Performance evaluation and clinical application of the UA-5600 automatic dry chemistry urine analyzer in renal diseases
Highlight box
Key findings
• Mindray UA-5600 and test strips provide excellent analytical performance and clinical performance.
What is known, and what is new?
• Urinalysis is a commonly used laboratory test. Urine contains a large number of human metabolites that provide information about the body’s current physiological state and disease manifestations.
• In this study, the analytical performance and clinical performance of the Mindray UA-5600 and test strips were evaluated. In addition, through the establishment of machine-learning (ML) models, an optimal model was selected to improve the diagnosis of renal disease.
What is the implication, and what should change now?
• The Naïve-Bayes model with good screening ability after training with eight ML models constructed from the 11 urine test results detected by the Mindray UA-5600. This also provides new ideas for urine dry chemistry test results that could assist in screening for kidney disease.
Introduction
Urinalysis is a commonly used laboratory test. Urine contains a large number of human metabolites that provide information about the body’s current physiological state and disease manifestations (1) and can be used to identify kidney disease, urinary tract infections (UTIs), and diabetes. It can also be used as a part of health screenings (2,3). Urine dry chemistry testing is an important part of urinalysis, is convenient to perform, and provides results from a number of urinary science and chemistry programs in a single test. Over the past two decades, more and more automated urine analyzers have been introduced (4), and the number of items that can be tested by urine dry chemical analyzers has also increased.
With the widespread use of urine flow cytometry to detect red blood cells (RBCs), white blood cells (WBCs), bacteria, and epithelial cells in human urine (5), urine dry chemistry detection is irreplaceable. Xie et al. (6) found that dry urine chemistry was even superior to the fully automated urine analyzer in terms of the area under the curve (AUC), sensitivity, and specificity for the detection of RBCs and WBCs in the diagnosis of UTIs. In addition to UTIs, urine chemistry analysis is also widely used in the auxiliary diagnosis of other diseases (1). When a large number of samples need to be analyzed or when an immediate urine analysis is required in emergency situations, semi-automatic or fully automatic urine dry chemistry analyzers can be used for standardized, high-throughput screening (7), and the results produced by the test can help to differentiate between samples without abnormalities and those outside the normal reference range. Positive samples may require further microscopic sediment analysis or microbiological examination. Urine dry chemistry testing, as a wide range of screening tools, can provide multiple test results in a single proposal. Accurate dry chemistry testing can better assist clinicians in diagnosis and decision-making, and can also reduce certain retesting rates and reduce the workload of examiners. The UA-5600 is a new urine dry chemistry analyser, and there have been no previous articles evaluating its performance aspects, so it is essential to verify the accuracy of its results.
In recent years, machine-learning (ML) models and artificial intelligence (AI), such as logistic regression (LR), Naïve Bayes (NB), K-nearest neighbor (KNN), support vector machines (SVMs), random forest (RF), multilayer perceptron (MLP), extreme gradient boosting (XGBOOST), and artificial neural networks (ANNs), have been increasingly applied in various branches of medicine (8), and have all been used in auxiliary diagnosis and prognosis prediction (9-11). Jang et al. constructed an XGBoost derivation model that can help to detect chronic renal disease (CKD) at an early stage and enables the timely referral of patients to nephrologists by providing two basic pieces of information about proteinuria and renal dysfunction (12). Some patients may start with only urine dry chemistry, which is an important screening tool and can provide multiple results, but clinics tend to focus on certain parameters, and constructing a ML model with 11 dry chemistry results can provide better assistance in screening for disease.
In summary, urine dry chemistry analysis has received attention in auxiliary clinical detection, and accurate urine dry chemistry detection is necessary in daily applications. This study sought to evaluate the analytical performance and clinical performance of the Mindray UA-5600 and the test strips. In addition, through the establishment of ML models, an optimal model was selected to improve the diagnosis of renal disease. We present this article in accordance with the STARD reporting checklist (available at https://tau.amegroups.com/article/view/10.21037/tau-24-189/rc).
Methods
Sample source
In this study, an in vitro diagnostic clinical assessment was performed using the remaining sample of the assessment tool report issued by The First Affiliated Hospital of Sun Yat-sen University without infringing on the privacy and interests of the patients. The data of 1,253 outpatient, inpatient, and physical examination patients from The First Affiliated Hospital of Sun Yat-sen University from August to September 2022 were randomly collected for methodological comparison. After unqualified samples, such as urine from patients under 18 years old and turbid urine, were excluded, 1,228 urine samples remained. The patients were aged 18–96 years old, and 449 were male and 779 were female. The samples were kept at room temperature of 18–25 ℃, and the test was completed within 2 hours of collection.
Instruments and reagents
The following instruments were used in this study: the UA-5600 automatic dry chemistry urine analyzer and URS-11MRQ urine test strips (Shenzhen Mindray Bio-Medical Electronics Co., Ltd., Shenzhen, China), Yihua Multi-Project Urine Chemical Analysis Controls, Quality control for dry chemical urine test strips and dry chemical urine analysers, (Shanghai Yihua Medical Science and Technology Co., Ltd., Shanghai, China), the EH-2090 urine formed element analyzer (Shenzhen Mindray Bio-Medical Electronics Co., Ltd., Shenzhen, China), which served as the control instrument for the urine formed element analysis, and the Mindray BS-2800M (Shenzhen Mindray Bio-Medical Electronics Co., Ltd., Shenzhen, China), which served as the control instrument for the protein (PRO) and glucose (GLU) biochemical analysis. All the instruments were calibrated and monitored daily to ensure quality control. All the reagents were used within expiration dates.
Basic performance verification
Precision
Every day, after the UA-5600 was turned and instrument performance was stable, negative and positive quality control tests were performed two times consecutively as the first batch of tests; the interval between the tests was at least 4 hours, or after 10 different samples were tested. Negative and positive quality control tests were performed two consecutive times as the second batch of tests on the same day, and the test lasted for at least 20 days. To calculate the inter-day precision, the low value of the negative quality control was assumed to be the target value. The complete consistency rate was calculated as follows: Complete consistency rate = the number of occurrences of target value results/the total number of tests (n=80) × 100%. The general consistency rate was calculated as follows: General consistency rate = (target value ± number of results within 1 magnitude)/total number of tests (n=80) Times New Roman 100%. The calculation methods for the positive quality control were the same as those for the negative quality control.
Carry over
Residual urine without preservatives was used as the testing material, and the highest concentration (+++/++++) of each item (except the pH, Specific gravity, and Nitrite) was tested once. Next, the negative samples or normal saline was immediately tested once to obtain a dry chemical test result (negative samples should not produce positive results).
Consistency with the comparative method
A total of 402 fresh urine samples were used to test the consistency of the BLD (blood) parameter results (inclusion range: RBC <200) obtained from the UA-5600 and the EH-2090, and 471 fresh urine samples were used to test the consistency of the LEU (leucocyte) parameter results (inclusion range: WBC <500) obtained from the UA-5600 and the EH-2090. The tests were performed once, and the consistency between the results for the corresponding parameters between the two machines was calculated.
A total of 298 fresh urine samples were used to test the consistency of the PRO (protein) parameter results (inclusion range: PRO <300 mg/dL), and a total of 202 fresh urine samples were used to test the consistency of the GLU (glucose) parameter results. The results for the corresponding parameters were consistent between the two methods.
Table S1 sets out the conversion relationship between the quantitative results and semi-quantitative results for each parameter.
Construction of various AI-based ML models
In this study, a total of 1,718 urine samples were collected and tested on the UA-5600 system, yielding 11 parameter test results. Patients were excluded from the study for the following reasons: they were aged <18 years (13 patients), and/or they had incomplete urine test results (14 patients). Of the above samples, 1,691 patients were included. Based on the hospital’s LIS system (Laboratory Information System) and the LIS diagnosis grouping, this cross-sectional survey retrospectively examined the charts of 346 patients (135 males and 211 females, age range: 18 to 98 years) diagnosed with renal disease, and 1,345 patients (397 males and 948 females, age range: 18 to 92 years) with non-renal disease diagnosed with other conditions (Figure 1). Hospital data spanning the period from August 2022 to October 2022 were obtained. Ethical approval for this study was obtained from the Medical Ethics Committee of The First Affiliated Hospital of Sun Yat-sen University (approval No. [2021]660). Individual consent for this retrospective analysis was waived. The study was conducted in accordance with the Declaration of Helsinki (as revised in 2013).
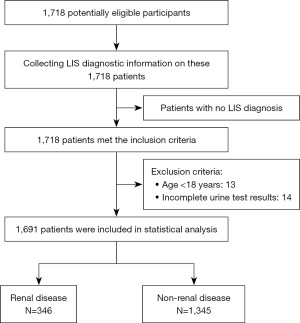
Data preprocessing
Python 3.10 (Python Software Foundation, Wilmington, DE, USA) were used to read the patient grouping and the UA-5600 detection results. The test results for the urine samples were pre-processed, which included data cleaning. Data cleaning involved reviewing and validating the data to delete redundant information, correct errors, and provide data consistency. Next, the complete data were randomly divided into a training set (70%) and a test set (30%) at a ratio of 7:3.
Construction of eight learning models
The following eight learning models were constructed:
- ANN (artificial neural network): an ANN simulates neuronal activity using a mathematical model based on an information processing system that imitates the structure and function of the brain’s neural network.
- LR (logistic regression): which is a special class of classification algorithm with “regression” in its name, is used to solve classification problems. Solutions are based on the concept of regression. The core idea of the algorithm is to find a line (surface) in space and classify the points based on the relative positions of the point to be located and the boundary line (interface). The sigmoid function is generally used as a step function to deal with the binary problem.
- GBDT (gradient boosting decision tree): a GBDT is an iterative decision-tree algorithm that constructs a set of weak learners (trees) and sums the results of multiple decision trees as the final predicted output. This algorithm effectively combines the decision tree concept and the ensemble approach.
- MLP (multiple layer perceptron): a MLP is a neural network that consists of a series of interconnected layers, with one end connected to the eigenvalue of the observed target and the other end connected to the corresponding target values, with a hidden layer in the middle. A MLP is essentially an expansion and deepening of a linear model.
- RF (random forest): a RF is composed of multiple decision trees, and the voting mechanism of multiple decision trees is used to improve the decision tree. A RF is a bagging algorithm with a decision tree as the estimator.
- SVM (support vector regression): a SVM classifies data by looking for a hyperplane that can maximize the classification gap in a training data set. When training samples are linearly inseparable, the kernel technique and the soft margin are maximized to map the training samples from the original space to a higher dimensional space so that the samples are linearly separable in this space.
- KNN (K-nearest neighbour): A KNN is a basic classification method that performs classification by measuring the distance between different features. If a majority of the k (proper noun) most similar samples in a feature space belong to a certain category, then the sample also belongs to this category.
- NB: NB is a classification algorithm based on the Bayes theorem. For a given item to be classified, the probability of an item occurring within each category under a certain condition is determined, and items with the largest probabilities are considered to occur in the same category.
In this study, using the 11-item output by the UA-5600, the following eight ML analysis models were constructed: LR, NB, KNN, SVM, RF, MLP, XGBoost, and ANN. The ML models were tested for their ability to screen renal disease samples from non-renal disease samples. The performance of different ML models was tested with the default thresholds and determined using the maximum AUC. The threshold and performance of different ML models when the precision and recall rate were balanced at the maximum (i.e., when the precision-recall curve was at the maximum) were examined to select the ML model with the best classification performance from different perspectives. The results were then output through the SHAP (Shapley Additive exPlanations) model.
Statistical analysis
Passing-Bablok regression analysis was performed on the comparison with the gold standard. The Passing-Bablok regression analysis used Analyse-it v 6.15 for statistical analysis. The box-plot analysis used GraphPad Prism 9.0.0(121) for statistical analysis.
Results
Precision
The general consistency and complete consistency of the test results for all the parameters of the negative control substance were 100%; the general consistency of the positive control substances, except for VC (Vitamin C) and KET (Ketone bodies), was greater than 90% (Tables S2,S3).
Carry over
The carry-over contamination rate results are set out in Table S4. The negative controls were tested immediately after the detection of the high value sample, and the negative controls all had no positive results, indicating that no carry-over contamination occurred.
Comparison with the control method
The box-plot results are shown in Figure 2. The semi-quantitative test results for BLD and LEU obtained using the UA-5600 and the quantitative test results for urine sediment obtained using the EH-2090 were well correlated (Figure 2A,2B). As the RBC and WBC content increased, the reflectance of the test strip decreased significantly (Figure 2E,2F).
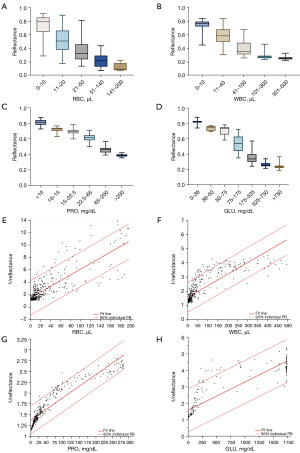
Similarly, the semi-quantitative test results for PRO and GLU obtained using the UA-5600 and the quantitative test results obtained using the BS-2800 M biochemical analyzer were well correlated (Figure 2C,2D). As the PRO and GLU content increased, the reflectance of the test strip decreased significantly (Figure 2G,2H).
Diagnostic performance
Tables 1,2 shows the distribution of false-negative and false-positive samples within the test results for each parameter assessed using the UA-5600 and using the reference method. Using the EH-2090 results as the reference standard, there were 23 false-negative samples and 48 false-positive samples for BLD, and 25 false-negative samples and 28 false-positive samples for LEU (Table 1). Using the BS-2800 M test results as the reference standard, there were 14 false-negative samples and 3 false-positive samples for PRO, and 6 false-negative samples and 1 false-positive sample for GLU (Table 2).
Table 1
Contingency table | EH-2090 results as the standard | Total | |
---|---|---|---|
Positive | Negative | ||
UA-5600 BLD | |||
Positive | 197 | 48 | 245 |
Negative | 23 | 134 | 157 |
Total | 220 | 182 | 402 |
UA-5600 LEU | |||
Positive | 254 | 28 | 282 |
Negative | 25 | 164 | 189 |
Total | 279 | 192 | 471 |
BLD, blood; LEU, leukocyte.
Table 2
Contingency table | BS-2800 results as the standard | Total | |
---|---|---|---|
Positive | Negative | ||
UA-5600 PRO | |||
Positive | 225 | 3 | 228 |
Negative | 14 | 56 | 70 |
Total | 239 | 59 | 298 |
UA-5600 GLU | |||
Positive | 119 | 1 | 120 |
Negative | 6 | 76 | 82 |
Total | 125 | 77 | 202 |
PRO, protein; GLU, glucose.
Using the EH-2090 results for RBCs and WBCs as the standard, the consistency rate for positive BLD detection obtained using the UA-5600 was 89.55%, and that for positive LEU detection was 91.04%. Using the BS-2800 results for PRO and GLU as the standard, the consistency rates of positive PRO and GLU detection by the UA-5600 were 94.14% and 95.20%, respectively (Table 3).
Table 3
Contingency table | Parameter | |||
---|---|---|---|---|
BLD | LEU | PRO | GLU | |
Positive consistency rate (%) | 89.55 | 91.04 | 94.14 | 95.20 |
Negative consistency rate (%) | 73.63 | 85.42 | 94.92 | 98.70 |
BLD, blood; LEU, leukocyte; PRO, protein; GLU, glucose.
ML model differentiation
The 11 parameters detected by the UA-5600 were used to construct 8 different ML models, and a validation set was then used to validate the ability of each model to differentiate between the presence and absence of renal diseases. The sensitivity and specificity results for each model are set out in Table 4. Receiver operating characteristic curves were drawn, and AUC values were calculated (Figure 3). Except for that for the KNN model, the AUCs of the other seven models were all greater than 0.9. Based on the sensitivity and specificity results, the NB model performed the best.
Table 4
Parameters | AUC | Sensitivity | Specificity |
---|---|---|---|
ANN | 0.929 | 0.5922 | 0.9407 |
LR | 0.959 | 0.7087 | 0.9753 |
NB | 0.947 | 0.7767 | 0.9457 |
KNN | 0.89 | 0.6408 | 0.9951 |
SVM | 0.966 | 0.7379 | 0.9802 |
RF | 0.958 | 0.6893 | 0.9852 |
MLP | 0.959 | 0.7087 | 0.9778 |
GBDT | 0.949 | 0.7184 | 0.9704 |
AUC, area under curve; ANN, artificial neural network; LR, logistic regression; NB, Naïve Bayes; KNN, K-nearest neighbour; SVM, support vector regression; RF, random forest; MLP, multiple layer perceptron; GBDT, gradient boosting decision tree.
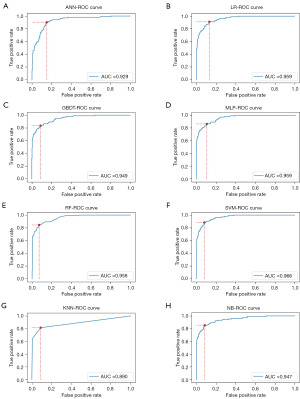
SHAP model analysis
The performance of the different ML models was compared, and the NB model performed the best in all aspects. Therefore, a confusion matrix was developed to further analyze the screening efficacy of the NB model using a validation set of 508 cases; among the results, there were 23 false-negative samples and 22 false-positive samples (Figure 4A). Next, a SHAP analysis was used to rank all the samples based on predicted feature importance. Each dot represented a sample, with red representing a high value of the feature and blue representing a low value of the feature; a positive SHAP value indicated an increased risk of renal disease (Figure 4B). As Figure 4 shows, PRO was the most important feature of the NB model constructed in this study.
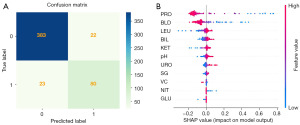
Discussion
Urinalysis includes physical, chemical, and sediment examinations. Generally, a series of urine dry chemistry analyzers and urine sediment analyzers form a urine analyzer assembly line. The urine formed element is generally considered the core of urinalysis; however, the formed element must be inspected manually by microscopy. Therefore, the detection speed is slow, the procedure is cumbersome, and different inspectors may report different results. Urine dry chemistry analysis can quickly provide semi-quantitative results for various urine components. However, as the test results are easily affected by various factors, dry chemistry test results are mostly used for screening. When positive for BLD and LEU, manual re-examination is required to rule out false-negative and false-positive results. In clinical practice, urine dry chemistry analyzers and urine sediment analyzers are used in combination for urinalysis to better meet the needs of clinical urological diagnosis and urological disease treatment. Therefore, urine dry chemistry analyzer results must be accurate. In this study, the clinical performance of Mindray’s new-generation automatic dry chemistry urine analyzer (the UA-5600) was evaluated to confirm whether the product can meet clinical application needs.
RBC, WBC, PRO, and GLU in urine are closely related to various diseases and have received clinical attention. Therefore, in this study, the four parameters BLD, LEU, PRO, and GLU were evaluated using the UA-5600. Urine RBCs are important for the diagnosis of immunoglobulin A (IgA) nephropathy, diabetes, systemic lupus erythematosus, and other diseases, and the monitoring of drug efficacy (13-16). The BLD test, which is part of the dry urine chemistry test, is an indicator used to screen for RBCs in the urine and can be performed regardless of the RBC morphology in the urine (i.e., regardless of whether it is intact or fragmented). In this study, the RBC detection ability of the UA-5600 within the semi-quantitative range of the test strip (RBC <200) was validated. The semi-quantitative test results obtained using the UA-5600 and the quantitative results obtained using the EH-2090 were well correlated (Figure 2A). As the RBC count increased, the reflectance of the test strip decreased significantly, and a downward trend was observed (Figure 2E).
Next, the consistency rate of the BLD parameter values obtained using the UA-5600 was validated against the RBC results obtained using the EH-2090 as the standard. Previous articles have verified that the RBC and WBC results of the EH-2090 correlate well with those of manual microscopy, so the EH-2090 was used in this study as the standard (17). The positive consistency rate between the two was 89.55%, and the negative consistency rate was 73.63% (Table 3). Using the EH-2090 detection result as the standard, there were 23 false-negative samples and 48 false-positive samples among the results obtained using the UA-5600. After the quantitative RBC results obtained using the EH-2090 were converted to semi-quantitative results (for a description of the methodology, see “Consistency with the comparative method”), the results of both false-negative and false-positive samples did not exceed the upper and lower gradients compared to the semi-quantitative results of the EH-2090 conversion and were within the clinically acceptable range.
The false-positives may have been caused by the fact that at present, the dry chemical detection of BLD mainly involves the detection of hemoglobin in RBCs, and dry chemical test strips generally have higher sensitivity for the detection of hemoglobin. For example, for samples of ruptured RBCs, the sediment test may be negative, but the BLD test may be positive; this may also be one of the causes of false-positive results. For false-negative samples, based on the EH-2090 test results, the RBC range in the samples was between 0 and 10, which is in the gray area. Because the threshold for RBC positivity is artificially defined, different thresholds can be set for different usage scenarios. The threshold can be determined based on the actual application to better assist clinical diagnosis.
WBCs in urine can be used as indicators of UTIs (18,19). There are no or very few leukocytes in the urine of normal people; when elevated in urine, leukocytes are mostly neutrophils. In urine dry chemistry, the presence of neutrophils is measured via the detection of granulocyte esterase, regardless of whether the neutrophils were crushed and lysed at the time of the test. In this study, the correlation between the results obtained using the UA-5600 semi-quantitative test [semi-quantitative range of the test strip (WBC <500)] and the EH-2090 quantitative test was good (Figure 2B), and as the WBC content in the urine sample increased, the reflectance of the test strip decreased significantly (Figure 2F).
Next, the consistency rate of the LEU parameter results obtained using the UA-5600 was validated using the WBC results obtained using the EH-2090 as the standard. The positive consistency rate between the two was 91.04%, and the negative consistency rate was 85.42% (Table 3). Using the EH-2090 test result as the standard, there were 25 false-negative samples and 28 false-positive samples among the results obtained using the UA-5600. After the quantitative WBC results obtained using the EH-2090 were converted to semi-quantitative results (for a description of the methodology, see “Consistency with the comparative method”), the results of both false-negative and false-positive samples did not exceed the upper and lower gradients compared to the semi-quantitative results of the EH-2090 conversion and were within the clinically acceptable range. Among the false-negative samples, the maximum WBC value obtained using the EH-2090 was 25.8. The results were all weakly positive after the conversion of the quantitative results to semi-quantitative results (Table S1) and did not cause clinical risk. The WBC detection range for the 28 false-positive samples was between 2.4 and 9.2, and the LEU test results were all weakly positive, and did not significantly interfere with the diagnoses. There was lymphocyte-positive urine among the true positive samples, and the semi-quantitative results obtained using the EH-2090 and UA-5600 differed by one gradient range. This difference was due to the dry chemistry detection method. Clinical attention should also be paid to this type of sample to avoid false-negative results.
In urine chemistry tests, PRO can be used as a very important screening indicator for renal diseases. Urine casts are closely related to PRO. Recent research has shown that proteinuria can also reflect the severity of renal function impairment caused by Coronavirus Disease 2019 (20). In adults, proteinuria is considered the excretion of more than 150 mg of urinary PRO per day and is suggestive of renal disease. Therefore, accurate PRO results are necessary for the screening and diagnosis of clinical diseases. The PRO parameter results obtained using the UA-5600 were compared with the results obtained using the BS-2800 biochemical detector. The consistency rates of negative and positive results between the two were both greater than 90%, indicating their superior detection performance. The consistency rates of the negative and positive urine GLU results obtained using the BS-2800M were both greater than 95%. These results indicate that the Mindray UA-5600 can be used as a reliable urine screening system to identify various abnormal samples in a timely and accurate manner and to reduce the workload of clinical laboratory physicians.
In recent years, the value of ML technology in differential diagnosis has increased (21), and ML is being widely used in early diagnosis, differential diagnosis, prognosis prediction, and survival analysis (22). The area under the curve (AUC), as an effective measure of accuracy has been considered with a meaningful interpretation, the AUC of 0.5 has the lowest fidelity and no application value, and the closer the AUC is to 1.0 the more authentic the detection method is. A perfect assay when sensitivity and specificity are 100% and AUC =1.0 (23,24). In this study, eight ML algorithms were used to establish diagnostic models of renal disease. Other than that for the KNN model, the AUCs for the other models were all greater than 0.9, and the NB model had the highest sensitivity. Therefore, the NB model had the best results (Table 4). To validate the screening efficacy of the NB model, the NB model was tested using a validation set of 508 cases and a confusion matrix. Among the results, there were 23 false-negative samples and 22 false-positive samples (Figure 4A). An analysis of the 23 false-negative samples showed that there were 15 cases of CKD, 4 cases of IgA nephropathy, and 2 cases each of latent nephritis and purpura nephritis. At different stages of CKD, the clinical manifestations differ. Before stage 3 CKD, patients may have no symptoms or only mild discomfort, such as fatigue, backache, and nocturia; a small number of patients may have loss of appetite, metabolic acidosis, and mild anemia; after stage 3 CKD, the above symptoms are more common. Obviously, symptoms worsen further after patients enter renal failure. These patients may not have abnormal urine test results. The 4 IgA nephropathy patients were all receiving treatment, and the patients with latent nephritis and purpura nephritis were under medication review.
The model constructed in this study was based on the results of 11 urine dry chemistry tests conducted using the UA-5600; there were only urine test results, and there were false-negative results. Next, a SHAP model (Figure 4B) was used to evaluate the importance of features, which were ranked based on the predicted feature importance in all the samples. Each point represented a sample, and the top three variables that contributed to the model were PRO, BLD, and LEU. Red represented a high feature value, and blue represented a low feature value. A positive SHAP value indicated an increased risk of renal disease. A higher PRO value represented higher PRO reflectance (lower PRO). As Figure 4B shows, red was negatively correlated with renal disease development, while lower PRO reflectance (higher PRO) was positively correlated with renal disease.
Our study had a number of limitations. First, the samples used in this study were from retrospective (not prospective) trials, resulting in inevitable selection bias. Second, the positive sample size was relatively small; therefore, studies with more samples need to be conducted to reduce the problems caused by data imbalances and further improve the diagnostic ability of the model. Third, only the detection parameters of the UA-5600 were used, while the results of other biochemical or blood tests were not used. Therefore, the inclusion of other detection results into the ML models may yield better performance. These suggestions provide opportunities for future research.
Conclusions
The Mindray UA-5600 demonstrates robust detection abilities for both BLD and LEU, and its results for PRO and GLU align closely with those obtained from the chemistry analyzer. The NB model had a good screening ability for the 11 tests it detected after being trained with eight ML models. By combining urine test results with machine learning, this also provides new ideas for urine dry chemistry test results to assist in the screening of kidney disease.
Acknowledgments
Funding: This study was funded by
Footnote
Reporting Checklist: The authors have completed the STARD reporting checklist. Available at https://tau.amegroups.com/article/view/10.21037/tau-24-189/rc
Data Sharing Statement: Available at https://tau.amegroups.com/article/view/10.21037/tau-24-189/dss
Peer Review File: Available at https://tau.amegroups.com/article/view/10.21037/tau-24-189/prf
Conflicts of Interest: All authors have completed the ICMJE uniform disclosure form (available at https://tau.amegroups.com/article/view/10.21037/tau-24-189/coif). The authors have no conflicts of interest to declare.
Ethical Statement: The authors are accountable for all aspects of the work in ensuring that questions related to the accuracy or integrity of any part of the work are appropriately investigated and resolved. Ethical approval for this study was obtained from the Medical Ethics Committee of The First Affiliated Hospital of Sun Yat-sen University (approval No. [2021]660). Individual consent for this retrospective analysis was waived. The study was conducted in accordance with the Declaration of Helsinki (as revised in 2013).
Open Access Statement: This is an Open Access article distributed in accordance with the Creative Commons Attribution-NonCommercial-NoDerivs 4.0 International License (CC BY-NC-ND 4.0), which permits the non-commercial replication and distribution of the article with the strict proviso that no changes or edits are made and the original work is properly cited (including links to both the formal publication through the relevant DOI and the license). See: https://creativecommons.org/licenses/by-nc-nd/4.0/.
References
- Kavuru V, Vu T, Karageorge L, et al. Dipstick analysis of urine chemistry: benefits and limitations of dry chemistry-based assays. Postgrad Med 2020;132:225-33. [Crossref] [PubMed]
- Coppens A, Speeckaert M, Delanghe J. The pre-analytical challenges of routine urinalysis. Acta Clin Belg 2010;65:182-9. [Crossref] [PubMed]
- European urinalysis guidelines. Scand J Clin Lab Invest Suppl 2000;231:1-86. [PubMed]
- Oyaert MN, Himpe J, Speeckaert MM, et al. Quantitative urine test strip reading for leukocyte esterase and hemoglobin peroxidase. Clin Chem Lab Med 2018;56:1126-32. [Crossref] [PubMed]
- Kaido M, Yasuda M, Komeda H, et al. Prediction of presence of fastidious bacteria by the Fully Automated Urine Particle Analyzer UF-1000i in the case of ineffective antimicrobial therapy for urinary tract infection. J Infect Chemother 2023;29:443-52. [Crossref] [PubMed]
- Xie R, Li X, Li G, et al. Diagnostic value of different urine tests for urinary tract infection: a systematic review and meta-analysis. Transl Androl Urol 2022;11:325-35. [Crossref] [PubMed]
- Oyaert M, Delanghe JR. Semiquantitative, fully automated urine test strip analysis. J Clin Lab Anal 2019;33:e22870. [Crossref] [PubMed]
- Moor M, Banerjee O, Abad ZSH, et al. Foundation models for generalist medical artificial intelligence. Nature 2023;616:259-65. [Crossref] [PubMed]
- Aguirre U, Urrechaga E. Diagnostic performance of machine learning models using cell population data for the detection of sepsis: a comparative study. Clin Chem Lab Med 2022;61:356-65. [Crossref] [PubMed]
- Syed-Abdul S, Firdani RP, Chung HJ, et al. Artificial Intelligence based Models for Screening of Hematologic Malignancies using Cell Population Data. Sci Rep 2020;10:4583. [Crossref] [PubMed]
- Gould MK, Huang BZ, Tammemagi MC, et al. Machine Learning for Early Lung Cancer Identification Using Routine Clinical and Laboratory Data. Am J Respir Crit Care Med 2021;204:445-53. [Crossref] [PubMed]
- Jang EC, Park YM, Han HW, et al. Machine-learning enhancement of urine dipstick tests for chronic kidney disease detection. J Am Med Inform Assoc 2023;30:1114-24. [Crossref] [PubMed]
- Wu Y, Zhang J, Wang Y, et al. The association of hematuria on kidney clinicopathologic features and renal outcome in patients with diabetic nephropathy: a biopsy-based study. J Endocrinol Invest 2020;43:1213-20. [Crossref] [PubMed]
- Chen P, Mao M, Wang C, et al. Preliminary study on the efficacy of rituximab in the treatment of idiopathic membranous nephropathy: A single-centre experience. Front Endocrinol (Lausanne) 2023;14:1044782. [Crossref] [PubMed]
- Deng M, Wu R, Zhou X, et al. Analyses of the clinical and immunological characteristics of patients with lupus erythematosus. Indian J Dermatol 2022;67:205. [PubMed]
- Duan SB, Pan P, Xu Q, et al. Preliminary study of Huai Qi Huang granules delay the development of primary glomerular diseases in human. Ren Fail 2014;36:1407-10. [Crossref] [PubMed]
- Bai L, Xu Q, Wu Z. Performance analysis of urine formed element analyzer EH-2090 was found to have good accuracy in detecting RBCs and WBCs when compared to manual microscopic. Transl Androl Urol 2024;13:218-29. [Crossref] [PubMed]
- Szmulik M, Trześniewska-Ofiara Z, Mendrycka M, et al. A novel approach to screening and managing the urinary tract infections suspected sample in the general human population. Front Cell Infect Microbiol 2022;12:915288. [Crossref] [PubMed]
- Chen Y, Zhang Z, Diao Y, et al. Combination of UC-3500 and UF-5000 as a quick and effective method to exclude bacterial urinary tract infection. J Infect Chemother 2023;29:667-72. [Crossref] [PubMed]
- Yaghmour YM, Said SA, Ahmad AM. Biochemical and hematological findings and risk factors associated with kidney impairment in patients with COVID-19. J Med Biochem 2023;42:35-46. [Crossref] [PubMed]
- Shehab M, Abualigah L, Shambour Q, et al. Machine learning in medical applications: A review of state-of-the-art methods. Comput Biol Med 2022;145:105458. [Crossref] [PubMed]
- Niel O, Bastard P. Artificial Intelligence in Nephrology: Core Concepts, Clinical Applications, and Perspectives. Am J Kidney Dis 2019;74:803-10. [Crossref] [PubMed]
- Hajian-Tilaki K. Receiver Operating Characteristic (ROC) Curve Analysis for Medical Diagnostic Test Evaluation. Caspian J Intern Med 2013;4:627-35. [PubMed]
- Roumeliotis S, Schurgers J, Tsalikakis DG, et al. ROC curve analysis: a useful statistic multi-tool in the research of nephrology. Int Urol Nephrol 2024; Epub ahead of print. [Crossref] [PubMed]