A contrast-enhanced computed tomography-based radiomics nomogram for preoperative differentiation between benign and malignant cystic renal lesions
Highlight box
Key findings
• We developed a contrast-enhanced computed tomography-based comprehensive nomogram to distinguish between benign and malignant cystic renal lesions.
What is known and what is new?
• There remains a certain ambiguity that radiologists estimate probability of a malignancy among those classified as Class ≥ II (especially II, IIF and III) according to Bosniak v2019.
• Our developed nomogram combines radiomics features with clinical factors by means of the light gradient boosting machine algorithm.
What is the implication, and what should change now?
• The developed nomogram holds the potential to improve diagnostic accuracy and patient management in the assessment of cystic renal lesions.
Introduction
Compared with other traditional noninvasive diagnostic approaches, computed tomography (CT) scan has emerged as the most extensively utilized imaging modality for preoperative assessment and risk stratification of complex cystic renal masses, leading to the development of the Bosniak classification for cystic renal masses in 1986 (1,2). Based on plain scan and contrast-enhanced CT (CECT), the Bosniak classification system captures information related to the edge of the mass, cystic components, the shape and thickness of the partition, the shape and number of wall nodules, and fine enhancement in the tissue, providing more information for the preoperative qualitative diagnosis of cystic renal lesions (CRLs) (3). It has been recognized by radiologists and urologists for over 30 years. In the 2019 version of the Bosniak classification of cystic renal masses, additional quantitative and discriminative criteria were introduced to further enhance the specificity in predicting the likelihood of malignancy in CRLs (4). On the other hand, no significant improvement in diagnostic performance and interreader agreement is shown between v2005 and v2019 (5). Interobserver agreement remains to be solved mainly in Classes II, IIF (in which F represents follow-up) and III. High-risk CRLs (Classes IIF, III, and IV) also have a certain possibility of being benign. Tremendous previous Bosniak III lesions are inclined to be reidentified as Class IIF according to Bosniak v2019, leading to lessened sensitivity (6,7). The incorrect dependence on the Bosniak classification may bring about unexpected consequences such as renal impairment, unplanned reoperation and potential neoplastic transplantation (8).
Although routine interpretation of CT scan primarily focuses on the qualitative analysis of imaging features, there is a host of extra quantitative information that can be utilized for further research to enhance the overall accuracy of preoperative differentiation between benign cystic renal lesions (BCRLs) and malignant cystic renal lesions (MCRLs). Radiomics, a noninvasive reproducible low-cost technique extracting high-dimensional features from routinely acquired images, has been applied in oncology and development of machine learning methods, showing great prospects in differential diagnosis, treatment response assessment and prognosis prediction for diverse cancers (9,10). Machine learning and textural analysis have been successfully applied into the differential diagnosis of different kinds of renal cell carcinoma (RCC) in solid renal lesions, as reported in the literature (11-14). Yet, corresponding research on CRL has rarely been reported. Therefore, to enhance diagnosis sensitivity and overcome the limitations of biased visual image evaluation, we were dedicated to investigating the role of the CECT-based radiomics nomogram in preoperative differentiation between BCRL and MCRL. We present this article in accordance with the TRIPOD reporting checklist (available at https://tau.amegroups.com/article/view/10.21037/tau-23-656/rc).
Methods
Data acquisition
The study was approved by the Ethics Committee of The Affiliated Hospital of Xuzhou Medical University (No. XYFY2023-KL118-01), and the ethics committee clarified that informed consent could be waived due to data anonymization. The study was conducted in accordance with the Declaration of Helsinki (as revised in 2013). During data collection and analysis, the privacy and confidentiality of the participants’ information were strictly protected. There were 192 patients with histologically confirmed BCRL or MCRL from January 2014 to December 2023 included in our study on the ground of specific inclusion and exclusion criteria (Figure 1). Patients’ genernal clinical information (age, gender, hematuria, etc.) and imaging features (Bosniak class, maximum diameter, etc.) were obtained from medical records and CT images.
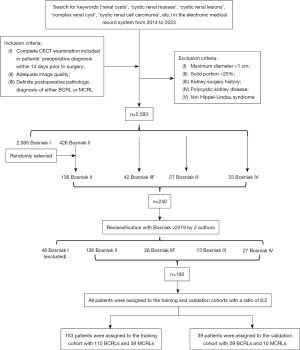
Imaging equipment
Each CT scan was conducted using the same CT scanner (Philips, Ingenuity CT, Suzhou, China) with the following parameters: tube voltage of 120 kVp; tube current of 260 mA; slice thickness of 1.5 mm; slice increment of 1.5 mm; field-of-view of 400 mm; image matrix of 512×512; iterative reconstruction algorithm (iDose). The scanning range included the inferior margin of the diaphragm to the pelvic cavity. An 80–100 mL volume of non-ionic contrast agent (loversol, Jiangsu Henrui Pharmaceutical Co., LTD., China) was injected into the anterior vein of the elbow using an electric syringe at an injection rate of 2.5 mL/s. CECT images of cortical phase, medullary phase and excretory phase were acquired at 30, 70 and 150 seconds after the injection. Voxel of each image is uniformly set to 0.5×0.5×3.
Image evaluation and segmentation
CT imaging analysis was performed independently by two senior radiologists, both of whom had at least 15 years of experience in urinary system imaging and were blind to participants’ medical records. By referring to Bosniak v2019, they perused CT images to elaborate the following features and reached a consensus: wall thickness, wall calcification, intracystic fluid density, internal septa, and the size and number of cysts. Any discrepancies or disagreements were resolved through consultation. The region of interest (ROI) segmentation was also performed by two experienced radiologists independently using the ITK-SNAP software (version 4.0, http://www.itksnap.org). Firstly, the CT images of each patient were loaded into the software. Then, the radiologists manually outlined the boundaries of the cystic renal masses through each consecutive axial slice on the images of the medullary phase, carefully including all relevant structures and excluding any adjacent normal tissues and artifacts. To ensure consistency and minimize interobserver variability, a consensus meeting was held to resolve any disagreements or discrepancies in the ROI segmentation between the two radiologists, which guaranteed the accuracy and reliability of the segmented ROIs. After the ROI segmentation, image preprocessing techniques were applied to standardize the CT images and correct for any variations in image acquisition and patient positioning. This step included intensity normalization, image resampling, and noise reduction, ensuring that all CT images were in a uniform format for subsequent radiomics feature extraction and analysis. Figure 2 depicts the analysis workflow of radiomics.
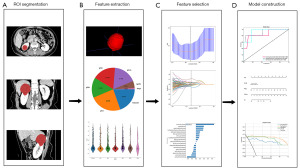
Radiomics feature extraction
The handcrafted features were categorized into 3 types: geometry, intensity and texture. Geometric features encompassed the shape and size characteristics of the ROI in the image. Intensity features focused on the statistical properties of pixel intensities within the ROI, including mean, standard deviation, maximum and minimum values. Texture features captured the spatial distribution and patterns of pixel intensities within the ROI using methods such as gray-level co-occurrence matrix (GLCM), gray-level dependence matrix (GLDM), gray-level run length matrix (GLRLM), gray-level size zone matrix (GLSZM) and neighborhood gray-tone difference matrix (NGTDM) (15). All of the aforementioned features were extracted with PyRadiomics, a Python package specifically designed for medical image analysis, which allows for the extraction of a wide range of quantitative features from medical images. For detailed information and usage instructions, please refer to its official website: https://pyradiomics.readthedocs.io.
Development of the radiomics model, clinical model and radiomics nomogram
Two feature selection methods for dimension reduction, Pearson’s correlation coefficient and the least absolute shrinkage and selection operator (LASSO), were put into application to remove redundant information, improve computational efficiency and make data visualized. Firstly, Pearson’s correlation coefficient was utilized to assess the linear relationship between different features and identify redundant or highly correlated ones. Secondly, the LASSO algorithm was applied to select and retain the most relevant and informative features for further analysis. Based on the tuning parameter λ, LASSO shrank all regression coefficients to zero, effectively setting the coefficients of many irrelevant features to precisely zero. To find the optimal λ, a fivefold cross-validation approach was employed, where the final value of λ yielded the lowest cross-validation error. The retained features with nonzero coefficients were combined to form a radiomics signature, and each patient’s radiomics score (Rad-score) was obtained by weighting the selected features with their respective LASSO model coefficients. Afterwards, we attained a Rad-score for each patient that captured the most discriminative radiomics features, enabling a more precise and informative characterization of the studied images.
The differences in clinical data and CT features between BCRL and MCRL were assessed using univariate analysis. Univariate Logistic regression analysis was first performed to determine the relationship between each clinical factor and the malignant potential of CRL. The significant features identified from the univariate logistic regression analysis in the training cohort were then included in the stepwise multivariate logistic regression to construct the clinical signature. Odds ratios (ORs) and 95% confidence intervals (CIs) were calculated for each independent factor.
A comprehensive model was built, combining the radiomics signature with the clinical model by means of the light gradient boosting machine (LightGBM) algorithm. After finishing LASSO feature screening, the final features were input to construct the risk model through fivefold cross-validation. Furthermore, to evaluate the incremental prognostic value of the radiomics signature in combination with clinical risk factors, a radiomics nomogram was constructed and applied to the validation cohort using the LightGBM analysis.
Statistical analysis
Statistical analysis was performed with the Statistical Product Service Solutions (SPSS) software (version 24.0, IBM). Continuous variables following a normal distribution were compared using the t-test, while the Mann-Whitney U test was used for non-normally distributed continuous variables. Categorical variables were compared using the Chi-squared test or Fisher’s exact test as appropriate. Calibration efficiency of the nomogram was assessed through calibration curves, and Hosmer-Lemeshow analytical fit was employed to assess its calibration ability.
Results
Clinical variables and construction of the clinical model
There were 192 patients enrolled in our study (Table 1). The univariate logistic regression analysis demonstrated there were significant differences in maximum diameter and Bosniak classification between BCRL and MCRL patients (P<0.05). As was shown in the multivariate logistic regression analysis, maximum diameter and Bosniak class were independent clinical factors in the clinical model (Table 2). Larger maximum diameter [odds ratio (OR) =0.959; 95% CI: 0.903–1.000; P=0.003] and higher Bosniak class (OR =1.542; 95% CI: 1.446–1.644; P<0.001) were more frequently observed in patients with MCRL. The ultimate clinical model exhibited an area under the curve (AUC) of 0.948 (95% CI: 0.917–0.980) in the training cohort and 0.936 (95% CI: 0.859–1.000) in the validation cohort.
Table 1
Clinical factors | Training cohort (n=153) | Validation cohort (n=39) | |||||||
---|---|---|---|---|---|---|---|---|---|
All | BCRLs | MCRLs | P value | All | BCRLs | MCRLs | P value | ||
Age (years) | 59.15±11.48 | 59.47±11.35 | 58.18±11.96 | 0.45 | 57.44±14.60 | 58.86±14.25 | 53.30±15.58 | 0.27 | |
BMI (kg/m2) | 25.15±3.18 | 24.94±2.66 | 25.78±4.38 | 0.21 | 25.70±3.79 | 25.66±3.18 | 25.82±5.41 | 0.69 | |
Maximum diameter (cm) | 5.93±1.83 | 6.16±1.65 | 5.25±2.18 | 0.02 | 6.25±1.97 | 6.84±1.45 | 4.52±2.32 | 0.005 | |
Gender | 0.17 | 0.77 | |||||||
Male | 108 (70.59) | 85 (73.91) | 23 (60.53) | 23 (58.97) | 18 (62.07) | 5 (50.00) | |||
Female | 45 (29.41) | 30 (26.09) | 15 (39.47) | 16 (41.03) | 11 (37.93) | 5 (50.00) | |||
Hypertension | 0.57 | 0.87 | |||||||
Yes | 37 (24.18) | 26 (22.61) | 11 (28.95) | 9 (23.08) | 6 (20.69) | 3 (30.00) | |||
No | 116 (75.82) | 89 (77.39) | 27 (71.05) | 30 (76.92) | 23 (79.31) | 7 (70.00) | |||
Hematuria, n (%) | >0.99 | >0.99 | |||||||
Yes | 2 (1.31) | 1 (0.87) | 1 (2.63) | 1 (2.56) | 1 (3.45) | Null | |||
No | 151 (98.69) | 114 (99.13) | 37 (97.37) | 38 (97.44) | 28 (96.55) | 10 (100.00) | |||
Lumbago, n (%) | 0.42 | 0.29 | |||||||
Yes | 42 (27.45) | 34 (29.57) | 8 (21.05) | 6 (15.38) | 6 (20.69) | Null | |||
No | 111 (72.55) | 81 (70.43) | 30 (78.95) | 33 (84.62) | 23 (79.31) | 10 (100.00) | |||
Amount of masses, n (%) | 0.36 | 0.62 | |||||||
Simple | 93 (60.78) | 67 (58.26) | 26 (68.42) | 31 (79.49) | 22 (75.86) | 9 (90.00) | |||
Multiple | 60 (39.22) | 48 (41.74) | 12 (31.58) | 8 (20.51) | 7 (24.14) | 1 (10.00) | |||
Bosniak class, n (%) | <0.001 | <0.001 | |||||||
II | 104 (67.97) | 93 (80.87) | 11 (28.95) | 22 (56.41) | 21 (72.41) | 1 (10.00) | |||
IIF | 20 (13.07) | 17 (14.78) | 3 (7.89) | 6 (15.38) | 5 (17.24) | 1 (10.00) | |||
III | 11 (7.19) | 5 (4.35) | 6 (15.79) | 2 (5.13) | 2 (6.90) | Null | |||
IV | 18 (11.76) | Null | 18 (47.37) | 9 (23.08) | 1 (3.45) | 8 (80.00) |
Data are presented as mean ± SD or n (%). CRLs, cystic renal lesions; BCRLs, benign cystic renal lesions; MCRLs, malignant cystic renal lesions; SD, standard deviation; BMI, body mass index.
Table 2
Clinical factors | Univariate logistic regression analysis | Multivariate logistic regression analysis | |||||
---|---|---|---|---|---|---|---|
P value | OR | 95% CI | P value | OR | 95% CI | ||
Maximum diameter | 0.007 | 0.950 | 0.921–0.980 | 0.003 | 0.959 | 0.937–0.981 | |
Bosniak class | <0.001 | 1.552 | 1.454–1.657 | <0.001 | 1.542 | 1.446–1.644 | |
Gender | 0.12 | 0.887 | 0.781–1.006 | – | – | – | |
Age | 0.55 | 0.998 | 0.993–1.003 | – | – | – | |
BMI | 0.16 | 1.016 | 0.997–1.035 | – | – | – | |
Hypertension | 0.43 | 1.067 | 0.931–1.221 | – | – | – | |
Hematuria | 0.41 | 1.290 | 0.774–2.151 | – | – | – | |
Lumbago | 0.31 | 0.923 | 0.811–1.051 | – | – | – | |
Amount of masses | 0.27 | 0.923 | 0.820–1.040 | – | – | – |
OR, odds ratio; CI, confidence interval; BMI, body mass index.
Construction of radiomics signature and radiomics model
There were 1,834 radiomics features extracted from CT images in the medullary phase, including 7 categories: 14 shape-based features, 360 firstorder statistics features, 440 GLCM features, 280 GLDM features, 320 GLRLM features, 320 GLSZM features and 100 NGTDM features. One hundred and six features were first selected by Pearson’s correlation coefficient, and 21 meaningful features were then selected by LASSO. Mean standard error (MSE) and coefficients of fivefold cross-validation were calculated (Figure 3). The coefficients value in the final selected nonzero features are included in the Rad-score calculation as follows:
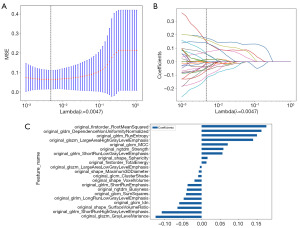
The variables A to U represent the selected radiomics features (Table 3). The radiomics model showed good discrimination ability in the training cohort (AUC =0.990; 95% CI: 0.979–1.000). In the validation cohort, the AUC was 0.959 (95% CI: 0.903–1.000).
Table 3
Variables | Radiomics feature name |
---|---|
A | original_firstorder_RootMeanSquared |
B | original_firstorder_TotalEnergy |
C | original_glcm_ClusterShade |
D | original_glcm_Idn |
E | original_glcm_MCC |
F | original_glcm_SumSquares |
G | original_gldm_DependenceNonUniformityNormalized |
H | original_glrlm_LongRunLowGrayLevelEmphasis |
I | original_glrlm_RunEntropy |
J | original_glrlm_ShortRunEmphasis |
K | original_glrlm_ShortRunHighGrayLevelEmphasis |
L | original_glrlm_ShortRunLowGrayLevelEmphasis |
M | original_glszm_GrayLevelVariance |
N | original_glszm_LargeAreaHighGrayLevelEmphasis |
O | original_glszm_LargeAreaLowGrayLevelEmphasis |
P | original_ngtdm_Busyness |
Q | original_ngtdm_Strength |
R | original_shape_Maximum3DDiameter |
S | original_shape_Sphericity |
T | original_shape_SurfaceVolumeRatio |
U | original_shape_VoxelVolume |
glcm, gray-level co-occurrence matrix; Idn, inverse difference normalized; MCC, Matthews correlation coefficient; gldm, gray-level dependence matrix; glrlm, gray-level run length matrix; glszm, gray-level size zone matrix; ngtdm, neighborhood gray-tone difference matrix; 3D, three-dimensional.
Construction and evaluation of the combined model and the nomogram
The Rad-score and independent clinical variables including maximum diameter and Bosniak class were combined to create the radiomics nomogram. The nomogram model utilizing the LightGBM algorithm and combining the clinical signature with the radiomics signature demonstrates the best performance in the receiver operating characteristic (ROC) curves for both the training cohort (AUC =0.989; 95% CI: 0.977–1.000) and the validation cohort (AUC =0.962; 95% CI: 0.905–1.000) (Figure 4A,4B, Table 4).
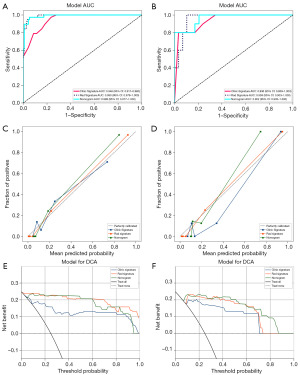
Table 4
Models | ACC | AUC (95% CI) | SENS | SPEC | PPV | NPV | Precision | Recall | F1 | Threshold |
---|---|---|---|---|---|---|---|---|---|---|
Training cohort (n=153) | ||||||||||
Clinical model | 0.837 | 0.948 (0.917–0.980) | 0.895 | 0.817 | 0.618 | 0.959 | 0.618 | 0.895 | 0.731 | 0.183 |
Radiomics model | 0.948 | 0.990 (0.979–1.000) | 0.947 | 0.948 | 0.857 | 0.982 | 0.857 | 0.947 | 0.900 | 0.335 |
Radiomics nomogram | 0.948 | 0.989 (0.977–1.000) | 0.947 | 0.948 | 0.857 | 0.982 | 0.857 | 0.947 | 0.900 | 0.216 |
Validation cohort (n=39) | ||||||||||
Clinical model | 0.744 | 0.936 (0.859–1.000) | 0.800 | 0.897 | 0.571 | 0.963 | 0.571 | 0.800 | 0.667 | 0.179 |
Radiomics model | 0.897 | 0.959 (0.903–1.000) | 0.900 | 0.897 | 0.750 | 0.963 | 0.750 | 0.900 | 0.818 | 0.157 |
Radiomics nomogram | 0.923 | 0.962 (0.905–1.000) | 0.700 | 1.000 | 1.000 | 0.906 | 1.000 | 0.700 | 0.824 | 0.468 |
ACC, accuracy; AUC, area under the curve; CI, confidence interval; SENS, sensitivity; SPEC, specificity; PPV, positive predictive value; NPV, negative predictive value; F1, F1 score.
The nomogram calibration curves yielded acceptable agreement in predicting and observing MCRL between the training and validation cohort (Figure 4C,4D). The decision curve analysis (DCA) was assessed for each model’s clinical utility (Figure 4E,4F). Compared to scenarios where no prediction model is used (i.e., treat-all or treat-none scheme), the radiomics nomogram demonstrates a significant benefit for intervention in patients with a prediction probability compared to the clinical model.
The Hosmer-Lemeshow test P values for the clinical model, the radiomics model and the radiomics nomogram indicate no significant difference in both the training and validation cohorts (P>0.05) (Table 5). Additionally, in order to compare these three models, DeLong’s test was utilized. In the training cohort, there was a significant difference between the AUC of the radiomics nomogram and the clinical model (P=0.002), while there was no significant difference between the AUC of the radiomics nomogram and the radiomics model (P=0.23). In the validation cohort, there was a significant difference in AUC between the radiomics nomogram and the clinical model (P=0.048), while there was no significant difference in AUC between the radiomics nomogram and the radiomics model (P=0.20).
Table 5
Models | Training cohort | Validation cohort |
---|---|---|
Clinical model | 0.053 | 0.58 |
Radiomics model | 0.98 | 0.16 |
Radiomics nomogram | 0.14 | 0.16 |
We also developed a nomogram to visualize the combined model, which allows adding points for each variable to the respective axes, thus assessing the risk of MCRL (Figure 5). The higher total scores a patient gets, the greater risk of MCRL the patient is exposed to.
Discussion
Our study developed a CECT-based comprehensive model to distinguish between BCRL and MCRL, which combines radiomics features, maximum diameter and Bosniak class, demonstrating excellent predictive value and fit with an AUC of 0.989 in the training cohort and 0.962 in the validation cohort. Besides, the combined model outperformed the clinical model alone in terms of predictive performance in both the training and validation cohorts. As it has been revealed, a radiomics nomogram is a noninvasive reproducible low-cost technique for differential diagnosis between BCRL and MCRL and related clinical decisions.
Despite the effectiveness of the Bosniak classification as a preoperative diagnostic tool for complex cystic renal masses since its introduction in 1986 (16), there remains a certain ambiguity that radiologists estimate probability of a malignancy among those classified as Class ≥ II (especially II, IIF and III) according to Bosniak v2019. Not in the slightest is Bosniak I of malignant potential, defined by a series of strict criteria, and any CRLs that do not satisfy these criteria ought to be “complicated” or “atypical” rather than “simple” (4,17). The composition and internal structure of Bosniak II lesions, compared to Class I, assumes a certain degree of complexity and a low risk of malignancy (accounting for approximately 1–5%) (9,18,19). As for Bosniak ≥ IIF lesions, there exists a higher risk that approximately 10–20% of Bosniak IIF lesions, 50% of Bosniak III lesions and 80% Bosniak IV lesions are diagnosed as malignancy (4,18,20,21). In short, none of the classes except Class I are adequately predictive of the lesion (22), and there is manifested interobserver variability among Bosniak II, IIF and III lesions (23). More attention should be paid to the preoperative screening for malignant cystic renal neoplasms. To address this, the present CECT-based radiomics nomogram to distinguish between BCRL and MCRL was developed, aiming to pursue better treatment strategies and improve patients’ medical experience.
Clinical and imaging information is in favor of correct differentiation between BCRL and MCRL. Previous research linked male gender, younger age, obesity, hypertension history to the likelihood of malignancy in CRL (24,25); whereas, the present study did not find significant correlations between these clinical factors [i.e., gender, age, body mass index (BMI) and hypertension] and the risk of malignancy in CRL, which may require a larger cohort study to validate these findings. Hematuria and lumbago serve as warning signs that necessitates further evaluation and imaging leading to a diagnosis and treatment plan (26). Based on the Bosniak classification, CECT is widely recognized as the diagnostic criterion for assessing CRL (20). The 2019 version of the Bosniak classification system employs several parameters to evaluate the malignant potential of renal cysts, including the thickness of the cyst wall, the degree of wall enhancement, the presence of wall nodules, internal enhancement patterns and the existence of internal hemorrhage or calcification (4). The clinical model predicted a higher probability of malignancy for CRL with a higher Bosniak class observed in the medullary phase. Moreover, maximum diameter, which is not directly involved in the Bosniak classification, turned out associated with the malignancy probability of CRL in our study. Volpe et al. (27) showed that if small renal masses that were presumed to be RCCs were managed conservatively and monitored with regular imaging, approximately one-third of them would show growth. Masses with larger sizes are more likely to have a risk of malignancy than those with smaller sizes.
The rationale for utilizing CT texture analysis to discriminate between BCRL and MCRL is predicated on three key considerations. Firstly, heterogeneous cystic masses are more likely to exhibit malignancy compared to their homogeneous counterparts. Secondly, texture analysis offers an automated approach, thereby minimizing the influence of reader interpretation. Lastly, the feasibility of applying texture analysis to single-phase CT scans is of paramount importance, given the frequent detection of cystic renal masses through such scans. Notably, the assignment of Bosniak class in CT often necessitates a comprehensive examination, encompassing scans both prior to and following intravenous contrast administration. Hence, the ability to assess CRL solely through single-phase CT scans holds the potential to obviate the need for supplementary examinations.
Previous studies showed that texture analysis of CT images can be utilized to differentiate between BCRL and MCRL. Dana et al. (28) structured a decision algorithm in combination with consensus radiological readings of Bosniak classification and radiomics-based risks, and the result showed that the machine learning model demonstrated excellent diagnostic accuracy (AUC =0.96; balanced accuracy =94%) in predicting malignant tumors within the validation cohorts, superior to the Bosniak classification management guidelines. Wang et al. (29) optimized a machine learning model by combining a logistic regression classifier and the synthetic minority oversampling technique algorithm. They established a nomogram that incorporated the radiomics signature and independent clinical variables, resulting in an impressive AUC of 0.972 (95% CI: 0.942–1.000). Miskin et al. (30) conducted an efficiency analysis of three texture-based machine learning algorithms (random forest, multivariate logistic regression and support vector machine) of 144 CRLs, all of which showed fine performance with an AUC of 0.79, 0.80 and 0.76, respectively. Alhussaini et al. (31) used 5 maximum likelihood (ML) algorithms to train models of 3 cohorts and gained an AUC of 1.00±0.000, 1.00±0.000 and 0.87±0.073 in the best model, respectively, which proved that ML-based radiomics signatures were potentially useful for distinguishing chromophobe RCC and renal oncocytomas. For predicting low- and high-grade clear cell RCC, He et al. (32) constructed five predictive models by selecting conventional image features and radiomic features, in which the CIF-CMP-minMSE (CIF, conventional image feature; CMP, cortico-medullary phase; minMSE, minimum mean squared error) was the optimal predictive model, with an AUC of 0.986. Compared with the aforementioned investigations, our study using the LightGBM algorithm highlights the potential of the radiomics nomogram as a valuable tool for distinguishing between BCRL and MCRL. The utilization of advanced radiomics techniques in combination with machine learning algorithms allows for a comprehensive analysis of complex imaging data. The superior discriminative capability of the radiomics nomogram, as evidenced by higher AUC values, suggests its potential as a non-invasive and reliable method for accurate tumor identification. Furthermore, the incorporation of diverse radiomic features into the nomogram offers a more comprehensive representation of the underlying tumor characteristics, enhancing its predictive power. This comprehensive approach may aid clinicians in making well-informed decisions, leading to more personalized and effective treatment strategies for patients with CRLs.
Although our study has demonstrated promising results in differentiating between BCRL and MCRL using the CECT-based radiomics nomogram, there are several limitations that should be acknowledged. Firstly, the sample size of our study is relatively small. The ratio between benign and malignant is 75%:25% indicating a potential class imbalance, which may restrict the generalizability of our findings to larger and more diverse populations. Secondly, the data were assembled from a single institution, which could introduce institutional bias and may not fully represent the broader patient population. Thirdly, although we have taken measures to ensure data quality and accuracy, inherent variability in image acquisition and radiomic feature extraction may still be present. Future prospective studies with larger and multi-institutional cohorts are warranted to validate the performance and reproducibility of the radiomics nomogram in clinical practice. While the radiomics nomogram exhibits promising performance, its clinical implementation will require rigorous validation and integration into existing diagnostic protocols.
Conclusions
Our study proposes a CECT-based radiomics nomogram as an advanced and promising tool for preoperative differentiation between BCRL and MCRL. The developed nomogram demonstrates favorable discriminative capability, providing valuable insights for clinical decision-making and patient management. With further validation and integration into routine clinical practice, this radiomics approach holds the potential to enhance diagnostic accuracy and improve patient outcomes in the assessment of CRLs.
Acknowledgments
Funding: None.
Footnote
Reporting Checklist: The authors have completed the TRIPOD reporting checklist. Available at https://tau.amegroups.com/article/view/10.21037/tau-23-656/rc
Data Sharing Statement: Available at https://tau.amegroups.com/article/view/10.21037/tau-23-656/dss
Peer Review File: Available at https://tau.amegroups.com/article/view/10.21037/tau-23-656/prf
Conflicts of Interest: All authors have completed the ICMJE uniform disclosure form (available at https://tau.amegroups.com/article/view/10.21037/tau-23-656/coif). The authors have no conflicts of interest to declare.
Ethical Statement: The authors are accountable for all aspects of the work in ensuring that questions related to the accuracy or integrity of any part of the work are appropriately investigated and resolved. The study was conducted in accordance with the Declaration of Helsinki (as revised in 2013). The study was approved by the Ethics Committee of The Affiliated Hospital of Xuzhou Medical University (No. XYFY2023-KL118-01), and the ethics committee clarified that informed consent could be waived due to data anonymization.
Open Access Statement: This is an Open Access article distributed in accordance with the Creative Commons Attribution-NonCommercial-NoDerivs 4.0 International License (CC BY-NC-ND 4.0), which permits the non-commercial replication and distribution of the article with the strict proviso that no changes or edits are made and the original work is properly cited (including links to both the formal publication through the relevant DOI and the license). See: https://creativecommons.org/licenses/by-nc-nd/4.0/.
References
- Leveridge MJ, Bostrom PJ, Koulouris G, et al. Imaging renal cell carcinoma with ultrasonography, CT and MRI. Nat Rev Urol 2010;7:311-25. [Crossref] [PubMed]
- Bosniak MA. The current radiological approach to renal cysts. Radiology 1986;158:1-10. [Crossref] [PubMed]
- Bosniak MA. The use of the Bosniak classification system for renal cysts and cystic tumors. J Urol 1997;157:1852-3. [Crossref] [PubMed]
- Silverman SG, Pedrosa I, Ellis JH, et al. Bosniak Classification of Cystic Renal Masses, Version 2019: An Update Proposal and Needs Assessment. Radiology 2019;292:475-88. [Crossref] [PubMed]
- Dana J, Gauvin S, Zhang M, et al. CT-based Bosniak classification of cystic renal lesions: is version 2019 an improvement on version 2005? Eur Radiol 2023;33:1297-306. [Crossref] [PubMed]
- Schoots IG, Zaccai K, Hunink MG, et al. Bosniak Classification for Complex Renal Cysts Reevaluated: A Systematic Review. J Urol 2017;198:12-21. [Crossref] [PubMed]
- Yan JH, Chan J, Osman H, et al. Bosniak Classification version 2019: validation and comparison to original classification in pathologically confirmed cystic masses. Eur Radiol 2021;31:9579-87. [Crossref] [PubMed]
- Soputro NA, Kapoor J, Zargar H, et al. Malignant ascites following radical nephrectomy for cystic renal cell carcinoma. BMJ Case Rep 2021;14:e243103. [Crossref] [PubMed]
- Lambin P, Leijenaar RTH, Deist TM, et al. Radiomics: the bridge between medical imaging and personalized medicine. Nat Rev Clin Oncol 2017;14:749-62. [Crossref] [PubMed]
- Chen J, Remulla D, Nguyen JH, et al. Current status of artificial intelligence applications in urology and their potential to influence clinical practice. BJU Int 2019;124:567-77. [Crossref] [PubMed]
- Hodgdon T, McInnes MD, Schieda N, et al. Can Quantitative CT Texture Analysis be Used to Differentiate Fat-poor Renal Angiomyolipoma from Renal Cell Carcinoma on Unenhanced CT Images? Radiology 2015;276:787-96. [Crossref] [PubMed]
- Leng S, Takahashi N, Gomez Cardona D, et al. Subjective and objective heterogeneity scores for differentiating small renal masses using contrast-enhanced CT. Abdom Radiol (NY) 2017;42:1485-92. [Crossref] [PubMed]
- Schieda N, Thornhill RE, Al-Subhi M, et al. Diagnosis of Sarcomatoid Renal Cell Carcinoma With CT: Evaluation by Qualitative Imaging Features and Texture Analysis. AJR Am J Roentgenol 2015;204:1013-23. [Crossref] [PubMed]
- Lubner MG, Stabo N, Abel EJ, et al. CT Textural Analysis of Large Primary Renal Cell Carcinomas: Pretreatment Tumor Heterogeneity Correlates With Histologic Findings and Clinical Outcomes. AJR Am J Roentgenol 2016;207:96-105. [Crossref] [PubMed]
- Gillies RJ, Kinahan PE, Hricak H. Radiomics: Images Are More than Pictures, They Are Data. Radiology 2016;278:563-77. [Crossref] [PubMed]
- Weibl P, Klatte T, Waldert M, et al. Complex renal cystic masses: current standards and controversies. Int Urol Nephrol 2012;44:13-8. [Crossref] [PubMed]
- Hélénon O, Crosnier A, Verkarre V, et al. Simple and complex renal cysts in adults: Classification system for renal cystic masses. Diagn Interv Imaging 2018;99:189-218. [Crossref] [PubMed]
- Ascenti G, Mazziotti S, Cicero G. Considerations Regarding Bosniak II Lesions. Radiology 2020;296:E127-8. [Crossref] [PubMed]
- Sevcenco S, Spick C, Helbich TH, et al. Malignancy rates and diagnostic performance of the Bosniak classification for the diagnosis of cystic renal lesions in computed tomography - a systematic review and meta-analysis. Eur Radiol 2017;27:2239-47. [Crossref] [PubMed]
- Bosniak MA. Diagnosis and management of patients with complicated cystic lesions of the kidney. AJR Am J Roentgenol 1997;169:819-21. [Crossref] [PubMed]
- Smith AD, Carson JD, Sirous R, et al. Active Surveillance Versus Nephron-Sparing Surgery for a Bosniak IIF or III Renal Cyst: A Cost-Effectiveness Analysis. AJR Am J Roentgenol 2019;212:830-8. [Crossref] [PubMed]
- Cloix P, Martin X, Pangaud C, et al. Surgical management of complex renal cysts: a series of 32 cases. J Urol 1996;156:28-30. [Crossref] [PubMed]
- Siegel CL, McFarland EG, Brink JA, et al. CT of cystic renal masses: analysis of diagnostic performance and interobserver variation. AJR Am J Roentgenol 1997;169:813-8. [Crossref] [PubMed]
- Han HH, Choi KH, Oh YT, et al. Differential diagnosis of complex renal cysts based on lesion size along with the Bosniak renal cyst classification. Yonsei Med J 2012;53:729-33. [Crossref] [PubMed]
- Oh TH, Seo IY. The role of Bosniak classification in malignant tumor diagnosis: A single institution experience. Investig Clin Urol 2016;57:100-5; discussion 105. [Crossref] [PubMed]
- Gray RE, Harris GT. Renal Cell Carcinoma: Diagnosis and Management. Am Fam Physician 2019;99:179-84. [PubMed]
- Volpe A, Panzarella T, Rendon RA, et al. The natural history of incidentally detected small renal masses. Cancer 2004;100:738-45. [Crossref] [PubMed]
- Dana J, Lefebvre TL, Savadjiev P, et al. Malignancy risk stratification of cystic renal lesions based on a contrast-enhanced CT-based machine learning model and a clinical decision algorithm. Eur Radiol 2022;32:4116-27. [Crossref] [PubMed]
- Wang T, Yang H, Hao D, et al. A CT-based radiomics nomogram for distinguishing between malignant and benign Bosniak IIF masses: a two-centre study. Clin Radiol 2023;78:590-600. [Crossref] [PubMed]
- Miskin N, Qin L, Silverman SG, et al. Differentiating Benign From Malignant Cystic Renal Masses: A Feasibility Study of Computed Tomography Texture-Based Machine Learning Algorithms. J Comput Assist Tomogr 2023;47:376-81. [Crossref] [PubMed]
- Alhussaini AJ, Steele JD, Nabi G. Comparative Analysis for the Distinction of Chromophobe Renal Cell Carcinoma from Renal Oncocytoma in Computed Tomography Imaging Using Machine Learning Radiomics Analysis. Cancers (Basel) 2022;14:3609. [Crossref] [PubMed]
- He X, Zhang H, Zhang T, et al. Predictive models composed by radiomic features extracted from multi-detector computed tomography images for predicting low- and high- grade clear cell renal cell carcinoma: A STARD-compliant article. Medicine (Baltimore) 2019;98:e13957. [Crossref] [PubMed]