Identification and expression of prognostic-related genes in kidney renal clear cell carcinoma and their possible regulatory mechanisms
Highlight box
Key findings
• Identification of multiple prognostic-related genes (PRGs) in kidney renal clear cell carcinoma (KIRC).
• Significant differences in immune cell infiltration between KIRC and adjacent normal tissues.
• Validation of PRG expression using quantitative real-time polymerase chain reaction (qRT-PCR).
What is known and what is new?
• Early diagnosis and prognosis prediction are critical for improving KIRC patient outcomes. Various factors influence KIRC prognosis.
• This study integrates data from multiple databases (Gene Expression Omnibus, The Cancer Genome Atlas, University of Alabama at Birmingham cancer data analysis Portal, Tumor Immune Estimation Resource) and uses R language and FunRich software to identify PRGs. It validates their expression and highlights the importance of the immune microenvironment and vascular endothelial growth factor (VEGF)/VEGF receptor signaling in KIRC prognosis.
What is the implication, and what should change now?
• These findings enhance the understanding of KIRC’s molecular mechanisms and the role of the immune microenvironment.
• Implementation of these identified PRGs in clinical settings for early diagnosis, prognosis prediction, and personalized treatment of KIRC patients.
Introduction
Kidney renal clear cell carcinoma (KIRC) is the most prevalent type of renal cell carcinoma (RCC), accounting for about 75% of all RCC cases (1,2). Metastasis is the leading cause of death in KIRC patients (3). However, the early clinical manifestation of KIRC is not obvious, making the early diagnosis of KIRC patients difficult. Surgical treatment can achieve good results in some KIRC patients; however, the 5-year survival rate of KIRC patients with metastasis remains very low (4,5). Additionally, the survival time of KIRC patients is significantly affected by intra-tumor heterogeneity (6). The prognosis of patients with KIRC, even those that have the same pathological grade, tumor node metastasis stage, and have undergone similar treatments (7,8), may be quite different, which shows the high heterogeneity of KIRC. Therefore, it is particularly important to find a suitable method to estimate the survival and prognosis of KIRC patients, and to diagnose KIRC early.
With the development of bioinformatics technology, a large number of genomics data are stored in public databases, such as The Cancer Genome Atlas (TCGA) and Gene Expression Omnibus (GEO), which can be used by researchers to integrate and study massive resources. In this study, we combined the GSE40439 and GSE46699 datasets to examine the immune infiltration of KIRC patients, and identify the differentially expressed genes (DEGs) of KIRC tissues compared with adjacent normal tissues. We also determined the main biological processes (BPs) and pathways involved in the DEGs by Gene Ontology (GO) and Kyoto Encyclopedia of Genes and Genomes (KEGG) analyses. In addition, several prognostic-related genes (PRGs) were identified by combining data from the GEO and TCGA databases. Finally, we verified our findings about some PRGs by using the University of Alabama at Birmingham cancer data analysis Portal (UALCAN) database and quantitative real-time polymerase chain reaction (qRT-PCR), and further speculated as to why PRGs affect the prognosis of KIRC using the Tumor Immune Estimation Resource (TIMER) database. We present this article in accordance with the STREGA reporting checklist (available at https://tau.amegroups.com/article/view/10.21037/tau-24-299/rc).
Methods
Acquisition and analysis of GEO data
We first downloaded the following two bulk-RNA sequencing datasets containing KIRC and adjacent normal tissue data from the GEO database: GSE40435 and GSE46699. The GSE40435 dataset included 101 KIRC tissues and 101 paracancerous normal tissues, and the GSE46699 dataset included 67 KIRC tissues and 63 paracancerous normal tissues. CIBERSORT was employed to analyze and compare the immune cell infiltration levels between the KIRC tissues and adjacent normal tissues in the two datasets (8). We also compared the DEGs of the KIRC and paracancerous tissues in the two datasets using the “limma” package (https://bioconductor.org/packages/release/bioc/html/limma.html). The DEGs were defined as genes with a P value <0.05, and an absolute log fold change (FC) >1. The genes with a P value <0.01 and an absolute logFC >3 were displayed in a volcano plot. Moreover, we identified the DEGs in both the GSE40435 and GSE46699 datasets using the “venn” (https://cran.r-project.org/web/packages/gplots/) and “randomcoloR” (https://cran.r-project.org/web/packages/randomcoloR/) packages. Meanwhile, the R packages “org.Hs.eg.db” (https://bioconductor.org/packages/release/data/annotation/html/org.Hs.eg.db.html) and “clusterProfiler” (https://bioconductor.org/packages/release/bioc/html/clusterProfiler.html) were used for the GO and KEGG analyses of the DEGs shared by the GSE40435 and GSE46699 datasets.
Data processing of KIRC and paracancerous normal tissues in TCGA database
We downloaded data from TCGA for 539 KIRC and adjacent normal tissue samples. Using Perl (strawberry-perl) software (http://strawberryperl.com/), we compiled the gene expression matrices and clinical data for these samples. We combined the gene expression matrix (using the DEGs from both GSE40435 and GSE46699) with the survival data of TCGA samples using “limma” package (https://bioconductor.org/packages/release/bioc/html/limma.html) in R language. A forest map of the PRGs of KIRC was constructed by a univariate Cox analysis by combining the common DEGs in the GSE40435 and GSE46699 datasets and the expression matrix and survival information of TCGA samples. We conducted a Pearson correlation analysis to construct a co-expression network by analyzing the PRGs identified in the forest maps and the transcription factors (TFs) associated with oncogenesis and progression (7,9,10).
Protein-protein interaction (PPI) network
FunRich (3.1.3exe) (http://www.funrich.org) is a powerful tool for analyzing human PPI networks and can show the signaling pathways in which genes or proteins are mainly enriched (11). We conducted a PPI analysis of the PRGs through FunRich, and identified the main signaling pathways involved in the PRGs.
UALCAN
The UALCAN database allows researchers to examine the relative gene expression levels of various cancer types and normal tissue samples using straightforward procedures. It also provides insights into relative gene expression in relation to tumor grades, cancer stages, or other clinicopathological characteristics (12). The UALCAN database was used to investigate the expression levels of the four genes with the highest and lowest hazard ratios (HRs) in both the KIRC and adjacent tissues, as well as their expression levels across different stages and tumor grades.
qRT-PCR
The expression levels of the PRGs in the KIRC and paracancerous tissues of 4 KIRC patients were compared by qRT-PCR. Table 1 presents the pathology and basic information of the 4 patients. All subjects gave their informed consent for inclusion in the study before they participated. The study was conducted in accordance with the Declaration of Helsinki (as revised in 2013), and the protocol was approved by the Ethics Committee of Changde Hospital, Xiangya School of Medicine, Central South University (The First People’s Hospital of Changde City) (approval number: 2024-061-01). RNA from KIRC and paracancerous tissues was extracted using the Vazyme extraction kit (RC112-01, Vazyme Biotech Co., Ltd., China). Reverse transcription was then performed on the extracted RNA using PrimeScript™ RT Reagent Kit (Perfect Real Time) (RR037A, Takara Bio Inc. Japan). Finally, qRT-PCR was conducted using the LightCycler 96 instrument (Roche Diagnostics, Switzerland) and ChamQ Universal SYBR qPCR Master Mix (Q711-02/03, Vazyme Biotech Co., Ltd., Nanjing, China). Each sample was analyzed in triplicate. The relative expression levels of the target genes in the KIRC and adjacent normal tissues were calculated using the 2−∆∆Ct method. The primer information for qRT-PCR is shown in Table 2.
Table 1
Patients | Sex | Age (years) | TNM stage | Pathological type |
---|---|---|---|---|
Patient 1 | Female | 74 | T1bN0M0 | KIRC |
Patient 2 | Female | 56 | T1bN0M0 | KIRC |
Patient 3 | Male | 67 | T1aN0M0 | KIRC |
Patient 4 | Male | 52 | T2bN0M0 | KIRC |
qRT-PCR, quantitative real-time polymerase chain reaction; TNM, tumor, node, metastasis; KIRC, kidney renal clear cell carcinoma.
Table 2
Gene name | Forward primer | Reverse primer |
---|---|---|
GAPDH | GTGGACCTGACCTGCCGTCTAG | GAGTGGGTGTCGCTGTTGAAGTC |
FCGR1B | TGGGTCAGCGTGTTCCAAG | GTCACTTCGCCCTGAGAGAC |
ISG20 | TCTACGACACGTCCACTGACA | CTGTTCTGGATGCTCTTGTGC |
PRC1 | ATCACCTTCGGGAAATATGGGA | TCTTTCTGACAGACGGATATGCT |
NUSAP1 | AGCCCATCAATAAGGGAGGG | ACCTGACACCCGTTTTAGCTG |
BPHL | TTCGGCACCTCGGTAACCT | GGACTGCGTGATCTCCCTCT |
PLCL1 | AAAGTCCGGCCAAATTCTCG | TTTCCGTGTTTTTCCCCAGTC |
CLIC5 | CTTGACCCCTGAAAAGTACCC | ACTTGGAAAAGATGTCGATGCC |
HIBCH | GCAATTTCGAGTGGCTACAGA | CCTTGGAGTCGTGGCAAGAA |
qPCR, quantitative real-time polymerase chain reaction.
TIMER
TIMER is a database that allows users to evaluate the immunE−related characteristics of specific tumors according to specific functional parameters (13). We evaluated the correlations between multiple PRGs and the infiltration of immune cells in KIRC using the TIMER database.
Statistical analysis
All statistical analyses were conducted using R programming (Version 4.0.2). Differential expression between KIRC tissues and adjacent normal tissues was analyzed using the “limma” package, with significant genes identified at a P value <0.05 and an absolute logFC >1. CIBERSORT was used to assess immune cell infiltration. Pearson correlation analysis was applied to explore relationships between PRGs and TFs. Gene Ontology and KEGG pathway analyses were performed using “clusterProfiler”. Survival outcomes based on PRG expression were evaluated using univariate Cox proportional hazards models. qRT-PCR reactions were conducted in triplicate, and gene expression was quantified using the 2−∆∆Ct method. Results with a P-value <0.05 were deemed significant.
Results
Analysis of the immune infiltration of KIRC
To investigate the expression characteristics of the cancer tissues of the KIRC patients, we first analyzed immune cell infiltration of the KIRC and adjacent normal tissues in the GSE40435 (Figure 1A) and GSE46699 (Figure 1B) datasets using the CIBERSORT algorithm. In the GSE40435 dataset, the KIRC tissues showed a significant decrease in naive B cells, memory resting cluster of differentiation (CD)4+ T cells, plasma cells, resting dendritic cells, monocytes, activated natural killer cells, eosinophils, and activated dendritic cells compared to the adjacent normal tissues (Figure 1C), but the proportion of memory activated CD4+T cells, gamma delta T cells, regulatory T cells, CD8+T cells, neutrophils, follicular helper T cells, M2 macrophages, and M1 macrophages was significantly higher in the KIRC tissues than the paracancerous tissue (Figure 1C). In the GSE46699 dataset, the proportion of memory B cells, plasma cells, memory resting CD4+T cells, regulatory T cells, resting dendritic cells, and activated mast cells was significantly lower in the KIRC tissues than the paracancerous tissue (Figure 1D), but the proportion of follicular helper T cells, M2 macrophages, gamma delta T cells, monocytes, M1 macrophages, M0 macrophages, neutrophils, and activated dendritic cells was significantly higher in the KIRC tissues than the paracancerous tissues (Figure 1D). According to these results, KIRC and normal tissues have a significantly different immune composition.
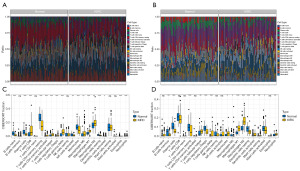
Identification of DEGs between the KIRC and paracancerous tissues
To further explore the characteristics of KIRC, we compared the DEGs between the KIRC and paracancerous tissues. As the volcano map shows (Figure 2A), in the GSE40435 dataset, 484 genes were significantly increased, and 599 genes were significantly decreased in the KIRC tissues compared with paracancerous tissues. While in the GSE46699 dataset, 511 genes were significantly increased and 558 genes were significantly decreased in the KIRC tissues compared with the paracancerous tissues (Figure 2B). Moreover, there were 261 upregulated genes and 290 downregulated genes in both the GSE40435 and GSE46699 datasets (Figure 2C,2D).
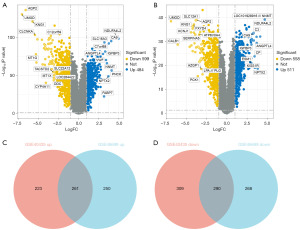
GO and KEGG analyses of common DEGs of GSE40435 and GSE46699
We conducted an analysis of the common DEGs in both the GSE40435 and GSE46699 datasets using the GO and KEGG pathways to examine the biological alterations in the KIRC tissues compared to the normal tissues. The results showed that the co-upregulated 261 DEGs were mainly involved in the regulation of immune-related BPs, such as lymphocyte proliferation, T cell activation, and leukocyte cell-cell adhesion (Figure 3A), and the main KEGG pathways included the nuclear factor Kappa-light-chain-enhancer of activated B cells (NF-Kappa B), phosphoinositide 3-kinase-protein kinase B signaling pathway (PI3K-Akt), and tumor necrosis factor (TNF) signaling pathways (Figure 3B). The co-downregulated 290 DEGs were mainly involved in kidney development and the BPs related to metabolism (Figure 3C), and the main KEGG pathways included the peroxisome proliferator-activated receptor (PPAR) signaling pathway, peroxisome, and other metabolic-related signaling pathways (Figure 3D).
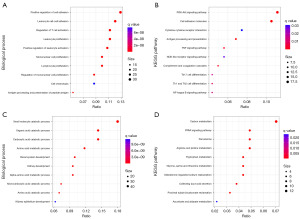
Identifying the PRGs in KIRC by combining TCGA and GEO data and constructing a regulatory network
After identifying the DEGs in both the GEO datasets, we combined the gene expression matrix with survival information from TCGA database to identify the genes that significantly affected the survival of the KIRC patients. Of the 261 DEGs upregulated in both the GSE40435 and GSE46699 datasets, we identified 60 PRGs of KIRC patients by combining TCGA-KIRC data (Figure 4A). Of the 290 DEGs downregulated by both the GSE40435 and GSE46699 datasets, we identified 53 PRGs by combining the TCGA-KIRC data (Figure 4B). Moreover, we combined TCGA-KIRC data with the DEGs in the GSE40435 and GSE46699 datasets to construct a regulatory network of the PRGs and TFs to explore the incidence and development of KIRC (Figure 4C). RARRES2 (Retinoic Acid Receptor Responder 2) expression was negatively correlated with ETS1 (E26 Transformation Specific 1) expression. ALDH6A1 (Aldehyde Dehydrogenase 6 Family Member A1), BPHL (Aldehyde Dehydrogenase 6 Family Member A1), PDZK1 (PDZ Domain Containing 1), and ACADM (Acyl-CoA Dehydrogenase Medium Chain) were negatively correlated with CEBPB (CCAAT Enhancer Binding Protein Beta) expression, but other PRGs were positively correlated with the expression of TFs (Table S1). Additionally, 113 PRGs were analyzed to examine the PPIs to further understand the possible reasons why the PRGs affected the prognosis of patients with KIRC. The results showed that there were complex interactions between these PRGs, and these PRGs were mainly related to the enrichment of the VEGF/VEGFR signaling network (Figure 4D).
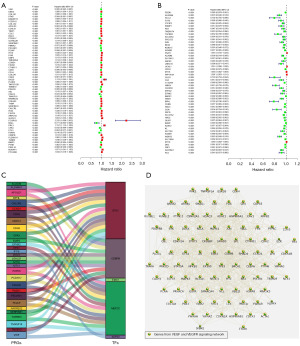
UALCAN database and qRT-PCR verified the expression of the PRGs in KIRC
To further confirm the reliability of the PRGs obtained by combining TCGA and GEO data, we used the UALCAN database to validate the expression of the four KIRC upregulated genes with the largest HRs (i.e., FCGR1B, ISG20, PRC1, and NUSAP1), and the four KIRC downregulated genes with the smallest HRs (i.e., BPHL, PLCL1, CLIC5, and HIBCH), and their effects on the prognosis of KIRC. The results showed that the expression levels of FCGR1B, ISG20, PRC1, and NUSAP1 were significantly higher in the KIRC tissues than the paracancerous tissues (Figure 5A-5D). The expression levels of FCGR1B, ISG20, PRC1, and NUSAP1 in the cancer tissues increased as KIRC stage (Figure 5E-5H), grade (Figure 5I-5L), and the occurrence of lymph node metastasis (Figure 5M-5P) increased. Moreover, the high expression of FCGR1B, ISG20, PRC1, and NUSAP1 significantly suppressed the survival time of patients with KIRC (Figure 5Q-5T). In addition, the expression levels of BPHL, PLCL1, CLIC5, and HIBCH were significantly lower in the KIRC tissues than the paracancerous tissues (Figure 6A-6D). Further, as KIRC stage (Figure 6E-6H), grade (Figure 6I-6L), and the occurrence of lymph node metastasis (Figure 6M-6P) increased, the expression levels of BPHL, PLCL1, CLIC5, and HIBCH decreased in the cancer tissues. Meanwhile, the KIRC patients with high expression levels of BPHL, PLCL1, CLIC5, and HIBCH had a significantly longer survival time compared to those with low expression (Figure 6Q-6T). The results of the UALCAN database were consistent with our findings, and the expression levels of the PRGs were further analyzed in terms of the different lymph node metastasis statuses, and grades and stages of KIRC. These results demonstrated the practicability and reliability of the PRGs identified by combining TCGA and GEO data. Finally, the qRT-PCR also verified that FCGR1B, ISG20, PRC1, and NUSAP1 were significantly more abundant in the KIRC tissues than the paracancerous tissues (Figure 7A-7D), and the expression levels of BPHL, PLCL1, CLIC5, and HIBCH were significantly lower in the KIRC tissues than the paracancerous tissues (Figure 7E-7H).
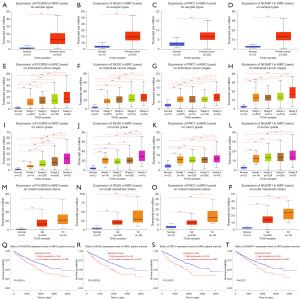
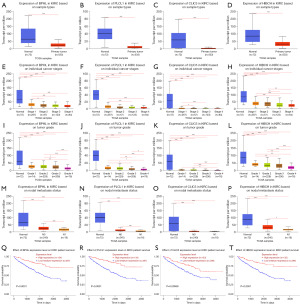
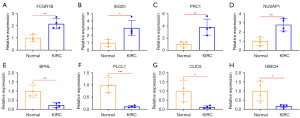
The relationship between the PRGs and immune cell infiltration in KIRC
To extend our understanding of how the PRGs influenced KIRC, we analyzed the relationship between the expression levels of the KIRC PRGs (i.e., FCGR1B, ISG20, PRC1, NUSAP1, BPHL, PLCL1, CLIC5 and HIBCH) and the infiltration levels of immune cells using the TIMER database. The expression levels of the four PRGs with the highest HRs (i.e., FCGR1B, ISG20, PRC1, and NUSAP1) were positively correlated with the infiltration levels of dendritic cells, CD8+T cells, B cells, macrophages, CD4+T cells, and neutrophils (Figure 8A-8D). Moreover, the expression levels of the four PRGs with the lowest HRs (i.e., BPHL, PLCL1, CLIC5, and HIBCH) were positively correlated with the infiltration levels of various immune cells (Figure 8E-8H), but the expression levels of the four genes with the highest HRs were more closely associated with the infiltration levels of immune cells. These results suggest that the effect of PRGs on the prognosis of patients with KIRC is closely related to changes in the tumor immune microenvironment.
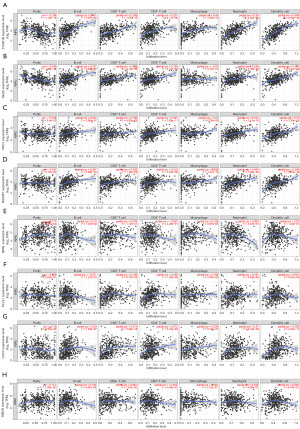
Discussion
The prognosis of different KIRC patients varies greatly. To achieve the best outcomes, the early diagnosis and treatment of KIRC is critical. Thus, it is particularly important to identify genes that can be used in both the early diagnosis and prognosis prediction of KIRC. This study sought to explore the histological characteristics of KIRC by integrating KIRC data from the GEO and TCGA databases, and using multiple gene expression levels to jointly diagnose KIRC early and estimate the prognosis of KIRC. Our findings could contribute to the early diagnosis and personalized treatment of KIRC patients.
First, we compared the immune cell infiltration of KIRC and adjacent normal tissues in both the GSE40435 and GSE46699 datasets. Our results revealed significant differences in the immune composition of the KIRC and adjacent normal tissues. Moreover, similarities and obvious differences were observed in the immune infiltration of the KIRC tissues between the GSE40435 and GSE46699 datasets, which indicated the commonality and heterogeneity of KIRC in terms of immune infiltration. The difference in immune infiltration is an important reason for prognosis differences in KIRC patients (14,15). Therefore, finding commonalities among the differences to evaluate the prognosis of patients with KIRC is very important.
We compared the DEGs between the KIRC and paracancerous tissues in the GSE40435 and GSE46699 datasets, and analyzed the common DEGs of the two datasets. The co-upregulated DEGs in the two GEO datasets mainly involved the TNF, NF-kappa B, and PI3K-Akt signaling pathways. A large number of studies have shown that the activation of the NF-kappa B pathway is related to the occurrence and progression of tumor metastasis (16). For example, the activation of the NF-kappa B pathway promotes the progression of RCC (17), the metastasis and chemotherapy resistance of intrahepatic bile duct carcinoma (18), and the angiogenesis and blood metastasis of bladder cancer (19). In addition, the activation of the TNF signaling pathway promotes KIRC proliferation (20), and the stimulation of the PI3K-Akt signaling pathway is associated with the formation of many tumors (21-23). Our results are consistent with the above reports, and comprehensively explain the formation and development of KIRC in many ways.
Further, we identified multiple PRGs of KIRC by combining TCGA and GEO data. There are extensive interactions among these PRGs, and they are mainly related to the VEGF/VEGFR signaling network. Research has revealed a direct relationship between VEGF signaling and lymphatic and blood vessel neovascularization, which has a potential correlation with the poor prognosis of KIRC patients (24,25). The continuous activation of the VEGF pathway causes the uncontrolled progress of KIRC (26). According to the above reports and the findings of our study, the enhancement or attenuation of VEGF signaling by PRGs affects the prognosis of KIRC patients.
Additionally, we utilized the UALCAN database to validate the expression of the four KIRC upregulated genes with the highest HRs—FCGR1B, ISG20, PRC1, and NUSAP1—as well as the four downregulated genes with the lowest HRs—BPHL, PLCL1, CLIC5, and HIBCH. Existing researches have reported on the impact of these genes in KIRC. For instance, a study by Xu et al. demonstrated that ISG20 promotes cell proliferation and metastasis by regulating the expression of MMP9/CCND1, and it may serve as a potential biomarker and therapeutic target in clear cell renal cell carcinoma (ccRCC) (27). Research by El-Hussieny et al. indicated that NUSAP1 is highly expressed in KIRC, and its expression level is associated with poor prognosis in KIRC patients (28). Pan et al. showed that PLCL1 inhibits tumor progression in renal cell carcinoma by regulating AMPK/mTOR-mediated autophagy (29). We also verified the expression of these PRGs in terms of different lymph node metastasis statuses, and grades and stages of KIRC. The factors affecting the 5-year survival rate of KIRC included lymph node metastasis status, tumor grade, and tumor stage (30-32). However, the expression of these PRGs obtained by combining TCGA and GEO data varied significantly with the stage, grade, and lymph node metastasis of KIRC, and the expression of these PRGs was significantly changed in the early stage of KIRC. This shows that the survival time of KIRC patients can be reliably evaluated using these PRGs, and these PRGs may contribute to the early diagnosis of KIRC patients.
Tumor development and treatment response are closely related to the tumor immune microenvironment (33,34). More and more studies have shown that the different percentage of immune cell populations in tumors and the heterogeneity of immune-related genes are important reasons for significant differences in prognosis (35-37). Therefore, we further explored the association between eight representative PRGs in KIRC and immune cell infiltration. These high-risk genes (FCGR1B, ISG20, PRC1, and NUSAP1) and low-risk genes (BPHL, PLCL1, CLIC5, and HIBCH) were significantly positively correlated with the infiltration of immune cells. This shows that immune cell infiltration is a double-edged sword, which may be beneficial or harmful to the prognosis of KIRC patients (38,39). The positive correlation between high-risk PRGs and immune cell infiltration indicates that these genes may regulate immunosuppressive cells or signals within the tumor microenvironment, thereby maintaining the state of immune escape. This contributes to the tumor’s evasion of immune surveillance and adversely impacts patient prognosis. Such high-risk PRGs might suggest potential resistance to treatments, particularly those aimed at modulating the immune environment, as the associated immunosuppressive milieu could compromise the efficacy of immunotherapeutic agents. Conversely, the positive correlation of low-risk PRGs with immune cell infiltration suggests that these PRGs may activate the tumor immune environment, enhancing the ability of immune cells to attack tumor cells. This indicates that low-risk PRGs could serve as potential biomarkers to identify patients more likely to respond to immunotherapies, thereby facilitating more personalized and effective treatment approaches. Our study linked the immune cell population to the expression characteristics of the KIRC PRGs, which is helpful in understanding the interaction between tumor-specific signatures characteristics and the immune microenvironment. Future research should therefore focus on conducting clinical trials that incorporate PRG profiling to evaluate its practical utility in predicting drug response and personalizing therapy regimens.
Our findings will assist in predicting the survival prognosis and early diagnosis of KIRC patients. However, our study also had certain limitations. The data were mainly obtained from public databases, and prospective cohort validation is lacking. However, our results were derived from the combined results of multiple databases, which increases the reliability of the results. Our study has considerable instructive implications for predicting the survival and early diagnosis of KIRC patients, and reveals multiple possible mechanisms influencing KIRC prognosis.
Conclusions
The degrees of immune cell infiltration in KIRC and paracancerous tissues differed significantly. The PRGs of KIRC played a significant role in the immune microenvironment and VEGF/VEGFR signaling. Compared with the paracancerous tissues, the expression of the representative PRGs we identified showed significant changes in early-stage, low-grade, and non-lymph node metastasis of KIRC. Our findings could assist in determining the prognosis of KIRC patients, selecting personalized treatments, and facilitating the early diagnosis of KIRC.
Acknowledgments
Funding: This work was supported by
Footnote
Reporting Checklist: The authors have completed the STREGA reporting checklist. Available at https://tau.amegroups.com/article/view/10.21037/tau-24-299/rc
Data Sharing Statement: Available at https://tau.amegroups.com/article/view/10.21037/tau-24-299/dss
Peer Review File: Available at https://tau.amegroups.com/article/view/10.21037/tau-24-299/prf
Conflicts of Interest: Both authors have completed the ICMJE uniform disclosure form (available at https://tau.amegroups.com/article/view/10.21037/tau-24-299/coif). The authors have no conflicts of interest to declare.
Ethical Statement: The authors are accountable for all aspects of the work in ensuring that questions related to the accuracy or integrity of any part of the work are appropriately investigated and resolved. All subjects gave their informed consent for inclusion in the study before they participated. The study was conducted in accordance with the Declaration of Helsinki (as revised in 2013), and the protocol was approved by the Ethics Committee of Changde Hospital, Xiangya School of Medicine, Central South University (The First People’s Hospital of Changde City) (approval number: 2024-061-01).
Open Access Statement: This is an Open Access article distributed in accordance with the Creative Commons Attribution-NonCommercial-NoDerivs 4.0 International License (CC BY-NC-ND 4.0), which permits the non-commercial replication and distribution of the article with the strict proviso that no changes or edits are made and the original work is properly cited (including links to both the formal publication through the relevant DOI and the license). See: https://creativecommons.org/licenses/by-nc-nd/4.0/.
References
- Linehan WM, Ricketts CJ. The Cancer Genome Atlas of renal cell carcinoma: findings and clinical implications. Nat Rev Urol 2019;16:539-52. [Crossref] [PubMed]
- Zou X, Guo Y, Mo Z. TLR3 serves as a novel diagnostic and prognostic biomarker and is closely correlated with immune microenvironment in three types of cancer. Front Genet 2022;13:905988. [Crossref] [PubMed]
- Li Y, Gong Y, Ning X, et al. Downregulation of CLDN7 due to promoter hypermethylation is associated with human clear cell renal cell carcinoma progression and poor prognosis. J Exp Clin Cancer Res 2018;37:276. [Crossref] [PubMed]
- Tornberg SV, Nisen H, Visapää H, et al. Outcome of surgery for patients with renal cell carcinoma and tumour thrombus in the era of modern targeted therapy. Scand J Urol 2016;50:380-6. [Crossref] [PubMed]
- Heidenreich A, Wilop S, Pinkawa M, et al. Surgical resection of urological tumor metastases following medical treatment. Dtsch Arztebl Int 2012;109:631-7. [Crossref] [PubMed]
- Tabata M, Sato Y, Kogure Y, et al. Inter- and intra-tumor heterogeneity of genetic and immune profiles in inherited renal cell carcinoma. Cell Rep 2023;42:112736. [Crossref] [PubMed]
- Wu X, Liang Y, Chen X, et al. Identification of Survival Risk and ImmunE−Related Characteristics of Kidney Renal Clear Cell Carcinoma. J Immunol Res 2022;2022:6149369. [Crossref] [PubMed]
- Wei JH, Feng ZH, Cao Y, et al. Predictive value of singlE−nucleotide polymorphism signature for recurrence in localised renal cell carcinoma: a retrospective analysis and multicentre validation study. Lancet Oncol 2019;20:591-600. [Crossref] [PubMed]
- Mei S, Qin Q, Wu Q, et al. Cistrome Data Browser: a data portal for ChIP-Seq and chromatin accessibility data in human and mouse. Nucleic Acids Res 2017;45:D658-62. [Crossref] [PubMed]
- Zheng R, Wan C, Mei S, et al. Cistrome Data Browser: expanded datasets and new tools for gene regulatory analysis. Nucleic Acids Res 2019;47:D729-35. [Crossref] [PubMed]
- Fonseka P, Pathan M, Chitti SV, et al. FunRich enables enrichment analysis of OMICs datasets. J Mol Biol 2021;433:166747. [Crossref] [PubMed]
- Chandrashekar DS, Bashel B, Balasubramanya SAH, et al. UALCAN: A Portal for Facilitating Tumor Subgroup Gene Expression and Survival Analyses. Neoplasia 2017;19:649-58. [Crossref] [PubMed]
- Li T, Fan J, Wang B, et al. TIMER: A Web Server for Comprehensive Analysis of Tumor-Infiltrating Immune Cells. Cancer Res 2017;77:e108-10. [Crossref] [PubMed]
- Hu J, Wang SG, Hou Y, et al. Multi-omic profiling of clear cell renal cell carcinoma identifies metabolic reprogramming associated with disease progression. Nat Genet 2024;56:442-57. [Crossref] [PubMed]
- Montemagno C, Jacquel A, Pandiani C, et al. Unveiling CXCR2 as a promising therapeutic target in renal cell carcinoma: exploring the immunotherapeutic paradigm shift through its inhibition by RCT001. J Exp Clin Cancer Res 2024;43:86. [Crossref] [PubMed]
- Seaton G, Smith H, Brancale A, et al. Multifaceted roles for BCL3 in cancer: a proto-oncogene comes of age. Mol Cancer 2024;23:7. [Crossref] [PubMed]
- Oya M, Takayanagi A, Horiguchi A, et al. Increased nuclear factor-kappa B activation is related to the tumor development of renal cell carcinoma. Carcinogenesis 2003;24:377-84. [Crossref] [PubMed]
- Kang FP, Chen ZW, Liao CY, et al. Escherichia coli-Induced cGLIS3-Mediated Stress Granules Activate the NF-κB Pathway to Promote Intrahepatic Cholangiocarcinoma Progression. Adv Sci (Weinh) 2024;11:e2306174. [Crossref] [PubMed]
- Xie J, Zhang H, Wang K, et al. M6A-mediated-upregulation of lncRNA BLACAT3 promotes bladder cancer angiogenesis and hematogenous metastasis through YBX3 nuclear shuttling and enhancing NCF2 transcription. Oncogene 2023;42:2956-70. [Crossref] [PubMed]
- Liu W, Wang H, Jian C, et al. The RNF26/CBX7 axis modulates the TNF pathway to promote cell proliferation and regulate sensitivity to TKIs in ccRCC. Int J Biol Sci 2022;18:2132-45. [Crossref] [PubMed]
- Li D, Wang J, Tuo Z, et al. Natural products and derivatives in renal, urothelial and testicular cancers: Targeting signaling pathways and therapeutic potential. Phytomedicine 2024;127:155503. [Crossref] [PubMed]
- Du L, Dou KK, Liang NN, et al. MiR-194-5p suppresses the Warburg effect in ovarian cancer cells through the IGF1R/PI3K/AKT axis. Biocell 2023;47:547-54. [Crossref]
- Qian CN, Furge KA, Knol J, et al. Activation of the PI3K/AKT pathway induces urothelial carcinoma of the renal pelvis: identification in human tumors and confirmation in animal models. Cancer Res 2009;69:8256-64. [Crossref] [PubMed]
- Dorević G, Matusan-Ilijas K, Babarović E, et al. Hypoxia inducible factor-1alpha correlates with vascular endothelial growth factor A and C indicating worse prognosis in clear cell renal cell carcinoma. J Exp Clin Cancer Res 2009;28:40. [Crossref] [PubMed]
- Zhang J, Lu T, Lu S, et al. SinglE−cell analysis of multiple cancer types reveals differences in endothelial cells between tumors and normal tissues. Comput Struct Biotechnol J 2022;21:665-76. [Crossref] [PubMed]
- Ruan H, Li S, Bao L, et al. Correction: Enhanced YB1/EphA2 axis signaling promotes acquired resistance to sunitinib and metastatic potential in renal cell carcinoma. Oncogene 2023;42:165-7. [Crossref] [PubMed]
- Xu T, Ruan H, Gao S, et al. ISG20 serves as a potential biomarker and drives tumor progression in clear cell renal cell carcinoma. Aging (Albany NY) 2020;12:1808-27. [Crossref] [PubMed]
- El-Hussieny M, Thabet DM, Tawfik HM, et al. The Overexpression of NUSAP1 and GTSE1 Could Predict An Unfavourable Prognosis and Shorter Disease Free Survival in ccRenal Cell Carcinoma. Asian Pac J Cancer Prev 2024;25:2551-9. [Crossref] [PubMed]
- Pan Z, Huang J, Song H, et al. PLCL1 suppresses tumour progression by regulating AMPK/mTOR-mediated autophagy in renal cell carcinoma. Aging (Albany NY) 2023;15:10407-27. [Crossref] [PubMed]
- Lam JS, Shvarts O, Said JW, et al. Clinicopathologic and molecular correlations of necrosis in the primary tumor of patients with renal cell carcinoma. Cancer 2005;103:2517-25. [Crossref] [PubMed]
- Dall'Oglio MF, Ribeiro-Filho LA, Antunes AA, et al. Microvascular tumor invasion, tumor size and Fuhrman grade: a pathological triad for prognostic evaluation of renal cell carcinoma. J Urol 2007;178:425-8; discussion 428. [Crossref] [PubMed]
- Lam JS, Klatte T, Patard JJ, et al. Prognostic relevance of tumour size in T3a renal cell carcinoma: a multicentre experience. Eur Urol 2007;52:155-62. [Crossref] [PubMed]
- Quail DF, Joyce JA. Microenvironmental regulation of tumor progression and metastasis. Nat Med 2013;19:1423-37. [Crossref] [PubMed]
- Fridman WH, Zitvogel L, Sautès-Fridman C, et al. The immune contexture in cancer prognosis and treatment. Nat Rev Clin Oncol 2017;14:717-34. [Crossref] [PubMed]
- Zeng D, Li M, Zhou R, et al. Tumor Microenvironment Characterization in Gastric Cancer Identifies Prognostic and Immunotherapeutically Relevant Gene Signatures. Cancer Immunol Res 2019;7:737-50. [Crossref] [PubMed]
- Zhang D, He W, Wu C, et al. Scoring System for Tumor-Infiltrating Lymphocytes and Its Prognostic Value for Gastric Cancer. Front Immunol 2019;10:71. [Crossref] [PubMed]
- Wan B, Liu B, Huang Y, et al. Prognostic value of immunE−related genes in clear cell renal cell carcinoma. Aging (Albany NY) 2019;11:11474-89. [Crossref] [PubMed]
- Zhang S, Zhang E, Long J, et al. Immune infiltration in renal cell carcinoma. Cancer Sci 2019;110:1564-72. [Crossref] [PubMed]
- Zuo S, Wei M, Wang S, et al. Pan-Cancer Analysis of Immune Cell Infiltration Identifies a Prognostic ImmunE−Cell Characteristic Score (ICCS) in Lung Adenocarcinoma. Front Immunol 2020;11:1218. [Crossref] [PubMed]
(English Language Editor: L. Huleatt)