A platelet-related signature for predicting the prognosis and immunotherapy benefit in bladder cancer based on machine learning combinations
Highlight box
Key findings
• The present work created a platelet-related signature to forecast bladder cancer prognosis and treatment benefit.
What is known and what is new?
• Platelets play vital functions in angiogenesis and tumor progression by releasing vascular endothelial growth factor and platelet-derived growth factor.
• Our study comprehensively explored the prognostic value of platelet related genes in bladder cancer.
What is the implication, and what should change now?
• Platelet-related signature served as a tool for prognosis prediction, risk stratification, and treatment guidance for bladder cancer patients.
Introduction
Bladder cancer carries a large societal burden, with over 570,000 newly diagnosed cases and 210,000 deaths globally each year (1). Non-muscle invasive and muscle invasive bladder cancer have distinct molecular subtypes with multiple pathogenic pathways (2). Cigarette smoking is the most common risk factor for the development and aggressiveness of bladder cancer (3). Bladder cancer is currently managed with surgery, chemoradiotherapy, targeted therapy, and immunotherapy; nonetheless, patient outcomes are not satisfactory, possibly because of the disease’s high incidence of progression and recurrence (2,4). Moreover, there are only limited biomarkers that can be applied” to evaluate the prognosis and drug sensitivity of bladder cancer patients. Therefore, it is necessary to explore and identify novel biomarkers for predicting the clinical outcomes and drug sensitivity of bladder cancer cases.
Platelets, one of main subtypes of blood cells, are involved in hemostasis and thrombosis (5). When the number of platelets reduces, spot bleeding, bruising, or purple spots may be resulted in. A severely reduced platelet count may result in bleeding in the brain or lung (6). Interestingly, increasing evidences highlight the vital role of platelets in cancer. Study indicates that upregulation of platelets is common in solid cancers (7). Platelets also play vital functions in angiogenesis and tumor progression by releasing vascular endothelial growth factor and platelet-derived growth factor (8). The level of platelets is correlated with the drug sensitivity in chemotherapy and immunotherapy (9-11). By interacting with many different immune cells, including T cells, macrophages and neutrophils, platelets engage in many biological processes in tumor (12,13). Thus, it is necessary to fully elucidate the role of platelet-related genes (PRGs) in bladder cancer.
Previous study showed that platelet-related signature (PRS) could serve as a prognostic marker in certain types of cancer. In triple-negative breast cancer, PRS acted as a biomarker for prognosis of patients (14). Another study also developed a PRS in pancreatic adenocarcinoma, providing new insights into prognosis evaluating and therapeutic decision-making (15). Moreover, PRS showed association with the prognosis in hepatocellular carcinoma by regulating cycling T cells (16). However, the role of PRS in bladder cancer is still unclear.
In this study, we explored the prognostic value of PRGs in bladder cancer and developed a PRS using the datasets from The Cancer Genome Atlas (TCGA) and Gene Expression Omnibus (GEO). The role of PRS in evaluating the clinical outcomes and drug sensitivity in bladder cancer was also investigated. We present this article in accordance with the TRIPOD reporting checklist (available at https://tau.amegroups.com/article/view/10.21037/tau-24-80/rc).
Methods
Data and PRGs acquisition
The study was conducted in accordance with the Declaration of Helsinki (as revised in 2013). Gene expression data of bladder cancer cases for PRS construction were downloaded from TCGA dataset (n=396), GSE13507 (n=165), GSE31684 (n=90), GSE32894 (n=223) and GSE48276 (n=73) dataset. Another three datasets, IMvigor210 dataset (n=298), GSE91061 (n=98), and GSE78220 dataset (n=28) were chosen as immunotherapy cohorts. Differentially expressed genes (DEGs) between in bladder cancer cases and normal tissues were isolated by “limma” packages and |log2fold change (FC)| value >1.5 and P value <0.05 were set as the cut-off. By researching the keyword “platelet”, we collected PRGs from gene set enrichment analysis (GSEA) gene sets (https://www.gsea-msigdb.org/gsea/index.jsp/) and a total 480 PRGs were obtained in our study (Box S1).
An optimal PRS developed with machine learning algorithms
After submitting DEGs into univariate cox analysis for the identification of potential prognostic biomarkers in bladder cancer, we then developed a reliable PRS with integrative machine learning analysis procedures including 10 machine learning methods. The analysis step was followed as described in previous study (R scripts in https://github.com/Zaoqu-Liu/IRLS) (17,18). PRS was developed with three steps: (I) in TCGA data set, prediction model was fitted with 101 algorithms combinations based on potential biomarkers; (II) these 101 algorithm combinations were verified in GEO cohorts; (III) across all cohorts, we calculated C-index of each model. According to the expression of PRS genes and their coefficient, we could obtain the risk score (PRS score) of each bladder cancer case.
The function of PRS in forecasting bladder cancer patients’ clinical results
Cases of bladder cancer were split into two groups (high and low risk score group), with the “surv_cutpoint” function in the R package “survminer” being used to determine the optimal cutoff. Overall survival curves of these two groups were drawn by Kaplan-Meier method. We then constructed the C-index curve and receiver operating characteristic (ROC) curve to assess PRS’s performance. Moreover, we then collected 52 gene signatures (Table S1) that have been constructed in bladder cancer and compared their C-index with PRS, with which We could assess how well PRS and other signatures in foretelling patients’ prognoses with bladder cancer. The risk factors for the prognosis of bladder cancer cases were determined by univariate and multivariate Cox analyses. A predictive nomogram was also constructed by “nomogramEx” package based on risk factors. Using the calibration curve, the discrepancy between actual and anticipated survival was displayed.
Immune infiltration analysis
Using ESTIMATE algorithm, we calculated the immune score and ESTIMATE score of each bladder cancer case (19). To evaluate the association between PRS and immune cells, we used seven methods, which were TIMER, xCell, MCP-counter, CIBERSORT, CIBERSORT-ABS, EPIC, and quanTIseq (20). Using “GSVA” package, we then calculated gene set score of immune cells and immune related functions.
Drug sensitivity analysis
We collected each bladder cancer case’s tumor mutation burden (TMB) score from the TCGA database. Immunophenoscore of each bladder cancer case was determined by The Cancer Immunome Atlas website (https://tcia.at/home). Using DEPTH2 method, an algorithm for evaluating intratumor heterogeneity, we calculated the intratumor heterogeneity (ITH) score of bladder cancer cases (21). Next, from TIDE website (https://tide.dfci.harvard.edu/), we acquired the bladder cancer cases’ tumor immune dysfunction and exclusion (TIDE) score. These scores were used to evaluate the likelihood of immune escape and immunotherapy benefits of bladder cancer cases. The half maximal inhibitory concentration (IC50) of drugs in bladder cancer cases were calculated by the “oncoPredict R” package based on the data of Genomics of Drug Sensitivity in Cancer (https://www.cancerrxgene.org/).
The Human Protein Atlas (https://www.proteinatlas.org/), a Swedish program that maps all human proteins in cells, tissues, and organs, provided the immunohistochemistry of the chosen gene (22).
Cell lines and knockdown of TUBA1C
Shanghai Institute of Biochemistry and Cell Biology (Shanghai, China) provided the bladder cancer cell lines (RT4, T24, J82, UM-UC-3, and 5637) and the normal bladder cell line (SV-HUC-1). Cells were kept in 37 ℃ conditions with 5% CO2 and 95% saturated humidity using the appropriate ATCC medium. Fetal bovine serum (FBS) (Gibco, California, USA) and 1% penicillin-streptomycin (Sigma-Aldrich, St. Louis, USA) were added to the medium. Following the manufacturer’s instructions, UM-UC-3 and 5637 cells were transfected with α1C-tubulin (TUBA1C) siRNA or scrambled negative control (NC) siRNA using Lipofectamine 3000 transfection reagent (Invitrogen, Thermo Fisher Scientific, Massachusetts, USA). The TUBA1C siRNA was obtained from RiboBio (Guangzhou, China) with the sequences were as follows: NC-siRNA (5'-UUCUCCGAACGUGUCACGUTT-3'), TUBA1C-siRNA #1 (5'-GCTTCAAGGTTGGCATTAA-3'); TUBA1C-siRNA #2 (5'-GAGCAATACCACAGCTGTT-3').
Real-time quantitative polymerase chain reaction (RT-qPCR), proliferation assay and wound healing assay
We extracted the RNA from the cells using TRIzol (Takara Bio, Dalian, China), and then we used an oligo (dT) primer to reverse transcribe the RNA into cDNA. We then used SYBR Premix Ex Taq (Takara Bio) for RT-qPCR, based on the ABI 7900HT detection system (Thermo Fisher Scientific). The levels of gene expression were compared to the endogenous GAPDH. The primers of TUBA1C were: forward primer, 5'-GACCTCGTGTTGGACCGAAT-3', reverse primer, 5'-CGAGGTGAACCCAGAACCAG-3'. Bladder cancer cell lines were plated in 96-well plates (5,000 cells/well in triplicate) for the proliferation assay. At the designated times, cells were supplemented with cell counting kit-8 (CCK-8; Beyotime, Shanghai, China). The ratio of the input cells’ OD value to the OD value at the specified time was used to compute the proliferation index.
Statistical analysis
The identification of prognostic value of PRGs was determined by Cox regression analysis. The unpaired Student’s t-test, one-way analysis of variance (ANOVA), the Chi-squared test, or Fisher’s exact test was used for analysis as appropriate. Statistical analyses were performed using R software 3.5.0.
Results
Identification of prognostic biomarkers in bladder cancer
A total of 5,084 DEGs were obtained in bladder cancer with |log2FC| ≥1.5 and P value <0.05 as the cutoff (Figure 1A), including 480 PRGs (Figure 1B). Further univariate cox analysis suggested 19 genes among these PRGs as potential prognostic biomarkers for bladder cancer patients, including P2RY1, PDPN, DGKQ, GATA3, P2RY12, MMRN1, RSU1, SRC, TUBB6, GNB3, HGF, GNB4, TUBA1C, MFN2, RABGAP1L, ORM1, PIK3R5, KIF1B, and TGFB2 (Figure 1C).
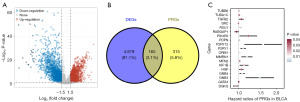
Machine learning developed a prognostic PRS
We then submitted these 19 genes into the machine learning-based integrative procedures for constructing a PRS. We fitted 101 different types of prediction models using the LOOCV framework in the TCGA cohort, as indicated in Figure 2A. We then determined the C-index for each model across all validation cohorts. The data showed that Enet (alpha =0.4) algorithm-based PRS developed with 10 genes had the highest average C-index of 0.73 and it should be the optimal PRS (Figure 2A). Enet (alpha =0.4) algorithm-based PRS score (risk score) of bladder cancer cases were calculated as followed: Risk score = 0.2011× P2RY1exp + 0.1512 × PDPNexp + (−0.1285) × DGKQexp + 0.1692 × MMRN1exp + 0.1568 × RSU1exp + 0.2870 × GNB3exp + 0.7020 × TUBA1Cexp + 0.0880 × MFN2exp + (−0.0941) × RABGAP1Lexp + 0.0006 × KIF1Bexp. Bladder cancer cases were separated into high and low PRS score groups using the best cut-off. As shown in Figure 2B-2F, bladder cancer patients with high PRS score portended a worse overall survival rate in TCGA, GSE13507, GSE31684, GSE32894 and GSE48276 datasets, with the area under the curves of 1-, 3- and 5-year ROC being 0.754, 0.779 and 0.806 in TCGA cohort; 0.627, 0.679 and 0.737 in GSE13507 cohort; 0.631, 0.694 and 0.707 in GSE31684 cohort; 0.788, 0.774 and 0.809 in GSE32894 cohort, 0.837, 0.729 and 0.722 in GSE48276 cohort, respectively.
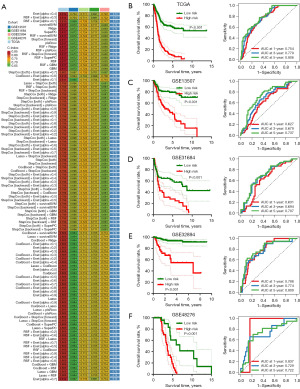
Evaluation of the performance of PRS
As shown in Figure 3A,3B, in the TCGA and all GEO datasets, the results of univariate and multivariate cox regression analyses showed PRS as an independent risk factor for the overall survival rate of patients with bladder cancer (all P<0.05). Compared with other clinical characters (age, gender, tumor grade and clinical stage), the C-index of PRS was higher in both TCGA and all GEO datasets (Figure 3C). We then compared the performance of PRS and many other signatures that have been constructed for evaluating the clinical outcomes of bladder cancer cases. The results found that the C-index of PRS was higher than these prognostic signatures in TCGA cohort (Figure 3D), suggesting the powerful and stable performance of PRS in predicting the clinical outcomes of bladder cancer cases. To predict the overall survival rate of bladder cancer patients, we also constructed a predicting nomogram with the risk factors (Figure 3E). Interestingly, we found that the nomogram was in good agreement with the observed 1-, 3- and 5-year overall survival rates in the TCGA cohort (Figure 3F).
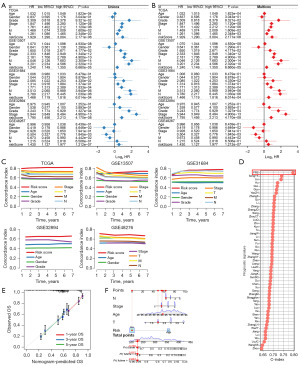
Analysis of the relationship between PRS and the tumor immunological milieu
The overall relationships between the PRS score and immune cell abundance are displayed in Figure 4A. PRS score showed negative correlations with the level of immune-activated cells, including CD8+ T cell, natural killer (NK) cell and macrophage M1 (Figure 4B-4D, all P<0.05). We also found a lower score of many immune cells in the high PRS score group in bladder cancer, including B cells, CD8+ T cells, mast cells, NK cells, Th1 and TIL (Figure 4E). As shown in Figure 4F, high PRS score indicated lower gene set scores correlated with APC_co_stimulation, cytolytic activity and T cell co-stimulation. Compared with the low PRS score group, the high PRS score group had a lower stromal score, immune score and ESTIMATE score in bladder cancer (Figure 4G-4I, all P<0.05).
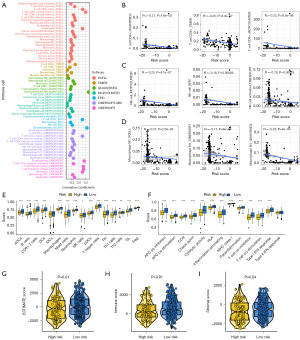
PRS as an indicator for predicting therapy benefits in bladder cancer
Higher level of HLA-related gene and immune checkpoints indicated higher likelihoods of immunotherapy benefits (23). As shown in Figure 5A,5B, bladder cancer patients with low PRS score presented higher expressions of immune checkpoints and HLA-related genes (all P<0.05). Immunophenoscore and TMB score were immunotherapy benefits indicators and higher Immunophenoscore and higher TMB score suggested better immunotherapy benefits (24). Compared with the high PRS score group, the low PRS score had a higher TMB score and programmed cell death protein 1 (PD-1) & cytotoxic T-lymphocyte associated protein 4 (CTLA4) immunophenoscore (Figure 5C,5D, all P<0.05). TIDE score and intratumor heterogeneity (ITH) score also played a vital role in predicting immunotherapy benefits (21,25,26). The results found a higher immune escape score, higher ITH score, and higher TIDE score in bladder cancer patients with high PRS score (Figure 5E-5G, all P<0.05). Thus, bladder cancer cases with low PRS score may have better immunotherapy benefits. We also verify the results using three immunotherapy datasets. In bladder cancer patients receiving anti-PD-1 therapy (IMvigor210 dataset), the data suggested a higher PRS score in non-responders (Figure 5H, P<0.05). Patients with high PRS score portended a worse overall survival rate (Figure 5H, P=0.002). Moreover, higher PRS score patients indicated a lower immunotherapy response rate (P<0.01). Similar results were obtained in another two immunotherapy datasets (GSE91061 and GSE78220) (Figure 5I-5J). Considering the vital function of chemotherapy and targeted therapy in the management of bladder cancer cases, we then investigated the IC50 value of some drugs in different PRS score groups. We found a lower IC50 value of docetaxel, cisplatin, 5-fluorouracil, paclitaxel, axitinib, crizotinib, foretinib and lapatinib in bladder cancer patients with low PRS score (Figure 6A,6B, P<0.05).
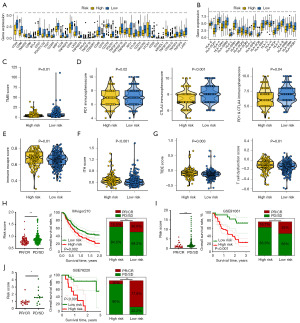
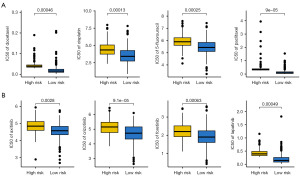
Dissection of the functional enrichment difference in different PRS score group
We then explored functional enrichment differences in different PRS score groups, which may clarify how PRS genes leading to the development of bladder cancer. As shown in Figure 7A, the low PRS score was correlated with lower gene set score involved in protein secretion, P53 pathway, NOTCH signaling, mTORC1 signaling, IL2-STAT5 signaling, hypoxia, glycolysis, DNA repair, coagulation, and angiogenesis, coagulation, glycolysis, hedgehog signaling, hypoxia, NOTCH signaling in bladder cancer. GSEA analysis demonstrated significant correlation between high PRS score and JAK-STAT signaling, Toll-like receptor signaling and focal adhesion (Figure 7B). Low PRS score was significantly correlated with cytokine-cytokine receptor interaction, asthma, and tryptophan metabolism (Figure 7C).
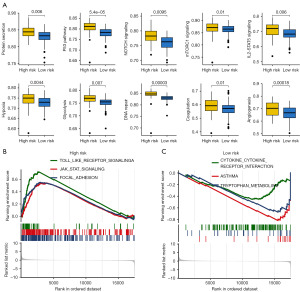
Biological roles played by the chosen gene
We chose TUBA1C, which made the largest contribution to the PRS (with the highest coefficient among all the genes in the PRS) for further analysis, in order to further confirm the PRS performance. Typical immunohistochemical labeling from Human Protein Atlas revealed that TUBA1C expression was higher in bladder cancer tissues, which is in line with the earlier findings (Figure 8A, TUBA1C image for bladder cancer tissues: https://www.proteinatlas.org/ENSG00000167553-TUBA1C/pathology/urothelial+cancer#img; TUBA1C image for normal bladder tissues: https://www.proteinatlas.org/ENSG00000167553-TUBA1C/tissue/urinary+bladder#img). Next, we investigated TUBA1C expression in bladder cancer cell lines, showing that majority of the cell lines had greater TUBA1C expression (Figure 8B). The CCK-8 assay results in the follow-up investigation demonstrated that TUBA1C knockdown clearly suppressed the proliferation of 5637 and UM-UC-3 cells (Figure 8C,8D, all P<0.05).
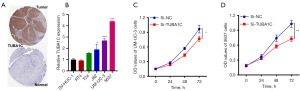
Discussion
In our study, we developed a powerful PRS for bladder cancer cases using 10 integrative machine learning methods. The data showed that Enet (alpha =0.4) algorithm-based PRS developed with 10 genes had the highest average C-index of 0.73 and it should be the optimal PRS. We found that PRS acted as an indicator and had a good performance in predicting the clinical outcomes and immunotherapy benefits of bladder cancer cases.
The PRS incorporated 10 potential prognostic biomarkers, including P2RY1, PDPN, DGKQ, MMRN1, RSU1, GNB3, TUBA1C, MFN2, RABGAP1L, KIF1B. These genes are involved in the development of bladder cancer. P2RY1 is correlated with multidrug-chemoresistance in bladder cancer (27). High PDPN expression indicates a poor prognosis in bladder cancer cases after surgery (28). GNB3 subunit C825T polymorphism is involved in disease progression in bladder cancer (29). TUBA1C acts as a prognostic biomarker and accelerates tumor progression by regulating the cell cycle in bladder cancer (30). MFN2 suppresses tumor proliferation and invasion via Wnt/β-catenin pathway in bladder cancer (31). In our study, we find that the current PRS acts as a prognostic marker and an independent risk factor in bladder cancer. Not only in bladder cancer, PRS can also predict the prognosis of triple-negative breast cancer (14). Similarly, PRS can predict the clinical outcomes of pancreatic adenocarcinoma patients (32).
Immunotherapy plays a vital role in the therapeutic strategy of bladder cancer cases (33,34). The results of our study show that bladder cancer with low PRS score portends a higher TMB score, higher PD-1 & CTLA4 immunophenoscore, lower TIDE score, lower immune escape score, lower ITH score and higher response rate, suggesting PRS as a potential indicator for immunotherapy benefit. TMB is an indicator in immunotherapy and higher TMB score is correlated with a better response to immunotherapy (35,36). Patients with low TIDE score have a less likelihood of immune escape (25). Therefore, bladder cancer patients with low PRS score are associated with a favorable immunotherapy benefit.
We then explore functional enrichment differences in different PRS score groups, which may clarify how PRS genes leading to the development of bladder cancer. The results show that bladder cancer patients with higher PRS score are correlated with higher gene set score involved in cancer hallmarks, including P53 pathway, NOTCH signaling, mTORC1 signaling, hypoxia, glycolysis, DNA repair, coagulation, and angiogenesis. Glycolysis plays a vital role in the tumorigenesis of bladder cancer (37). Angiogenesis is involved in tumor growth and metastasis in bladder cancer, suggesting as a prognostic marker and target (38). NOTCH signaling is also involved in tumor progression of cancer (39). Moreover, hypoxia-driven ITH is correlated with immune evasion in cancer (40).
There are some limitations in our study. The performance of PRS and other immunotherapy indicators, including TMB and immunophenoscore, in predicting immunotherapy effects, has not been compared. Moreover, the clinical applicability of PRS should be further verified using a larger real-world data.
Conclusions
The present study developed an ideal PRS for bladder cancer, which may be used as a predictor of prognosis, a risk classification system, and a therapy guide.
Acknowledgments
Funding: None.
Footnote
Reporting Checklist: The authors have completed the TRIPOD reporting checklist. Available at https://tau.amegroups.com/article/view/10.21037/tau-24-80/rc
Peer Review File: Available at https://tau.amegroups.com/article/view/10.21037/tau-24-80/prf
Conflicts of Interest: All authors have completed the ICMJE uniform disclosure form (available at https://tau.amegroups.com/article/view/10.21037/tau-24-80/coif). The authors have no conflicts of interest to declare.
Ethical Statement:
Open Access Statement: This is an Open Access article distributed in accordance with the Creative Commons Attribution-NonCommercial-NoDerivs 4.0 International License (CC BY-NC-ND 4.0), which permits the non-commercial replication and distribution of the article with the strict proviso that no changes or edits are made and the original work is properly cited (including links to both the formal publication through the relevant DOI and the license). See: https://creativecommons.org/licenses/by-nc-nd/4.0/.
References
- Sung H, Ferlay J, Siegel RL, et al. Global Cancer Statistics 2020: GLOBOCAN Estimates of Incidence and Mortality Worldwide for 36 Cancers in 185 Countries. CA Cancer J Clin 2021;71:209-49. [Crossref] [PubMed]
- Dyrskjøt L, Hansel DE, Efstathiou JA, et al. Bladder cancer. Nat Rev Dis Primers 2023;9:58. [Crossref] [PubMed]
- Patel VG, Oh WK, Galsky MD. Treatment of muscle-invasive and advanced bladder cancer in 2020. CA Cancer J Clin 2020;70:404-23. [Crossref] [PubMed]
- Grayson M. Bladder cancer. Nature 2017;551:S33. [Crossref] [PubMed]
- Holinstat M. Normal platelet function. Cancer Metastasis Rev 2017;36:195-8. [Crossref] [PubMed]
- Vinholt PJ. The role of platelets in bleeding in patients with thrombocytopenia and hematological disease. Clin Chem Lab Med 2019;57:1808-17. [Crossref] [PubMed]
- Buergy D, Wenz F, Groden C, et al. Tumor-platelet interaction in solid tumors. Int J Cancer 2012;130:2747-60. [Crossref] [PubMed]
- Wojtukiewicz MZ, Sierko E, Hempel D, et al. Platelets and cancer angiogenesis nexus. Cancer Metastasis Rev 2017;36:249-62. [Crossref] [PubMed]
- Bottsford-Miller J, Choi HJ, Dalton HJ, et al. Differential platelet levels affect response to taxane-based therapy in ovarian cancer. Clin Cancer Res 2015;21:602-10. [Crossref] [PubMed]
- Segal BH, Giridharan T, Suzuki S, et al. Neutrophil interactions with T cells, platelets, endothelial cells, and of course tumor cells. Immunol Rev 2023;314:13-35. [Crossref] [PubMed]
- Hinterleitner C, Strähle J, Malenke E, et al. Platelet PD-L1 reflects collective intratumoral PD-L1 expression and predicts immunotherapy response in non-small cell lung cancer. Nat Commun 2021;12:7005. [Crossref] [PubMed]
- Li Z, Ding Y, Liu J, et al. Depletion of tumor associated macrophages enhances local and systemic platelet-mediated anti-PD-1 delivery for post-surgery tumor recurrence treatment. Nat Commun 2022;13:1845. [Crossref] [PubMed]
- Tyagi T, Jain K, Yarovinsky TO, et al. Platelet-derived TLT-1 promotes tumor progression by suppressing CD8+ T cells. J Exp Med 2023;220:e20212218. [Crossref] [PubMed]
- Xie J, Zou Y, Ye F, et al. A Novel Platelet-Related Gene Signature for Predicting the Prognosis of Triple-Negative Breast Cancer. Front Cell Dev Biol 2022;9:795600. [Crossref] [PubMed]
- Zhao JG, Li YJ, Wu Y, et al. Revealing platelet-related subtypes and prognostic signature in pancreatic adenocarcinoma. BMC Med Genomics 2023;16:106. [Crossref] [PubMed]
- Peng C, Wang Y, Zhang H, et al. The platelet-related genes associated with the prognosis of HCC by regulating cycling T cell and prolif-TAMs. Heliyon 2024;10:e26798. [Crossref] [PubMed]
- Liu Z, Liu L, Weng S, et al. Machine learning-based integration develops an immune-derived lncRNA signature for improving outcomes in colorectal cancer. Nat Commun 2022;13:816. [Crossref] [PubMed]
- Li Z, Guo M, Lin W, et al. Machine Learning-Based Integration Develops a Macrophage-Related Index for Predicting Prognosis and Immunotherapy Response in Lung Adenocarcinoma. Arch Med Res 2023;54:102897. [Crossref] [PubMed]
- Yoshihara K, Shahmoradgoli M, Martínez E, et al. Inferring tumour purity and stromal and immune cell admixture from expression data. Nat Commun 2013;4:2612. [Crossref] [PubMed]
- Li T, Fu J, Zeng Z, et al. TIMER2.0 for analysis of tumor-infiltrating immune cells. Nucleic Acids Res 2020;48:W509-14. [Crossref] [PubMed]
- Song D, Wang X. DEPTH2: an mRNA-based algorithm to evaluate intratumor heterogeneity without reference to normal controls. J Transl Med 2022;20:150. [Crossref] [PubMed]
- Colwill KRenewable Protein Binder Working Group. Gräslund S. A roadmap to generate renewable protein binders to the human proteome. Nat Methods 2011;8:551-8. [Crossref] [PubMed]
- Lin A, Yan WH. HLA-G/ILTs Targeted Solid Cancer Immunotherapy: Opportunities and Challenges. Front Immunol 2021;12:698677. [Crossref] [PubMed]
- Palmeri M, Mehnert J, Silk AW, et al. Real-world application of tumor mutational burden-high (TMB-high) and microsatellite instability (MSI) confirms their utility as immunotherapy biomarkers. ESMO Open 2022;7:100336. [Crossref] [PubMed]
- Fu J, Li K, Zhang W, et al. Large-scale public data reuse to model immunotherapy response and resistance. Genome Med 2020;12:21. [Crossref] [PubMed]
- Lin A, Zhang J, Luo P. Crosstalk Between the MSI Status and Tumor Microenvironment in Colorectal Cancer. Front Immunol 2020;11:2039. [Crossref] [PubMed]
- Tan Y, Zhang T, Zhou L, et al. MiR-34b-3p Represses the Multidrug-Chemoresistance of Bladder Cancer Cells by Regulating the CCND2 and P2RY1 Genes. Med Sci Monit 2019;25:1323-35. [Crossref] [PubMed]
- Okajima E, Tomizawa M, Shimada K, et al. D2-40/podoplanin expression in cancer stroma by immunohistochemical staining is associated with poor prognosis in bladder cancer patients after radical cystectomy. Urol Oncol 2020;38:797.e7-797.e13. [Crossref] [PubMed]
- Eisenhardt A, Siffert W, Rosskopf D, et al. Association study of the G-protein beta3 subunit C825T polymorphism with disease progression in patients with bladder cancer. World J Urol 2005;23:279-86. [Crossref] [PubMed]
- Jiang Y, Zhu C, Huang H, et al. TUBA1C is a potential new prognostic biomarker and promotes bladder urothelial carcinoma progression by regulating the cell cycle. BMC Cancer 2023;23:716. [Crossref] [PubMed]
- Pang G, Xie Q, Yao J. Mitofusin 2 inhibits bladder cancer cell proliferation and invasion via the Wnt/β-catenin pathway. Oncol Lett 2019;18:2434-42. [Crossref] [PubMed]
- Yin Z, Zhao Y, Zhou W, et al. A 20-Gene Signature Predicting Survival in Patients with Clear Cell Renal Cell Carcinoma Based on Basement Membrane. J Oncol 2023;2023:1302278. [Crossref] [PubMed]
- Ruiz-Cordero R, Devine WP. Targeted Therapy and Checkpoint Immunotherapy in Lung Cancer. Surg Pathol Clin 2020;13:17-33. [Crossref] [PubMed]
- Ward Grados DF, Ahmadi H, Griffith TS, et al. Immunotherapy for Bladder Cancer: Latest Advances and Ongoing Clinical Trials. Immunol Invest 2022;51:2226-51. [Crossref] [PubMed]
- Liu L, Bai X, Wang J, et al. Combination of TMB and CNA Stratifies Prognostic and Predictive Responses to Immunotherapy Across Metastatic Cancer. Clin Cancer Res 2019;25:7413-23. [Crossref] [PubMed]
- Seo I, Lee HW, Byun SJ, et al. Neoadjuvant chemoradiation alters biomarkers of anticancer immunotherapy responses in locally advanced rectal cancer. J Immunother Cancer 2021;9:e001610. [Crossref] [PubMed]
- Wang JZ, Zhu W, Han J, et al. The role of the HIF-1α/ALYREF/PKM2 axis in glycolysis and tumorigenesis of bladder cancer. Cancer Commun (Lond) 2021;41:560-75. [Crossref] [PubMed]
- Streeter EH, Harris AL. Angiogenesis in bladder cancer--prognostic marker and target for future therapy. Surg Oncol 2002;11:85-100. [Crossref] [PubMed]
- Lim JS, Ibaseta A, Fischer MM, et al. Intratumoural heterogeneity generated by Notch signalling promotes small-cell lung cancer. Nature 2017;545:360-4. [Crossref] [PubMed]
- Terry S, Engelsen AST, Buart S, et al. Hypoxia-driven intratumor heterogeneity and immune evasion. Cancer Lett 2020;492:1-10. [Crossref] [PubMed]