Machine learning developed an intratumor heterogeneity signature for predicting clinical outcome and immunotherapy benefit in bladder cancer
Highlight box
Key findings
• The current study developed an optimal intratumor heterogeneity (ITH) related signature for bladder cancer patients.
What is known and what is new?
• ITH is involved in tumor progression and metastasis and high ITH can accelerate tumor evolution.
• Our study comprehensively explored the prognosis of ITH-related genes in bladder cancer.
What is the implication, and what should change now?
• ITH related signature acted as an indicator for predicting prognosis, stratifying risk and guiding treatment for bladder cancer patients.
Introduction
Bladder cancer carries a large societal burden, with over 570,000 new diagnosed cases and 210,000 deaths globally each year (1). Although multidisciplinary comprehensive approaches combining surgical excision, chemoradiotherapy and immune therapy have been used to treat bladder cancer cases, many patients still experience tumor progress and relapse, leading to treatment failure (2,3). And the primary reasons are the intratumor heterogeneity (ITH) and the complex mechanism of tumor development (4,5). Currently, limited effective markers have been developed to predict the prognosis and therapy benefits of bladder cancer patients.
ITH is referred to the phenomenon in which individual tumor cells exhibit different genomic and phenotypic characteristics (6). Study has revealed the correlation between ITH and the randomness of gene mutation and environmental factors (7). ITH is involved in tumor progression and metastasis and high ITH can accelerate tumor evolution (8). Cancer patients with high ITH score have been shown to present resistance to treatment and inferior clinical outcomes (9,10). Considering the vital role of ITH, comprehensive exploration of the genes mediating ITH in bladder cancer and identification of their role in evaluating the clinical outcome and therapy benefits in bladder cancer seem particularly necessary.
We identified those genes correlated with ITH in bladder cancer and developed an ITH-related signature (IRS) based on the data from the Cancer Genome Atlas (TCGA) and Gene Expression Omnibus (GEO). We also explored the role of IRS in predicting the prognosis, immune infiltration, and therapy benefits in bladder cancer, providing insights into prognosis prediction and immune landscape in bladder cancer. We present this article in accordance with the TRIPOD reporting checklist (available at https://tau.amegroups.com/article/view/10.21037/tau-24-5/rc).
Methods
Data acquisition and ITH score of bladder cancer cases
The study was conducted in accordance with the Declaration of Helsinki (as revised in 2013). Following inclusion criteria (pathological diagnosis of bladder urothelium carcinoma with completed prognostic information) and exclusion criteria (followed up for less than one month or died within one month after surgery or with other malignancies), we acquired mRNA data of bladder cancer patients from TCGA dataset (n=396), gene series expression (GSE)13507 (n=165), GSE31684 (n=90), GSE32984 (n=223) and GSE48276 (n=73) dataset. Three datasets [IMvigor210 dataset (bladder cancer, n=298), GSE91061 (skin cutaneous melanoma, n=98), and GSE78220 (skin cutaneous melanoma, n=28)] with patients receiving anti-programmed cell death protein 1 (PD-1) or cytotoxic T-lymphocyte associated protein 4 (CTLA4) therapy were applied to evaluate the role of IRS in drug sensitivity of immunotherapy. Using DEPTH2 method, an algorithm for evaluating ITH, we calculated the ITH score of bladder cancer cases in TCGA dataset (11). After separating bladder cancer cases into low and high ITH score, we then explored with correlation between ITH score and the clinical “characteristics of bladder cancer patients. With “limma” packages, we identified differentially expressed genes (DEGs) between low and high ITH score (|Log2 fold change| value >1.5 and P value <0.05).
Integrative machine learning algorithms constructed an optimal IRS
DEGs were submitted for univariate cox analysis to screen potential prognostic biomarkers in bladder cancer. This was followed by construction of a stable prognostic IRS with integrative machine learning analytical procedure based on these potential prognostic biomarkers. A total of 10 machine learning methods were included in the analysis procedure as described in the previous study (12). Following the process of previous studies (R scripts in https://github.com/Zaoqu-Liu/IRLS) (12,13), we constructed the IRS with the following steps: (I) predictive signatures of TCGA dataset was fitted with 101 algorithm combinations using potential biomarkers; (II) all algorithm combinations were performed in GEO cohorts; (III) in all cohorts, we then calculated C-index. Based on the IRS genes and their coefficient, the IRS score (risk score) of bladder cancer patients was determined and separated bladder cancer cases into two groups (high and low risk score) using the best cutoff, which was decided by “surv_cutpoint” function in the R package “survminer”.
The prognostic role of IRS in bladder cancer
The generation of the survival curves was determined by Kaplan-Meier survival method. We drew receiver operating characteristic (ROC) curve and C-index curve with the “survivalROC” R package. We also collected 52 gene signatures (Table S1) that had been constructed for bladder cancer and determined with their C-index. By comparing the C-index of our IRS and 52 gene signatures, we could evaluate the performance of IRS and other signatures in predicting the clinical outcome of bladder cancer patients. Using univariate and multivariate Cox analyses, we then identified the risk factors for the prognosis of bladder cancer patients. To predict the clinical outcome of bladder cancer patients, a predictive nomogram was developed with IRS-based risk score and other clinical parameters by “nomogramEx” package. The difference between actual and predicted survival was visualized using the calibration curve.
Immune infiltration analysis
The immune score and ESTIMATE score of bladder cancer cases were determined by ESTIMATE algorithm (14). The correlation between IRS and immune cells was evaluated by seven methods [TIMER (15), xCell (16), MCP-counter (17), CIBERSORT (18), CIBERSORT-ABS (19), EPIC (20), and quanTIseq (21)] (22). We used ssGSEA method to evaluate gene set score correlated with immune cells and immune-related activities or functions in bladder cancer with “GSVA” package. Relative level of human leukocyte antigen (HLA)-related genes and immune checkpoints in high and low IRS score group of bladder cancer cases was visualized with “ggpubr” or “ggplot” R package.
Drug sensitivity analysis
From TIDE website (https://tide.dfci.harvard.edu/) (23), we obtained tumor immune dysfunction and exclusion (TIDE) score of bladder cancer patients. Immunophenoscore was obtained from The Cancer Immunome Atlas website (https://tcia.at/home) (24). Tumor mutation burden (TMB) score was downloaded from TCGA database. These scores were used to evaluate the performance of IRS in predicting the immunotherapy response of bladder cancer cases. Based on the data of Genomics of Drug Sensitivity in Cancer (https://www.cancerrxgene.org/), the half maximal inhibitory concentration (IC50) of drugs in each bladder cancer case was determined with the “oncoPredict R” package. A higher IC50 value indicated lower sensitivity.
Statistical analysis
Cox (proportional hazards) regression analysis was performed to identify prognostic value of IRGs in bladder cancer. Integrative procedure containing 10 machine learning methods was applied to develop an IRS. The unpaired Student’s t-test, one-way analysis of variance (ANOVA), the Chi-square test, or Fisher’s exact test was used for analysis as appropriate. Statistical analyses were performed using R software 3.5.0.
Results
The ITH score of bladder cancer cases
Table S2 shows the ITH score of bladder cancer cases. Bladder cancer patients with high clinical stage and T stage had a higher ITH score (Figure 1A). After separating bladder cancer into low and high ITH score, we found a lower overall survival (OS) rate in patients with high ITH score (Figure 1B, P<0.001). We then explored the DEGs between low and high ITH score group for identifying genes mediating the ITH of bladder cancer. As shown in Figure 1C, a total of 625 genes were obtained (fold change =1.5, P<0.05). Further analysis suggested 20 genes (EPS8, SLC14A1, FKBP5, CTSH, FLOT2, SNCG, NLRP2, GNPTAB, TUBB6, BTG2, RFC4, RAB9A, LTB4R, PDHA1, CORO1C, SERPINE2, NFIA, NAP1L3, EMP1, PPARG) as potential biomarkers for the prognosis of bladder cancer patients (Figure 1D).
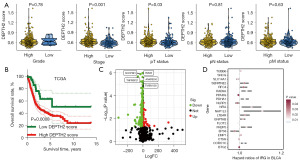
Machine learning developed a prognostic IRS
These 20 genes were submitted into the machine learning-based integrative procedure, with which we constructed an IRS. We fitted 101 kinds prediction models via the LOOCV framework in TCGA cohort, and further calculated the C-index of each model across all GEO cohorts (Figure 2A). The IRS constructed by Enet (alpha =0.2) algorithm had a highest average C-index of 0.69, which was suggested as the optimal IRS (Figure 2A). Based on Enet (alpha =0.2) algorithm, the IRS was developed using 17 genes and the IRS score (risk score) of bladder cancer cases were calculated using following the formula: risk score = (−0.1176) × EPS8exp + (−0.0080) × SLC14A1exp + (−0.1366) × FLOT2exp + 0.0051 × SNCGexp + (−0.2515) × NLRP2exp + 0.1574 × GNPTABexp + (−0.0024) × TUBB6exp + (−0.0365) × BTG2exp + 0.0766 × RFC4exp + 0.1402 × RAB9Aexp + 0.0304 × LTB4Rexp + 0.1462 × PDHA1exp + 0.0483 × SERPINE2exp + 0.0737 × NFIAexp + 0.1300 × NAP1L3exp + 0.0756 × EMP1exp + (−0.0319) × PPARG exp. To separate bladder cancer into high and low IRS score group, we used the best cut-off determined by “surv_cutpoint” function in the R package “survminer”. We found that bladder cancer patients with low IRS score had a favorable OS rate in TCGA, GSE13507, GSE31684, GSE32894 and GSE48276 datasets, with 1-, 3- and 5-year area under curve s of 0.744, 0.791 and 0.816 in TCGA cohort; 0.679, 0.680 and 0.705 in GSE13507 cohort; 0.677, 0.690 and 0.697 in GSE31684 cohort; 0.772, 0.789 and 0.783 in GSE32894 cohort, not available (NA), 0.655 and 0.720 in GSE48276 cohort, respectively (Figure 2B-2F).
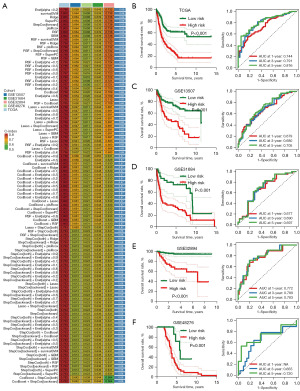
Evaluation of the performance of IRS
As shown in Figure 3A, high ITH score indicated an advanced clinical stage in bladder cancer in TCGA, GSE13507, and GSE32894 dataset. The C-index of IRS and these clinical parameters were calculated for comparing their role in predicting OS rate of bladder cancer cases. The result revealed a higher C-index of IRS in all TCGA and GEO datasets than that of clinical parameters (age, gender, tumor grade and clinical stage) (Figure 3B). Moreover, by performing univariate and multivariate cox regression analyses, we found that IRS acted as an independent risk factor for the clinical outcome of bladder cancer cases in TCGA and all GEO datasets (Figure 3C,3D, all P<0.05). Numerous prognostic models have been constructed for bladder cancer. To compare the performance of our IRS and other signatures in evaluating the prognosis of bladder cancer, we then randomly collected 52 published prognostic models (Table S1) and compared with their C-index. As shown Figure 3E, the C-index of IRS was higher than these prognostic models in TCGA cohort. For predicting the clinical outcome of bladder cancer patients, we constructed a nomogram with risk score and stage (Figure 3F). The results of the nomogram were in good agreement with the observed 1-, 3- and 5-year OS rates in the TCGA cohort (Figure 3G).
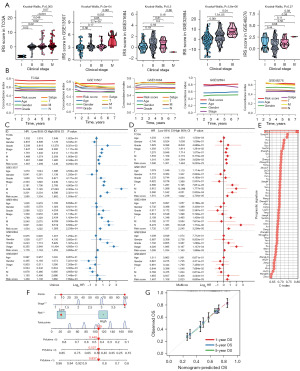
The difference of functional enrichments in different IRS score groups
Figure 4A shows the overall correlation between IRS score and the abundance of immune cells based on seven evaluating methods. As shown in Figure 4B-4D, there were negative correlations between IRS score and the abundance of immune-activated cells, including CD8+ T cells, NK cell and macrophages M1. ssGSEA analysis suggested a higher score of many immune cells, including B cells, CD8+ T cells, DCs, mast cells, NK cells, and TIL (Figure 4E, all P<0.05). In bladder cancer patients with low IRS score, they had a higher gene set score correlated with APC_co_stimulation, CCR, checkpoints, cytolytic activity, T cell co-stimulation and IFN response (Figure 4F, all P<0.05). The results also suggested a higher stromal score, immune score and ESTIMAE score in bladder cancer patients with low IRS score (Figure 4G-4I, all P<0.001).
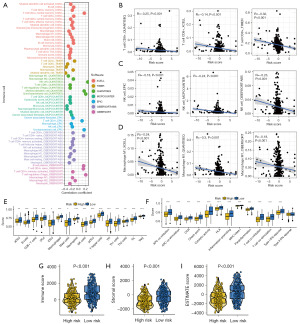
IRS as an indicator for predicting therapy benefits in bladder cancer
Immunophenoscore and TMB were indicators for predicting immunotherapy benefits and high Immunophenoscore and TMB correlated with higher immunotherapy benefits (25). In our study, the data suggested a higher PD1 & CTLA4 immunophenoscore and TMB score in bladder cancer patients with low IRS score (Figure 5A,5B, all P<0.05). Previous study highlighted the vital role of TIDE score in predicting the response to immunotherapy (23,26). As shown in Figure 5C,5D, bladder cancer patients with high IRS score had a higher score of TIDE, T cell exclusion and dysfunction and immune escape (all P<0.05). A wider range of antigen presentation in cancer patients with high HLA-related gene and immune checkpoints expression could increase the likelihood of immunotherapy benefits (27). The results suggested higher expression of immune checkpoints and HLA-related genes in bladder cancer patients with low IRS score (Figure 5E,5F, all P<0.05). These results may suggest a better immunotherapy benefit in bladder cancer with low IRS score. To further evaluate the performance of IRS in predicting immunotherapy benefits, we then calculated the IRS score in immunotherapy datasets. In bladder cancer patients receiving anti-PD1 therapy, responders had a lower IRS score versus non-responders (Figure 5G, P<0.05). Moreover, there was a worse clinical outcome in bladder cancer patients with high IRS score (P=0.006). Compared with high IRS score patients, low IRS score patients had a higher immunotherapy response rate (P<0.01). Similar results were obtained in another two immunotherapy datasets (GSE91061 and GSE78220) (Figure 5H,5I). Chemotherapy and targeted therapy also play a vital role in the therapy of bladder cancer. Thus, we also explore the IC50 value of some drug for bladder cancer therapy. As shown in Figure 6A,6B, bladder cancer patients with low IRS score had a lower IC50 value of camptothecin, cisplatin, docetaxel, cyclophosphamide, mitoxantrone, dasatinib, linsitinib, nilotinib, trametinib, and foretinib, demonstrating a better sensitivity to chemotherapy and targeted therapy in bladder cancer patients with low IRS score.
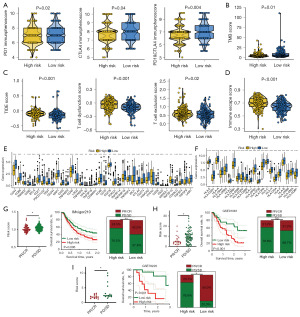
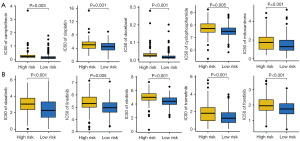
Dissection of the functional enrichment difference in different IRS score group
To clarify how IRS genes contributing to the progression and ITH of bladder cancer, we then explored functional enrichment difference in different IRS score group. A higher gene sets score involved in angiogenesis, coagulation, glycolysis, hedgehog signaling, hypoxia, mTORC1 signaling, NOTCH signaling, IL2-STAT5 signaling, P53 pathway and IL6-JAK-STAT3 signaling were obtained in bladder cancer with high IRS score (Figure 7A). Figure 7B,7C show the results of GSEA analysis, suggesting that high IRS score was significantly correlated with NOTCH signaling pathway, JAK-STAT signaling and NOD like receptor signaling while low IRS score was significantly correlated with chemokine signaling and cytokine-cytokine receptor interaction.
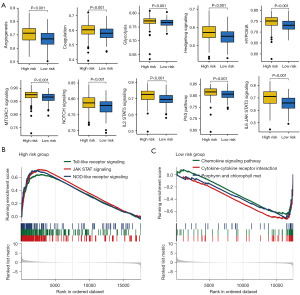
Discussion
In our study, a total of 10 integrative machine learning methods were used to construct a powerful IRS for bladder cancer. The predicted model constructed by Enet (alpha =0.2) algorithm had a highest average C-index of 0.69, which has been suggested as the optimal IRS, and it had a good performance in predicting the clinical outcome of bladder cancer cases. Moreover, the results also suggested IRS as indicator for predicting immunotherapy benefits.
Based on 17 potential prognostic biomarkers, including EPS, SLC14A1, FLOT2, SNCG, NLRP2, GNPTAB, TUBB6, BTG, RFC4, RAB9A, LTB4R, PDHA1, SERPINE2, NFIA, NAP1L3, EMP1, PPARG, we constructed the current IRS. SLC14A1 was suggested as a novel target for bladder cancer and correlates with tumor progression (28). SNCG correlated with the proliferation and invasiveness of bladder cancer (29). In bladder cancer, Kim et al. identified TUBB6 as an indicator for muscle-invasion and poor prognosis (30). High expression of NFIA is correlated with early pT stages and lower grade in bladder cancer (31). Loss of EMP1 accelerates tumor metastasis in bladder cancer (32). PPARG signaling could control bladder cancer subtype and immune exclusion (33).
Our results suggested IRS as an independent risk factor in bladder cancer and it had a powerful performance in predicting the clinical outcome of patients. Previous study has suggested ITH signature as indicator for predicting the clinical outcome of patients with other types of cancer. In colon adenocarcinoma, IRS could predict patients’ prognosis and chemotherapy response (34). IRS is correlated with the prognosis of hepatocellular carcinoma patients (35).
Increasing evidences highlight the vital role of immunotherapy in the therapeutic strategy of bladder cancer (36,37). In our investigation, we found a lower TIDE score, lower immune escape score, higher PD1 & CTLA4 immunophenoscore, higher TMB score, higher response rate and better prognosis in bladder cancer patients with low IRS score. TMB is an indicator in immunotherapy and high TMB correlates a better response to immunotherapy (38,39). Moreover, avelumab survival benefit is positively associated with TMB score (40). Patients with low TIDE score have a less likelihood of immune escape (23). Thus, we suggested IRS as an indicator for predicting immunotherapy benefits and low IRS score correlated with a favorable immunotherapy benefit. Recent study show that fibroblast growth factor receptor 3 (FGFR3) alterations indicates a higher objective response rate in bladder cancer patients received checkpoint inhibitors (41).
To clarify how IRS genes contributing to the progression and ITH of bladder cancer, we then explored functional enrichment difference in different IRS score group. We found a higher gene sets score involved in angiogenesis, coagulation, glycolysis, hedgehog signaling, hypoxia, mTORC1 signaling, NOTCH signaling, IL2-STAT5 signaling, P53 pathway and IL6-JAK-STAT3 signaling in bladder cancer with high IRS score. Pathways connected to angiogenesis might have been correlated with reduced survival benefit in bladder cancer (40). Glycolysis was involved in the tumorigenesis of bladder cancer (42). Correlated with tumor growth and metastasis, angiogenesis has been suggested as prognostic marker and target for bladder cancer (43). NOTCH signaling favors IITH, resulting in tumor progression of cancer (44). Hypoxia is correlated with ITH and immune evasion in cancer (45).
There are some limitations in our study. The result of our study was not verified using in-house cohort. Moreover, it would be better to explore the functional mechanism of IRS in bladder cancer.
Conclusions
The current study developed an optimal IRS for bladder cancer patients, which acted as an indicator for predicting prognosis, stratifying risk and guiding treatment for bladder cancer patients.
Acknowledgments
Funding: None.
Footnote
Reporting Checklist: The authors have completed the TRIPOD reporting checklist. Available at https://tau.amegroups.com/article/view/10.21037/tau-24-5/rc
Peer Review File: Available at https://tau.amegroups.com/article/view/10.21037/tau-24-5/prf
Conflicts of Interest: All authors have completed the ICMJE uniform disclosure form (available at https://tau.amegroups.com/article/view/10.21037/tau-24-5/coif). The authors have no conflicts of interest to declare.
Ethical Statement: The authors are accountable for all aspects of the work in ensuring that questions related to the accuracy or integrity of any part of the work are appropriately investigated and resolved. The study was conducted in accordance with the Declaration of Helsinki (as revised in 2013).
Open Access Statement: This is an Open Access article distributed in accordance with the Creative Commons Attribution-NonCommercial-NoDerivs 4.0 International License (CC BY-NC-ND 4.0), which permits the non-commercial replication and distribution of the article with the strict proviso that no changes or edits are made and the original work is properly cited (including links to both the formal publication through the relevant DOI and the license). See: https://creativecommons.org/licenses/by-nc-nd/4.0/.
References
- Sung H, Ferlay J, Siegel RL, et al. Global Cancer Statistics 2020: GLOBOCAN Estimates of Incidence and Mortality Worldwide for 36 Cancers in 185 Countries. CA Cancer J Clin 2021;71:209-49. [Crossref] [PubMed]
- Dyrskjøt L, Hansel DE, Efstathiou JA, et al. Bladder cancer. Nat Rev Dis Primers 2023;9:58. [Crossref] [PubMed]
- Grayson M. Bladder cancer. Nature 2017;551:S33. [Crossref] [PubMed]
- Xu H, Jiao D, Liu A, et al. Tumor organoids: applications in cancer modeling and potentials in precision medicine. J Hematol Oncol 2022;15:58. [Crossref] [PubMed]
- Lavallee E, Sfakianos JP, Mulholland DJ. Tumor Heterogeneity and Consequences for Bladder Cancer Treatment. Cancers (Basel) 2021;13:5297. [Crossref] [PubMed]
- Yadav SS, Stockert JA, Hackert V, et al. Intratumor heterogeneity in prostate cancer. Urol Oncol 2018;36:349-60. [Crossref] [PubMed]
- Andor N, Graham TA, Jansen M, et al. Pan-cancer analysis of the extent and consequences of intratumor heterogeneity. Nat Med 2016;22:105-13. [Crossref] [PubMed]
- Gerlinger M, Rowan AJ, Horswell S, et al. Intratumor heterogeneity and branched evolution revealed by multiregion sequencing. N Engl J Med 2012;366:883-92. [Crossref] [PubMed]
- Li J, Byrne KT, Yan F, et al. Tumor Cell-Intrinsic Factors Underlie Heterogeneity of Immune Cell Infiltration and Response to Immunotherapy. Immunity 2018;49:178-193.e7. [Crossref] [PubMed]
- Dagogo-Jack I, Shaw AT. Tumour heterogeneity and resistance to cancer therapies. Nat Rev Clin Oncol 2018;15:81-94. [Crossref] [PubMed]
- Song D, Wang X. DEPTH2: an mRNA-based algorithm to evaluate intratumor heterogeneity without reference to normal controls. J Transl Med 2022;20:150. [Crossref] [PubMed]
- Li Z, Guo M, Lin W, et al. Machine Learning-Based Integration Develops a Macrophage-Related Index for Predicting Prognosis and Immunotherapy Response in Lung Adenocarcinoma. Arch Med Res 2023;54:102897. [Crossref] [PubMed]
- Liu Z, Liu L, Weng S, et al. Machine learning-based integration develops an immune-derived lncRNA signature for improving outcomes in colorectal cancer. Nat Commun 2022;13:816. [Crossref] [PubMed]
- Yoshihara K, Shahmoradgoli M, Martínez E, et al. Inferring tumour purity and stromal and immune cell admixture from expression data. Nat Commun 2013;4:2612. [Crossref] [PubMed]
- Li T, Fan J, Wang B, et al. TIMER: A Web Server for Comprehensive Analysis of Tumor-Infiltrating Immune Cells. Cancer Res 2017;77:e108-10. [Crossref] [PubMed]
- Aran D, Hu Z, Butte AJ. xCell: digitally portraying the tissue cellular heterogeneity landscape. Genome Biol 2017;18:220. [Crossref] [PubMed]
- Helmink BA, Reddy SM, Gao J, et al. B cells and tertiary lymphoid structures promote immunotherapy response. Nature 2020;577:549-55. [Crossref] [PubMed]
- Chen B, Khodadoust MS, Liu CL, et al. Profiling Tumor Infiltrating Immune Cells with CIBERSORT. Methods Mol Biol 2018;1711:243-59. [Crossref] [PubMed]
- Steen CB, Liu CL, Alizadeh AA, et al. Profiling Cell Type Abundance and Expression in Bulk Tissues with CIBERSORTx. Methods Mol Biol 2020;2117:135-57. [Crossref] [PubMed]
- Racle J, Gfeller D. EPIC: A Tool to Estimate the Proportions of Different Cell Types from Bulk Gene Expression Data. Methods Mol Biol 2020;2120:233-48. [Crossref] [PubMed]
- Plattner C, Finotello F, Rieder D. Deconvoluting tumor-infiltrating immune cells from RNA-seq data using quanTIseq. Methods Enzymol 2020;636:261-85. [Crossref] [PubMed]
- Li T, Fu J, Zeng Z, et al. TIMER2.0 for analysis of tumor-infiltrating immune cells. Nucleic Acids Res 2020;48:W509-14. [Crossref] [PubMed]
- Fu J, Li K, Zhang W, et al. Large-scale public data reuse to model immunotherapy response and resistance. Genome Med 2020;12:21. [Crossref] [PubMed]
- Charoentong P, Finotello F, Angelova M, et al. Pan-cancer Immunogenomic Analyses Reveal Genotype-Immunophenotype Relationships and Predictors of Response to Checkpoint Blockade. Cell Rep 2017;18:248-62. [Crossref] [PubMed]
- Palmeri M, Mehnert J, Silk AW, et al. Real-world application of tumor mutational burden-high (TMB-high) and microsatellite instability (MSI) confirms their utility as immunotherapy biomarkers. ESMO Open 2022;7:100336. [Crossref] [PubMed]
- Lin A, Zhang J, Luo P. Crosstalk Between the MSI Status and Tumor Microenvironment in Colorectal Cancer. Front Immunol 2020;11:2039. [Crossref] [PubMed]
- Lin A, Yan WH. HLA-G/ILTs Targeted Solid Cancer Immunotherapy: Opportunities and Challenges. Front Immunol 2021;12:698677. [Crossref] [PubMed]
- Hou R, Kong X, Yang B, et al. SLC14A1: a novel target for human urothelial cancer. Clin Transl Oncol 2017;19:1438-46. [Crossref] [PubMed]
- Chen Z, Zhang F, Zhang S, et al. The down-regulation of SNCG inhibits the proliferation and invasiveness of human bladder cancer cell line 5637 and suppresses the expression of MMP-2/9. Int J Clin Exp Pathol 2020;13:1873-9. [PubMed]
- Kim B, Jung M, Moon KC, et al. Quantitative proteomics identifies TUBB6 as a biomarker of muscle-invasion and poor prognosis in bladder cancer. Int J Cancer 2023;152:320-30. [Crossref] [PubMed]
- Kaczorowski M, Matysiak J, Kielb P, et al. Nuclear Factor IA Is Down-regulated in Muscle-invasive and High-grade Bladder Cancers. Anticancer Res 2022;42:493-500. [Crossref] [PubMed]
- Liu S, Shi J, Wang L, et al. Loss of EMP1 promotes the metastasis of human bladder cancer cells by promoting migration and conferring resistance to ferroptosis through activation of PPAR gamma signaling. Free Radic Biol Med 2022;189:42-57. [Crossref] [PubMed]
- Tate T, Xiang T, Wobker SE, et al. Pparg signaling controls bladder cancer subtype and immune exclusion. Nat Commun 2021;12:6160. [Crossref] [PubMed]
- Liu C, Liu D, Wang F, et al. An Intratumor Heterogeneity-Related Signature for Predicting Prognosis, Immune Landscape, and Chemotherapy Response in Colon Adenocarcinoma. Front Med (Lausanne) 2022;9:925661. [Crossref] [PubMed]
- Liang J, Chen W, Ye J, et al. Single-cell transcriptomics analysis reveals intratumoral heterogeneity and identifies a gene signature associated with prognosis of hepatocellular carcinoma. Biosci Rep 2022;42:BSR20212560. [Crossref] [PubMed]
- Ruiz-Cordero R, Devine WP. Targeted Therapy and Checkpoint Immunotherapy in Lung Cancer. Surg Pathol Clin 2020;13:17-33. [Crossref] [PubMed]
- Ward Grados DF, Ahmadi H, Griffith TS, et al. Immunotherapy for Bladder Cancer: Latest Advances and Ongoing Clinical Trials. Immunol Invest 2022;51:2226-51. [Crossref] [PubMed]
- Liu L, Bai X, Wang J, et al. Combination of TMB and CNA Stratifies Prognostic and Predictive Responses to Immunotherapy Across Metastatic Cancer. Clin Cancer Res 2019;25:7413-23. [Crossref] [PubMed]
- Seo I, Lee HW, Byun SJ, et al. Neoadjuvant chemoradiation alters biomarkers of anticancer immunotherapy responses in locally advanced rectal cancer. J Immunother Cancer 2021;9:e001610. [Crossref] [PubMed]
- Powles T, Sridhar SS, Loriot Y, et al. Avelumab maintenance in advanced urothelial carcinoma: biomarker analysis of the phase 3 JAVELIN Bladder 100 trial. Nat Med 2021;27:2200-11. [Crossref] [PubMed]
- Komura K, Hirosuna K, Tokushige S, et al. The Impact of FGFR3 Alterations on the Tumor Microenvironment and the Efficacy of Immune Checkpoint Inhibitors in Bladder Cancer. Mol Cancer 2023;22:185. [Crossref] [PubMed]
- Wang JZ, Zhu W, Han J, et al. The role of the HIF-1α/ALYREF/PKM2 axis in glycolysis and tumorigenesis of bladder cancer. Cancer Commun (Lond) 2021;41:560-75. [Crossref] [PubMed]
- Streeter EH, Harris AL. Angiogenesis in bladder cancer--prognostic marker and target for future therapy. Surg Oncol 2002;11:85-100. [Crossref] [PubMed]
- Lim JS, Ibaseta A, Fischer MM, et al. Intratumoural heterogeneity generated by Notch signalling promotes small-cell lung cancer. Nature 2017;545:360-4. [Crossref] [PubMed]
- Terry S, Engelsen AST, Buart S, et al. Hypoxia-driven intratumor heterogeneity and immune evasion. Cancer Lett 2020;492:1-10. [Crossref] [PubMed]