A dynamic nomogram integrated with blood inflammation markers for predicting overall survival in patients with upper tract urothelial carcinoma
Highlight box
Key findings
• A dynamic nomogram was constructed based on inflammation markers and pathologic data to predict overall survival in patients with upper tract urothelial carcinoma.
What is known and what is new?
• The current prognostic tools and factors can no longer meet the demand of clinic. Blood inflammatory markers have shown promising prognostic value in a variety of cancers. However, until now, few inflammatory markers have been integrated into the nomogram models in UTUC.
• We developed a convenient dynamic nomogram that includes inflammatory markers, which performed well in both the training set and the validation set.
What is the implication, and what should change now?
• The prediction model may be useful for surgeons or oncologists in risk stratification and patient selection for more intensive therapy and closer follow-up.
Introduction
Although upper tract urothelial carcinoma (UTUC) accounts for only 5–10% of all urothelial malignancies (1), it has shown a gradual increase in incidence (2). Radical nephroureterectomy (RNU) with ipsilateral bladder-cuff excision is the industry-recognized standard surgical procedure for patients with localized disease (3). Due to the highly malignant nature of UTUC, approximately 40% of UTUC are fatal within 5 years (4). At present, some predictors related to oncological outcomes have been identified, such as pathological stage, pathological grade, status of lymph node, and lymphovascular invasion (LVI) (3). These prognostic factors, however, are still insufficient to predict the prognosis in UTUC. Hence, it makes sense to investigate new prognostic factors to determine the patient populations that would benefit from adjuvant chemotherapy or closer monitoring.
The importance of inflammation and the inflammatory microenvironment in tumorigenesis and tumor progression is being supported by a growing body of evidence (5). Inflammatory marker, as one of the objective indicators of reflecting the degree of host inflammation, has been increasingly associated with the prognosis of cancer. Previous studies have suggested that blood inflammation markers, including neutrophil-to-lymphocyte ratio (NLR), platelet-to-lymphocyte ratio (PLR), monocyte-to-lymphocyte ratio (MLR), prognostic nutritional index (PNI), albumin-to-globulin ratio (AGR), systemic immune−inflammation index (SII), etc., could be informative in predicting outcomes for urological tumors (6-11). Remarkably, one of them was hemoglobin-to-red cell distribution width ratio (HRR, Hb/RDW), which is a novel biomarker first proposed by Sun et al. in 2016 (12,13). However, because of the rarity of UTUC, most predictors were obtained based on pathological characteristics, and readily available preoperative indicators such as blood parameters were often ignored. Our primary goal was to identify powerful predictors from a large pool of potential biomarkers and to develop and externally validate a novel prediction model incorporating inflammation markers to predict overall survival (OS) in UTUC patients. We present this article in accordance with the TRIPOD reporting checklist (available at https://tau.amegroups.com/article/view/10.21037/tau-23-133/rc).
Methods
Patient selection
We included consecutive patients with non-metastatic UTUC treated with RNU at Huadong Hospital Affiliated to Fudan University from March 2010 to July 2022 in the training cohort. In addition, between January 2010 and April 2017, patients diagnosed with non-metastatic UTUC and underwent RNU at Huashan Hospital Affiliated to Fudan University were available for external validation. The exclusion criteria were shown as below: (I) patients who accepted neoadjuvant therapy; (II) patients who underwent red blood cell transfusions within one month before radical resection; (III) incomplete clinicopathological data and follow-up information. (IV) follow-up time <3 months. (V) incomplete blood tests within one week before RNU; (VI) patients who had concomitant autoimmune diseases, acute and chronic infectious diseases, hematological diseases or chronic liver diseases. In total, 167 and 137 subjects were enrolled in the training cohort and validation cohort respectively, and the patient selection process is illustrated by the flowchart in Figure 1.
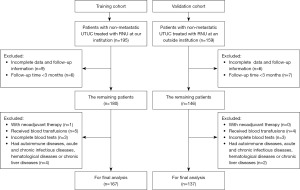
The study was conducted in accordance with the Declaration of Helsinki (as revised in 2013). Approval was granted by the Ethics Committee of Huadong Hospital Affiliated to Fudan University (No. 2022K116) and the Institutional Review Board of Huashan Hospital, Fudan University (No. 2019-010). Informed consent was waived by Ethics Committees due to the retrospective nature of the study.
Definitions and follow-up
The formulas for calculating the NLR, PLR, MLR, PNI, SII, AGR, and HRR were as follows: [NLR = neutrophil count (×109/L)/lymphocyte count (×109/L) (14)]; [PLR = platelet count (×109/L)/lymphocyte count (×109/L) (15)]; [MLR = monocyte count (×109/L)/lymphocyte count (×109/L) (16)];[PNI = serum albumin (g/L) + 5 × lymphocyte count (×109/L) (17)]; [SII = neutrophil count (×109/L) × platelet count (×109/L)/lymphocyte count (×109/L) (18)]; [AGR = serum albumin (g/L)/(total protein − serum albumin) (g/L) (19)]; [HRR = hemoglobin (g/dL)/red cell distribution width (%) (12)]; Laboratory analyses were performed using the Sysmex XN-2000 analyzer (Japan) and Hitachi 7170 Automatic Biochemical Analyzer (Japan) in training cohort, while blood tests were analysed using the Sysmex XN-1000 analyzer (Japan) and Hitachi 7600 Automatic Biochemical Analyzer (Japan) in validation cohort.
The pathological grade and stage were assessed in accordance with the 2022 World Health Organization (20) and the 8th Edition American Joint Committee of Cancer (AJCC) Tumor-Node-Metastasis (TNM) classification (21), respectively. Ipsilateral hydronephrosis state was determined from radiographic reports of preoperative computed tomography (CT) or magnetic resonance imaging (MRI). When the tumor grows in the renal pelvis and ureter simultaneously, tumor location was consistent with the location of dominant lesion determined in the following order of priority: stage, grade, and size.
Doctors assessed the patients every 3–4 months during the first year, and every 6 months for the following 2 years, and then annually thereafter. The way of evaluation contained history, physical examination, blood tests, urinary cytology, cystoscopy, chest radiography and abdominopelvic CT scan. OS was calculated as the interval from the date of surgery until the last follow-up visit or death from any cause.
Statistical analysis
Group comparison analyses were using the Student’s t-test or Mann-Whitney test (without normal distribution) for continuous variables and using Fisher ’s exact test and chi-squared test for noncontinuous variables. The receiver operating characteristic (ROC) curve was applied for selecting the best cut-off values. Variables with P<0.05 in a univariate Cox regression and considered potentially significant based on clinical experience and prior studies were included in the multivariate Cox regression analysis. Covariates multicollinearity was checked before constructing the model. Variance inflation factor (VIF) >4.0 in Collinearity diagnosis indicates the existence of close relations among the selected variables (22,23), which leads to false associations and potentially unreliable effect estimates.
Nomogram was generated based on multivariable regression coefficients of training cohort. The DynNom package was applied to construct a convenient web-based survival rate calculator. Discrimination validity of the prediction model was quantified by Harrell’s concordance index (c-index) and the areas under the ROC curves at 1-, 3- and 5-year. Calibration plots at 1-, 3- and 5-year were conducted with 1,000 bootstrap resampling to evaluate the calibration performance by comparing the model-predicted probabilities with the actual observed frequencies. To visualize and evaluate the clinical benefit of the nomogram, additionally we performed decision curve analysis (DCA). Finally, based on the total points from nomogram of each patient, the validation cohort was divided into three risk subgroups. The X-tile plot enables a comprehensive evaluation of every method for dividing a population into high- and low-risk subsets for survival. The “minimum P value” approach is then used to determine the best cut-off value for continuous variables (24). We used the Kaplan-Meier curve to assess OS, and the log-rank test for comparison among subgroups. All the above steps were conducted using R software (version 4.2.1, with survival, rms, ggprism, ggplot2, ggsci, Hmisc, riskRegression, prodlim, ggDCA, DynNom packages), IBM SPSS (version 26.0) and X-tile software (version 3.6.1). A two-tailed value of P<0.05 was considered statistically significant.
Results
Baseline characteristics
Table 1 provided a summary of baseline data of the two sets. In the training and validation cohorts, a total of 46 (27.5%) and 43 (31.4%) patients died of UTUC, and 57 (34.1%) and 51 (37.2%) patients died of any cause, respectively. Owing to the patient heterogeneities in different medical institutions, statistical differences in gender (P=0.02) and pathological stage (P=0.036) were discovered between the training set and the validation set.
Table 1
Variable | All patients (n=304) | Training cohort (n=167) | Validation cohort (n=137) | P value |
---|---|---|---|---|
Age, years, mean (±SD) | 67.09 (±10.38) | 67.95 (±10.35) | 66.05 (±10.36) | 0.567 |
Gender, n (%) | 0.020 | |||
Male | 189 (62.2) | 94 (56.3) | 95 (69.3) | |
Female | 115 (37.8) | 73 (43.7) | 42 (30.7) | |
Follow-up, months, median (IQR) | – | 55.5 (37.8–93.1) | 51.0 (25.1–74.8) | – |
Smoking history, n (%) | 0.432 | |||
Yes | 52 (17.1) | 26 (15.6) | 26 (19.0) | |
No | 252 (82.9) | 141 (84.4) | 111 (81.0) | |
Previous UCB, n (%) | 0.476 | |||
Yes | 23 (7.6) | 11 (6.6) | 12 (8.8) | |
No | 281 (92.4) | 156 (93.4) | 125 (91.2) | |
Hydronephrosis, n (%) | 0.717 | |||
Yes | 203 (66.8) | 113 (67.7) | 90 (65.7) | |
No | 101 (33.2) | 54 (32.3) | 47 (34.3) | |
Tumor location, n (%) | 0.679 | |||
Renal pelvis | 146 (48.0) | 82 (49.1) | 64 (46.7) | |
Ureter | 158 (52.0) | 85 (50.9) | 73 (53.3) | |
Pathological grade, n (%) | 0.227 | |||
High | 259 (85.2) | 146 (87.4) | 113 (82.5) | |
Low | 45 (14.8) | 21 (12.6) | 24 (17.5) | |
Pathological stage, n (%) | 0.036 | |||
Ta | 75 (24.7) | 44 (26.3) | 31 (22.6) | |
T1/Tis | 53 (17.4) | 29 (17.4) | 24 (17.5) | |
T2 | 50 (16.4) | 18 (10.8) | 32 (23.4) | |
T3 | 111 (36.5) | 65 (38.9) | 46 (33.6) | |
T4 | 15 (4.9) | 11 (6.6) | 4 (2.9) | |
Multifocality, n (%) | 0.414 | |||
Yes | 71 (23.4) | 42 (25.1) | 29 (21.2) | |
No | 233 (76.6) | 125 (74.9) | 108 (78.8) | |
LVI, n (%) | 0.320 | |||
Yes | 59 (19.4) | 29 (17.4) | 30 (21.9) | |
No | 245 (80.6) | 138 (82.6) | 107 (78.1) | |
Lymph node status, n (%) | 0.150 | |||
pN0 | 20 (6.6) | 15 (9.0) | 5 (3.6) | |
pNx | 275 (90.5) | 148 (88.6) | 127 (92.7) | |
pN+ | 9 (3.0) | 4 (2.4) | 5 (3.6) | |
Surgical margin, n (%) | 0.879 | |||
Positive | 28 (9.2) | 15 (9.0) | 13 (9.5) | |
Negative | 276 (90.8) | 152 (91.0) | 124 (90.5) | |
Surgical approach, n (%) | 0.108 | |||
Open | 149 (49.0) | 91 (54.5) | 58 (42.3) | |
Laparoscopic | 145 (47.7) | 71 (42.5) | 74 (54.0) | |
Robot‑assisted | 10 (3.3) | 5 (3.0) | 5 (3.6) |
SD, standard deviation; IQR, interquartile range; UCB, urothelial carcinoma of bladder; LVI, lymphovascular invasion.
Development of the dynamic nomogram
The general statistical characteristics of the seven inflammatory markers were presented in Table 2. The optimal cut-off points for NLR, PLR, MLR, PNI, SII, AGR, and HRR were identified as 2.334, 110.503, 0.462, 44.969, 681.783, 1.588, and 1.020 by calculating the maximal Youden Index. Hence, based on each threshold, all patients could be separated into two groups (low level and high level). High SII, low AGR, and low HRR were found to be significantly related to poor OS in the univariate regression analysis (P=0.022, 0.002, 0.011, respectively; Table 3). Hydronephrosis (P=0.009), advanced stage (P<0.001), multiple tumors (P=0.04), and positive surgical margin (P=0.012) were also associated with poor outcomes. Given the importance of pathological grade in predicting prognosis, pathological grade was also incorporated into the final Cox regression analysis (P=0.111).
Table 2
Representative value | NLR | PLR | MLR | PNI | SII | AGR | HRR |
---|---|---|---|---|---|---|---|
Mean | 3.371 | 152.722 | 0.295 | 47.979 | 723.605 | 1.538 | 0.963 |
Median | 2.719 | 135.790 | 0.255 | 48.004 | 602.090 | 1.519 | 0.960 |
Range | 19.262 | 409.178 | 0.728 | 26.673 | 3188.662 | 1.766 | 0.849 |
Standard deviation | 2.369 | 67.375 | 0.143 | 4.956 | 520.953 | 0.293 | 0.133 |
Cut-off points | 2.334 | 110.503 | 0.462 | 44.969 | 681.783 | 1.588 | 1.020 |
NLR, neutrophil-to-lymphocyte ratio; PLR, platelet-to-lymphocyte ratio; MLR, monocyte-to-lymphocyte ratio; PNI, prognostic nutritional index; SII, systemic immune−inflammation index; AGR, albumin-to-globulin ratio; HRR, hemoglobin-to-red cell distribution width ratio.
Table 3
Variables | Univariate Cox regression | VIF | Multivariate Cox regression | ||
---|---|---|---|---|---|
HR (95% CI) | P value | HR (95% CI) | P value | ||
Age, years (<68 vs. ≥68) | 1.293 (0.767–2.179) | 0.333 | |||
Gender (male vs. female) | 0.872 (0.518–1.470) | 0.607 | |||
Smoking history | 1.110 (0.561–2.196) | 0.765 | |||
Previous UCB | 1.113 (0.403–3.079) | 0.836 | |||
Hydronephrosis | 2.491 (1.258–4.931) | 0.009 | 1.105 | 1.796 (0.870–3.710) | 0.113 |
Tumor location (ureter vs. renal pelvis) | 1.146 (0.681–1.928) | 0.607 | |||
Pathologic grade (high vs. low) | 2.284 (0.826–6.313) | 0.111 | 1.388 | 0.477 (0.102–2.230) | 0.347 |
Pathological stage | – | <0.001 | 1.372 | – | <0.001 |
Ta | 1.000 | Referent | 1.000 | Referent | |
T1/Tis | 2.254 (0.782–6.499) | 0.133 | 2.527 (0.613–10.420) | 0.200 | |
T2 | 2.810 (0.905–8.721) | 0.074 | 3.545 (0.772–16.290) | 0.104 | |
T3 | 4.282 (1.785–10.271) | 0.001 | 4.196 (1.178–14.940) | 0.027 | |
T4 | 6.693 (2.148–20.849) | 0.001 | 11.344 (2.493–51.610) | 0.002 | |
Multifocality | 1.782 (1.026–3.096) | 0.040 | 1.050 | 1.362 (0.767–2.420) | 0.292 |
LVI | 1.434 (0.757–2.715) | 0.268 | |||
Surgical margin (positive vs. negative) | 2.642 (1.241–5.626) | 0.012 | 1.032 | 2.391 (1.082–5.290) | 0.031 |
Surgical approach | – | 0.070 | |||
Open | 1.000 | Referent | |||
Laparoscopic | 0.504 (0.274–0.926) | 0.027 | |||
Robot‑assisted | 0.963 (0.233–3.986) | 0.958 | |||
NLR (high level vs. low level) | 1.470 (0.833–2.593) | 0.184 | |||
PLR (high level vs. low level) | 1.504 (0.778–2.906) | 0.225 | |||
MLR (high level vs. low level) | 1.797 (0.930–3.472) | 0.081 | |||
PNI (low level vs. high level) | 0.891 (0.471–1.686) | 0.723 | |||
SII (high level vs. low level) | 1.840 (1.093–3.097) | 0.022 | 1.052 | 1.421 (0.828–2.440) | 0.202 |
AGR (low level vs. high level) | 2.580 (1.411–4.718) | 0.002 | 1.091 | 2.330 (1.240–4.380) | 0.009 |
HRR (low level vs. high level) | 2.437 (1.231–4.825) | 0.011 | 1.096 | 2.250 (1.104–4.590) | 0.026 |
VIF, variance inflation factor; UCB, urothelial carcinoma of the bladder; LVI, lymphovascular invasion; CI, confidence interval; HR, hazard ratio; NLR, neutrophil-to-lymphocyte ratio; PLR, platelet-to-lymphocyte ratio; MLR, monocyte-to-lymphocyte ratio; PNI, prognostic nutritional index; SII, systemic immune−inflammation index; AGR, albumin-to-globulin ratio; HRR, hemoglobin-to-red cell distribution width ratio.
VIF were all <4, which means that no variables entered into the multivariate regression had significant multicollinearity (Table 3). By adopting a forward selection method, multivariate regression analysis revealed that pathological stage (P<0.001), surgical margin (P=0.031), AGR level (P=0.009), HRR level (P=0.026) were independently associated with OS (Table 3). The prognostic nomogram was generated based on multivariate regression coefficients of these predictors (Figure 2). For convenience, we further constructed a web-based survival rate calculator (available from: https://dids.shinyapps.io/DynNomappOS/). With the help of this tool, it is quick and easy to determine the survival probability and its 95% confidence interval (95% CI) for UTUC patients at any time point after RNU (Figure 3). For example, for patients with T2 stage, negative margins, AGR <1.588 and HRR ≥1.020, the probability of OS at 18 months was approximately 0.88 (95% CI: 0.770–1.000) (Figure 3A,3B).
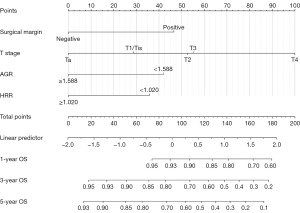
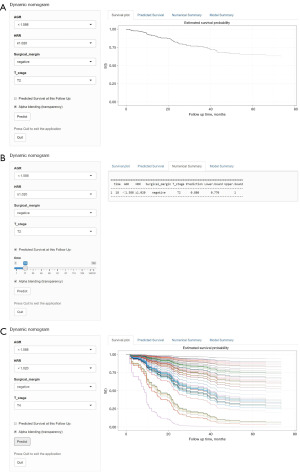
Performance and clinical utility of nomogram
The c-index value was 0.726 (95% CI: 0.666–0.786) in the training cohort while 0.761 (95% CI: 0.692–0.830) in the validation cohort. In the primary sample, the AUC of nomogram at 1-, 3- and 5-year were 0.765, 0.755, 0.763, respectively (Figure 4A). Meanwhile, the AUC of nomogram at 1-, 3- and 5-year were 0.791, 0.833, 0.802 in the validation sample (Figure 4B), which indicated that this model achieved a favorable discrimination accuracy. Additionally, both the internal and external validation calibration plots showed a subtle distinction between the predicted and the actual probabilities (Figure 5).
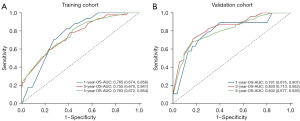
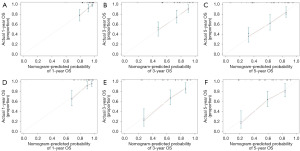
DCA offers a visual representation of clinical net benefit for model compared with scheme of intervention for all patients (oblique line) and for none (horizontal line). When the risk threshold was 0.2–0.9, the model showed favorable clinical net benefit gains in predicting 5-year OS (Figure 6A). Furthermore, using the nomogram to make treatment decision for the validation sample was satisfactory as long as the risk threshold was >0.2 (Figure 6B). When comparing our nomogram to the AJCC staging system, we found the nomogram performed better across nearly all risk thresholds in both the training and validation cohorts (Figure 6).
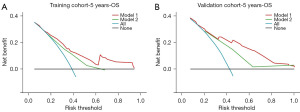
Use in risk stratification
The best cut-off values of total points were 94.5 and 130.5 by using X-tile (Figure 7A,7B), and the validation cohort was then divided into low risk subgroup (points ≤94.5), moderate risk subgroup (94.5< points ≤130.5), and high risk subgroup (points >130.5). The Kaplan-Meier curve and log-rank test showed that survival rates in the three risk subsets were statistically different from one another (5-year OS in low, moderate, high-risk subgroup were 81.3%, 61.0%, 19.6%, respectively, P<0.05; Figure 7C).
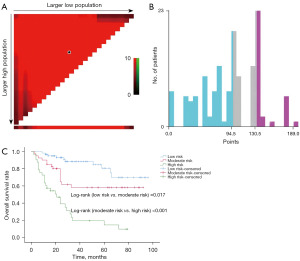
Discussion
Due to the peculiar nature of anatomic structures in the renal pelvis and ureter, preoperative evaluation of UTUC using modern medical imaging methods is currently difficult and may lead to under- or over-treatment (25). Preoperative blood inflammation markers may be useful in screening the cases that are suitable for kidney-sparing surgery with low-risk features or accepting neoadjuvant chemotherapy with a high likelihood of adverse outcomes (26,27). Unfortunately, none of the six prognostic models (28-33) recommended by the EAU guideline (3) took inflammatory markers into account in both univariate and multivariate analyses. Our study included a relatively comprehensive set of inflammatory indicators, aiming to select the powerful predictors from a large pool of biomarkers. The findings suggested that in addition to advanced T staging and positive surgical margin, low AGR (≤1.588) and low HRR (≤1.020) were independent risk factors of UTUC. As far as we know, this is the first prediction nomogram in UTUC that incorporated either AGR or HRR. In comparison with the AJCC staging system, the nomogram led to superior net benefit across nearly all threshold probabilities in both the training and validation cohorts. Finally, we divided the validation cohort into three risk subgroups according to the total points of patients from nomogram. The results showed that the high-risk subgroup was significantly worse than the other two subgroups in OS, suggesting that the high-risk subgroup may benefit more from a more aggressive treatment strategy.
By integrating multiple prognostic factors, the nomogram can predict the prognosis of patients and thus guide medication, operation and follow-up. The prognostic impact of T2 is very close to that of T3 as seen in our established nomogram, suggesting that the significance of preoperative differentiation between T2 and T3 may be limited, while preoperative identification of T4 is necessary. We also carried out a subgroup analysis in light of the possibility that pT3 renal pelvis carcinoma and pT3 ureteral carcinoma have different prognosis (34,35). Unfortunately, there was no statistically significant difference in OS between pT3 renal pelvis carcinoma, pT3 ureteral carcinoma, and pT2 UTUC (Figure S1). It might be due to the relatively small sample size. Nomograms may be useful, but it is not easy to convince doctors to use them in clinical practice. Therefore, we built a web-based tool that can dynamically predict the OS of UTUC patients based on the nomogram. We found a high risk of death in the first 3.5 years after RNU (Figure 3C) and therefore strongly recommend regular follow-up and treatment in the first 3.5 years for all cases.
Sufficient evidence supports that inflammation participates in disease progression of UTUC (36,37). As one of the components of AGR, serum albumin (ALB) is closely related to inflammation and cancer. Firstly, malignant tumors can induce systemic inflammation response and stimulate the production of cytokines such as interleukin (IL)-1β, IL-6 and tumor necrosis factor (TNF), which inhibited ALB synthesis and increase capillary permeability, thus leading to more ALB loss (38). Secondly, as an antioxidant, ALB produces an effect on keeping DNA replication stable and inhibiting abnormal cell proliferation (39,40). Lastly, albumins, as vital transporters, in turn affect the distribution and efficacy of anticancer drugs. In addition, hyperglobulinemia also reflects the status of an accumulative effect of exposure to inflammation (41). AGR consists of two identified predictors and is therefore more compelling in reflecting physical condition and reducing the perturbations of blood dilution or hemoconcentration. Interestingly, in addition to finding that low preoperative AGR was an independent risk factor for poor disease-free and OS, Fukushima et al. discovered that those patients with postoperative AGR recovery had a better prognosis than those without, but it did not achieve statistical significance (42). Although the recovery of the markers is much more affected by intensive postoperative medications (human albumin infusion, red blood cell transfusion, etc.), it provides for us a new perspective on how to optimize the prediction model in the future.
Since the HRR is a newly emerging biomark, studies involving its prognostic value in urothelial carcinoma continue to be scarce. Consistent with the previous study (43), the present study demonstrated that low HRR was associated with inferior survival and we obtained a cut-off value similar to that found in the previous study (1.02 and 1.05, respectively). Furthermore, the present study showed that low HRR had a comparable hazard ratio to positive margin status and low AGR (HR =2.250, 2.391, and 2.330, respectively) in predicting OS for non-metastatic UTUC treated RNU. According to the evidence presented above, it can be concluded that HRR is a reliable, efficient, and powerful predictor of UTUC.
The mechanism of alterations of HRR on oncogenesis and tumor development has not been fully elucidated at present. Cancer-related inflammation inhibits the production of erythropoietin (EPO) and promotes the release of immature red blood cells, thus leading to an increase in RDW and a drop in Hb (44). These alterations in RDW and Hb, in turn, will contribute to tumor invasion and metastasis further. The hypoxic microenvironment accelerates tumor neovascularization by stimulating the production of vascular endothelial growth factor (VEGF) when the number of red blood cells is reduced or their function is impaired (45). Compared to Hb or RDW alone, HRR could help to minimize the confounding factors caused by non-neoplastic diseases.
The present study is limited by the retrospective nature of design. Moreover, due to the retrospective nature of the study, laboratory analysis and adjuvant chemotherapy dose schedule were difficult to standardize across the two medical institutions. Also, patients with positive nodes were rare as regional or extended node dissection was not done routinely. Eventually the status of lymph nodes was not included in the regression analysis due to the imbalanced sample distribution. And finally, since the data of pathological stage and surgical margin were obtained postoperatively, the nomogram in this study is not capable of guiding neoadjuvant chemotherapy or lymph node dissection. However, at least we have confirmed the important prognostic value of AGR and HRR in UTUC, and we expect that AGR and HRR will be applied to pre-operative prediction models in the future. Therefore, further large-scale, prospective analyses are warranted to provide higher-level evidence.
Conclusions
In patients with UTUC after RNU, lower preoperative AGR and HRR were independent predictors of inferior survival. We developed a blood inflammation markers-based dynamic nomogram that predicted OS with good accuracy and calibration. Hence, these biomarkers and the proposed nomogram will be helpful to assist surgeons or oncologists in risk stratification and selection of patients for more intensive therapy and closer follow-up. Multi-institutional prospective validation work is necessary for future clinical use.
Acknowledgments
Funding: This work was supported by the Science and Technology Commission of Shanghai Municipality (No. 18411960700); and Key Specialized Disease of Huadong Hospital (No. ZDZB2222).
Footnote
Reporting Checklist: The authors have completed the TRIPOD reporting checklist. Available at https://tau.amegroups.com/article/view/10.21037/tau-23-133/rc
Data Sharing Statement: Available at https://tau.amegroups.com/article/view/10.21037/tau-23-133/dss
Peer Review File: Available at https://tau.amegroups.com/article/view/10.21037/tau-23-133/prf
Conflicts of Interest: All authors have completed the ICMJE uniform disclosure form (available at https://tau.amegroups.com/article/view/10.21037/tau-23-133/coif). The authors have no conflicts of interest to declare.
Ethical Statement: The authors are accountable for all aspects of the work in ensuring that questions related to the accuracy or integrity of any part of the work are appropriately investigated and resolved. The study was conducted in accordance with the Declaration of Helsinki (as revised in 2013). Approval was granted by the Ethics Committee of Huadong Hospital Affiliated to Fudan University (No. 2022K116) and the Institutional Review Board of Huashan Hospital, Fudan University (No. 2019-010). Informed consent was waived by Ethics Committees due to the retrospective nature of the study.
Open Access Statement: This is an Open Access article distributed in accordance with the Creative Commons Attribution-NonCommercial-NoDerivs 4.0 International License (CC BY-NC-ND 4.0), which permits the non-commercial replication and distribution of the article with the strict proviso that no changes or edits are made and the original work is properly cited (including links to both the formal publication through the relevant DOI and the license). See: https://creativecommons.org/licenses/by-nc-nd/4.0/.
References
- Siegel RL, Miller KD, Jemal A. Cancer statistics, 2016. CA Cancer J Clin 2016;66:7-30. [Crossref] [PubMed]
- van Doeveren T, van der Mark M, van Leeuwen PJ, et al. Rising incidence rates and unaltered survival rates for primary upper urinary tract urothelial carcinoma: a Dutch population-based study from 1993 to 2017. BJU Int 2021;128:343-51. [Crossref] [PubMed]
- Rouprêt M, Babjuk M, Burger M, et al. European Association of Urology Guidelines on Upper Urinary Tract Urothelial Carcinoma: 2020 Update. Eur Urol 2021;79:62-79. [Crossref] [PubMed]
- Ploussard G, Xylinas E, Lotan Y, et al. Conditional survival after radical nephroureterectomy for upper tract carcinoma. Eur Urol 2015;67:803-12. [Crossref] [PubMed]
- Coussens LM, Werb Z. Inflammation and cancer. Nature 2002;420:860-7. [Crossref] [PubMed]
- Widz D, Mitura P, Buraczynski P, et al. Preoperative Neutrophil-lymphocyte Ratio as a Predictor of Overall Survival in Patients with Localized Renal Cell Carcinoma. Urol J 2020;17:30-5. [PubMed]
- Wu R, Li D, Zhang F, et al. Prognostic Value of Platelet-to-Lymphocyte Ratio in Non-Muscle Invasive Bladder Cancer Patients: Intravesical Bacillus Calmette-Guerin Treatment After Transurethral Resection of Bladder Tumor. Front Surg 2022;9:907485. [Crossref] [PubMed]
- Chung JW, Ha YS, Kim SW, et al. The prognostic value of the pretreatment serum albumin to globulin ratio for predicting adverse pathology in patients undergoing radical prostatectomy for prostate cancer. Investig Clin Urol 2021;62:545-52. [Crossref] [PubMed]
- Ou N, Song Y, Liu M, et al. Development and Validation of a Nomogram to Predict Lymph Node Metastasis in Patients With T1 High-Grade Urothelial Carcinoma of the Bladder. Front Oncol 2020;10:532924. [Crossref] [PubMed]
- Ninomiya S, Kawahara T, Miyoshi Y, et al. A retrospective study on the possible systematic inflammatory response markers to predict the prognosis of patients with bladder cancer undergoing radial cystectomy. Mol Clin Oncol 2020;13:47. [Crossref] [PubMed]
- Rajwa P, Schuettfort VM, D'Andrea D, et al. Impact of systemic Immune-inflammation Index on oncologic outcomes in patients treated with radical prostatectomy for clinically nonmetastatic prostate cancer. Urol Oncol 2021;39:785.e19-27. [Crossref] [PubMed]
- Sun P, Zhang F, Chen C, et al. The ratio of hemoglobin to red cell distribution width as a novel prognostic parameter in esophageal squamous cell carcinoma: a retrospective study from southern China. Oncotarget 2016;7:42650-60. [Crossref] [PubMed]
- Yılmaz A, Yılmaz H, Tekin SB, et al. The prognostic significance of hemoglobin-to-red cell distribution width ratio in muscle-invasive bladder cancer. Biomark Med 2020;14:727-38. [Crossref] [PubMed]
- Racz JM, Cleghorn MC, Jimenez MC, et al. Predictive Ability of Blood Neutrophil-to-Lymphocyte and Platelet-to-Lymphocyte Ratios in Gastrointestinal Stromal Tumors. Ann Surg Oncol 2015;22:2343-50. [Crossref] [PubMed]
- Hu X, Tian T, Sun Q, et al. Prognostic value of the neutrophil-to-lymphocyte ratio and platelet-to-lymphocyte ratio in laryngeal cancer: What should we expect from a meta-analysis? Front Oncol 2022;12:945820. [Crossref] [PubMed]
- Nouri-Vaskeh M, Mirza-Aghazadeh-Attari M, Pashazadeh F, et al. Prognostic Impact of Monocyte to Lymphocyte Ratio in Clinical Outcome of Patients with Hepatocellular Carcinoma: A Systematic Review and Meta-analysis. Galen Med J 2020;9:e1948. [Crossref] [PubMed]
- Buzby GP, Mullen JL, Matthews DC, et al. Prognostic nutritional index in gastrointestinal surgery. Am J Surg 1980;139:160-7. [Crossref] [PubMed]
- Chen JH, Zhai ET, Yuan YJ, et al. Systemic immune-inflammation index for predicting prognosis of colorectal cancer. World J Gastroenterol 2017;23:6261-72. [Crossref] [PubMed]
- Suh B, Park S, Shin DW, et al. Low albumin-to-globulin ratio associated with cancer incidence and mortality in generally healthy adults. Ann Oncol 2014;25:2260-6. [Crossref] [PubMed]
- WHO. WHO classification of tumours: urinary and male genital tumours: International Agency for Research on Cancer; 2022.
- Amin MB, Greene FL, Edge SB, et al. The Eighth Edition AJCC Cancer Staging Manual: Continuing to build a bridge from a population-based to a more "personalized" approach to cancer staging. CA Cancer J Clin 2017;67:93-9.
- Yang X, Luo W, Han S, et al. Prevalence of high-risk coronary plaques in patients with and without metabolic syndrome and the relationship with prognosis. BMC Cardiovasc Disord 2020;20:73. [Crossref] [PubMed]
- Wu J, Zhang H, Li L, et al. A nomogram for predicting overall survival in patients with low‐grade endometrial stromal sarcoma: A population‐based analysis. Cancer Commun (Lond) 2020;40:301-12. [Crossref] [PubMed]
- Camp RL, Dolled-Filhart M, Rimm DL. X-tile: a new bio-informatics tool for biomarker assessment and outcome-based cut-point optimization. Clin Cancer Res 2004;10:7252-9. [Crossref] [PubMed]
- Janisch F, Shariat SF, Baltzer P, et al. Diagnostic performance of multidetector computed tomographic (MDCTU) in upper tract urothelial carcinoma (UTUC): a systematic review and meta-analysis. World J Urol 2020;38:1165-75. [Crossref] [PubMed]
- Tanaka N, Kikuchi E, Kanao K, et al. Impact of Combined Use of Blood-based Inflammatory Markers on Patients with Upper Tract Urothelial Carcinoma Following Radical Nephroureterectomy: Proposal of a Cumulative Marker Score as a Novel Predictive Tool for Prognosis. Eur Urol Focus 2015;1:54-63. [Crossref] [PubMed]
- Kim M, Moon KC, Choi WS, et al. Prognostic value of systemic inflammatory responses in patients with upper urinary tract urothelial carcinoma. World J Urol 2015;33:1439-57. [Crossref] [PubMed]
- Rouprêt M, Hupertan V, Seisen T, et al. Prediction of cancer specific survival after radical nephroureterectomy for upper tract urothelial carcinoma: development of an optimized postoperative nomogram using decision curve analysis. J Urol 2013;189:1662-9. [Crossref] [PubMed]
- Cha EK, Shariat SF, Kormaksson M, et al. Predicting clinical outcomes after radical nephroureterectomy for upper tract urothelial carcinoma. Eur Urol 2012;61:818-25. [Crossref] [PubMed]
- Yates DR, Hupertan V, Colin P, et al. Cancer-specific survival after radical nephroureterectomy for upper urinary tract urothelial carcinoma: proposal and multi-institutional validation of a post-operative nomogram. Br J Cancer 2012;106:1083-8. [Crossref] [PubMed]
- Seisen T, Colin P, Hupertan V, et al. Postoperative nomogram to predict cancer-specific survival after radical nephroureterectomy in patients with localised and/or locally advanced upper tract urothelial carcinoma without metastasis. BJU Int 2014;114:733-40. [Crossref] [PubMed]
- Ku JH, Moon KC, Jung JH, et al. External validation of an online nomogram in patients undergoing radical nephroureterectomy for upper urinary tract urothelial carcinoma. Br J Cancer 2013;109:1130-6. [Crossref] [PubMed]
- Krabbe LM, Eminaga O, Shariat SF, et al. Postoperative Nomogram for Relapse-Free Survival in Patients with High Grade Upper Tract Urothelial Carcinoma. J Urol 2017;197:580-9. [Crossref] [PubMed]
- Tai YS, Chen CH, Huang CY, et al. The effect of tumor location on oncologic outcomes in patients with upper urinary tract urothelial carcinoma stratified by pathologic stage. Urol Oncol 2016;34:4.e19-25. [Crossref] [PubMed]
- Yamada Y, Nakagawa T, Miyakawa J, et al. Subclassification of pT3 upper tract urothelial carcinoma: a multicenter retrospective study. World J Urol 2023;41:767-76. [Crossref] [PubMed]
- Goto K, Honda Y, Ikeda K, et al. Tumor heterogeneity evaluated by computed tomography detects muscle-invasive upper tract urothelial carcinoma that is associated with inflammatory tumor microenvironment. Sci Rep 2021;11:14251. [Crossref] [PubMed]
- Kang CH, Chiang PH, Huang SC. Correlation of COX-2 expression in stromal cells with high stage, high grade, and poor prognosis in urothelial carcinoma of upper urinary tracts. Urology 2008;72:153-7. [Crossref] [PubMed]
- Gupta D, Lis CG. Pretreatment serum albumin as a predictor of cancer survival: a systematic review of the epidemiological literature. Nutr J 2010;9:69. [Crossref] [PubMed]
- Roche M, Rondeau P, Singh NR, et al. The antioxidant properties of serum albumin. FEBS Lett 2008;582:1783-7. [Crossref] [PubMed]
- Anraku M, Shintomo R, Taguchi K, et al. Amino acids of importance for the antioxidant activity of human serum albumin as revealed by recombinant mutants and genetic variants. Life Sci 2015;134:36-41. [Crossref] [PubMed]
- Azab B, Kedia S, Shah N, et al. The value of the pretreatment albumin/globulin ratio in predicting the long-term survival in colorectal cancer. Int J Colorectal Dis 2013;28:1629-36. [Crossref] [PubMed]
- Fukushima H, Kobayashi M, Kawano K, et al. Prognostic Value of Albumin/Globulin Ratio in Patients with Upper Tract Urothelial Carcinoma Patients Treated with Radical Nephroureterectomy. Anticancer Res 2018;38:2329-34. [PubMed]
- Su YC, Wen SC, Li CC, et al. Low Hemoglobin-to-Red Cell Distribution Width Ratio Is Associated with Disease Progression and Poor Prognosis in Upper Tract Urothelial Carcinoma. Biomedicines 2021;9:672. [Crossref] [PubMed]
- Hirahara N, Tajima Y, Fujii Y, et al. Comprehensive Analysis of Red Blood Cell Distribution Width as a Preoperative Prognostic Predictor in Gastric Cancer. Anticancer Res 2019;39:3121-30. [Crossref] [PubMed]
- Wei YS, Zhou YG, Wang GY, et al. The impact of chemotherapy-associated hemoglobin on prognosis of colorectal cancer patients receiving adjuvant chemotherapy. Cancer Biomark 2017;20:627-35. [Crossref] [PubMed]