system (Millipore, Bedford, USA).
Sample preparation
The frozen samples were then prepared following published procedures (6). The samples were thawed at 4 ℃, vortexed for 1 min, and then mixed evenly. Accurately transferred, an appropriate amount of the sample was transferred to a 2-mL centrifuge tube, to which 400 µL methanol was added (stored at −20 ℃) and vortexed for 1 min, followed by centrifugation for 10 min at 12,000 rpm at 4 ℃. The supernatant was transferred to a new 2-mL centrifuge tube, which was concentrated and dried. The dried sample was added to 150 µL of 2-chloro-l-phenylalanine (4 ppm) solution prepared with 80% methanol water (stored at 4 ℃) to redissolve the sample; the resulting supernatant was filtered through a 0.22-µm membrane and transferred into the detection bottle for the LC-MS step.
Liquid chromatography conditions
LC analysis was performed on a Vanquish UHPLC System (Thermo Fisher Scientific, USA). Chromatography was carried out with an ACQUITY UPLC® HSS T3 (150×2.1 mm, 1.8 µm) (Waters, Milford, MA, USA). The column was maintained at 40 ℃, and the flow rate and injection volume were set at 0.25 mL/min and 2 µL, respectively. In the positive ion mode, the mobile phases consisted of (C) 0.1% formic acid in acetonitrile (v/v) and (D) 0.1% formic acid in water (v/v). The gradient elution procedure: 0–1 min, 2% C; 1–9 min, 2%–50% C; 9–12 min, 50–98% C; 12–13.5 min, 98% C; 13.5–14 min, 98–2% C; 14–20 min, 2% C. In the negative ion mode, the analyses were carried out with (A) acetonitrile and (B) ammonium formate (5 mM). The gradient elution procedure: 0–1 min, 2% A; 1–9 min, 2–50% A; 9–12 min, 50–98% A; 12–13.5 min, 98% A; 13.5–14 min, 98–2% A; 14–17 min, 2% A (7) (Figure 1).
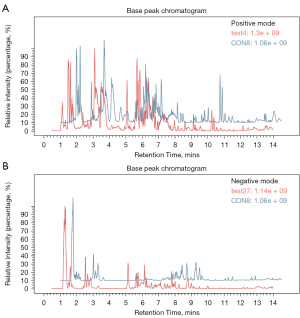
Mass spectroscopy conditions
Mass spectrometric detection of metabolites was performed on an Orbitrap Exploris 120 (Thermo Fisher Scientific) with an ESI ion source. Simultaneous positive and negative ion modes were used to collect data. The positive ion spray voltage was 3.50 kV, the negative ion spray voltage was −2.50 kV, the sheath gas was 30 arb, and the auxiliary gas was 10 arb. The capillary temperature was 325 ℃, the first level scanning was performed at a resolution of 60,000 FWHM; the first level ion scanning range was 100–1,000 m/z, and the second level cracking was performed by HCD, the collision voltage was 30%, the second level resolution was 15,000 FWHM; and the 4 ions were crushed before the signal acquisition. Dynamic exclusion was used to remove unnecessary MS/MS information (8).
Data processing and multivariate analysis
The MSConvert tool in the Proteowizard software package (v3.0.8789) (9) was used to convert the raw data into mzXML format, and XCMS (v3.12.0) (10) was used for feature detection, retention time correction and alignment to obtain substance quantification. The parameter settings were as follows: bw =2, ppm =15, peak width =c[5,30], mzwid =0.015, mzdiff =0.01, and method = “centWave”. The metabolites were identified based on accuracy mass (<30 ppm) and MS/MS data, which were matched with Human Metabolome Database (HMDB) (11) (http://www.hmdb.ca), massbank (12) (http://www.massbank.jp/), LipidMaps (13) (http://www.lipidmaps.org), mzcloud (14) (https://www.mzcloud.org), Kyoto Encyclopedia of Genes and Genomes (KEGG) (15) (http://www.genome.jp/kegg/) and self-built material bank for substance identification. The quality control-based robust locally estimated scatterplot smoothing (LOESS) signal correction (QC-RLSC) (16) was applied for data normalization to correct for any systematic bias. After normalization, only ion peaks with relative standard deviation (RSD) values less than 30% in QC were retained to ensure proper metabolite identification.
Ropls (17) software (v1.22.0) was used for multivariate data analyses and modeling. Models were built on PCA, partial least-square discriminant analysis (PLS-DA) and OPLS-DA. The metabolic profiles were visualized as score plots, in which each point represented a sample. The corresponding loading plot and S-plot were generated to provide information on the metabolites that would influence clustering of the samples. All the models evaluated were tested for overfitting with permutation tests. Details can be found in Figures 2-4 by R2X (cumulative) and R2Y (cumulative) values, and the prediction performance was measured by Q2 (cumulative) and a permutation test. The permuted model should not be able to predict classes: R2 and Q2 values at the Y-axis intercept, which must be lower than those of Q2 and the R2 of the nonpermuted model. Variable importance projection (VIP) produced by OPLS-DA and fold change (FC) were applied to discover the contributable variable for classification. OPLS-DA allowed the determination of discriminating metabolites using the VIP. Finally, metabolite molecules were considered statistically significant when the P value was <0.05 and the VIP value was >1.
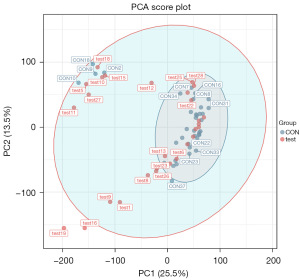
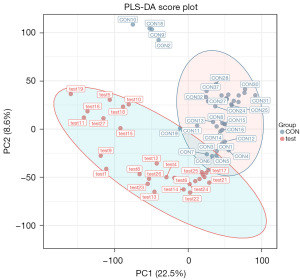
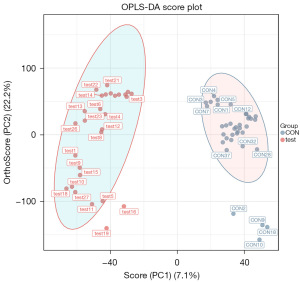
Statistical analysis
The Statistical Package for Social Sciences for Windows (SPSS 26, Chicago, IL, USA) was used for statistical analyses. Differences were considered statistically significant when the P value was <0.05. The distribution of data was evaluated by the Kolmogorov-Smirnov test. Student’s t-test or Mann-Whitney Wilcoxon test were used for comparisons.
Pathway analysis
The identified differential metabolites were subjected to pathway analysis using MetaboAnalyst (v3.0) (18), which combined results from powerful pathway enrichment analysis with pathway topology analysis. Then, the identified metabolites from the metabolomics analysis were mapped to the KEGG pathway for biological interpretation of higher-level systemic functions. The metabolites and corresponding pathways were visualized using the KEGG Mapper tool.
Results
Semen test results
There were no statistically significant differences in age or abstinence time between the two groups (P>0.05); statistically significant differences were found between the two groups in terms of semen volume, pH, sperm concentration, forward motility percentage, normal sperm morphology percentage, sperm survival rate, seminal plasma neutral α-glucosidase, and seminal plasma zinc (P<0.05) (Table 1).
Base peak chromatogram
The components were eluted by chromatographic separation, which continuously entered the mass spectrum. A mass spectrogram was obtained in each scan, and the ion with the highest intensity in each mass spectrogram was selected to be continuously depicted. The ion intensity was taken as the ordinate, the time was taken as the abscissa, and the resulting spectrum was called the base peak chromatogram.
Quality control (QC) and quality assurance (QA)
QC
In mass spectrometry-based metabolomics studies, to obtain reliable and high-quality metabolomics data, QC is needed. In this experiment, QC samples were used for QC during LC-MSMS (Figure 5). Theoretically, QC samples were all the same, but there were systematic errors during sample extraction and detection analysis, which resulted in differences between QC samples. A smaller difference indicates higher stability of the method and better data quality, which was reflected in the dense distribution of QC samples on the PCA diagram. The data were reliable.
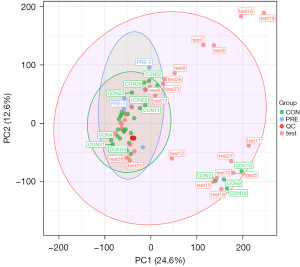
QA
To discover biomarkers, the coefficient of variation of the RSD of potential characteristic peaks cannot exceed 30% in QC samples, and the noncompliant feature peaks should be removed. Therefore, on the basis of QC, QA is usually carried out to remove features with poor repeatability in QC samples to obtain a higher quality dataset, which is more favorable for biomarker detection. In the QC sample, the proportion of characteristic peaks with RSD <30% reached approximately 65%, which is an indication of the high precision of the data, demonstrating that the data are good (Figures 6,7).
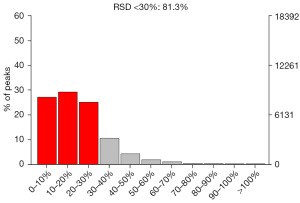
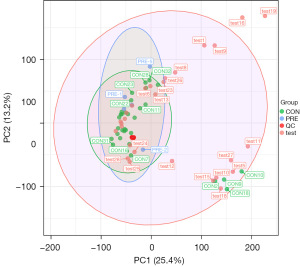
Overall metabolite cluster heatmap
Cluster analysis was used to determine the metabolic patterns of metabolites under different experimental conditions. Metabolites with similar metabolic patterns had similar functions in the same metabolic process or cellular pathway. Therefore, by clustering metabolites with the same or similar metabolic patterns, the functions of unknown metabolites or known metabolites can be inferred. The relative values of metabolites under different experimental conditions were used as metabolic levels for hierarchical clustering analysis. Different colored areas represented different clustering information, and the metabolic expression patterns within the same group were similar, which may have similar functions or participated in the same biological process. A heatmap represented a matrix of data that used color gradients to visualize differences between the data, which can be scaled to preserve large differences while also highlighting smaller ones (Figure 8).
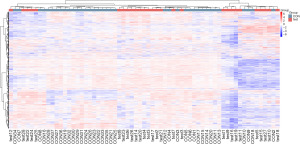
Data processing
PCA
PCA generates new characteristic variables by linearly combining metabolite variables according to a certain weight, classifying each group of data via the main new variable (principal component) and removing samples with poor repeatability (outlier samples) and abnormal samples. From the PCA score plot (Figure 2), the degree of aggregation and dispersion of samples can be obtained. The closer the sample distribution points, the closer the composition and concentration of the variables (molecules) present in these samples; conversely, the further the sample points are, the greater the difference. In the cross-validation of the model, R2X is the interpretability of the model. Typically, an R2 value greater than 0.5 is better. In the case of positive ion model parameters, R2X =0.531.
PLS-DA
At present, PLS-DA is the most frequently used classification method in metabolomics data analysis. It reduces dimensionality while combining regression models, which allows for discriminant analysis on the regression results using a certain discriminant threshold. The cross-validation of the model mainly uses parameters such as R2X, R2Y, and Q2 as a reference. R2X is the interpretability of the Model X variable (independent variable), R2Y is the interpretability rate of the Model Y variable (dependent variable), and Q2 is the predictability of the model. Theoretically, the closer the R2X and Q2 values are to 1, the better the model, whereas the lower the value is, the worse the fitting accuracy of the model. Under normal circumstances, it is better for R2 and Q2 to be greater than 0.5; the difference between the two should not be too large; and R2 and Q2 should have a maximum value of 1. When the R2 value is small, it often indicates poor repeatability of the test set (when background noise is high). When the Q2 value is small, it indicates high background noise in the test set or the presence of more abnormal samples (outliers) in the model. In the case of positive ion model validation parameters, R2X =0.588, R2Y =0.996, and Q2 =0.93 (Figure 3).
OPLS-DA
In total, 24,923 metabolites were detected, and samples were further analyzed using the OPLS-DA model to accurately identify differential metabolites in seminal plasma. OPLS-DA is a commonly used regression modeling method in multivariate statistical analysis. With this approach, the model is simplified, and the explanatory power is improved without affecting the predictive ability of the model, thus allowing optimal visualization of between-group differences. Similar to the PLS-DA model, OPLS-DA is able to evaluate the classification effect of the model through the use of R2X, R2Y, Q2, CV ANOVA, and OPLS-DA score plots. Through the use of VIP values to reveal variables (characteristic peaks), the importance of the X dataset and the associated Y dataset can be explained. The sum of the squares of all VIP values is equal to the total number of variables in the model, and hence its average value is 1. Then, VIP is >1 for a variable, which indicates that the variable is important and is often used as one of the screening criteria for potential biomarkers. The positive ion model validation parameters are R2X =0.464, R2Y =0.941, and Q2 =0.77 (Figure 4).
Differential analysis
Hierarchical clustering heatmap of differential metabolites—MS/MS
The differential metabolites were selected from the list of primary substances in the sample and screened with the preset P value and VIP threshold (19). The total number of metabolites was 24,923, of which 7,523 were differential metabolites (5,585 upregulated and 1,938 downregulated). This experiment used agglomerate hierarchical clustering: Each object was classified into one category, and these categories were merged into increasingly larger objects until the end. The dataset was scaled using the Pheatmap package in R to obtain a hierarchical clustering diagram of relative quantitative values of metabolites, as shown in Figure 9.
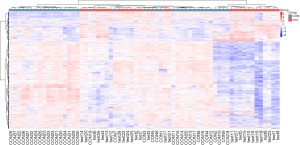
Identification results of differential metabolites
Metabolite identification was first confirmed based on the precise molecular weight, followed by MS/MS fragmentation mode for HMDB (http://www.hmdb.ca), massbank (http://www.massbank.jp/), LipidMaps (http://www.lipidmaps.org), mzcloud (https://www.mzcloud.org), as well as Nomi Metabolism’s self-developed reference database to confirm annotations and obtain the metabolites. A search was conducted for differential metabolites from the list of primary substances in the sample, which were screened with the preset P value and VIP threshold in the statistical test (19). The total number of metabolites obtained was 574, of which 194 were differential metabolites (129 upregulated and 65 downregulated). Only the top 10 differential metabolites are listed (Table 2).
Table 2
Name | MZ | VIP | Log2(FC) | P value | Formula | KEGG | Regulation |
---|---|---|---|---|---|---|---|
Choline | 104.1065 | 1.2 | −0.31 | 3.05E-05 | C5H14NO | C00114 | Down |
Benzaldehyde | 107.0488 | 1.8 | −0.52 | 7.52E-05 | C7H6O | C00261 | Down |
Pyrazinamide | 123.0392 | 2.4 | −0.69 | 1.54E-06 | C5H5N3O | C01956 | Down |
5-aminoimidazole-4-carboxamide | 127.0609 | 1.1 | 0.34 | 1.11E-03 | C4H6N4O | C04051 | Up |
Dihydrothymine | 128.0699 | 1.1 | −0.37 | 6.16E-03 | C5H8N2O2 | C00906 | Down |
Pyrrolidonecarboxylic acid | 130.0482 | 1.6 | 0.48 | 6.08E-03 | C5H7NO3 | C02237 | Up |
2-hydroxyglutarate | 131.0334 | 1.4 | 0.64 | 2.51E-04 | C5H8O5 | C02630 | Up |
Creatine | 132.0761 | 1.5 | −0.8 | 1.83E-03 | C4H9N3O2 | C00300 | Down |
5,6-dihydro-5-fluorouracil | 133.0313 | 1.3 | −0.53 | 5.42E-03 | C4H5FN2O2 | C16630 | Down |
p-hydroxyphenylacetic acid | 135.0438 | 1.8 | 0.79 | 3.82E-03 | C8H8O3 | C00642 | Up |
Name, name of the identified substance; MZ, mass-to-charge ratio; VIP, OPLS-DA first principal component variable importance value projection; log2(FC), log2 values of ploidy changes; P value, statistical P values, with smaller values indicating more significant differences; KEGG, KEGG compound number; Regulation, whether the material is up or down.
Differential metabolite pathway analysis using KEGG
MetPA is a part of MetaboAnalyst (www.metaboanalyst.ca) and is based predominantly on KEGG metabolic pathways. The MetPA database identifies possible metabolic pathways that have been subject to biological perturbations through metabolic pathway enrichment analysis and psychological analysis and then analyzes the metabolic pathways of metabolites. The MetPA database can be used to analyze the relevant metabolic pathways of two groups of differential metabolites. The data analysis algorithm uses a hypergeometric test, whereas the pathway topology analysis uses relative betweenness centrality. Based on the MetPA analysis results, the relative response value of the metabolic pathway was obtained according to the relative response value of metabolites identified in the metabolic pathway and the dimensionality reduction algorithm (Table 3). The correlation coefficient between the metabolic pathways was then calculated, and the metabolic pathway association network diagram was drawn.
Table 3
Pathway name | Total | Hits | P value | FDR | Impact |
---|---|---|---|---|---|
Arachidonic acid metabolism | 75 | 14 | 5.80E-08 | 3.14E-05 | 0.1867 |
Steroid hormone biosynthesis | 99 | 13 | 1.09E-05 | 2.97E-03 | 0.1313 |
Alanine, aspartate and glutamate metabolism | 28 | 6 | 2.00E-04 | 2.76E-02 | 0.2143 |
Bile secretion | 97 | 11 | 2.00E-04 | 2.76E-02 | 0.1134 |
Prostate cancer | 11 | 4 | 3.00E-04 | 2.82E-02 | 0.3636 |
Pathway name, name of the target pathway; Total, total number of metabolites in the target metabolic pathway; Hits, overall number of differential metabolites in the target metabolic pathway; P value, P value of the hypergeometric distribution test, where the smaller the P value, the more significant the pathway; FDR, false-positive correction value, which is calculated using the Benjamin and Hochberg method, where the greater the value, the higher the probability of false-positives; Impact, metabolic pathway influence value, where the higher the value, the greater the impact of differential metabolites on the target pathway.
Discussion
As an important part of systems biology, metabolomics correlates the dynamic changes in metabolites with biological processes through quantitative and qualitative analysis of small molecule metabolites present in the organism’s body fluids, tissues or organs at a particular point in time. Through metabolomics, investigators can elucidate the metabolic nature—at the molecular level—of the physiological functions and the mechanisms of action of medicine under the influence of internal and external factors. Metabolomics facilitates the visualization of the complex metabolic changes in the progression of disease and the effects of therapeutic drugs in vivo, thus advancing the study of the pathogenesis of the disease, as well as diagnosis and the identification of possible new drug targets (20).
Necrozoospermia is defined as a relatively high level of sperm mortality, with the percentage of living spermatozoa being less than 58% (21). There are multiple causes of necrozoospermia: testicular causes, posttesticular causes, or both (22). In 20% of cases, necrozoospermia is idiopathic (2). At present, the seminal plasma metabolome alterations in necrozoospermia remain unknown. Seminal plasma, a chemical component of semen, can be obtained through noninvasive sampling, making it particularly suitable for the detection of biomarkers and, ultimately, the diagnosis of unexplained male infertility as well as further insights into its mechanism.
In the present study, LC-MS was used to analyze seminal plasma metabolites via a nontargeted metabolomics approach. A total of 24,923 metabolites were obtained, of which 7,523 were differential metabolites (5,585 upregulated and 1,938 downregulated). Two groups were screened for differential metabolites via the preset P value and VIP threshold in the statistical test. A total of 574 metabolites were obtained, of which 194 were differentially expressed (129 upregulated and 65 downregulated). There were significant differences in seminal plasma metabolomics between necrozoospermia patients and patients whose sperm showed normal motility. The top five differential metabolites were choline, benzaldehyde, 5-hydroxypyrazinamide, 5-aminoimidazole-4-carboxamide, and dihydrothymine.
From the results of the present study, it was found that the content of choline in the seminal plasma of patients with necrospermia was low. Choline is an essential water-soluble B-group vitamin (23), and phosphatidylcholine is involved in the synthesis of the biomembrane composition. Choline deficiency results in dysfunction of the mitochondria and can increase oxidative stress. Choline plays an important role in the regulation of sperm membrane nuclei and fluidity and is a crucial factor in the maturation and fertilizing capacity of spermatozoa. Additionally, choline may be needed as an energy source for sperm motility (24). In addition, choline supplementation has been shown to facilitate fluoride-induced testicular toxicity (25). Detection of high Cho metabolites in the spectrum before testicular sperm extraction (TESE) in NOA patients suggests the chance of obtaining sperm in micro-TESE (26). Choline dehydrogenase (CHDH) also plays an important role in choline metabolism in the inner mitochondrial membrane. The diminished sperm motility was due to deletion of the Chdh gene in mice (27); sperm from Chdh2/2 males had decreased ATP concentrations, which likely stemmed from abnormal sperm mitochondrial morphology and function in these cells (28), which suggests that the occurrence of necrozoospermia may be related to a decrease in choline content in the seminal plasma. However, studies have found altered levels of choline in the seminal plasma of patients with asthenozoospermia (29,30), and the conclusions diametrically opposed to ours may be related to the different patients.
2-hydroxyglutarate (2-HG) accumulates abundantly in the testis (31), and there are two forms, D- and L-enantiomers. 2-HG is an L-enantiomer in the testis. Under hypoxic conditions, L-2-HG is generated from glutamine (32), and 2-HG was high in the seminal plasma of patients with necrospermia, which suggests that the occurrence of necrozoospermia may be related to hypoxia in the testis. The impaired motility of sperm suggests that glutamate metabolism plays an important role in energy production for sperm motility.
The results also showed the significance of arachidonic acid (AA) metabolism pathways in necrozoospermia seminal plasma. AA plays a significant role in lipid metabolism and was found to have a concentration-dependent inhibitory effect on sperm motility and sperm AA levels (33). The abnormal AA metabolic network reduces sperm motility via P38 MAPK, which is activated through three pathways: cyclooxygenase (COX), lipoxygenase (LOX) and cytochrome P450 (CYP450) (34). Therefore, we deduce that abnormalities in the AA metabolism pathway may be the cause of necrospermia. Further research is required to elucidate the pathological mechanism.
Conclusions
In summary, using nontargeted LC-MS, alterations in seminal plasma metabolites, such as choline, were found in necrozoospermia patients. The signaling pathways that involve these differential metabolites include the arachidonic acid metabolic pathway. It is speculated that the occurrence of necrozoospermia may be related to the decrease in seminal plasma choline, and abnormalities in the arachidonic acid metabolism pathway may be the pathological mechanism of necrozoospermia. Further study is required to uncover the exact mechanism.
Acknowledgments
Funding: The study was funded by the Shenzhen Key Medical Discipline Construction Fund (No. SZXK031).
Footnote
Reporting Checklist: The authors have completed the MDAR reporting checklist. Available at https://tau.amegroups.com/article/view/10.21037/tau-23-14/rc
Data Sharing Statement: Available at https://tau.amegroups.com/article/view/10.21037/tau-23-14/dss
Peer Review File: Available at https://tau.amegroups.com/article/view/10.21037/tau-23-14/prf
Conflicts of Interest: All authors have completed the ICMJE uniform disclosure form (available at https://tau.amegroups.com/article/view/10.21037/tau-23-14/coif). The authors have no conflicts of interest to declare.
Ethical Statement: The authors are accountable for all aspects of the work in ensuring that questions related to the accuracy or integrity of any part of the work are appropriately investigated and resolved. The study was conducted in accordance with the Declaration of Helsinki (as revised in 2013) and approved by the Ethics Committee of the Shenzhen Maternal and Child Health Care Hospital (No. SFYLS[2021]038). Written informed consent forms were obtained from each participant.
Open Access Statement: This is an Open Access article distributed in accordance with the Creative Commons Attribution-NonCommercial-NoDerivs 4.0 International License (CC BY-NC-ND 4.0), which permits the non-commercial replication and distribution of the article with the strict proviso that no changes or edits are made and the original work is properly cited (including links to both the formal publication through the relevant DOI and the license). See: https://creativecommons.org/licenses/by-nc-nd/4.0/.
References
- World Health Organization. WHO laboratory manual for the examination and processing of human semen, 6th ed. Geneva: World Health Organization; 2021.
- Boursier A, Dumont A, Boitrelle F, et al. Necrozoospermia: The tree that hides the forest. Andrology 2022;10:642-59. [Crossref] [PubMed]
- Courant F, Antignac JP, Monteau F, et al. Metabolomics as a potential new approach for investigating human reproductive disorders. J Proteome Res 2013;12:2914-20. [Crossref] [PubMed]
- Murgia F, Corda V, Serrenti M, et al. Seminal Fluid Metabolomic Markers of Oligozoospermic Infertility in Humans. Metabolites 2020;10:64. [Crossref] [PubMed]
- Cooper TG, Noonan E, von Eckardstein S, et al. World Health Organization reference values for human semen characteristics. Hum Reprod Update 2010;16:231-45. [Crossref] [PubMed]
- Demurtas A, Pescina S, Nicoli S, et al. Validation of a HPLC-UV method for the quantification of budesonide in skin layers. J Chromatogr B Analyt Technol Biomed Life Sci 2021;1164:122512. [Crossref] [PubMed]
- Zelena E, Dunn WB, Broadhurst D, et al. Development of a robust and repeatable UPLC-MS method for the long-term metabolomic study of human serum. Anal Chem 2009;81:1357-64. [Crossref] [PubMed]
- Want EJ, Masson P, Michopoulos F, et al. Global metabolic profiling of animal and human tissues via UPLC-MS. Nat Protoc 2013;8:17-32. [Crossref] [PubMed]
- Smith CA, Want EJ, O'Maille G, et al. XCMS: processing mass spectrometry data for metabolite profiling using nonlinear peak alignment, matching, and identification. Anal Chem 2006;78:779-87. [Crossref] [PubMed]
- Navarro-Reig M, Jaumot J, García-Reiriz A, et al. Evaluation of changes induced in rice metabolome by Cd and Cu exposure using LC-MS with XCMS and MCR-ALS data analysis strategies. Anal Bioanal Chem 2015;407:8835-47. [Crossref] [PubMed]
- Wishart DS, Guo A, Oler E, et al. HMDB 5.0: the Human Metabolome Database for 2022. Nucleic Acids Res 2022;50:D622-31. [Crossref] [PubMed]
- Horai H, Arita M, Kanaya S, et al. MassBank: a public repository for sharing mass spectral data for life sciences. J Mass Spectrom 2010;45:703-14. [Crossref] [PubMed]
- Sud M, Fahy E, Cotter D, et al. LMSD: LIPID MAPS structure database. Nucleic Acids Res 2007;35:D527-32. [Crossref] [PubMed]
- Abdelrazig S, Safo L, Rance GA, et al. Metabolic characterisation of Magnetospirillum gryphiswaldense MSR-1 using LC-MS-based metabolite profiling. RSC Adv 2020;10:32548-60. [Crossref] [PubMed]
- Kanehisa M, Goto S. KEGG: kyoto encyclopedia of genes and genomes. Nucleic Acids Res 2000;28:27-30. [Crossref] [PubMed]
- Gagnebin Y, Tonoli D, Lescuyer P, et al. Metabolomic analysis of urine samples by UHPLC-QTOF-MS: Impact of normalization strategies. Anal Chim Acta 2017;955:27-35. [Crossref] [PubMed]
- Thévenot EA, Roux A, Xu Y, et al. Analysis of the Human Adult Urinary Metabolome Variations with Age, Body Mass Index, and Gender by Implementing a Comprehensive Workflow for Univariate and OPLS Statistical Analyses. J Proteome Res 2015;14:3322-35. [Crossref] [PubMed]
- Xia J, Wishart DS. Web-based inference of biological patterns, functions and pathways from metabolomic data using MetaboAnalyst. Nat Protoc 2011;6:743-60. [Crossref] [PubMed]
- Kieffer DA, Piccolo BD, Vaziri ND, et al. Resistant starch alters gut microbiome and metabolomic profiles concurrent with amelioration of chronic kidney disease in rats. Am J Physiol Renal Physiol 2016;310:F857-71. [Crossref] [PubMed]
- Wishart DS. Emerging applications of metabolomics in drug discovery and precision medicine. Nat Rev Drug Discov 2016;15:473-84. [Crossref] [PubMed]
- Agarwal A, Sharma RK, Gupta S, et al. Sperm Vitality and Necrozoospermia: Diagnosis, Management, and Results of a Global Survey of Clinical Practice. World J Mens Health 2022;40:228-42. [Crossref] [PubMed]
- Dumont A, Barbotin AL, Lefebvre-Khalil V, et al. Necrozoospermia: From etiologic diagnosis to therapeutic management. Gynecol Obstet Fertil Senol 2017;45:238-48. [PubMed]
- Wiedeman AM, Barr SI, Green TJ, et al. Dietary Choline Intake: Current State of Knowledge Across the Life Cycle. Nutrients 2018;10:1513. [Crossref] [PubMed]
- Geer BW. Dietary choline requirements for sperm motility and normal mating activity in Drosophila melanogaster. Biol Bull 1967;133:548-66. [Crossref] [PubMed]
- Zhang J, Zhang Y, Liang C, et al. Choline supplementation alleviates fluoride-induced testicular toxicity by restoring the NGF and MEK expression in mice. Toxicol Appl Pharmacol 2016;310:205-14. [Crossref] [PubMed]
- Karakus C, Ozyurt R. Correlation between high Choline metabolite signal in spectroscopy and sperm retrieval chance at micro-TESE. Eur Rev Med Pharmacol Sci 2022;26:1125-30. [PubMed]
- Johnson AR, Craciunescu CN, Guo Z, et al. Deletion of murine choline dehydrogenase results in diminished sperm motility. FASEB J 2010;24:2752-61. [Crossref] [PubMed]
- Johnson AR, Lao S, Wang T, et al. Choline dehydrogenase polymorphism rs12676 is a functional variation and is associated with changes in human sperm cell function. PLoS One 2012;7:e36047. [Crossref] [PubMed]
- Zhang X, Diao R, Zhu X, et al. Metabolic characterization of asthenozoospermia using nontargeted seminal plasma metabolomics. Clin Chim Acta 2015;450:254-61. [Crossref] [PubMed]
- Reynolds S, Calvert SJ, Walters SJ, et al. NMR spectroscopy of live human asthenozoospermic and normozoospermic sperm metabolism. Reprod Fertil 2022;3:77-89. [Crossref] [PubMed]
- Dodo M, Kitamura H, Shima H, et al. Lactate dehydrogenase C is required for the protein expression of a sperm-specific isoform of lactate dehydrogenase A. J Biochem 2019;165:323-34. [Crossref] [PubMed]
- Intlekofer AM, Wang B, Liu H, et al. L-2-Hydroxyglutarate production arises from noncanonical enzyme function at acidic pH. Nat Chem Biol 2017;13:494-500. [Crossref] [PubMed]
- Hong CY, Shieh CC, Wu P, et al. Effect of phosphatidylcholine, lysophosphatidylcholine, arachidonic acid and docosahexaenoic acid on the motility of human sperm. Int J Androl 1986;9:118-22. [Crossref] [PubMed]
- Yu L, Yang X, Ma B, et al. Abnormal arachidonic acid metabolic network may reduce sperm motility via P38 MAPK. Open Biol 2019;9:180091. [Crossref] [PubMed]