Reliable prognostic definition and immunotherapy response prediction in bladder cancer, based on a novel aging-associated 5-gene signature model
Highlight box
Key findings
• Our findings indicate that there is a strong correlation between aging-related genes (ARGs) and the progression of bladder cancer (BC), which means that this predictive model could provide a new reference for the clinical diagnosis and treatment of BC.
What is known and what is new?
• ARGs play an important role in the occurrence and progression of other tumors.
• Association of ARGs are associated with prognosis and immunotherapy in patients with BC.
What is the implication, and what should change now?
• The function of ARGs in BC was comprehensively evaluated in terms of prognosis, immune microenvironment, tumor mutational load, and immunotherapy in BC patients.
Introduction
Bladder cancer (BC), with approximately 549,000 new cases worldwide each year (1), is one of the most prevalent genitourinary cancers for which the main identified cause is currently tobacco smoking (2). According to whether the muscular layer of the urinary bladder wall is invaded by tumor, BC can be categorized into non-muscle invasive BC (NMIBC) and muscle invasive BC (MIBC); the former accounts for the vast majority (approximately 75%). The treatment procedure for NMIBC, principally and initially, is transurethral resection of bladder tumor (TURBT). MIBC is routinely treated by radical surgery and urinary diversion with or without combined adjuvant therapy (3). Although the 5-year survival rate after initial resection has improved to 90%, the current issue for NMIBC is the lack of effective strategies to address its high recurrence rate (4,5). In the case of MIBC, the rapid metastatic progression of the tumor and the high mortality rate are the main problems (6). With the rise of immunotherapy, the prognosis for BC patients has been improved dramatically (7). However, only a few patients benefit from immunotherapy, and it faces challenges such as immune resistance and lack of response to immunotherapy (8).
Cellular senescence can be induced by different stimuli, both in vivo and in vitro (9). The most prominent feature of senescence is progressive loss of function or degeneration at different levels, from the molecular and cellular levels to tissue and organismal levels. In general, senescence is associated with the development of several degenerative pathologies, and it also promotes the progression of proliferative diseases, especially cancer (10). Based on the available data, it is hypothesized that a close relationship between senescence and carcinogenesis may exist. Generally speaking, the metabolic proliferation of cells will slow down when they enter the senescent state. At the same time, immune cells are attracted, with the purpose of killing senescent cells, thus inhibiting the progression of tumors (11-13). However, surprisingly, as cells enter senescence for an extended duration, they not only provide a tissue microenvironment for the development and growth of tumors (10), but also have the ability to shift the tissue phenotype from epithelial to mesenchymal, thus enhancing tumor invasion and distant metastasis (14). Furthermore, the examination of related cytokines is performed to protect senescent cells that have been harmed by DNA radiation or chemotherapeutic agents, aiming to shield these tumor cells from radiotherapy and consequently inducing drug resistance (15,16). In summary, cellular senescence may act as a cancer suppressor early in life, but, over time, it may disrupt normal tissue structure and function and drive the progression of associated degenerative and proliferative degenerative disease as senescent cells accumulate later in life (10). Therefore, further understanding of the mechanisms of aging-related genes (ARGs) in tumors will help us to find new therapeutic strategies and select the most effective treatments upfront.
In this study, we integrated 33 ARGs that have been characterized in previous studies and developed a 5-gene model. The data were derived from The Cancer Genome Atlas (TCGA) database. The function of ARGs in BC was comprehensively evaluated in terms of prognosis, immune microenvironment, tumor mutational burden (TMB), and immunotherapy in BC patients. We present this article in accordance with the TRIPOD reporting checklist (available at https://tau.amegroups.com/article/view/10.21037/tau-23-422/rc).
Methods
Data collection and processing
We obtained the transcriptome data of 428 bladder samples which included 19 normal samples and 409 BC samples. The tumor samples were collected from TCGA. Fragments per kilobase per million mapped fragments (FPKM) values were first normalized with transcripts per million (TPM) method and then transformed using log2. Excluding patients without overall survival (OS) information, we obtained a total of 398 BC samples for the follow-up study. The limma package (17) (normalize between arrays) function was used to eliminate possible batch effects between different sequencing batches of BC samples. The study was conducted in accordance with the Declaration of Helsinki (as revised in 2013).
The occurrence rate of copy number variation (CNV) in ARGs
We obtained CNV information for BC from the University of California, Santa Cruz (UCSC) Xena database (https://xena.ucsc.edu/). We then calculated the variant frequencies of 33 ARGs and visualized them using lollipop plots. With the Rcircos package, we mapped the chromosomal location of these ARGs.
Construction and evaluation of prognostic models for ARGs
By univariate Cox regression analysis, we identified ARGs that exhibited a significant association with prognosis, with TCGA samples used as the cohort, which were then considered for subsequent analysis (P<0.05). Based on the screened ARGs, we utilized the “glmnet” R package (18). To construct statistical prognostic features, least absolute shrinkage and selection operator (LASSO) Cox regression analyses were performed. The risk score model was calculated by multiplying the respective coefficients with their corresponding variables: risk score = MAPK1 × 0.00979931139016805 + MAPK3 × 0.341316763794631 + CDK6 × 0.127860972192279 + ID1 × (−0.0418185591809889) + UBC × 0.0131539686643873. We then partitioned the TCGA cohort into the training and validation sets using a 1:1 ratio through random assignment. We divided BC patients into high- and low-risk groups in the training and validation sets on the basis of median risk score. For the purpose of evaluating the feasibility of the model, we calculated Kaplan-Meier (KM) curves with the help of the “survival” and “survminer” R packages to compare high- and low-risk groups’ survival rates. Next, the predictive performance of risk scores was assessed on the survivorship at 1-, 3-, and 5-year intervals by creating time-dependent receiver operating characteristic (ROC) curves through the utilization of “survival” and “timeROC” R packages. The “ggpubr” package was used to draw box plots to compare differences in risk scores with other clinicopathological parameters, including survival status, tumor-node-metastasis (TNM) stage, T stage, and N stage. With the “pheatmap” package, we plotted a heat map of gene-clinical feature correlation to construct a risk score model.
Construction and evaluation of column line diagrams
To assess whether the risk score has an independent effect for the prognosis of BC patients, univariate and multifactorial Cox regression analyses were applied on clinical information, which included age, gender, TNM stage, and risk score in the TCGA cohort, and hazard ratios (HRs) and P values were calculated for each variable. Based on the appeal results, column line plots were constructed for predicting the survival of BC patients at 1, 3, and 5 years. Calibration curves and ROC curves were used to evaluate the stability and reliability of the column line graphs.
Differential gene identification and enrichment analysis
Using the “limma” package, we performed a difference-in-difference analysis for high- and low-risk groups. Differentially expressed genes (DEGs) with adjusted P values <0.05 and absolute fold changes (FCs) >1 were considered significant, which were then used for further analysis. Based on the obtained DEGs, Gene Ontology (GO) and Kyoto Encyclopedia of Genes and Genomes (KEGG) enrichment analyses were conducted using the R package “clusterProfiler” (19).
Correlation analysis between risk score and tumor immune microenvironment
The Estimation of Stromal and Immune Cells in Malignant Tumors using Expression data (ESTIMATE) algorithm (20) was applied, and we then calculated the ESTIMATE score, immune score, stromal score, and tumor purity in the TCGA cohort to assess the correlation between risk scores and the tumor microenvironment (TME). Subsequently, we determined the measurements of 29 common immune cells and immune functions present in each BC sample by single-sample gene set enrichment analysis (ssGSEA) algorithm, which were then compared between the high- and low-risk groups and visualized the results of ssGSEA analysis with box plots. Afterwards, on the basis of the results of scoring common immune cells by seven types of scoring software, we proceeded with correlations between risk scores and immune cells using Spearman correlation analysis. It was considered statistically significant if P<0.05 for the Wilcoxon test.
Correlation analysis of risk scores with tumor mutation burden and immunotherapy
The TCGA database was used to download single nucleotide variant (SNV) data of BC patients and TMB was then calculated. The correlation between risk scores and TMB was studied with the help of Spearman correlation analysis. The study used KM curves to assess disparities in TMB and prognostic outcomes between high- and low-risk groups. In addition, the “maftools” R package (21) was applied to analyze the number of non-synonymous point mutations in the somatic cells of each sample. We compared risk scores and common immune checkpoints by Wilcoxon rank sum test and correlated the two by Spearman test. Afterwards, we obtained an immunotherapy cohort with the help of the Gene Expression Omnibus (GEO) database: the Metastatic Uroepithelial Tumor Cohort (IMvigor210: http://research-pub.gene.com/IMvigor210CoreBiologies/) (22). Using the R package “EdgeR”, raw data were filtered and normalized. In a Wilcoxon rank sum test, differences in risk scores were compared between the response and non-response groups, and survival analyses revealed the prognosis after receiving immunotherapy. We then acquired data from The Cancer Immunome Atlas (TCIA) database (https://tcia.at/) (23). Data of BC patients who underwent immunotherapy were downloaded, and Wilcoxon rank sum test was performed to compare the difference between high- and low-risk groups in receiving immunotherapy (P<0.05 was considered statistically significant).
Statistical analysis
Wilcoxon test was employed to compare differences between the two groups. KM analysis was performed using the “surv” package. Spearman test was applied for the purpose of correlation analysis and correlation coefficient calculations. R software (version 4.2.0; The R Foundation for Statistical Computing, Vienna, Austria) was applied for all statistical analyses, with statistical significance defined as a P value less than 0.05.
Results
Genetic variation and differential expression of ARGs in BC
We collected 33 ARGs for studying their role in BC and their developmental mechanisms based on the reported literature. First, we summarized the somatic mutation profiles of 33 ARGs, which were mutated in 255 out of 411 BC samples with a mutation frequency of (62.04%), and we found that the mutation frequency for TP53 was 48%, followed by RB1 (18%), and CDKN2A (6%) (Figure 1A). Our next step was to analyze the CNV frequency. We observed that CNV alterations were prevalent in ARGs and mostly focused on copy number amplification, whereas almost half of the ARGs had a higher deletion frequency (Figure 1B). We further showed the mosomal location of ARGs (as revealed in Figure 1C). Subsequently, we further explored whether the messenger RNA (mRNA) expression of senescence-associated genes in normal versus tumor tissues was correlated with the CNV frequency. The results showed that ARGs with significant amplification or deletion were significantly different in normal and tumor tissues. For example, ARGs which exhibited the highest amplification frequency (E2F3, TFDP1, etc.) and the highest deletion frequency (CDKN2A, etc.) showed considerably higher expression in tumor tissues in comparison with normal tissues (Figure 1D). This implies that significant CNV alterations have a corresponding impact on the transcriptional process of ARGs. Subsequently, we further obtained the interaction relationships of 33 ARGs from the Search Tool for the Retrieval of Interaction Genes/Proteins (STRING) database. As revealed in Figure 1E, CDKN2A, CDK4, and AGO1 had more interactions with other genes, suggesting their potential significance in the development of BC. In summary, we found significant heterogeneity in the genetic variation and expression profiles of ARGs in BC, which may have a notable influence on the development of BC.
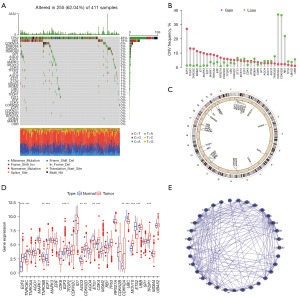
Construction of a 5-gene model of aging-related risk
In order to evaluate the function of aging-associated genes in BC, we first performed univariate Cox regression analysis for screening prognosis-related ARGs. As shown in Figure 2A, we found that MAPK1, MAPK3, CDK6, ID1, CDK4, UBC, and TFDP1 had a dramatic link with prognosis (P<0.05). The HR indicated that MAPK1, MAPK3, CDK6, CDK4, UBC, and TFDP1 were risk factors (HR >1), whereas ID1 was a protective factor (HR <1). To prevent the risk of overfitting, we further screened the above seven genes using LASSO Cox regression analysis. Ultimately, five core ARGs were identified to build a prognostic risk score model, which was calculated as follows: risk score = MAPK1 × 0.00979931139016805 + MAPK3 × 0.341316763794631 + CDK6 × 0.127860972192279 + ID1 × (−0.0418185591809889) + UBC × 0.0131539686643873 (Figure 2B,2C). Subsequently, we excluded 11 tumor samples without complete survival information from the TCGA cohort, yielding 398 tumor samples for subsequent analysis. We randomly grouped the TCGA cohort in a 1:1 ratio to obtain the training and validation sets, respectively, with the training and validation sets each comprising 199 samples.
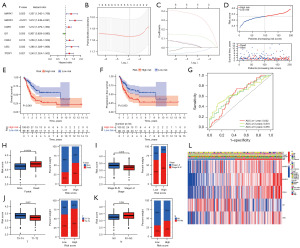
The training set was divided into two categories, high-risk (n=100) and low-risk (n=99), on the basis of the median value of the risk score and the distribution and survival status of the population in the high- and low-risk groups are shown in Figure 2D. As revealed, the population belonging to the high-risk group exhibited a higher mortality rate. By KM survival analysis, patients in the high-risk group had poorer OS and progression-free survival (PFS) compared to those in the low-risk group (P<0.05) (Figure 2E,2F). After that, we assessed the reliability and stability of the risk score in predicting 1-, 3-, and 5-year survival utilizing time-dependent ROC curves, where the area under the curve (AUC) was 0.642, 0.670, and 0.655 at 1, 3, and 5 years, respectively (Figure 2G).
Subsequently, to further assess the utility and validity of the risk prognosis model in predicting clinicopathological parameters, a clinical correlation study was conducted. As shown in Figure 2H-2K, we found that the high-risk group had a higher mortality rate and the majority of it was in the middle-to-late stages of tumor progression, which is consistent with a worse prognosis for the high-risk group. We found that MAPK1, MAPK3, CDK6, and UBC were more highly expressed in the high-risk group, whereas ID1 was more highly expressed in the low-risk group, Besides, the high-risk group had significant differences in TNM stage and clinicopathological T-stage compared with the low-risk group population with most BC patients in the mid-to-late stage (Figure 2L). Therefore, we gleaned that the prognostic risk score provided a superior diagnostic and predictive ability for BC, potentially serving as a novel avenue for BC treatment.
Internal cohort validation of the reliability of the risk scoring model
We conducted the follow-up analysis using the validation set from the TCGA cohort to assess the reliability and stability of the risk score model, and each sample was given a risk score by the same formula. According to the median value of the risk scores, we grouped the validation set into a high-risk group (n=100) population and a low-risk group (n=99) population. As with the training set, the high-risk group population had a worse survival advantage (Figure 3A). As shown by the time-dependent ROC curve analysis, the risk score model had AUC values of 0.666, 0.626, and 0.650 in predicting 1-, 3-, and 5-year survival rates in BC patients, respectively (Figure 3B). In addition, patients with high-risk scores in the validation set still had higher mortality rates (Figure 3C). Based on this, we continued to evaluate the association between the risk score model and clinical characteristics. We obtained similar conclusions as in the training set in Figure 3D-3G. High-risk patients had higher mortality rates and were mostly in the middle to late stage of the tumor. The clinical correlation heat map also demonstrated that in the high-risk group, MAPK1, MAPK3, CDK6, and UBC had higher expression, whereas ID1 had higher expression in the low-risk group (Figure 3H). As revealed above, the risk scoring model exhibited not only better stability but also better reliability. As a result, it is possible to become a novel approach and strategy in the realm of BC detection, diagnosis, and treatment.
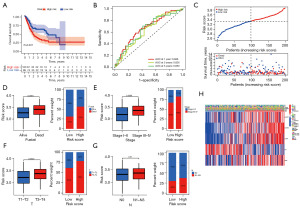
Construction and evaluation of column line diagrams
To explore the link between risk score and prognosis as well as clinical characteristics, we performed univariate and multifactorial Cox regression analyses, which revealed that age, TNM stage, and risk score function independently in prognosis of BC (Figure 4A,4B). To provide a quantitative tool for the clinic, we utilized age, TNM stage, and risk score as components of the column line graph on the basis of the findings of both univariate and multifactorial Cox regression analysis (Figure 4C). Subsequently, we further assessed the stability and reliability of the column line graphs using calibration curves and ROC curves. The calibration curve indicated that the predicted values were in high agreement with the actual observed values (Figure 4D). Additionally, the ROC curve demonstrated a higher predictive efficacy with the AUC of 0.730, 0.732, and 0.761 for estimating the OS of BC patients at 1-, 3-, and 5-year intervals, respectively (Figure 4E). As is revealed above, column line graphs incorporating clinical characteristics have more reliable and more accurate predictive ability, and could possibly be used as a new assessment method for BC in the clinical setting.
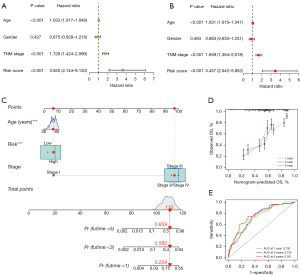
Identification of differential genes in high- and low-risk groups and enrichment analysis
To delve deeper into the molecular mechanisms, a differential analysis was performed in the high- and low-risk groups. By false discovery rate (FDR) <0.05 and |log2FC| >1, we obtained a total of 2,060 genes that were significantly different in the two groups. Next, we conducted enrichment analyses of differential genes using GO and KEGG enrichment analyses (Figure 5). GO enrichment analysis revealed that DEGs were primarily enriched in cell growth and development, as well as cellular chemotaxis, including extracellular matrix (ECM), ossification, chondrogenesis, connective tissue development, collagen fibers, granulocyte chemotaxis, leukocyte migration, and cell-substrate adhesion. KEGG enrichment analysis revealed that myocarditis or cardiomyopathy, inflammation, and tumor metastasis were significantly enriched pathways. These pathways encompassed conditions such as myocarditis, dilated cardiomyopathy, hypertrophic cardiomyopathy, arrhythmogenic right ventricular cardiomyopathy, rheumatoid arthritis, Staphylococcus aureus infection, chemokine signaling pathways, cell adhesion molecules, ECM-receptor interactions, cytokine-cytokine receptor interactions, PI3K-Akt signaling pathway, and so on. In summary, we found that ARGs have a key impact on the development of BC, in addition to being closely linked to growth and development.
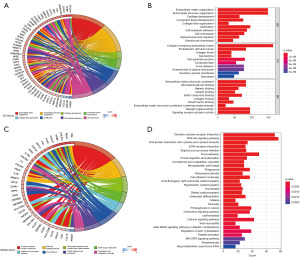
Correlation analysis of risk scores and immune microenvironment
According to recent studies, it has been found that the TME has a crucial impact on tumorigenesis and progression (24,25). As a result, our initial step was to perform a thorough analysis of both risk scores and the TME. In this case, we scored the patients in the TCGA cohort to obtain their immune score, stromal score, ESTIMATE score, and tumor purity by the ESTIMATE algorithm. Figure 6A-6D shows that patients classified in the high-risk group had higher immune scores, mechanism scores, and ESTIMATE scores, and correspondingly, they obtained lower tumor purity. Subsequently, we further studied the link between immune cell infiltration and risk scores. We scored 29 common immune cells and functions by ssGSEA algorithm, the vast majority of which were significantly enriched in the high-risk group. It included the immune cells such as dendritic cells, CD8+ T cells, B cells, macrophages, neutrophils, tumor-infiltrating lymphocytes, and helper T cells, and correspondingly immune functions such as antigen-presenting functions, cellular chemokines, immune checkpoints, pro-inflammatory responses, and class interferon responses (Figure 6E,6F). The heat map also provided a visual demonstration that most immune functions were remarkably enhanced in the high-risk group (Figure 6G). Besides, seven different software were applied to assess immune cell infiltration, the result of which indicated that the majority of immune cells have a positive correlation with risk scores (Figure 6H). Taken together, compared to the low-risk group, multiple assessments suggested that patients in the high-risk group had a greater abundance of immune infiltrates, which implies that they may reap better therapeutic benefits in terms of antitumor therapy and immunotherapy.
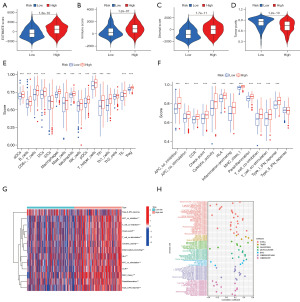
Correlation analysis of risk scores with TBM and immunotherapy
It has been shown that TMB is associated with infiltrating CD8+ T cells, which produce intense tumor killing upon anti-programmed cell death ligand 1 (PD-L1) treatment (22). At the same time, patients with high TMB produce better outcomes after anti-PD-L1 treatment (26). Next, we endeavored to find out the link between risk score and TMB, taking all these findings into account. Initially, we conducted an analysis on the distribution of gene mutations within both the high- and low-risk groups and the top 20 genes with the highest mutation frequencies were demonstrated by waterfall plot. In the low-risk group, TTN (42%) was the most commonly mutated gene, whereas TP53 (58%) was the most frequently mutated gene in the high-risk group (Figure 7A,7B). Subsequently, we can conclude that patients with high tumor mutations tended to have better survival rates by survival analysis (P<0.001, Figure 7C). Also, stratified survival analysis suggested that patients with high TMB had a better prognosis, whereas those with a low TMB and in the high-risk group had the worst prognosis (P<0.001, Figure 7D).
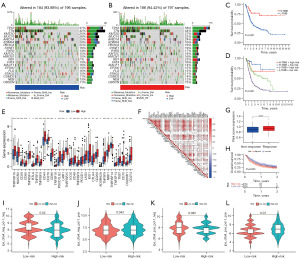
Subsequently, we analyzed the common immune checkpoints. As is revealed in Figure 7E, the high-risk group showed high levels of large log immune checkpoints. Correlation analysis verified that the risk score is in direct proportion to the majority of immune checkpoints (Figure 7F). This implies that individuals in the high-risk group were inclined to have better curative effect and greater benefit from receiving immunotherapy. In addition, we evaluated patients in the IMvigor210 cohort after immunotherapy and found out that there was a better response to treatment among patients of high-risk group (Figure 7G). Further survival analysis revealed that compared to the low-risk group, patients who are in the high-risk group had worse OS (P=0.035, Figure 7H). This may be because most of the patients in the high-risk group were intermediate-to-advanced stage patients, whereas most of the low-risk patients were early-stage patients, and although the former had better immunotherapy results, they still did not achieve satisfactory treatment outcomes. Subsequently, we explored the link between risk scores and immunotherapy by performing International Prognostic Scores (IPS) on BC patients. Compared to the low-risk group, we can conclude that patients in the high-risk group had better efficacy when treated with PD-1 only (P=0.043); there was no significant difference when treated with CTLA4 only (P=0.085); and better benefit was produced when treated with the combination of PD-1 and CTLA4 (P=0.01, Figure 7I-7L). In conclusion, individuals in the high-risk group showed a better response to immunotherapy.
Discussion
As growing evidence shows cellular senescence is a prominent factor in the inflammation, immunity, and tumorigenesis (27). In contrast, current studies on cellular senescence are limited to finite senescence genes, neglecting the exploration of the interplay of multiple senescence genes and their impacts on BC. Hence, the development of a new senescence scoring model can enhance our comprehension of the mechanism of ARGs in BC and the response to immune response, and offer innovative approaches and orientations for treating BC in clinical practice.
Here, a novel 5-gene risk score model on the basis of 33 ARGs by one-way Cox regression analysis and LASSO Cox regression analysis was built in order to further explore the relationship between the risk score model and prognosis in BC patients. The TCGA cohort was grouped into a training cohort and a validation cohort at a ratio of 1:1. Then, a risk score was computed for each sample by analyzing the expression levels of five ARGs (MAPK1, MAPK3, CDK6, ID1, UBC). According to the median value of the risk score, the samples in the training set and validation sets were classified into high- and low-risk groups. We discovered that patients belonging to the high-risk group tended to have a more unfavorable prognosis for survival. Afterwards, the link between the risk score model and clinical characteristics was explored in more detail. The high-risk group had a higher mortality rate and those included were mostly in the middle and late stages of tumor progression. This is consistent with the fact that they had a worse prognosis. Subsequently, we further performed univariate and multifactorial Cox regression analyses based on clinical characteristics and risk scores, and risk scores were shown to be independent predictors, according to the outcomes.
Subsequently, the results obtained from the risk score and multifactorial Cox analysis were combined to construct an integrated column line graph model, which showed better gains than clinical characteristics in predicting 1-, 3-, and 5-year OS in BC patients. This further validates the reliability of the model we constructed in predicting the prognosis of BC, thus offering a fresh avenue for diagnosing and treating BC in the clinical field.
Furthermore, we investigated the differences in biological functions between the high- and low-risk groups with the help of enrichment analysis. GO and KEGG enrichment analysis were performed on the basis of the obtained DEGs, which indicated that DEGs were predominantly enriched in cell growth and development, self-healing, and chemotaxis. Additionally, KEGG enrichment analysis showed that inflammation, infection, and metastasis of tumors were the entries of significant enrichment. The current study found that the presence of senescent cells is a significant factor in embryonic development and wound healing (28,29). Besides, the current study also shows that inflammation is a common marker of tissue aging and that inflammation leads to decreased tissue repair and production, which leads to a variety of diseases (30-32). In contrast, the role of aging for tumors appears contradictory. In general, cellular metabolism and proliferation are progressively weakened or even stalled after aging, which inhibits tumor growth and development, but studies over the past decade have shown that under certain conditions, persistently senescent cells can acquire pro-tumorigenic properties and thus promoting tumor development (33-35). Therefore, further dissection of the function of ARGs in tumor development may provide a new vision and entry point for tumor treatment.
Afterwards, we conducted a more in-depth analysis to examine the correlation between tumor immune microenvironment and risk scores. We observed higher immune scores, mechanism scores, and estimation scores in the high-risk group and correspondingly, lower tumor purity. The immune infiltration analysis revealed a significant enrichment of most immune cells or functions in the high-risk group, including dendritic cells, CD8+ T cells, B cells, macrophages, neutrophils, tumor-infiltrating lymphocytes, helper T cells, antigen-presenting functions, cellular chemokines, immune checkpoints, and pro-inflammatory responses. Available studies have shown that the immunostimulatory receptor CD40 is significantly highly expressed on dendritic cells in the BC TME and exerts a better immunotherapeutic effect by significantly reducing CD8+ T cell depletion through administration of CD40 agonist receptors (36). Meanwhile, it was shown that CD40 agonists induced antitumor immune responses with IFN-γ (37), IL-12 (38), and T cells (37) also playing an important role. Simultaneously, it was also discovered that BC cells attract a larger population of CD4+ T cells compared to normal bladder cells, which potentially heightens the risk of invasion (37). Based on these findings, we speculate that significant immune cell and function enrichment may produce better immunotherapeutic outcomes, and it is also suggested that immune infiltration may have a non-negligible impact on the development of BC.
TMB has now been shown to be significantly associated with immunotherapy, and patients with high TMB have displayed greater benefit in receiving immunotherapy (39). As a result, we delved deeper into the potential mechanisms of risk scores and TMB. As described above, patients who had high tumor mutations had better survival (P<0.001). By stratified survival analysis, it is obvious that patients in the high TMB and low-risk group had a superior prognosis. On the contrary, patients in the low TMB and high-risk group had the worst prognosis (P<0.001). In addition, the waterfall plot also demonstrates the top 20 genes with the highest mutation frequency. In the low-risk group, TTN (42%) had the highest mutation frequency, whereas in the high-risk group, TP53 (58%) had the highest mutation frequency. Among them, TP53 is one of the most frequently reported prognostic risk factors for cancer survival and has been widely recognized to have a link with cancer development (40). In contrast, TTN has also been shown to have a pro-cancer effect and is related to poor prognosis in many cancers (41). The rise of immunotherapy in recent years has also significantly altered the prognosis of BC patients, attaining good results in treating those with PD-1/PD-L1 inhibitors (42). Our study also revealed that the high-risk group had significantly elevated expression levels of most immune checkpoints, suggesting a favorable treatment outcome for patients belonging to this group. Analysis of individuals in the IMvigor210 cohort showed that those who received immunotherapy also responded better to treatment. Nevertheless, the survival rate of patients in the high-risk group after immunotherapy was lower than that of the low-risk group, probably because most high-risk patients had intermediate-to-advanced tumors.
In contrast, analysis of the IPS scores of BC patients revealed that treatment with PD-1 and the combination of PD-1 and CTLA4 improved outcomes for individuals in the high-risk group. Therefore, immune checkpoint inhibitor treatment provides a new treatment method for BC patients (43), but it is still only applicable to a small proportion of patients, and further reduction of immunotherapy resistance and lack of response to immunotherapy are still new directions that urgently need to be studied and explored.
Conclusions
Our findings indicate that there is a strong correlation between ARGs and the progression of BC, which means that this predictive model could provide a new reference for the clinical diagnosis and treatment of BC.
Acknowledgments
Funding: This article was funded by
Footnote
Reporting Checklist: The authors have completed the TRIPOD reporting checklist. Available at https://tau.amegroups.com/article/view/10.21037/tau-23-422/rc
Peer Review File: Available at https://tau.amegroups.com/article/view/10.21037/tau-23-422/prf
Conflicts of Interest: All authors have completed the ICMJE uniform disclosure form (available at https://tau.amegroups.com/article/view/10.21037/tau-23-422/rc). The authors have no conflicts of interest to declare.
Ethical Statement: The authors are accountable for all aspects of the work in ensuring that questions related to the accuracy or integrity of any part of the work are appropriately investigated and resolved. The study was conducted in accordance with the Declaration of Helsinki (as revised in 2013).
Open Access Statement: This is an Open Access article distributed in accordance with the Creative Commons Attribution-NonCommercial-NoDerivs 4.0 International License (CC BY-NC-ND 4.0), which permits the non-commercial replication and distribution of the article with the strict proviso that no changes or edits are made and the original work is properly cited (including links to both the formal publication through the relevant DOI and the license). See: https://creativecommons.org/licenses/by-nc-nd/4.0/.
References
- Bray F, Ferlay J, Soerjomataram I, et al. Global cancer statistics 2018: GLOBOCAN estimates of incidence and mortality worldwide for 36 cancers in 185 countries. CA Cancer J Clin 2018;68:394-424. [Crossref] [PubMed]
- Patel VG, Oh WK, Galsky MD. Treatment of muscle-invasive and advanced bladder cancer in 2020. CA Cancer J Clin 2020;70:404-23. [Crossref] [PubMed]
- Xu Y, Luo C, Wang J, et al. Application of nanotechnology in the diagnosis and treatment of bladder cancer. J Nanobiotechnology 2021;19:393. [Crossref] [PubMed]
- Chamie K, Litwin MS, Bassett JC, et al. Recurrence of high-risk bladder cancer: a population-based analysis. Cancer 2013;119:3219-27. [Crossref] [PubMed]
- Sylvester RJ, van der Meijden AP, Oosterlinck W, et al. Predicting recurrence and progression in individual patients with stage Ta T1 bladder cancer using EORTC risk tables: a combined analysis of 2596 patients from seven EORTC trials. Eur Urol 2006;49:466-5; discussion 475-7. [Crossref] [PubMed]
- Shinagare AB, Ramaiya NH, Jagannathan JP, et al. Metastatic pattern of bladder cancer: correlation with the characteristics of the primary tumor. AJR Am J Roentgenol 2011;196:117-22. [Crossref] [PubMed]
- Bellmunt J, de Wit R, Vaughn DJ, et al. Pembrolizumab as Second-Line Therapy for Advanced Urothelial Carcinoma. N Engl J Med 2017;376:1015-26. [Crossref] [PubMed]
- Crispen PL, Kusmartsev S. Mechanisms of immune evasion in bladder cancer. Cancer Immunol Immunother 2020;69:3-14. [Crossref] [PubMed]
- Sun JX, Liu CQ, Xu JZ, et al. A Four-Cell-Senescence-Regulator-Gene Prognostic Index Verified by Genome-Wide CRISPR Can Depict the Tumor Microenvironment and Guide Clinical Treatment of Bladder Cancer. Front Immunol 2022;13:908068. [Crossref] [PubMed]
- Campisi J. Aging, cellular senescence, and cancer. Annu Rev Physiol 2013;75:685-705. [Crossref] [PubMed]
- Kang TW, Yevsa T, Woller N, et al. Senescence surveillance of pre-malignant hepatocytes limits liver cancer development. Nature 2011;479:547-51. [Crossref] [PubMed]
- Xue W, Zender L, Miething C, et al. Senescence and tumour clearance is triggered by p53 restoration in murine liver carcinomas. Nature 2007;445:656-60. [Crossref] [PubMed]
- Chien Y, Scuoppo C, Wang X, et al. Control of the senescence-associated secretory phenotype by NF-κB promotes senescence and enhances chemosensitivity. Genes Dev 2011;25:2125-36. [Crossref] [PubMed]
- Laberge RM, Awad P, Campisi J, et al. Epithelial-mesenchymal transition induced by senescent fibroblasts. Cancer Microenviron 2012;5:39-44. [Crossref] [PubMed]
- Sun Y, Campisi J, Higano C, et al. Treatment-induced damage to the tumor microenvironment promotes prostate cancer therapy resistance through WNT16B. Nat Med 2012;18:1359-68. [Crossref] [PubMed]
- Gilbert LA, Hemann MT. DNA damage-mediated induction of a chemoresistant niche. Cell 2010;143:355-66. [Crossref] [PubMed]
- Ritchie ME, Phipson B, Wu D, et al. limma powers differential expression analyses for RNA-sequencing and microarray studies. Nucleic Acids Res 2015;43:e47. [Crossref] [PubMed]
- Engebretsen S, Bohlin J. Statistical predictions with glmnet. Clin Epigenetics 2019;11:123. [Crossref] [PubMed]
- Yu G, Wang LG, Han Y, et al. clusterProfiler: an R package for comparing biological themes among gene clusters. OMICS 2012;16:284-7. [Crossref] [PubMed]
- Yoshihara K, Shahmoradgoli M, Martínez E, et al. Inferring tumour purity and stromal and immune cell admixture from expression data. Nat Commun 2013;4:2612. [Crossref] [PubMed]
- Mayakonda A, Lin DC, Assenov Y, et al. Maftools: efficient and comprehensive analysis of somatic variants in cancer. Genome Res 2018;28:1747-56. [Crossref] [PubMed]
- Mariathasan S, Turley SJ, Nickles D, et al. TGFβ attenuates tumour response to PD-L1 blockade by contributing to exclusion of T cells. Nature 2018;554:544-8. [Crossref] [PubMed]
- Charoentong P, Finotello F, Angelova M, et al. Pan-cancer Immunogenomic Analyses Reveal Genotype-Immunophenotype Relationships and Predictors of Response to Checkpoint Blockade. Cell Rep 2017;18:248-62. [Crossref] [PubMed]
- Jing X, Yang F, Shao C, et al. Role of hypoxia in cancer therapy by regulating the tumor microenvironment. Mol Cancer 2019;18:157. [Crossref] [PubMed]
- Zeng D, Li M, Zhou R, et al. Tumor Microenvironment Characterization in Gastric Cancer Identifies Prognostic and Immunotherapeutically Relevant Gene Signatures. Cancer Immunol Res 2019;7:737-50. [Crossref] [PubMed]
- Rizvi NA, Hellmann MD, Snyder A, et al. Cancer immunology. Mutational landscape determines sensitivity to PD-1 blockade in non-small cell lung cancer. Science 2015;348:124-8. [Crossref] [PubMed]
- He S, Sharpless NE. Senescence in Health and Disease. Cell 2017;169:1000-11. [Crossref] [PubMed]
- Demaria M, Ohtani N, Youssef SA, et al. An essential role for senescent cells in optimal wound healing through secretion of PDGF-AA. Dev Cell 2014;31:722-33. [Crossref] [PubMed]
- Kim SH, Rowe J, Fujii H, et al. Upregulation of chicken p15INK4b at senescence and in the developing brain. J Cell Sci 2006;119:2435-43. [Crossref] [PubMed]
- Ferrucci L, Fabbri E. Inflammageing: chronic inflammation in ageing, cardiovascular disease, and frailty. Nat Rev Cardiol 2018;15:505-22. [Crossref] [PubMed]
- Mazzaferro S, De Martini N, Rotondi S, et al. Bone, inflammation and chronic kidney disease. Clin Chim Acta 2020;506:236-40. [Crossref] [PubMed]
- Neves J, Sousa-Victor P. Regulation of inflammation as an anti-aging intervention. FEBS J 2020;287:43-52. [Crossref] [PubMed]
- Huang W, Hickson LJ, Eirin A, et al. Cellular senescence: the good, the bad and the unknown. Nat Rev Nephrol 2022;18:611-27. [Crossref] [PubMed]
- Da Silva-Álvarez S, Collado M. The Jekyll and Hyde of Senescence in Cancer: TIMP1 Controls the Switch from Tumor-Controlling to Tumor-Promoting Senescence. Cancer Cell 2021;39:13-5. [Crossref] [PubMed]
- Schmitt CA, Wang B, Demaria M. Senescence and cancer - role and therapeutic opportunities. Nat Rev Clin Oncol 2022;19:619-36. [Crossref] [PubMed]
- Garris CS, Wong JL, Ravetch JV, et al. Dendritic cell targeting with Fc-enhanced CD40 antibody agonists induces durable antitumor immunity in humanized mouse models of bladder cancer. Sci Transl Med 2021;13:eabd1346. [Crossref] [PubMed]
- Byrne KT, Vonderheide RH. CD40 Stimulation Obviates Innate Sensors and Drives T Cell Immunity in Cancer. Cell Rep 2016;15:2719-32. [Crossref] [PubMed]
- Garris CS, Arlauckas SP, Kohler RH, et al. Successful Anti-PD-1 Cancer Immunotherapy Requires T Cell-Dendritic Cell Crosstalk Involving the Cytokines IFN-γ and IL-12. Immunity 2018;49:1148-1161.e7. [Crossref] [PubMed]
- Chan TA, Yarchoan M, Jaffee E, et al. Development of tumor mutation burden as an immunotherapy biomarker: utility for the oncology clinic. Ann Oncol 2019;30:44-56. [Crossref] [PubMed]
- Gammall J, Lai AG. Pan-cancer prognostic genetic mutations and clinicopathological factors associated with survival outcomes: a systematic review. NPJ Precis Oncol 2022;6:27. [Crossref] [PubMed]
- Zheng QX, Wang J, Gu XY, et al. TTN-AS1 as a potential diagnostic and prognostic biomarker for multiple cancers. Biomed Pharmacother 2021;135:111169. [Crossref] [PubMed]
- Ghasemzadeh A, Bivalacqua TJ, Hahn NM, et al. New Strategies in Bladder Cancer: A Second Coming for Immunotherapy. Clin Cancer Res 2016;22:793-801. [Crossref] [PubMed]
- Mancini M, Righetto M, Noessner E. Checkpoint Inhibition in Bladder Cancer: Clinical Expectations, Current Evidence, and Proposal of Future Strategies Based on a Tumor-Specific Immunobiological Approach. Cancers (Basel) 2021;13:6016. [Crossref] [PubMed]