Gene methylation status in focus of advanced prostate cancer diagnostics and improved individual outcomes
Highlight box
Key findings
• This study introduces a dual-gene methylation diagnostic model for prostate cancer (PCa), featuring CCND2 and GSTP1, which exhibited excellent diagnostic accuracy.
• The model offering high sensitivity and specificity in diverse datasets and clinical samples.
• Pathway analysis revealed associations with cell cycle regulation and immune infiltration, indicating potential prognostic value.
What is known and what is new?
• DNA methylation plays a crucial role in cancer development, with specific genes displaying increased methylation in tumor tissues.
• This study introduces a novel dual-gene model, CCND2 and GSTP1, to enhance PCa diagnosis.
What is the implication, and what should change now?
• The dual-gene diagnostic model offers a promising approach for non-invasive PCa screening, potentially replacing traditional PSA-based methods. Further validation with liquid biopsy data is essential for clinical applications, enabling personalized and precise management.
Introduction
Prostate cancer (PCa) is the most prevalent type of male genitourinary tumor. The incidence and mortality of PCa in developing countries are steadily increasing, likely due to lifestyle changes and the widespread adoption of screening methods. Currently, PCa ranks as the second leading cause of cancer-related deaths worldwide (1,2).
Besides, the incidence of PCa showed younger trend with an increasing rate of metastatic PCa brought heavier social and economic burden (3). In this context, predictive, prevention and personalisation (3P) medicine is required for clinical management of PCa, which brings developing methods for early sensitive and economical diagnosis of PCa into focus (4,5).
The utilization of prostate-specific antigen (PSA) testing has significantly improved early detection, offering opportunities for timely intervention and better prognosis. However, PSA has its limitations. Conditions like benign prostatic hyperplasia (BPH) and digital rectal examination (DRE), as well as certain procedures, can elevate PSA levels, leading to unnecessary prostate biopsies or invasive tests. Moreover, a negative PSA result cannot entirely exclude the presence of cancer, particularly in patients with PSA levels ranging from 4 to 10 ng/mL, where distinguishing between benign and malignant cases based solely on screening results is challenging (6). Therefore, a more accurate screening method for PCa is necessary to enhance diagnostic precision and reduce false-positive results.
Tumor epigenetic changes, such as DNA methylation and histone modifications, have been extensively studied and are known to be closely associated with tumor initiation and progression (7). Detection of DNA methylation can help in the diagnosis of precancerous lesions and cancer (8). Furthermore, advancements in gene sequencing technology have lowered the cost of genetic testing, making epigenetic tumor testing more widely accessible. In this study, we established a reliable PCa DNA methylation diagnostic model by leveraging a DNA methylation database and validated it with clinical samples. This innovative approach conforms with principles of 3P medicine, and provides new insights and potential solutions for PCa screening and diagnosis. We present this article in accordance with the TRIPOD reporting checklist (available at https://tau.amegroups.com/article/view/10.21037/tau-23-405/rc).
Methods
Patients and samples
A total of 541 Genome-Wide DNA methylation profiles were collected from The Cancer Genome Atlas (TCGA) database, consisting of 491 PCa samples and 50 adjacent non-cancerous samples (with 50 paired cancerous samples included in the 491 tumor samples). These data were used to establish the diagnostic model. Additionally, we obtained 388 DNA methylation profiles from the Gene Expression Omnibus (GEO) database (GSE76938, GSE26126, GSE112047, and GSE84749), which included 219 PCa samples and 169 nontumor samples, for the purpose of validating the model. The development data and validation data shared the same criteria in eligibility outcomes, and predictors.
Regarding the datasets involved in this study, the DNA methylation data were generated using the Illumina Infinium Human Methylation 450 platform. Quality control and standardization for methylation followed the methods of the original research. In summary, the methylation beta score was calculated as: β = IntensityMethylated/(IntensityMethylated + IntensityUnmethylated). Beta scores with an associated detection P value of >0.01 were converted to “missing values”, and data were normalized using the ComBat R package. To further validate the model, we recruited 20 patients who underwent radical prostatectomy at Beijing Chao-Yang Hospital between June and October 2019. These patients had a pathological diagnosis of primary PCa and no history of radiotherapy, chemotherapy, or endocrine therapy before surgery. For each patient, we obtained both cancerous and adjacent non-cancerous tissue samples for DNA methylation analysis. The pathological results, as well as the judgment of tumors and adjacent samples, were carried out by pathological professionals.
The study was conducted in accordance with the Declaration of Helsinki (as revised in 2013). This study was approved by the ethics committee of Beijing Chao-Yang Hospital (No. 2021-54), and written informed consents for participants and publication were provided by all participants. The downloaded data in this study fully complied with the data access policies of GEO and TCGA.
Screening for differentially expressed genes and analysis of gene expression and DNA methylation
In previous studies and systematic reviews, six genes were identified as potential methylation markers in PCa (9-12). To validate the DNA methylation status of these genes, we employed the “minfi” R package, which allowed us to analyze the DNA methylation data from both TCGA and GEO databases. Additionally, we used the “MethylMix” R package to predict the impact of DNA methylation on gene expression, thereby identifying genes that were likely driven by DNA methylation. To assess the diagnostic performance of the identified genes, we employed receiver operating characteristic (ROC) curves.
Generation and validation of the diagnostic model
The diagnostic model was constructed using the Cox proportional hazards model. To evaluate the discriminatory ability of different categories within the Cox proportional hazards regression model, we calculated the Bayesian information criterion (BIC) values and Mallows’ Cp values. A lower BIC value or Mallows’ Cp value indicated a more effective diagnostic model.
To validate the model’s performance, we applied it to independent datasets, including GSE76938, GSE26126, GSE112047, GSE84749, and our own dataset. Risk score of each sample was calculated based on regression coefficients. We categorized samples into high- and low-risk groups based on the median risk score derived from the model. A higher model score was associated with a higher probability of cancer. Based on the scores obtained, we categorized all data into specific groups for further analysis and comparison.
DNA extraction and analysis of DNA methylation
Cancer and para-cancerous tissues for validation were obtained immediately after surgery, and isolated from paraffin section samples. DNA extraction was carried out using the GeneRead DNA FFPE kit (QIAGEN, 180134, Duesseldorf, Germany). Primers for the candidate genes were designed using the “Methylation FastTarget V4.1” and “primer3” (http://primer3.ut.ee) software. The optimized primers were combined into a multiplex polymerase chain reaction (PCR) primer panel. Subsequently, the EZ DNA Methylation-Gold Kit (Zymo Research, D5005, Orange County, California, USA) was utilized for DNA sample processing.
The target region of the transformed samples was amplified using the multiplex PCR primer panel, and the resulting PCR products from the same sample were mixed in equal proportions. Index sequences and specific tag sequences compatible with the Illumina platform were introduced via PCR. After the sample recovery process, the Agilent 2100 Bioanalyzer was employed to verify the length of sample DNA fragments. Subsequently, high-throughput sequencing was performed on the Illumina Hiseq platform using the 2×150 bp paired-end sequencing mode by a qualified genetic testing company.
Gene set enrichment analysis (GSEA) and immune infiltration
GSEA was utilized to explore potential biological processes and pathways with annotations from the msigdb.v7.0.entrez.gmt database. Pathway screening was performed by considering absolute values of negative normalized enrichment score (NES) greater than 2.0 and q-values less than or equal to 0.05.
For immune infiltration analysis, we utilized the Tumor Immune Estimation Resource (TIMER) database. Data from TCGA PCa samples were obtained, and the relationship between the expression of the target genes and the levels of tumor purity, B cells, CD4+ T cells, CD8+ T cells, macrophages, neutrophils, and dendritic cells was analyzed.
Statistical analysis
Statistical analysis was conducted through R (version 4.2.0). Differences in methylation levels were assessed by Wilcoxon rank sum test, P<0.05 was considered statistically significant.
Results
Identification and verification of the differentially expressed genes in PCa
In previous studies and systematic reviews (9-12), we identified APC, Cyclin-D2 (CCND2), glutathione S-transferase pi-1 (GSTP1), PRKY, RARB, and RASSF1 as potential methylation markers in PCa. To validate the differential methylation patterns of these six genes in PCa, we analyzed 491 PCa samples and 50 adjacent normal samples from the TCGA database (Figure 1A), characteristics of the datasets we used are shown in Table S1.
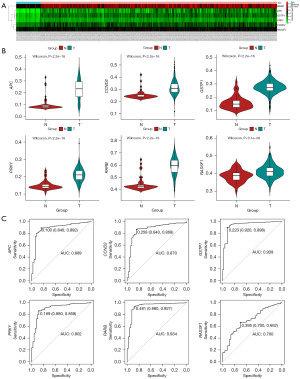
Comparing the methylation levels in PCa samples to adjacent normal samples, we found that each of the selected genes exhibited hypermethylation in PCa cases (Figure 1B). Additionally, we conducted ROC analysis, which demonstrated that each gene had a favorable diagnostic ability and could effectively distinguish tumor tissues from adjacent normal tissues. Among the six single-gene diagnostic models, GSTP1 showed the highest diagnostic accuracy, with an area under the curve (AUC) of 0.939 (Figure 1C).
Dual-gene diagnostic model for PCa
To enhance the diagnostic efficacy of our model, we sought to identify a more effective diagnostic combination from the six candidate genes. By evaluating the BIC and Mallows’ Cp value, we determined that the model comprising two genes exhibited the most favorable BIC and Mallows’ Cp values, indicating superior diagnostic performance (Figure 2A,2B).
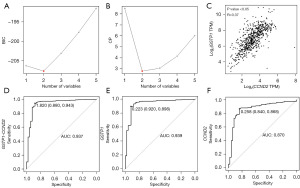
We further assessed the adjusted R2 values (adjR2) of each gene and found that CCND2 and GSTP1 had the two highest adjR2 values. Thus, we selected CCND2 and GSTP1 as the optimal combination for the PCa diagnostic model. Additionally, to ensure that there was no correlation or interaction between the two genes, we calculated the Variance Inflation Factor (VIF), which confirmed that the VIF for these two genes was 1.314, signifying no significant interaction between them (Figure 2C).
Remarkably, the dual-gene model (regression coefficients of CCND2, 0.5469989; GSTP1, 2.6733119) demonstrated excellent diagnostic ability, achieving an AUC of 0.937, outperforming the diagnostic ability of either the GSTP1 or CCND2 single-gene models (Figure 2D-2F).
Evaluation of diagnostic efficacy
To validate the robustness of the CCND2 and GSTP1 dual-gene diagnostic model, we tested its diagnostic performance on four external datasets (GSE76938, GSE26126, GSE112047, GSE84749). In all four external datasets, the methylation levels of the CCND2 and GSTP1 genes were significantly elevated in tumor tissues compared to normal tissues (Figure 3).
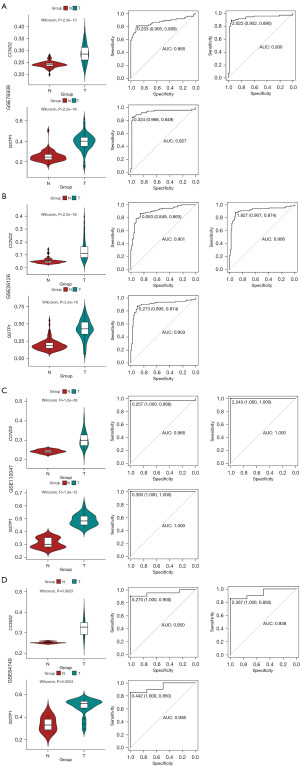
After calculating risk scores of each sample by our model based on CCND2 and GSTP1 methylation, the ROC demonstrated outstanding diagnostic performance. Specifically, the AUC values for the GSE76938, GSE26126, GSE112047, and GSE84749 datasets were 0.930, 0.906, 1.000, and 0.938, respectively. Importantly, the diagnostic performance of the dual-gene model surpassed that of any single-gene diagnostic models based on CCND2 or GSTP1 alone (Figure 3). Detailed data can be found in the online table (available at https://cdn.amegroups.cn/static/public/tau-23-405-1.pdf).
These results confirm that the CCND2 and GSTP1 dual-gene diagnostic model exhibits consistent and superior diagnostic ability across multiple datasets, making it a promising tool for PCa diagnosis.
CCND2 and GSTP1 may be methylation driver genes
In light of recent research, it has been observed that several genes do not show changes in gene expression despite significant alterations in their methylation levels. To investigate this further, we proceeded to examine the correlation between gene expression and methylation levels in CCND2 and GSTP1. For this purpose, we utilized the TCGA PCa dataset and the GSE84749 dataset.
The scatter plot analysis demonstrated a significant negative correlation between methylation levels and gene expression for both CCND2 and GSTP1 in both datasets (TCGA and GEO). This finding indicates that increased methylation levels of CCND2 and GSTP1 are associated with down-regulation of gene expression, suggesting that these two genes may be influenced by DNA methylation (Figure 4A,4B).
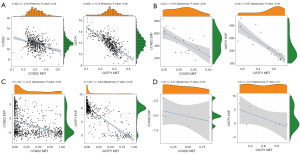
Subsequently, we performed correlation analysis of gene methylation and expression using the Cancer Cell Line Encyclopedia (CCLE) dataset. In pan-cancer samples (n=1,457), we observed significant negative correlations between CCND2 and GSTP1 methylation and expression, with correlation coefficients of −0.41 and −0.83, respectively. When specifically focusing on PCa samples (n=6), the small sample size limited statistical significance, but a negative correlation trend between methylation and expression still existed (Figure 4C,4D).
These results provide compelling evidence that the methylation levels of CCND2 and GSTP1 inversely influence their gene expression, supporting the notion that these genes are likely regulated by DNA methylation.
Pathway enrichment and immunoassay
The GSEA revealed that after screening, 2,977 signaling pathways or biological processes exhibited varying degrees of enrichment in high-score samples. Among these, ten pathways were related to PCa, cell cycle regulation, and immune infiltration, displaying high NES values. The cell cycle regulation pathways were positively correlated with the model score, while immune cell migration, chemotaxis, and immune regulation pathways showed a negative correlation with the model score. This suggests that higher model scores are associated with inhibited migration and chemotaxis of immune cells.
Moreover, the pathways classified by the model score displayed the same enrichment trend as PCa-related pathways in multiple gene sets, indicating the accuracy of the two-gene model for PCa diagnosis (Figure 5).
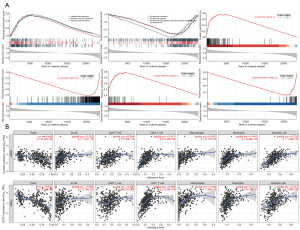
The TIMER analysis further revealed that in PCa samples, the expression of CCND2 and GSTP1 genes was negatively correlated with tumor purity, with R-values of −0.448 and −0.577, respectively. This indicates that increased expression of these two genes is linked to a significant reduction in tumor purity. Conversely, the infiltration of B cells, CD4+ T cells, CD8+ T cells, macrophages, neutrophils, and dendritic cells is enhanced with upregulated gene expression.
In summary, the findings suggest that the increased expression of CCND2 and GSTP1 leads to elevated immune cell infiltration, reduced tumor purity, and decreased tumor risk, which aligns with the outcomes predicted by our model.
Validation in clinical samples
To further validate the diagnostic performance of the dual-gene model in clinical samples, we obtained DNA from PCa and adjacent tissues of 20 PCa patients who underwent radical prostatectomy at our hospital. DNA methylation sequencing targeting the six candidate genes was conducted on these samples.
Among the 20 paired samples, it was found that six genes exhibited significantly elevated methylation levels in PCa tissues compared to adjacent tissues. Both CCND2 and GSTP1 demonstrated excellent diagnostic performance in the clinical samples. Remarkably, when these two targets were combined, the AUC reached 0.980 in the ROC analysis. This result provides compelling evidence that our model exhibits high-level diagnostic accuracy in clinical samples (Figure 6).
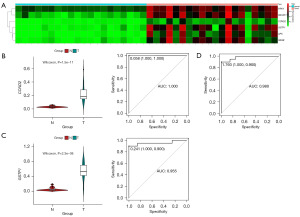
The findings from our analysis of clinical samples further support the robustness and reliability of the CCND2 and GSTP1 dual-gene diagnostic model for PCa diagnosis in a real-world clinical setting.
Discussion
In recent years, identifying the molecular characteristics of tumor tissue to determine tumor occurrence and prognosis has become a novel and essential research area (13). DNA methylation, an important epigenetic modification pathway, is frequently associated with the development and progression of tumors through changes in the methylation levels of proto-oncogenes and tumor suppressor genes (14). For instance, the APC gene exhibits high methylation levels in liver cancer, colorectal cancer, PCa, and other tumor tissues (15-17). Similarly, the GSTP1 gene shows high methylation levels in PCa, breast cancer, lung cancer, hepatocellular carcinoma, and other tumor tissues (18). Thus, the detection of methylation levels in specific genes can play a crucial role in tumor diagnosis. Moreover, epigenetic changes such as DNA methylation are more stable in tumors, making them promising biomarkers for clinical use (19).
In our study, we screened six genes with elevated methylation levels in various tumor tissues through a systematic review of research related to DNA methylation. These six genes exhibited acceptable diagnostic performance in PCa based on methylation data. However, the diagnostic performance of individual single-gene models has not yet reached the desired level of accuracy. To enhance the diagnostic ability of the model, we developed a dual-gene PCa diagnostic model consisting of the CCND2 and GSTP1 genes from the pool of six candidate genes. This dual-gene model demonstrated excellent diagnostic performance in the TCGA dataset with an AUC of 0.937, specificity of 88%, and sensitivity of 94.3%.
GSTP1 is a 3.2 kb gene comprising nine exons. It plays a crucial role in safeguarding cells from carcinogens and cytotoxins, exhibiting diverse physiological functions, such as neutralizing potentially genotoxic complexes, metabolizing various carcinogens, and minimizing cellular DNA damage (18). In lung cancer research, GSTP1 is responsible for eliminating carcinogens present in tobacco and is involved in the development of smoking-related lung cancer (20). In the context of epigenetic modifications, the promoter region of GSTP1 is frequently influenced by methylation. Hypermethylation in this region can lead to the silencing of GSTP1 expression, resulting in a compromised detoxification and antioxidant capacity. Numerous studies have established a significant association between GSTP1 methylation and the occurrence, recurrence, and prognosis of PCa, suggesting its potential as an informative epigenetic marker (21-23).
CCND2 is a crucial cell cycle regulator gene that has been identified as a proto-oncogene. Its overexpression has been linked to the progression and unfavorable prognosis of gastric cancer, colon cancer, ovarian cancer, and other tumors (24-26). Interestingly, elevated methylation in the promoter region of the CCND2 gene hinders its expression. Considering its role as a proto-oncogene, it might be expected that hypermethylation would inhibit tumor occurrence. However, it has been observed that reduced CCND2 expression resulting from promoter methylation is also associated with the advancement of breast cancer, lung cancer, and pancreatic cancer, suggesting that CCND2 silencing may contribute to tumor promotion (27,28).
Similarly, in PCa, increased CCND2 promoter methylation is linked to reduced CCND2 mRNA expression and is correlated with higher Gleason scores and tumor aggressiveness (29). Nevertheless, the potential of CCND2 as a biomarker for the early diagnosis of PCa has not been fully substantiated. Further research is required to elucidate its precise role and diagnostic significance in PCa detection.
In our study, we introduced a novel dual-gene model comprising CCND2 and GSTP1 as a combined diagnostic tool for PCa. This approach significantly enhanced the sensitivity and specificity of PCa diagnosis. The diagnostic performance of the model was further confirmed in multiple datasets, with specificity exceeding 85% and sensitivity exceeding 90.7%.
To bolster the reliability of our model, we included an additional 20 PCa samples in our study. These samples were analyzed to further validate and strengthen the diagnostic accuracy of the dual-gene model in real-world clinical settings.
The methylation sequencing analysis demonstrated hypermethylation of CCND2 and GSTP1 genes in clinical samples. The dual-gene diagnostic model exhibited excellent diagnostic performance in these samples, achieving an AUC of 0.980, sensitivity of 90%, and specificity of 100%. Compared to the PSA test, our model demonstrated evident diagnostic advantages, with significantly improved sensitivity and specificity. Although our model demonstrated excellent accuracy in diagnosis, 20 paired clinical samples are still relatively small, a larger number of samples are needed to further prove the accuracy of the model.
In the context of tumor development, only a few genes tend to play dominant or driving roles, while most gene expression changes are often associated with tumor progression. DNA methylation exhibits a similar effect, and it is important to identify whether changes in gene DNA methylation drive alterations in gene expression. Genes whose expression levels are influenced by methylation changes are often referred to as methylation-driven genes (30). To ascertain whether the genes involved in our diagnostic model are methylation-driven, we analyzed the TCGA PCa dataset and GSE84749 dataset. The expression of CCND2 and GSTP1 genes decreased significantly with an increase in their respective methylation levels, indicating that these two genes might be methylation-driven. This finding was further supported by the analysis of the CCLE database, where a significant negative correlation trend between methylation levels and gene expression was observed in pan-cancer tissue samples.
We conducted GSEA analysis to compare the gene expression profiles of different model scores and the pathways from multiple gene sets. We found that immune cell migration, chemotaxis, and immune regulation pathways were down-regulated in samples with high model scores, suggesting that our model has good performance in diagnosing PCa and may also have prognostic value.
Furthermore, our study analyzed the immune infiltration and tumor purity of CCND2 and GSTP1 genes in PCa tissue. It was confirmed that in PCa samples, higher expression levels of these two genes were associated with significantly decreased tumor purity and increased infiltration of B cells, T cells, macrophages, and other immune cells. This suggests that the overexpression of CCND2 and GSTP1 is linked to higher immune cell infiltration and lower tumor purity, and the methylation process of these two genes may reduce their expression, thereby increasing tumor risk.
Conclusions
In conclusion, the dual-gene methylation diagnosis model can serve as an independent and effective method for PCa diagnosis. When combined with liquid biopsy technology, it has the potential to enhance the accuracy of non-invasive diagnosis. Liquid biopsy methods are currently widely used for detecting various components like tumor cells, tumor DNA, RNA, and exosomes (31). A significant portion of cellular components present in urine originates from the prostate, making it an attractive choice for non-invasive PCa screening (32,33).
The diagnostic model developed in our study will undergo further validation using urine samples’ sequencing data. This new approach holds promise as a non-invasive and reliable PCa screening method, potentially replacing the traditional PSA-based screening method. By integrating the dual-gene diagnostic model with liquid biopsy-based urine testing, clinicians may have a more accurate and less invasive tool for early detection and diagnosis of PCa.
Urine-based liquid biopsies also facilitated stratification for PCa patients, as well as prognostic prediction (34-36), all of which contribute to personalized and precise management of individual patient to achieve the purpose of predictive preventive personalised medicine.
Acknowledgments
Funding: None.
Footnote
Reporting Checklist: The authors have completed the TRIPOD reporting checklist. Available at https://tau.amegroups.com/article/view/10.21037/tau-23-405/rc
Data Sharing Statement: Available at https://tau.amegroups.com/article/view/10.21037/tau-23-405/dss
Peer Review File: Available at https://tau.amegroups.com/article/view/10.21037/tau-23-405/prf
Conflicts of Interest: All authors have completed the ICMJE uniform disclosure form (available at https://tau.amegroups.com/article/view/10.21037/tau-23-405/coif). The authors have no conflicts of interest to declare.
Ethical Statement: The authors are accountable for all aspects of the work in ensuring that questions related to the accuracy or integrity of any part of the work are appropriately investigated and resolved. The study was conducted in accordance with the Declaration of Helsinki (as revised in 2013). This study was approved by the ethics committee of Beijing Chao-Yang Hospital (No. 2021-54), and written informed consents for participants and publication were provided by all participants.
Open Access Statement: This is an Open Access article distributed in accordance with the Creative Commons Attribution-NonCommercial-NoDerivs 4.0 International License (CC BY-NC-ND 4.0), which permits the non-commercial replication and distribution of the article with the strict proviso that no changes or edits are made and the original work is properly cited (including links to both the formal publication through the relevant DOI and the license). See: https://creativecommons.org/licenses/by-nc-nd/4.0/.
References
- Siegel RL, Miller KD, Fuchs HE, et al. Cancer statistics, 2022. CA Cancer J Clin 2022;72:7-33. [Crossref] [PubMed]
- Baciarello G, Gizzi M, Fizazi K. Advancing therapies in metastatic castration-resistant prostate cancer. Expert Opin Pharmacother 2018;19:1797-804. [Crossref] [PubMed]
- Kucera R, Pecen L, Topolcan O, et al. Prostate cancer management: long-term beliefs, epidemic developments in the early twenty-first century and 3PM dimensional solutions. EPMA J 2020;11:399-418. [Crossref] [PubMed]
- Mazurakova A, Samec M, Koklesova L, et al. Anti-prostate cancer protection and therapy in the framework of predictive, preventive and personalised medicine - comprehensive effects of phytochemicals in primary, secondary and tertiary care. EPMA J 2022;13:461-86. [Crossref] [PubMed]
- Ellinger J, Alajati A, Kubatka P, et al. Prostate cancer treatment costs increase more rapidly than for any other cancer-how to reverse the trend? EPMA J 2022;13:1-7. [Crossref] [PubMed]
- Albertsen PC. PSA testing, cancer treatment, and prostate cancer mortality reduction: What is the mechanism? Urol Oncol 2023;41:78-81. [Crossref] [PubMed]
- Dai E, Zhu Z, Wahed S, et al. Epigenetic modulation of antitumor immunity for improved cancer immunotherapy. Mol Cancer 2021;20:171. [Crossref] [PubMed]
- Crigna AT, Samec M, Koklesova L, et al. Cell-free nucleic acid patterns in disease prediction and monitoring-hype or hope? EPMA J 2020;11:603-27. [Crossref] [PubMed]
- Chen Y, Li J, Yu X, et al. APC gene hypermethylation and prostate cancer: a systematic review and meta-analysis. Eur J Hum Genet 2013;21:929-35. [Crossref] [PubMed]
- Gurioli G, Salvi S, Martignano F, et al. Methylation pattern analysis in prostate cancer tissue: identification of biomarkers using an MS-MLPA approach. J Transl Med 2016;14:249. [Crossref] [PubMed]
- Bakavicius A, Daniunaite K, Zukauskaite K, et al. Urinary DNA methylation biomarkers for prediction of prostate cancer upgrading and upstaging. Clin Epigenetics 2019;11:115. [Crossref] [PubMed]
- Dasari VK, Goharderakhshan RZ, Perinchery G, et al. Expression analysis of Y chromosome genes in human prostate cancer. J Urol 2001;165:1335-41. [Crossref] [PubMed]
- Grady WM, Yu M, Markowitz SD. Epigenetic Alterations in the Gastrointestinal Tract: Current and Emerging Use for Biomarkers of Cancer. Gastroenterology 2021;160:690-709. [Crossref] [PubMed]
- Hogg SJ, Beavis PA, Dawson MA, et al. Targeting the epigenetic regulation of antitumour immunity. Nat Rev Drug Discov 2020;19:776-800. [Crossref] [PubMed]
- Guo Y, Zhao YR, Liu H, et al. EHMT2 promotes the pathogenesis of hepatocellular carcinoma by epigenetically silencing APC expression. Cell Biosci 2021;11:152. [Crossref] [PubMed]
- Rajamäki K, Taira A, Katainen R, et al. Genetic and Epigenetic Characteristics of Inflammatory Bowel Disease-Associated Colorectal Cancer. Gastroenterology 2021;161:592-607. [Crossref] [PubMed]
- Şeref C, Acar Ö, Kılıç M, et al. Histologically benign PI-RADS 4 and 5 lesions contain cancer-associated epigenetic alterations. Prostate 2022;82:145-53. [Crossref] [PubMed]
- Cui J, Li G, Yin J, et al. GSTP1 and cancer: Expression, methylation, polymorphisms and signaling Int J Oncol 2020;56:867-78. (Review). [Crossref] [PubMed]
- Luo H, Wei W, Ye Z, et al. Liquid Biopsy of Methylation Biomarkers in Cell-Free DNA. Trends Mol Med 2021;27:482-500. [Crossref] [PubMed]
- Nørskov MS, Dahl M, Tybjærg-Hansen A. Genetic Variation in GSTP1, Lung Function, Risk of Lung Cancer, and Mortality. J Thorac Oncol 2017;12:1664-72. [Crossref] [PubMed]
- Porzycki P, Ciszkowicz E. Modern biomarkers in prostate cancer diagnosis. Cent European J Urol 2020;73:300-6. [PubMed]
- Maldonado L, Brait M, Loyo M, et al. GSTP1 promoter methylation is associated with recurrence in early stage prostate cancer. J Urol 2014;192:1542-8. [Crossref] [PubMed]
- Fiano V, Zugna D, Grasso C, et al. DNA methylation in repeat negative prostate biopsies as a marker of missed prostate cancer. Clin Epigenetics 2019;11:152. [Crossref] [PubMed]
- Shan YS, Hsu HP, Lai MD, et al. Cyclin D1 overexpression correlates with poor tumor differentiation and prognosis in gastric cancer. Oncol Lett 2017;14:4517-26. [Crossref] [PubMed]
- Zhuo S, Sun M, Bai R, et al. Long intergenic non-coding RNA 00473 promotes proliferation and migration of gastric cancer via the miR-16-5p/CCND2 axis and by regulating AQP3. Cell Death Dis 2021;12:496. [Crossref] [PubMed]
- Hua M, Qin Y, Sheng M, et al. miR-145 suppresses ovarian cancer progression via modulation of cell growth and invasion by targeting CCND2 and E2F3. Mol Med Rep 2019;19:3575-83. [Crossref] [PubMed]
- Matsubayashi H, Sato N, Fukushima N, et al. Methylation of cyclin D2 is observed frequently in pancreatic cancer but is also an age-related phenomenon in gastrointestinal tissues. Clin Cancer Res 2003;9:1446-52. [PubMed]
- Hung CS, Wang SC, Yen YT, et al. Hypermethylation of CCND2 in Lung and Breast Cancer Is a Potential Biomarker and Drug Target. Int J Mol Sci 2018;19:3096. [Crossref] [PubMed]
- Henrique R, Costa VL, Cerveira N, et al. Hypermethylation of Cyclin D2 is associated with loss of mRNA expression and tumor development in prostate cancer. J Mol Med (Berl) 2006;84:911-8. [Crossref] [PubMed]
- Traube FR, Carell T. The chemistries and consequences of DNA and RNA methylation and demethylation. RNA Biol 2017;14:1099-107. [Crossref] [PubMed]
- Ignatiadis M, Sledge GW, Jeffrey SS. Liquid biopsy enters the clinic - implementation issues and future challenges. Nat Rev Clin Oncol 2021;18:297-312. [Crossref] [PubMed]
- van der Pol Y, Moldovan N, Ramaker J, et al. The landscape of cell-free mitochondrial DNA in liquid biopsy for cancer detection. Genome Biol 2023;24:229. [Crossref] [PubMed]
- Matuszczak M, Schalken JA, Salagierski M. Prostate Cancer Liquid Biopsy Biomarkers' Clinical Utility in Diagnosis and Prognosis. Cancers (Basel) 2021;13:3373. [Crossref] [PubMed]
- Crocetto F, Russo G, Di Zazzo E, et al. Liquid Biopsy in Prostate Cancer Management-Current Challenges and Future Perspectives. Cancers (Basel) 2022;14:3272. [Crossref] [PubMed]
- McKiernan J, Donovan MJ, O'Neill V, et al. A Novel Urine Exosome Gene Expression Assay to Predict High-grade Prostate Cancer at Initial Biopsy. JAMA Oncol 2016;2:882-9. [Crossref] [PubMed]
- Kohaar I, Petrovics G, Srivastava S. A Rich Array of Prostate Cancer Molecular Biomarkers: Opportunities and Challenges. Int J Mol Sci 2019;20:1813. [Crossref] [PubMed]