The effect of Astragali Radix-Radix Angelica Sinensis on acute kidney injury: a network pharmacology and molecular docking study
Highlight box
Key findings
• We investigated the active ingredients and action targets of Astragali Radix (AR)-Radix Angelica Sinensis (RAS).
What is known and what is new?
• AR can dilate blood vessels, reduce blood pressure, protect red blood cells, increase renal blood flow, and protect the kidney. RAS has a wide range of pharmacological effects, including reducing serum creatinine concentration, increasing the activity of renal ATPase, and reducing renal ischemia-reperfusion injury.
• This study used a network pharmacology approach to investigate the connection among multicomponents, multitargets, and the regulation of multiple signal pathways related to the effect of AR-RAS on acute kidney injury (AKI).
What is the implication, and what should change now?
• Our study not only provides important information for further understanding the interaction mechanism of active components, targets and disease, but provides also research ideas for treatment of AKI with traditional Chinese medicine. However, based on data analysis, this study only provides a theoretical reference for revealing the pharmacological mechanism, and thus further experiments in vitro and in vivo are still needed to verify our findings.
Introduction
Acute kidney injury (AKI), which is also known as acute renal failure (ARF), is a common and serious condition caused by a variety of factors, such as nephrotoxic medications, ischemia, trauma and urinary tract blockage (1). It is an independent risk factor for death, with mortality rates of 25% overall (2). The pathogenesis of AKI involves vasoconstriction, oxidative stress, apoptosis, inflammation, and hypoxia (3-5). At present, except for intermittent hemodialysis and continuous renal replacement therapy, there are no effective drugs or treatment measures that can significantly contribute to AKI prevention. Therefore, new therapies with better efficacy and safety in treatment of AKI are urgently needed. In recent years, the research on drug treatment of AKI has subsequently intensified within this field.
Astragali Radix (AR)-Radix Angelica Sinensis (RAS) is made up of two specific herbs. AR can dilate blood vessels, reduce blood pressure, protect red blood cells, increase renal blood flow, and protect the kidney (6). Renowned for its active components, RAS has a wide range of pharmacological effects (7), including reducing serum creatinine concentration, increasing the activity of renal ATPase, and reducing renal ischemia-reperfusion injury (8). The AR-RAS combination has been shown to reduce the level of serum creatinine and renal tissue injury caused by acute ischemia-reperfusion in rats (9). Modern clinical data have effectively confirmed that AR-RAS are safe and effective herbs, which has significant effects on AKI and can profoundly promote recovery of renal function (10,11). However, the molecular mechanism of AR-RAS has yet to be fully understood, and research concerning AR-RAS in AKI treatment is limited. Therefore, this study aimed to deduce the bioactive AR-RAS components and their AKI-linked activity.
In recent years, computer technology, network pharmacology, and systems biology have developed vigorously. Network pharmacology, based on theoretical system biology, is an emerging field (12). Through the integration of multidisciplinary technology and content, a disease-drug-target network can be constructed to investigate the relationship between illnesses and medicines, which is consistent with the overall syndrome differentiation theory of traditional Chinese medicine (TCM) (13). This tool can be used to provide deeper insight into the true potential of certain medicines (14,15).
Using network pharmacology and molecular docking technologies, we set out to identify the active ingredients and action targets of AR-RAS. We present this article in accordance with the STREGA reporting checklist (available at https://tau.amegroups.com/article/view/10.21037/tau-23-562/rc).
Methods
Screening active components of AR-RAS
The Traditional Chinese Medicine Systems Pharmacology Database and Analysis Platform (TCMSP, http://tcmspw.com) was used to find the active chemicals of AR-RAS and their target proteins, and to discern the connections between illnesses, targets, and medicines (16). Oral bioavailability (OB) and drug-likeness (DL) of >30% and >0.18, respectively, were the screening criteria (17). Furthermore, in the UniProt (https://www.uniprot.org/) database, the appropriate targets of the aforementioned chemical components were identified, and the target gene annotation was completed by entering the species name “Homo sapiens”.
AKI-related target screening
The AKI-related targets were gathered from the five well-known disease databases: (I) GeneCards (18) (http://www.genecards.org); (II) Online Mendelian Inheritance in Man [OMIM (19); http://www.omim.org/ (III) PharmGkb (20) (https://www.pharmgkb.org/); (IV) TTD (21) (http://db.idrblab.net/ttd/; and (V) DrugBank (22) (https://www.drugbank.ca/). We used the term “acute renal damage” and the species name “Homo sapiens” to search the five databases. A Venn diagram was created using the associated genes of AKI.
Prediction of potential targets of AR-RAS in the treatment of AKI
Using the R programming language (The R Foundation of Statistical Computing) to match the annotated chemical targets to the summarized AKI illness targets, we identified the AR-RAS targets and the AKI targets that intersected on the Venn diagram, and these intersectional targets were deemed promising AR-RAS therapeutic targets in AKI.
Construction and analysis of a protein-protein interaction network
Using Cytoscape 3.8.0, the network diagram was created by selecting the points of intersection between AR-RAS and AKI. The common potential therapeutic targets in the Venn diagram were input into The Search Tool for the Retrieval of Interacting Genes/Proteins (STRING) database (23), which is capable of predicting interactions between proteins. Then, to determine the interaction connection between the objectives, the species name “Homo sapiens” was used. The TSV format file, which was downloaded from the STRING database, was imported into the Cytoscape 3.8.0 for analysis. This created a more complex protein interaction network diagram, where the node color and size were determined by the degree value, while the combined score determined the edge thickness.
Gene Ontology (GO) function and Kyoto Encyclopedia of Genes and Genomes (KEGG) pathway enrichment
GO is a global standard framework for categorizing gene activities. GO databases are classified into three types: cellular component (CC), molecular function (MF), and biological process (BP) (24). The KEGG is a collection of artificially created route maps that depict molecular interactions and reaction networks. In this process, P values of <0.01 were used with the GO keywords.
Molecular docking
Molecular docking, considered to be the key technology of structure-based drug discovery (25), facilitates the analysis of the binding site, molecular conformation, and macromolecular target affinity. First, the compound’s 2-dimensional (2D) structure diagrams were collected from the PubChem database (https://pubchem.ncbi.nlm.nih.gov) and input into Chem3D software for constructing 3-dimensional (3D) structural diagrams of energy optimization, which were subsequently saved to mol2 format. Core target protein PDB data were acquired from the Protein Data Bank (PDB; http://www.rcsb.org/pdb/home/home.do) and entered into the PyMOL program to extract data on heteromolecules and water molecules. In order to manage the data set of the aforementioned protein receptors and ligands, AutoDock Tools 1.5.6 software was used, as it can routinely save data into a PDBQT file and scan the active pockets. AutoDock Vina was used for molecular docking and bioinformatics research. PyMOL software-based visualization analysis revealed the optimum affinity configuration. The study was conducted in accordance with the Declaration of Helsinki (as revised in 2013).
Results
Active AR-RAS component screening
Review of TCMSP and the relevant literature yielded 87 AR components and 125 RAS components. A final number of 19 AR-RAS compounds with OB ≥30% and DL ≥0.18 were ultimately selected (Table 1).
Table 1
Herb name | Mol ID | Molecule name | OB (%) | DL |
---|---|---|---|---|
Astragali Radix | MOL000211 | Mairin | 55.3770733787 | 0.7761 |
MOL000239 | Jaranol | 50.8288167701 | 0.29148 | |
MOL000296 | Hederagenin | 36.9139058327 | 0.75072 | |
MOL000033 | (3S,8S,9S,10R,13R,14S,17R)-10,13-dimethyl-17-((2R,5S)-5-propan-2-yloctan-2-yl)-2,3,4,7,8,9,11,12,14,15,16,17-dodecahydro-1H-cyclopenta(a)phenanthren-3-ol | 36.2284705584 | 0.78288 | |
MOL000354 | Isorhamnetin | 49.604377053 | 0.306 | |
MOL000371 | 3,9-di-O-methylnissolin | 53.7415267252 | 0.47573 | |
MOL000378 | 7-O-methylisomucronulatol | 74.6861375238 | 0.29792 | |
MOL000379 | 9,10-dimethoxypterocarpan-3-O-β-D-glucoside | 36.736688011 | 0.9243 | |
MOL000380 | (6aR,11aR)-9,10-dimethoxy-6a,11a-Dihydro-6H-benzofurano(3,2-c)chromen-3-ol | 64.2554545232 | 0.42486 | |
MOL000387 | Bifendate | 31.0978239059 | 0.66553 | |
MOL000392 | Formononetin | 69.6738806088 | 0.21202 | |
MOL000417 | Calycosin | 47.7518278266 | 0.24278 | |
MOL000422 | Kaempferol | 41.8822495352 | 0.24066 | |
MOL000433 | FA | 68.9604362164 | 0.7057 | |
MOL000439 | Isomucronulatol-7,2'-di-O-glucosiole | 49.281055391 | 0.62065 | |
MOL000442 | 1,7-Dihydroxy-3,9-dimethoxy pterocarpene | 39.0454111203 | 0.47943 | |
MOL000098 | Quercetin | 46.4333481195 | 0.27525 | |
Radix Angelica Sinensis | MOL000358 | Beta-sitosterol | 36.9139058327 | 0.75123 |
MOL000449 | Stigmasterol | 43.8298515785 | 0.75665 |
AR-RAS, Astragali Radix-Radix Angelica Sinensis; OB, bioavailability; DL, drug-likeness.
A total of 7,197 AKI disease targets were gathered from the disease-related databases [see table online (available at https://cdn.amegroups.cn/static/public/tau-23-562-1.pdf)], including OMIM and GeneCards (see Figure 1). Through the comparison of AR-RAS with the targets linked to AKI, a final number of 101 genes were identified as possible AR-RAS targets in AKI treatment [see Figure 2; see table online (available at https://cdn.amegroups.cn/static/public/tau-23-562-2.pdf)].
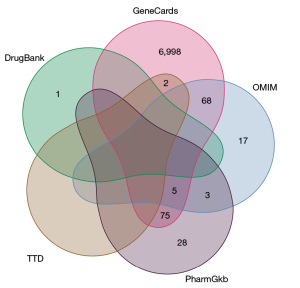
Construction of the active component-target network and protein-protein interaction network
The 193 node and 430 edge-containing regulatory networks of “herb-active ingredient-target” were created in Cytoscape 3.8.0 (see Figure 3). The 17 purple circular nodes indicate AR’s active ingredients, whereas the two red circular nodes represent RAS’s active ingredients. The target genes are represented by the 174 blue square nodes.
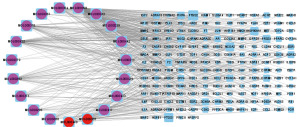
In order to investigate the interplay of core aims, we used the STRING database. The 101 AR-RAS and AKI common targets were used to construct protein interaction diagrams with STRING and Cytoscape 3.8.0, and the protein-protein interaction findings were produced. The network diagram, as illustrated in Figure 4, has 87 nodes and 195 interactive lines. In terms of degree value, the top 5 nodes in the network were AKT serine/threonine kinase 1 (AKT1) (17), nuclear receptor coactivator 1 (NCOA1) (14), JUN (13), estrogen receptor alpha (ESR1) (13), and mitogen-activated protein kinase 8 (MAPK8) (12). These nodes are the potential primary targets of AR-RAS; the bigger the node’s degree value is, the larger the target’s role in the network (see Figure 4).
GO function and KEGG pathway enrichment analysis
AR-RAS targets were imported into The Database for Annotation, Visualization and Integrated Discovery (DAVID) for GO analysis and KEGG pathway analysis for further investigation. A threshold (P<0.05) was used to filter the findings. The GO functional enrichment analysis produced 1,642 GO terms (P<0.05), which included 1,418 BP terms, 74 CC terms, and 150 MF terms. Figure 5 depicts the top 10 most substantially enriched GO terms. In regard to blood pressure, the targets were mostly connected to the cellular response to medication, the vascular process in the circulatory system, xenobiotic stimulus, antibiotics, steroid hormones, and lipopolysaccharides. For CC, the majority of the targets were involved with the integral component of the presynaptic membrane, along with the membrane’s microdomains, regions, plasma membrane rafts, and other rafts. Meanwhile, the most common MF targets were adrenergic receptor activity, nuclear receptor activity, G protein-coupled amine receptor activity, direct ligand regulated sequence-specific DNA binding steroid hormone receptor activity, and transcription factor activity (see Figure 5).
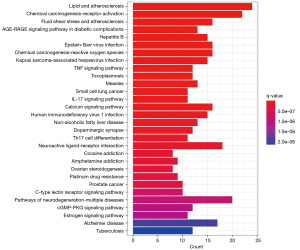
KEGG pathway enrichment yielded a total of 159 enriched pathways, the top 30 of which are displayed in Figure 6. The name of the route is shown on the Y-axis, while the fraction of genes is shown on the X-axis. The size of the bubble indicates the number of genes concentrated in the changed route in the bubble diagram, and the color of the point represents the enrichment’s importance. The enriched pathways mainly included the interleukin-17 (IL-17) signaling pathway, tumor necrosis factor (TNF) signaling pathway, T helper 17 (Th17) cell differentiation, calcium signaling pathway, chemical carcinogenesis-receptor activation, and lipid and atherosclerosis (see Figure 6).
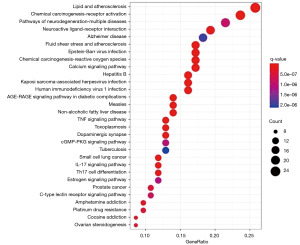
Molecular docking verification
In the protein-protein interaction analysis, the greater the degree value a node has, the greater its importance in the whole network. AKT1 and NCOA1 had higher degree values in the protein-protein interaction network and were thus considered the main protein receptors in this investigation.
In general, affinity <−7.00 kcal/mol indicates adequate binding strength, affinity <−5.00 kcal/mol indicates good binding strength, whilst affinity <−4.25 kcal/mol indicates the likelihood of ligand-receptor interaction, respectively (24-26).
Stigmasterol and beta-sitosterol showed the highest binding affinity for AKT1, with affinities of −11.1 and −10.1 kcal/mol, respectively. Kaempferol and quercetin showed the highest binding for NCOA1, with affinities of −8.2 kcal/mol. These findings suggest that the key active components of AR-RAS have a high affinity for the primary targets (see Figure 7 and Table 2).
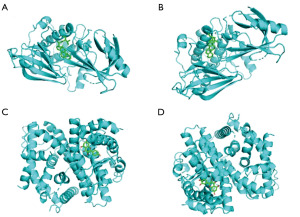
Table 2
Molecule name | Target name | |
---|---|---|
AKT1 (5KCV) | NCOA1 (4TV1) | |
Jaranol | −9.2 | −6.9 |
Hederagenin | −8.8 | −7.2 |
Isorhamnetin | −9.5 | −7.5 |
3,9-di-O-methylnissolin | −9.3 | −6.8 |
7-O-methylisomucronulatol | −8.7 | −6.7 |
Formononetin | −9.7 | −7.5 |
Calycosin | −9.9 | −7 |
Kaempferol | −9.5 | −8.2 |
Quercetin | −9.8 | −8.2 |
Beta-sitosterol | −10.1 | −7.2 |
Stigmasterol | −11.1 | −7.4 |
Discussion
TCM contains numerous components, multiple targets outcomes with a synergistic impact, and has long been used to treat a variety of illnesses. Network pharmacology, as an essential pharmacological research approach, can better illustrate the connection between components, drugs and targets. TCM network pharmacology offers a fresh approach for discovering active herbal components for certain illnesses or pathological processes. In addition, molecular docking is based on network pharmacological prediction and analysis. High-precision docking simulation and molecular pathway diagrams enable to show the selectivity of ligands, clarify the relationship between ligands and molecular networks, and evaluate the binding potential of protein ligands (27).
The approach of network pharmacology was used in this study for clarifying the pharmacological mechanism of AR-RAS in AKI treatment. TCMSP was examined for 19 active compounds with OB ≥30% and DL ≥0.18. There were 101 possible therapeutic targets found, and 6 main targets. Additionally, 1,642 enriched GO functional items and 160 KEGG pathways relevant to AKI illness were identified, showing that AR-RAS contained multiple components, multiple targets, and multiple pathways in treatment of AKI. Moreover, 19 components of AR-RAS were identified that had potential effects on AKI, including, among others, quercetin, kaempferol, 7-O-methylisomucronulatol, formononetin, and isorhamnetin.
Quercetin, a natural flavonoid present in many fruits and vegetables, exhibits a wide range of pharmacological properties such as anticancer effects (e.g., antioxidant, anti-inflammatory and antisenescence effects) along with antiviral activity (28-30). Quercetin can be used for treating AKI by inhibiting ferroptosis of renal tubular epithelial cells (31). Kaempferol has anticancer, anti-inflammatory, antioxidant, antibacterial, and antiviral properties. It can be used in the cure of different diseases, such as diabetes and osteoporosis (32-35). An interesting study has recently found that kaempferol can attenuate calcium oxalate crystal-induced renal injury through repressing oxidative stress and inflammation in the kidney (36). Formononetin is a natural and bioactive isoflavone isolated from herbal medicines (37), displaying various potential pharmacological and biological properties, such as anti-inflammatory (38), antioxidative (39), and anti-apoptotic effects (40). One study found that formononetin can protect cisplatin-induced AKI rats and cells by inhibiting oxidative stress, apoptosis, and inflammatory pathways (41). Isorhamnetin, a flavonoid, has potent anti-inflammatory (42), antioxidant (43,44), and ameliorative effects on diabetes-induced renal damage (45).
AR’s multicomponent and RAS’s and multitarget properties were represented in a component-target network. The protein-protein interaction network showed an interaction between the targets of AR-RAS. AKT1 (17), NCOA1 (14), JUN (13), ESR1 (13), and MAPK8 (12) were the five nodes with the highest degree value. We speculated that these potential core target genes might play a key role in the development of AKI. The protein-protein network, which shows protein-protein interaction, was created to investigate essential targets in AR-RAS for AKI, indicating that MAPK8, ESR1, JUN, NCOA1, AKT1, particularly JUN NCOA1, and AKT1 might be the core targets in AKI.
Akt/protein kinase B (PKB) is a serine/threonine kinase that can be involved in tubular apoptosis and inflammatory response during renal ischemia-reperfusion injury (46,47). The transcription factor hypoxia-inducible factor-1α (HIF-1α) (48) has been associated to glomerulosclerosis and renal interstitial fibrosis (49). NCOAs include coactivators and corepressors and significantly boost nuclear receptor transcriptional activity. A study has found that NCOA1, NCOA2, and NCOA3 are associated with HIF-1α in chronic kidney disease (50). The gene proto-oncogene JUN (c-Jun) is essential to the inflammatory response. When exposed to external stimuli, cellular c-Jun expression increases substantially in cells, enabling their ready adaptation to changes in their environment (51-54). These findings complement our previous findings regarding the significance of AKT1, NCOA1, and JUN in AR-RAS for AKI.
Through GO function enrichment and KEGG pathway analysis, we observed that the AR-RAS targets in AKI treatment mostly concentrated on the Th17 cell differentiation IL-17 signaling pathway, TNF signaling pathway and calcium signaling pathway.
The calcium signaling pathway is involved in nearly every physiological function of the normal cell cycle, including cell growth, proliferation, and differentiation (55). As calcium signaling is important for AKI, this process could be manipulated therapeutically to improve AKI (56). Moreover, IL-17 can promote renal tissue injury in crescentic glomerulonephritis (57), and IL-17 secretion participates in the pathogenesis of AKI-induced fibrosis, possibly via the recruitment of neutrophils (58). In addition, IL-17 signaling pathway is involved in the innate immune inflammation associated with kidney ischemia-reperfusion injury (59). Th17 cells were shown to be important mediators of renal injury associated with autoimmune-mediated nephritis induced via an experiment which injected sheep serum into mice (60). Th17 cells can induce an overall inflammatory response that leads to the recruitment of effectors cells or that precipitates vascular congestion and hypoxia, resulting in renal injury (61). Tissue fibrosis, AKI-to-chronic kidney disease, and ischemia-reperfusion-induced AKI are exacerbated by a strong Th17 inflammatory response (62). Taken together, the above results showed that the core components and key targets of AR-RAS play an important role in treatment of AKI.
The most frequently used method for assessing component-target interactions is molecular docking. From our docking results, it was found that the AKT1-stigmasterol, AKT1-beta-sitosterol, AKT1-calycosin, NCOA1-kaempferol, NCOA1-quercetin, and NCOA1-stigmasterol pairs had the lower binding energies. From these molecular docking findings, we clarified the effect of AR-RAS in the treatment of AKI. The molecular docking and network pharmacology results were consistent with each other, demonstrating network pharmacology’s dependability in target prediction.
Finally, this study used the network pharmacology approach to investigate the connection among multicomponents, multitargets, the regulation of multiple signal pathways, and AKI in AR-RAS. Our results can aid in clarifying the active component-target-disease interaction mechanism and informing further research into the treatment of AKI with TCM. However, as this study mainly used data analysis, we have only provided a theoretical reference for revealing the pharmacological mechanism, and thus further experiments in vitro and in vivo with these compounds would be needed to verify our findings.
Conclusions
In this study, network pharmacology identified 19 main active compounds in AR-RAS and 101 genes targeted by AKI. Through integrating network pharmacology, five core targets (AKT1, NCOA1, JUN, ESR1, and MAPK8) from complex networks were predicted to be the key targets for AR-RAS in AKI treatment. These results clarify the potential effective mechanism of AR-RAS in the treatment of AKI at multiple targets and pathways, and provide a basis for understanding the molecular mechanism of AR-RAS in the treatment of AKI.
Acknowledgments
Funding: None.
Footnote
Reporting Checklist: The authors have completed the STREGA reporting checklist. Available at https://tau.amegroups.com/article/view/10.21037/tau-23-562/rc
Peer Review File: Available at https://tau.amegroups.com/article/view/10.21037/tau-23-562/prf
Conflicts of Interest: All authors have completed the ICMJE uniform disclosure form (available at https://tau.amegroups.com/article/view/10.21037/tau-23-562/coif). The authors have no conflicts of interest to declare.
Ethical Statement: The authors are accountable for all aspects of the work in ensuring that questions related to the accuracy or integrity of any part of the work are appropriately investigated and resolved. The study was conducted in accordance with the Declaration of Helsinki (as revised in 2013).
Open Access Statement: This is an Open Access article distributed in accordance with the Creative Commons Attribution-NonCommercial-NoDerivs 4.0 International License (CC BY-NC-ND 4.0), which permits the non-commercial replication and distribution of the article with the strict proviso that no changes or edits are made and the original work is properly cited (including links to both the formal publication through the relevant DOI and the license). See: https://creativecommons.org/licenses/by-nc-nd/4.0/.
References
- Newman-Rivera AM, Kurzhagen JT, Rabb H. TCRαβ+ CD4-/CD8- "double negative" T cells in health and disease-implications for the kidney. Kidney Int 2022;102:25-37. [Crossref] [PubMed]
- Susantitaphong P, Cruz DN, Cerda J, et al. World incidence of AKI: a meta-analysis. Clin J Am Soc Nephrol 2013;8:1482-93. [Crossref] [PubMed]
- Guo S, Chen Y, Huo Y, et al. Comparison of early and delayed strategy for renal replacement therapy initiation for severe acute kidney injury with heart failure: a retrospective comparative cohort study. Transl Androl Urol 2023;12:715-26. [Crossref] [PubMed]
- Kellum JA, Romagnani P, Ashuntantang G, et al. Acute kidney injury. Nat Rev Dis Primers 2021;7:52. [Crossref] [PubMed]
- Zhang Q, Qi J, Luo Q, et al. Yishen Xiezhuo formula ameliorates the development of cisplatin-induced acute kidney injury by attenuating renal tubular epithelial cell senescence. Ann Transl Med 2022;10:1392. [Crossref] [PubMed]
- Chen J, Wu W, Yu Y, et al. Protective effect of Astragalus membranaceus on renal ischemia-reperfusion injury. Southeast NationaL Defense Medicine 2000;21:10-1.
- Chen XP, Li W, Xiao XF, et al. Phytochemical and pharmacological studies on Radix Angelica sinensis. Chin J Nat Med 2013;11:577-87. [Crossref] [PubMed]
- Meng L, Qu L, Tang J, et al. A combination of Chinese herbs, Astragalus membranaceus var. mongholicus and Angelica sinensis, enhanced nitric oxide production in obstructed rat kidney. Vascul Pharmacol 2007;47:174-83. [Crossref] [PubMed]
- Cai Q, Li X, Wang H. Protective and accelerated repair effects of Astragalus and Angelica Mixture on acute ischemia/reperfusion renal injury in rats. Chinese Medical Journal 2001;114:119-23. [PubMed]
- Bai Y. Discussion on the Application of Classic Prescriptions in Acute Renal Injury. Chinese Association of Chinese Medicine, Chinese Association of Chinese Medicine; 2014.
- Chen W. Observation of therapeutic effect of Huangqi injection on acute kidney injury caused by mannitol. Journal of Hainan Medical University 2011;17:1054-6.
- Giansanti P, Strating JRPM, Defourny KAY, et al. Dynamic remodelling of the human host cell proteome and phosphoproteome upon enterovirus infection. Nat Commun 2020;11:4332. [Crossref] [PubMed]
- Zhang R, Zhu X, Bai H, et al. Network Pharmacology Databases for Traditional Chinese Medicine: Review and Assessment. Front Pharmacol 2019;10:123. [Crossref] [PubMed]
- Zhang RZ, Yu SJ, Bai H, et al. TCM-Mesh: The database and analytical system for network pharmacology analysis for TCM preparations. Sci Rep 2017;7:2821. [Crossref] [PubMed]
- Chen L, Cao Y, Zhang H, et al. Network pharmacology-based strategy for predicting active ingredients and potential targets of Yangxinshi tablet for treating heart failure. J Ethnopharmacol 2018;219:359-68. [Crossref] [PubMed]
- Ru J, Li P, Wang J, et al. TCMSP: a database of systems pharmacology for drug discovery from herbal medicines. J Cheminform 2014;6:13. [Crossref] [PubMed]
- Xu XX, Bi JP, Ping L, et al. A network pharmacology approach to determine the synergetic mechanisms of herb couple for treating rheumatic arthritis. Drug Des Devel Ther 2018;12:967-79. [Crossref] [PubMed]
- Stelzer G, Rosen N, Plaschkes I, et al. The GeneCards Suite: From Gene Data Mining to Disease Genome Sequence Analyses. Curr Protoc Bioinformatics 2016;54:1.30.1-1.30.33.
- Amberger JS, Bocchini CA, Schiettecatte F, et al. OMIM.org: Online Mendelian Inheritance in Man (OMIM®), an online catalog of human genes and genetic disorders. Nucleic Acids Res 2015;43:D789-98. [Crossref] [PubMed]
- Whirl-Carrillo M, McDonagh EM, Hebert JM, et al. Pharmacogenomics knowledge for personalized medicine. Clin Pharmacol Ther 2012;92:414-7. [Crossref] [PubMed]
- Wang Y, Zhang S, Li F, et al. Therapeutic target database 2020: enriched resource for facilitating research and early development of targeted therapeutics. Nucleic Acids Res 2020;48:D1031-41. [PubMed]
- Wishart DS, Feunang YD, Guo AC, et al. DrugBank 5.0: a major update to the DrugBank database for 2018. Nucleic Acids Res 2018;46:D1074-82. [Crossref] [PubMed]
- Szklarczyk D, Franceschini A, Wyder S, et al. STRING v10: protein-protein interaction networks, integrated over the tree of life. Nucleic Acids Res 2015;43:D447-52. [Crossref] [PubMed]
- Ashburner M, Ball CA, Blake JA, et al. Gene ontology: tool for the unification of biology. The Gene Ontology Consortium. Nat Genet 2000;25:25-9. [Crossref] [PubMed]
- Torres PHM, Sodero ACR, Jofily P, et al. Key Topics in Molecular Docking for Drug Design. Int J Mol Sci 2019;20:4574. [Crossref] [PubMed]
- Gaillard T. Evaluation of AutoDock and AutoDock Vina on the CASF-2013 Benchmark. J Chem Inf Model 2018;58:1697-706. [Crossref] [PubMed]
- Hsin KY, Matsuoka Y, Asai Y, et al. systemsDock: a web server for network pharmacology-based prediction and analysis. Nucleic Acids Res 2016;44:W507-13. [Crossref] [PubMed]
- Patel RV, Mistry BM, Shinde SK, et al. Therapeutic potential of quercetin as a cardiovascular agent. Eur J Med Chem 2018;155:889-904. [Crossref] [PubMed]
- Yi H, Peng H, Wu X, et al. The Therapeutic Effects and Mechanisms of Quercetin on Metabolic Diseases: Pharmacological Data and Clinical Evidence. Oxid Med Cell Longev 2021;2021:6678662. [Crossref] [PubMed]
- Pathak S, Regmi S, Nguyen TT, et al. Polymeric microsphere-facilitated site-specific delivery of quercetin prevents senescence of pancreatic islets in vivo and improves transplantation outcomes in mouse model of diabetes. Acta Biomater 2018;75:287-99. [Crossref] [PubMed]
- Wang Y, Quan F, Cao Q, et al. Quercetin alleviates acute kidney injury by inhibiting ferroptosis. J Adv Res 2020;28:231-43. [Crossref] [PubMed]
- Wong G, Lu J, Zhang W, et al. Pseudorabies virus: a neglected zoonotic pathogen in humans? Emerg Microbes Infect 2019;8:150-4. [Crossref] [PubMed]
- Chen M, Xiao J, El-Seedi HR, et al. Kaempferol and atherosclerosis: From mechanism to medicine. Crit Rev Food Sci Nutr 2022; Epub ahead of print. [Crossref] [PubMed]
- Lei X, Guo J, Wang Y, et al. Inhibition of endometrial carcinoma by Kaempferol is interceded through apoptosis induction, G2/M phase cell cycle arrest, suppression of cell invasion and upregulation of m-TOR/PI3K signalling pathway. J BUON 2019;24:1555-61. [PubMed]
- Sharma N, Biswas S, Al-Dayan N, et al. Antioxidant Role of Kaempferol in Prevention of Hepatocellular Carcinoma. Antioxidants (Basel) 2021;10:1419. [Crossref] [PubMed]
- Yuan P, Sun X, Liu X, et al. Kaempferol alleviates calcium oxalate crystal-induced renal injury and crystal deposition via regulation of the AR/NOX2 signaling pathway. Phytomedicine 2021;86:153555. [Crossref] [PubMed]
- Zhang YZ, Zhang J, Tan L, et al. Preparation and evaluation of temperature and magnetic dual-responsive molecularly imprinted polymers for the specific enrichment of formononetin. J Sep Sci 2018;41:3060-8. [Crossref] [PubMed]
- Hwang JS, Kang ES, Han SG, et al. Formononetin inhibits lipopolysaccharide-induced release of high mobility group box 1 by upregulating SIRT1 in a PPARδ-dependent manner. PeerJ 2018;6:e4208. [Crossref] [PubMed]
- Mu H, Bai YH, Wang ST, et al. Research on antioxidant effects and estrogenic effect of formononetin from Trifolium pratense (red clover). Phytomedicine 2009;16:314-9. [Crossref] [PubMed]
- Nguyen LTH, Nguyen UT, Kim YH, et al. Astragali Radix and its compound formononetin ameliorate diesel particulate matter-induced skin barrier disruption by regulation of keratinocyte proliferation and apoptosis. J Ethnopharmacol 2019;228:132-41. [Crossref] [PubMed]
- Hao Y, Miao J, Liu W, et al. Formononetin protects against cisplatin-induced acute kidney injury through activation of the PPARα/Nrf2/HO-1/NQO1 pathway. Int J Mol Med 2021;47:511-22. [Crossref] [PubMed]
- Kim SY, Jin CY, Kim CH, et al. Isorhamnetin alleviates lipopolysaccharide-induced inflammatory responses in BV2 microglia by inactivating NF-κB, blocking the TLR4 pathway and reducing ROS generation. Int J Mol Med 2019;43:682-92. [PubMed]
- Choi YH. The cytoprotective effect of isorhamnetin against oxidative stress is mediated by the upregulation of the Nrf2-dependent HO-1 expression in C2C12 myoblasts through scavenging reactive oxygen species and ERK inactivation. Gen Physiol Biophys 2016;35:145-54. [Crossref] [PubMed]
- Tian X, Peng X, Lin J, et al. Isorhamnetin Ameliorates Aspergillus fumigatus Keratitis by Reducing Fungal Load, Inhibiting Pattern-Recognition Receptors and Inflammatory Cytokines. Invest Ophthalmol Vis Sci 2021;62:38. [Crossref] [PubMed]
- Kalai FZ, Boulaaba M, Ferdousi F, et al. Effects of Isorhamnetin on Diabetes and Its Associated Complications: A Review of In Vitro and In Vivo Studies and a Post Hoc Transcriptome Analysis of Involved Molecular Pathways. Int J Mol Sci 2022;23:704. [Crossref] [PubMed]
- Lan A, Du J. Potential role of Akt signaling in chronic kidney disease. Nephrol Dial Transplant 2015;30:385-94. [Crossref] [PubMed]
- Kim IY, Park YK, Song SH, et al. Role of Akt1 in renal fibrosis and tubular dedifferentiation during the progression of acute kidney injury to chronic kidney disease. Korean J Intern Med 2021;36:962-74. [Crossref] [PubMed]
- Zhu L, Mu J, Wu Y, et al. Role of HIF-1α in Cold Ischemia Injury of Rat Donor Heart Via the miR-21/PDCD4 Pathway. Transplant Proc 2020;52:383-91. [Crossref] [PubMed]
- Liu G, He L. Salidroside Attenuates Adriamycin-Induced Focal Segmental Glomerulosclerosis by Inhibiting the Hypoxia-Inducible Factor-1α Expression Through Phosphatidylinositol 3-Kinase/Protein Kinase B Pathway. Nephron 2019;142:243-52. [Crossref] [PubMed]
- Zhou T, Lin W, Lin S, et al. Association of Nuclear Receptor Coactivators with Hypoxia-Inducible Factor-1α in the Serum of Patients with Chronic Kidney Disease. Biomed Res Int 2020;2020:1587915. [Crossref] [PubMed]
- Jessen KR, Mirsky R. The Role of c-Jun and Autocrine Signaling Loops in the Control of Repair Schwann Cells and Regeneration. Front Cell Neurosci 2022;15:820216. [Crossref] [PubMed]
- Lamph WW, Wamsley P, Sassone-Corsi P, et al. Induction of proto-oncogene JUN/AP-1 by serum and TPA. Nature 1988;334:629-31. [Crossref] [PubMed]
- Duncan MC. New directions for the clathrin adaptor AP-1 in cell biology and human disease. Curr Opin Cell Biol 2022;76:102079. [Crossref] [PubMed]
- Casalino L, Talotta F, Cimmino A, et al. The Fra-1/AP-1 Oncoprotein: From the "Undruggable" Transcription Factor to Therapeutic Targeting. Cancers (Basel) 2022;14:1480. [Crossref] [PubMed]
- Kim DH, Bang E, Ha S, et al. Organ-differential Roles of Akt/FoxOs Axis as a Key Metabolic Modulator during Aging. Aging Dis 2021;12:1713-28. [Crossref] [PubMed]
- Wasnik S, Tang X, Bi H, et al. IGF-1 Deficiency Rescue and Intracellular Calcium Blockade Improves Survival and Corresponding Mechanisms in a Mouse Model of Acute Kidney Injury. Int J Mol Sci 2020;21:4095. [Crossref] [PubMed]
- Krohn S, Nies JF, Kapffer S, et al. IL-17C/IL-17 Receptor E Signaling in CD4(+) T Cells Promotes T(H)17 Cell-Driven Glomerular Inflammation. J Am Soc Nephrol 2018;29:1210-22. [Crossref] [PubMed]
- Mehrotra P, Collett JA, McKinney SD, et al. IL-17 mediates neutrophil infiltration and renal fibrosis following recovery from ischemia reperfusion: compensatory role of natural killer cells in athymic rats. Am J Physiol Renal Physiol 2017;312:F385-97. [Crossref] [PubMed]
- Cortvrindt C, Speeckaert R, Moerman A, et al. The role of interleukin-17A in the pathogenesis of kidney diseases. Pathology 2017;49:247-58. [Crossref] [PubMed]
- Paust HJ, Turner JE, Steinmetz OM, et al. The IL-23/Th17 axis contributes to renal injury in experimental glomerulonephritis. J Am Soc Nephrol 2009;20:969-79. [Crossref] [PubMed]
- Basile DP, Ullah MM, Collet JA, et al. T helper 17 cells in the pathophysiology of acute and chronic kidney disease. Kidney Res Clin Pract 2021;40:12-28. [Crossref] [PubMed]
- Noel S. Orai1: CRACing the Th17 response in AKI. J Clin Invest 2019;129:4583-6. [Crossref] [PubMed]
(English Language Editor: J. Gray)