Ferroptosis-related gene signature predicts prognosis and immune microenvironment in prostate cancer
Highlight box
Key findings
• A prognostic signature of eight ferroptosis-related genes (FRGs) that may accurately predict prostate cancer (PCa) patient outcomes was constructed and validated. A ferroptosis signature may contribute to anti-tumor immunity and serve as therapeutic targets in PCa.
What is known, and what is new?
• Ferroptosis is a form of cell death that differs from normal apoptosis in that it is caused by the accumulation of intracellular iron and lipid reactive oxygen species. Following the in-depth study of ferroptosis, some researchers have found that ferroptosis is closely related to the occurrence and development of cancer.
• In this study, we identified eight FRGs associated with PCa prognosis that potentially predict the clinical prognosis of PCa patients. The evaluation value of the model in terms of patient prognosis and tumor immune infiltration was analyzed using The Cancer Genome Atlas database and validated in the Gene Expression Omnibus database. Next, a further functional enrichment analysis was conducted to examine the relationship between ferroptosis and the immune microenvironment in PCa to provide a theoretical reference for PCa treatment strategies.
What is the implication, and what should change now?
• We constructed a PCa prognosis prediction model of eight FRGs through data analysis and validated it using both internal and external databases. This model may serve as an independent predictor of disease-free survival in PCa patients. We also found an association between this model and the immune microenvironment of PCa patients. Our findings provide a potential new direction for the immunotherapy of PCa patients.
Introduction
Prostate cancer (PCa), accounts for 26% of all male cancers, is the most common malignancy in men, and is the second leading cause of cancer-related death (after lung cancer) (1). Early localized PCa can be treated with surgery or radiation therapy with curative intent. If disease recurs after initial surgery or radiation therapy, androgen deprivation therapy (chemical castration or surgical castration) can be used (2). However, most patients eventually become resistant to this treatment and develop castration-resistant PCa, for which chemotherapy is one of the treatment options (3). The high recurrence rate and drug resistance of PCa have always been clinical challenges (4). Therefore, the identification of predictive markers of PCa is of great significance in monitoring PCa recurrence and establishing new therapeutic targets.
Ferroptosis, a form of cell death, differs from normal apoptosis in that it is caused by the accumulation of intracellular iron and lipid reactive oxygen species (5). Following the in-depth study of ferroptosis, some researchers have found that ferroptosis is closely related to the occurrence and development of cancer (6). A study has shown that activating ferroptosis can inhibit tumor growth, which provides a new strategy for the treatment of cancer (7). Targeting GPX4 or using GPX4 inhibitors can induce ferroptosis to treat cancer (8). Some small-molecule inducers and nanomaterial inducers of ferroptosis will be widely used in the future, such as System Xc-Inhibition, FIN56, and FINO2 (9).
PCa is a disease associated with ferroptosis (10,11). Liu et al. reported that cell migration-inducing protein (CEMIP) promotes extracellular matrix separation by inhibiting ferroptosis, thereby favoring PCa cell survival (12). Zhao et al. found that ATF6α promotes PCa progression by enhancing PLA2G4A-mediated arachidonic acid metabolism, which protects tumor cells from ferroptosis (13). However, current research on the relationship between PCa and ferroptosis is limited, and the relationship between ferroptosis and the prognosis of patients with PCa is unclear. Therefore, novel biomarkers associated with ferroptosis in PCa need to be identified. The identification of novel ferroptosis-related biomarkers is of significance in predicting the prognosis of PCa patients.
In recent years, immunotherapy (in which a patient’s immune system is targeted to induce an anti-tumor response) has been a rapidly evolving treatment option for many cancer types (14). Recent research in tumor immunotherapy has focused on the concept of immune checkpoints, a collection of molecules that act to limit an ongoing immune response (15,16). The most common approach in cancer immunotherapy is the use of antibodies to block the programmed death-ligand 1 (PD-L1)/programmed cell death-1 (PD-1) immune checkpoint (17,18). The antibody blockade of PD-L1 or PD-1 has been shown to significantly increase the survival of patients with a variety of cancers, including lung and bladder cancers (19). Unfortunately, immune checkpoint inhibition has only been successful in a small proportion of prostate cancer patients (20-23). Thus, a biomarker of immunotherapy sensitivity is an unmet medical need in the prostate cancer field. Currently, there are few studies on the relationship between ferroptosis and anti-tumor immunity (24,25). Exploring the relationship between ferroptosis and the tumor immune microenvironment will help us better understand the pathogenesis of PCa and provide additional potential therapeutic strategies for PCa. There is an urgent need to identify tumor immune microenvironment-related biomarkers beyond immune checkpoint blockade.
In this study, we identified eight ferroptosis-related genes (FRGs) associated with PCa prognosis. These FRGs can potentially predict the clinical prognosis of PCa patients. The evaluation value of the model in terms of patient prognosis and tumor immune infiltration was analyzed using The Cancer Genome Atlas (TCGA) database and validated in the Gene Expression Omnibus (GEO) database. Next, we conducted a functional enrichment analysis to examine the relationship between ferroptosis and the immune microenvironment in PCa. We present this article in accordance with the MDAR and TRIPOD reporting checklists (available at https://tau.amegroups.com/article/view/10.21037/tau-24-415/rc).
Methods
The study was conducted in accordance with the Declaration of Helsinki (as revised in 2013). The study was approved by the Ethical Committee Review Board of Lianshui People’s Hospital of Kangda College Affiliated to Nanjing Medical University (Huai’an, Jiangsu, China) (No. 20240424-02) and informed consent was taken from all the patients.
Patients and datasets
The RNA-sequencing data and corresponding clinical information of 478 PCa patients were downloaded from TCGA database (https://www.cancer.gov/tcga). The RNA-sequencing data and clinical information of an additional 90 PCa tumor samples were obtained from the GEO database (GSE70769). A total of 259 FRGs were downloaded from the Ferroptosis Database (FerrDb; http://www.zhounan.org/ferrdb/). Data from TCGA are publicly available, and the current study followed TCGA’s data access policy and publication guidelines.
Identification of FRGs
We downloaded FRGs from the FerrDb, the first database of ferroptosis regulators and markers. After sorting out the downloaded genes, we identified 259 FRGs. The 259 genes were divided into three groups of genes that promote ferroptosis, genes that inhibit ferroptosis, and ferroptosis marker genes.
Construction and validation of an eight-FRG prognostic signature
First, a univariate Cox regression analysis was performed of the 259 FRGs from the FerrDb, and 38 FRGs associated with PCa prognosis were identified. Subsequently, a multivariate Cox regression analysis was used to identify 19 FRGs associated with PCa prognosis. Of the 19 genes, eight were ferroptosis suppressor genes, and these eight genes were used to construct the eight-FRG prognostic signature. The log-rank test was used to analyze the relationship between the expression of these eight genes and the prognosis of PCa patients. The ferroptosis risk score was calculated as follows: ferroptosis risk score = e sum (each gene’s expression × corresponding regression coefficient). The 478 PCa patients in TCGA database were divided into high- and low-risk groups according to the median ferroptosis risk score. The overall survival (OS) and disease-free survival (DFS) of the high- and low-risk groups of PCa patients were analyzed by Kaplan-Meier analysis.
Functional enrichment analysis
A gene set enrichment analysis (GSEA), Gene Ontology (GO) enrichment analysis, and Kyoto Encyclopedia of Genes and Genomes (KEGG) pathway enrichment analysis were used to identify the biological processes and molecular functions associated with the FRGs between the high- and low-risk groups of PCa patients.
Immunity analysis
ESTIMATE was used to explore the correlation between the ferroptosis risk score and tumor microenvironment. CIBERSORT was used to identify the immune infiltrating cells between the high- and low-risk groups of PCa patients.
Cell culture
BPH-1 and LNCaP cell lines were purchased from the American Type Culture Collection (ATCC; Manassas, VA, USA). The cell lines were maintained in Roswell Park Memorial Institute-1640 medium (Solarbio, Beijing, China) supplemented with 10% fetal bovine serum (Solarbio), 100 ng/mL streptomycin, and 100 U/mL penicillin (Gibco, California, USA). All the cell lines were incubated under the condition of 37 ℃ with 5% carbon dioxide. The LNCaP cells were treated with ferroptosis inhibitor ferrostatin-1 (2 µM, 8 h).
RNA analysis and qRT-PCR
RNA was isolated using Trizol reagent (Invitrogen, California, USA) according to the manufacturer’s instructions. The complementary DNAs (cDNAs) were obtained using a reverse transcription kit (Roche, Basel, Switzerland). The resulting cDNA was analyzed by polymerase chain reaction (PCR) using the Applied Biosystems 7900 Real-Time PCR System (Thermo Scientific, Waltham, USA) and SYBR Green PCR Master Mix (Roche) according to the manufacturers’ instructions. Glyceraldehyde 3-phosphate dehydrogenase (GAPDH) was used as an internal control. The primer sequences are listed in Table S1.
Western blot
The cells were washed with cold phosphate-buffered saline (PBS) and lysed with radioimmunoprecipitation assay (RIPA) buffer. Equal amounts of protein samples were separated by 10% sodium dodecyl sulfate/polyacrylamide gel electrophoresis (SDS/PAGE) gel and transferred using polyvinylidene difluoride (PVDF) membranes. The membranes were incubated in 5% fat-free milk for 1 hour at room temperature, and then incubated with specific primary antibodies overnight at 4 ℃. The next day, the membranes were incubated with secondary antibody at room temperature for 1 hour. After Tris Buffered Saline with Tween 20 (TBST) washing, the membranes were prepared for exposure, and enhanced chemiluminescence reagent (Thermo Scientific) was used to visualize bands. The primary antibodies are listed in Table S2.
Immunohistochemistry (IHC)
All the tissue samples were obtained from Lianshui People’s Hospital of Kangda College Affiliated to Nanjing Medical University. We collected 16 tissue biopsy samples in total, 6 from benign prostatic hyperplasia (BPH) patients and 10 from PCa patients. Tissue specimens of BPH and prostate tumors were formalin fixed and paraffin embedded. Serial sections (4 µm) were prepared on charged glass slides. After deparaffinization and rehydration, non-specific bindings were blocked with antigen-repair solution. Next, the sections were incubated with primary antibodies at 4 ℃ overnight and exposed to second antibodies at 37 ℃ for 1 h. Standard 3,3'-diaminobenzidine (DAB) staining was carried out to detect IHC targets. Images were captured via light microscopy. The primary antibodies are listed in Table S2.
Statistical analysis
All data were analyzed using R software (version 4.0.2). The log-rank test was used to examine the relationship between the expression level of each gene and the prognosis of PCa patients. A prognostic signature for predicting PCa prognosis comprising eight FRGs was constructed using univariate and multivariate Cox regression analyses. The OS and DFS of the high- and low-risk groups were analyzed by Kaplan-Meier analysis. P values less than 0.05 were considered statistically significant.
Results
The flow chart is shown in Figure 1. We included a total of 478 PCa patients from TCGA database as a derivative cohort, and 90 PCa patients from the GEO TCGA as a validation cohort. The baseline clinical characteristics of the PCa patients in this study are shown in Table 1.
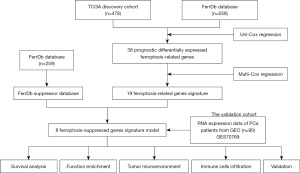
Table 1
Characteristics | TCGA (n=478) | GSE70769 (n=90) |
---|---|---|
Age (years) | ||
≤60 | 213 | NA |
>60 | 265 | NA |
ISUP | ||
≤2 | 182 | 56 |
>2 | 296 | 34 |
Laterality | ||
Bilateral | 422 | NA |
Left | 19 | NA |
Right | 37 | NA |
T stage | ||
T1–T2 | 184 | 47 |
T3–T4 | 294 | 43 |
BCR | ||
Yes | 59 | 45 |
No | 419 | 45 |
DFS | ||
Yes | 69 | NA |
No | 409 | NA |
TCGA, The Cancer Genome Atlas; GEO, Gene Expression Omnibus; ISUP, International Society of Urological Pathology; BCR, biochemical recurrence; DFS, disease-free survival; NA, not applicable.
Construction of an eight-FRG prognostic signature
We downloaded the RNA-sequencing data and corresponding clinical information of 478 PCa patients from TCGA database. In total, 259 FRGs were identified from the FerrDb. The results of the univariate Cox regression analysis showed that 38 genes were associated with the prognosis of PCa patients. By performing a multivariate Cox regression analysis, we identified 19 FRGs, of which eight were ferroptosis suppressor genes. Ultimately, we found that the eight ferroptosis suppressor genes were strongly associated with the prognosis of PCa patients by constructing a prognostic signature.
A survival analysis was performed to examine the expression of the eight ferroptosis suppressor genes, and we found that, with the exception of MT1G, the high expression of the other seven genes was associated with a poor prognosis in PCa patients (Figure 2A-2H). The ferroptosis risk score was calculated as follows: ferroptosis risk score = e sum (each gene’s expression × corresponding regression coefficient). Patients were divided into the high-risk group (n=239) and low-risk group (n=239) according to the median ferroptosis risk score (Figure 3A). A Kaplan-Meier analysis was used to compare DFS between the high- and low-risk groups (Figure 3B). The patients in the high-risk group were significantly more likely to die than those in the low-risk group. The Kaplan-Meier curves consistently showed that patients in the high-risk group had significantly lower DFS than those in the low-risk group. A time-dependent receiver operating characteristic (ROC) curve analysis was performed with the “timeROC” R package to assess the accuracy of the predictions of the eight-FRG prognostic signature. The areas under the curve (AUCs) in TCGA cohort reached 0.85 at 1 year, 0.82 at 3 years, and 0.76 at 5 years (Figure 3C).
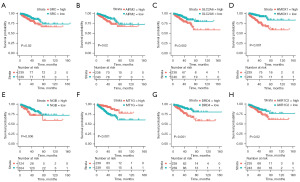
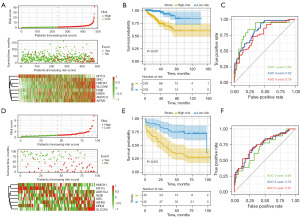
Validation of the eight-FRG prognostic signature in the external GEO database
To validate our prognostic signature, we used the GEO database as the validation cohort. The ferroptosis risk score of each PCa patient in the validation cohort was calculated using the same formula as that used in TCGA cohort, and the GEO cohort patients were divided into the high-risk group (n=45) and low-risk group (n=45) using the same cut-off value (Figure 3D). A Kaplan-Meier analysis and time-dependent ROC curve analysis were then conducted. The Kaplan-Meier analysis results showed that consistent with TCGA cohort, in the GEO cohort, patients in the high-risk group were more likely to die earlier (Figure 3E). The AUCs in the GEO cohort reached 0.69 at 1 year, 0.74 at 3 years, and 0.75 at 5 years (Figure 3F).
Independent prognostic value of the eight-FRG prognostic signature
To determine whether the ferroptosis risk score could serve as an independent prognostic predictor for PCa patients, we performed a univariate Cox regression analysis and a multivariate Cox regression analysis. The univariate Cox regression analysis showed that the ferroptosis risk score of TCGA cohort was significantly associated with DFS [hazard ratio (HR) =4.30, 95% confidence interval (CI): 2.14–8.66, P<0.001] (Figure 4A). After adjusting for the other confounding factors, the ferroptosis risk score remained an independent predictor of DFS in the multivariate Cox regression analysis (HR =5.47, 95% CI: 2.77–10.8, P<0.001) (Figure 4B). We also generated a heatmap showing the relationship between the prognostic features of the FRGs and clinicopathological manifestations (Figure S1). To further predict the prognosis of PCa patients, we constructed a nomogram containing clinicopathological variables and ferroptosis risk scores that could predict the prognosis of patients with PCa at 1, 3, and 5 years (Figure S2).
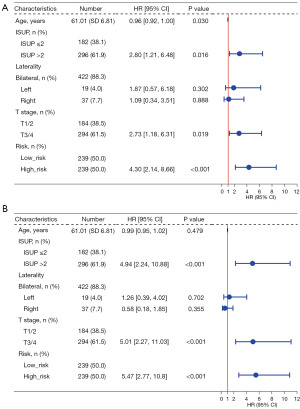
Subgroup survival analysis
The results of the subgroup survival analysis showed that there was significant difference between the prognosis of the high- and low-risk groups of PCa patients in terms of age, Gleason score, T-stage III–IV, and International Society of Urological Pathology (ISUP) stage (Figure 5A-5F). However, the eight-FRG prognostic signature was particularly applicable to the T-stage III–IV PCa patients. Among the T-stage III–IV PCa patients, high-ferroptosis risk scores were associated with significantly worse biochemical recurrence-free survival than low-ferroptosis risk scores. However, in the stage I–II PCa patients, there was no statistically significant difference in biochemical recurrence-free survival between the low- and high-risk groups (Figure 5G,5H).
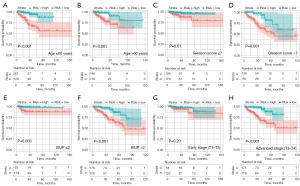
Functional analysis of the biological pathways associated with FRGs between the high- and low-risk groups of PCa patients
We performed a GSEA, GO enrichment analysis, and KEGG pathway enrichment analysis to explore the biological functions and signal transduction pathways associated with the FRGs between the high- and low-risk groups of PCa patients. Interestingly, the results of the GSEA, GO enrichment analysis, and KEGG pathway enrichment analysis all showed that the FRGs between the high- and low-risk groups were significantly enriched in many immune-related biological processes. The GSEA results showed that the upregulated genes in the high-risk group were mainly enriched in allograft rejection, epithelial-mesenchymal transition, and the inflammatory response (Figure 6A,6B). The GO enrichment analysis results showed that the upregulated genes in the high-risk PCa patients were mainly enriched in cytokine activity, cytokine receptor binding, and immune receptor activity (Figure 6C,6D). The KEGG pathway enrichment analysis results showed that the upregulated genes in the high-risk PCa patients were mainly enriched in the cytokine receptor interaction, primary immunodeficiency, and T cell receptor signaling pathway (Figure 6E,6F). The above results suggested that the significant involvement of immune-related pathways in the progression of PCa among high-risk patients.
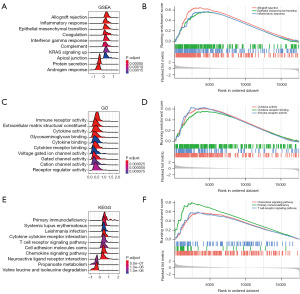
Association between the ferroptosis risk score and the tumor microenvironment and immune infiltrating cells
Through the pathway enrichment analysis, we found that the FRGs between the high- and the low-risk groups of PCa patients were mainly enriched in immune-related pathways. The CIBERSORT results showed that eight FRGs were associated with antigen-presenting cells (Figure 7A-7H). To further examine the correlation between the ferroptosis risk score of the FRG prognostic signature and the tumor microenvironment and immune status, the ESTIMATE algorithm was used. CIBERSORT was used to compare the different proportions of inferred immune infiltrating cells between the high- and the low-risk groups of PCa patients. The ESTIMATE results showed that the tumor purity of the high-risk group was lower than that of the low-risk group, and tumor purity was negatively correlated with the ferroptosis risk score (Figure 8A,8B). However, the ESTIMATE score, the Immune score, and the Stromal score of the high-risk group of PCa patients were higher than those of the low-risk group, and the ESTIMATE score, the Immune score, and the Stromal score were positively correlated with the ferroptosis risk score (Figure 8C-8H). The CIBERSORT analysis results showed that the proportion of inferred tumor-infiltrating immune cells in the high- and low-risk groups of PCa patients differed significantly. The correlation matrix of the proportions of all the tumor-infiltrating immune cells is shown in Figure S3. We used a violin plot to show the differences in the immune infiltrating cells between the high- and low-risk groups of PCa patients. The results showed that the high-risk group of PCa patients had lower proportions of tumor-infiltrating mast cells and plasma cells than the low-risk group, and the degree of mast-cell and plasma-cell infiltration was inversely correlated with the ferroptosis risk score (Figure 9A-9D). However, the proportion of inferred tumor-infiltrating regulatory T cells (Tregs) was higher in the high-risk group of PCa patients than the low-risk group, and the degree of Treg infiltration was positively correlated with the ferroptosis risk score (Figure 9E,9F). Subsequently, we also compared the expression of common immune checkpoint genes between the high- and the low-risk groups of PCa patients, and the results showed that the expression of the common immune checkpoint genes, such as PD1, PDL1, CTLA4, TIGIT, LAG3, TIM3, and BTLA, were higher in the high-risk group of PCa patients than the low-risk group (Figure S3C,S3D).
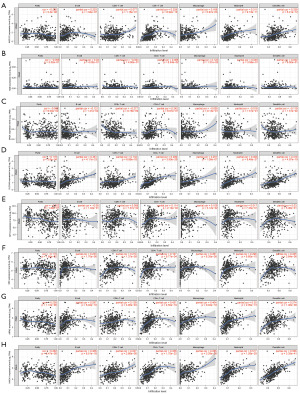
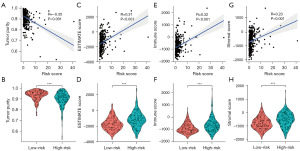
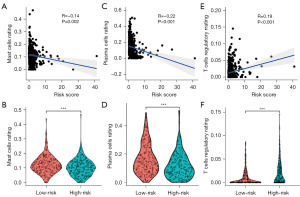
Validation of mRNA and protein expression levels of eight FRGs in BPH and PCa
The quantitative real-time polymerase chain reaction (qRT-PCR) results showed that the messenger RNA (mRNA) expression levels of DRD4, SRC, AKR1C2, and AIFM2 in the LNCaP cells were significantly increased compared with those in the BPH-1 cells (Figure 10A); meanwhile, the protein expression levels of DRD4, SRC, AKR1C2, and AIFM2 in the LNCaP cells were significantly increased compared with those in the BPH-1 cells (Figure 10B). Moreover, we obtained a total of 16 tissue biopsy specimens, comprising 6 from patients with BPH and 10 from patients with PCa. Detailed patient characteristics are presented in Table S3. The IHC results suggested that the protein expression levels of DRD4, SRC, AKR1C2, and AIFM2 in the PCa tissues were significantly increased compared with those in the BPH tissues (Figure 10C). Therefore, we found that four core prognostic genes (DRD4, SRC, AKR1C2, and AIFM2) were significantly increased in the PCa cells.
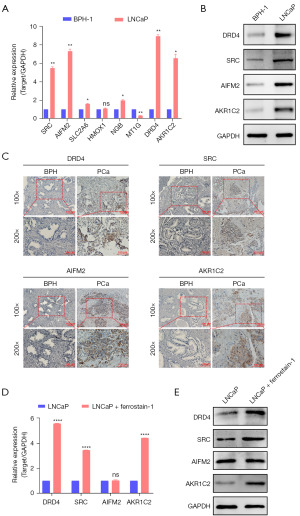
Effects of ferroptosis inhibitor ferrostatin-1 on the expression levels of the four core prognostic genes in PCa cell lines in vitro
The PCa cell line, LNCaP, was then treated with ferroptosis inhibitor, ferrostatin-1 to explore the role of the four core prognostic genes that were upregulated in PCa. QRT-PCR and Western blot were used to investigate the expression of the four core prognostic genes in the LNCaP cells after they had been treated with 2 µM of ferrostatin-1 for 8 h. The qRT-PCR and Western blot results suggested that the expression levels of DRD4, SRC, and AKR1C2 were increased in the LNCaP cells treated with ferrostatin-1. However, the expression level of AIFM2 was not significantly increased after ferrostatin-1 treatment (Figure 10D,10E).
Discussion
Ferroptosis plays an important role in a variety of cancers, such as breast, bladder, colon and prostate cancer (26-28). Ye et al. revealed that FBW7 can promote the ferroptosis process of pancreatic cancer cells by inhibiting the progression of pancreatic cancer, providing a new treatment target for pancreatic cancer (29). Tang et al. found that curcumin enhanced the therapeutic effect by inducing ferroptosis and activating autophagy in non-small cell lung cancer (30). Xu et al. demonstrated that targeting SLC7A11 specifically inhibited the progression of colorectal cancer stem cells by inducing ferroptosis (31).
PCa recurrence and metastatic disease are causes of morbidity and mortality, and has been associated with ferroptosis (32). However, the association between ferroptosis and DFS in patients with PCa remains largely unknown. Therefore, the further exploration of functional FRGs as prognostic indicators of PCa is necessary.
In this study, we developed an eight-gene ferroptosis signature associated with PCa prognosis, and further decoded the relationship between the expression levels of these eight genes and the prognosis of PCa patients. The FRG signature comprised the following genes: SLC2A6, DRD4, AIFM2, AKR1C2, SRC, NGB, MT1G, and HMOX1. Lee et al. found that the inhibition of DRD4 promotes the apoptosis of PCa cells, and that DRD4 may regulate tumor chemosensitivity by inhibiting ferroptosis (33). Dai et al. reported that AIFM2 is a negative regulator of ferroptosis, which negatively regulates ferroptosis through ESCRT-III (34). Zhou and Chen established a prognostic risk model, including the FRG AKR1C2, that could predict the prognosis of acute myeloid leukemia (AML) patients (35). Sun et al. reported that SRC could be used as a prognostic marker for bladder cancer, and the higher the expression level of SRC, the worse the prognosis of patients (36). Wu et al. reported that NGB could serve as an independent prognostic factor in breast cancer patients (37). Sun et al. found that MT1G made liver cancer cells resistant to sorafenib by inhibiting ferroptosis (38). Meng et al. found that the upregulation of HMOX1 was associated with increased ferroptosis during the development of diabetic atherosclerosis; thus, HMOX1 may be a potential therapeutic or drug development target for diabetic atherosclerosis (39).
Our FRG signature was shown to be independently associated with the DFS of patients in both the training and validation cohorts. In our research, the qRT-PCR, Western blot, and IHC results showed that the expression levels of DRD4, SRC, AKR1C2 and AIFM2 were significantly more increased in the PCa samples than the BPH samples. This could indicate that these four genes are associated with the progression of PCa and predict poor patient prognosis. Meanwhile, we also revealed that the expression levels of DRD4, SRC and AKR1C2 were increased in the LNCaP cells treated with ferrostatin-1. These results showed that the expression of DRD4, SRC, and AKR1C2 may be regulated by ferrostatin-1.
The mechanism behind ferroptosis and tumors has been studied previously (40,41); however, little is known about the relationship between ferroptosis and tumor immunity. We calculated ferroptosis risk scores based on our eight-FRG signature and then divided the PCa patients into high- and low-risk groups based on the median ferroptosis risk score. The functional enrichment analysis showed that the differential genes in the high-risk group were mainly enriched in immune-related pathways, such as immune receptor activity, T cell receptor signaling pathway, and primary immunodeficiency. This further suggests that ferroptosis may be closely related to the immune microenvironment of PCa. Moreover, tumor purity was significantly lower in the high-risk group than the low-risk group. However, the ESTIMATE, Immune, and Stromal scores were significantly higher in the high-risk group than the low-risk group. The proportions of mast cells and plasma cells were significantly lower in the high-risk group than the low-risk group of PCa patients. The proportion of Tregs was significantly higher in the high-risk group than the low-risk group.
There were significant differences in tumor immunity between the high- and low-risk groups. The tumor immune microenvironment is very important in the occurrence and development of tumors, and abnormal immune activation or inhibition is an important reason for the immune escape of tumor cells. PCa patients have different tumor immune microenvironment states (42). Research needs to be conducted to determine how to better identify which patients are suitable for immunotherapy (19). Interestingly, our CIBERSORT results showed that the expression of immune checkpoints (PD-1 and PD-L1) was significantly higher in the high-risk group than the low-risk group, and the expression levels of PD-1 and PD-L1 were positively correlated with the ferroptosis risk score.
Our study had several limitations. The eight-FRG predictive signature was obtained by analyzing existing data in TCGA database, and further validation with real clinical data is required. Our model was able to predict patients with high PD-1 and PD-L1 expression; however, the specific roles of PD-1 and PD-L1 still need to be further explored in future experiments. In addition, the sample size was relatively small, which affect the generalizability of our findings. We were unable to confirm the mast cell and plasma cell findings through IHC studies. Furthermore, we did not have the opportunity to examine the prognostic impact of the ferroptosis signature in relation to specific systemic therapies, such as hormonal therapy, chemotherapy, and immunotherapy. Further research is needed to address these limitations and validate our findings with clinical outcomes.
Conclusions
We constructed and validated a PCa prognosis prediction model of eight FRGs through data analysis. We showed that it could serve as a potential independent predictor of DFS in PCa patients using both internal and external databases. We also elucidated the connection between this model and the immune microenvironment of PCa patients. Our findings provide a potential direction for new immunotherapeutic strategies in PCa.
Acknowledgments
Funding: This work was supported by
Footnote
Reporting Checklist: The authors have completed the MDAR and TRIPOD reporting checklists. Available at https://tau.amegroups.com/article/view/10.21037/tau-24-415/rc
Data Sharing Statement: Available at https://tau.amegroups.com/article/view/10.21037/tau-24-415/dss
Peer Review File: Available at https://tau.amegroups.com/article/view/10.21037/tau-24-415/prf
Conflicts of Interest: All authors have completed the ICMJE uniform disclosure form (available at https://tau.amegroups.com/article/view/10.21037/tau-24-415/coif). E.S.A. reports receiving grants from the National Cancer Institute (NCI) grant (No. P30 CA077598) and the Department of Defense (DOD) grant (No. W81XWH-22-2-0025), and Novartis, Celgene, and Orion; E.S.A. reports grants and personal fees from Janssen, Sanofi, Bayer, Bristol Myers Squibb, Curium, MacroGenics, Merck, Pfizer, AstraZeneca, and Clovis; personal fees from Aadi Bioscience, Aikido Pharma, Astellas, Amgen, Blue Earth, Corcept Therapeutics, Exact Sciences, Hookipa Pharma, Invitae, Eli Lilly, Foundation Medicine, Menarini-Silicon Biosystems, Tango Therapeutics, Tempus and Z-alpha; and has a patent for an AR-V7 biomarker technology that has been licensed to Qiagen. W.W. reports receiving funding from the Natural Science Foundation of Huai’an (No. HAB202350), the Natural Science Foundation of Kangda College affiliated to Nanjing Medical University (No. KD2023KYJJ171). W.M. reports receiving funding from the Science and Technology Project of Xuzhou (No. XWKYHT20220111). The other authors have no conflicts of interest to declare.
Ethical Statement: The authors are accountable for all aspects of the work in ensuring that questions related to the accuracy or integrity of any part of the work are appropriately investigated and resolved. The study was conducted in accordance with the Declaration of Helsinki (as revised in 2013). The study was approved by the Ethical Committee Review Board of Lianshui People’s Hospital of Kangda College Affiliated to Nanjing Medical University (Huai’an, Jiangsu, China) (No. 20240424-02) and informed consent was taken from all the patients.
Open Access Statement: This is an Open Access article distributed in accordance with the Creative Commons Attribution-NonCommercial-NoDerivs 4.0 International License (CC BY-NC-ND 4.0), which permits the non-commercial replication and distribution of the article with the strict proviso that no changes or edits are made and the original work is properly cited (including links to both the formal publication through the relevant DOI and the license). See: https://creativecommons.org/licenses/by-nc-nd/4.0/.
References
- Siegel RL, Miller KD, Fuchs HE, et al. Cancer statistics, 2022. CA Cancer J Clin 2022;72:7-33. [Crossref] [PubMed]
- Damber JE, Aus G. Prostate cancer. Lancet 2008;371:1710-21. [Crossref] [PubMed]
- Litwin MS, Tan HJ. The Diagnosis and Treatment of Prostate Cancer: A Review. JAMA 2017;317:2532-42. [Crossref] [PubMed]
- Wang G, Zhao D, Spring DJ, et al. Genetics and biology of prostate cancer. Genes Dev 2018;32:1105-40. [Crossref] [PubMed]
- Zhao L, Zhou X, Xie F, et al. Ferroptosis in cancer and cancer immunotherapy. Cancer Commun (Lond) 2022;42:88-116. [Crossref] [PubMed]
- Zuo YB, Zhang YF, Zhang R, et al. Ferroptosis in Cancer Progression: Role of Noncoding RNAs. Int J Biol Sci 2022;18:1829-43. [Crossref] [PubMed]
- Chen Y, Fan Z, Hu S, et al. Ferroptosis: A New Strategy for Cancer Therapy. Front Oncol 2022;12:830561. [Crossref] [PubMed]
- Stockwell BR, Friedmann Angeli JP, Bayir H, et al. Ferroptosis: A Regulated Cell Death Nexus Linking Metabolism, Redox Biology, and Disease. Cell 2017;171:273-85. [Crossref] [PubMed]
- Seibt TM, Proneth B, Conrad M. Role of GPX4 in ferroptosis and its pharmacological implication. Free Radic Biol Med 2019;133:144-52. [Crossref] [PubMed]
- Feng D, Shi X, Xiong Q, et al. A Ferroptosis-Related Gene Prognostic Index Associated With Biochemical Recurrence and Radiation Resistance for Patients With Prostate Cancer Undergoing Radical Radiotherapy. Front Cell Dev Biol 2022;10:803766. [Crossref] [PubMed]
- Zaffaroni N, Beretta GL. Ferroptosis Inducers for Prostate Cancer Therapy. Curr Med Chem 2022;29:4185-201. [Crossref] [PubMed]
- Liu B, Li X, Wang D, et al. CEMIP promotes extracellular matrix-detached prostate cancer cell survival by inhibiting ferroptosis. Cancer Sci 2022;113:2056-70. [Crossref] [PubMed]
- Zhao R, Lv Y, Feng T, et al. ATF6α promotes prostate cancer progression by enhancing PLA2G4A-mediated arachidonic acid metabolism and protecting tumor cells against ferroptosis. Prostate 2022;82:617-29. [Crossref] [PubMed]
- Frisone D, Friedlaender A, Addeo A, et al. The Landscape of Immunotherapy Resistance in NSCLC. Front Oncol 2022;12:817548. [Crossref] [PubMed]
- Hamilton PT, Anholt BR, Nelson BH. Tumour immunotherapy: lessons from predator-prey theory. Nat Rev Immunol 2022;22:765-75. [Crossref] [PubMed]
- Ilie MD, Vasiljevic A, Jouanneau E, et al. Immunotherapy in aggressive pituitary tumors and carcinomas: a systematic review. Endocr Relat Cancer 2022;29:415-26. [Crossref] [PubMed]
- Munari E, Mariotti FR, Quatrini L, et al. PD-1/PD-L1 in Cancer: Pathophysiological, Diagnostic and Therapeutic Aspects. Int J Mol Sci 2021;22:5123. [Crossref] [PubMed]
- Nishimura CD, Pulanco MC, Cui W, et al. PD-L1 and B7-1 Cis-Interaction: New Mechanisms in Immune Checkpoints and Immunotherapies. Trends Mol Med 2021;27:207-19. [Crossref] [PubMed]
- Islam MK, Stanslas J. Peptide-based and small molecule PD-1 and PD-L1 pharmacological modulators in the treatment of cancer. Pharmacol Ther 2021;227:107870. [Crossref] [PubMed]
- Markowski MC, Taplin ME, Aggarwal R, et al. Bipolar androgen therapy plus nivolumab for patients with metastatic castration-resistant prostate cancer: the COMBAT phase II trial. Nat Commun 2024;15:14. [Crossref] [PubMed]
- Antonarakis ES, Piulats JM, Gross-Goupil M, et al. Pembrolizumab for Treatment-Refractory Metastatic Castration-Resistant Prostate Cancer: Multicohort, Open-Label Phase II KEYNOTE-199 Study. J Clin Oncol 2020;38:395-405. [Crossref] [PubMed]
- Antonarakis ES, Park SH, Goh JC, et al. Pembrolizumab Plus Olaparib for Patients With Previously Treated and Biomarker-Unselected Metastatic Castration-Resistant Prostate Cancer: The Randomized, Open-Label, Phase III KEYLYNK-010 Trial. J Clin Oncol 2023;41:3839-50. [Crossref] [PubMed]
- Boudadi K, Suzman DL, Anagnostou V, et al. Ipilimumab plus nivolumab and DNA-repair defects in AR-V7-expressing metastatic prostate cancer. Oncotarget 2018;9:28561-71. [Crossref] [PubMed]
- Yang X, Yin F, Liu Q, et al. Ferroptosis-related genes identify tumor immune microenvironment characterization for the prediction of prognosis in cervical cancer. Ann Transl Med 2022;10:123. [Crossref] [PubMed]
- Chen W, Chen Y, Liu L, et al. Comprehensive Analysis of Immune Infiltrates of Ferroptosis-Related Long Noncoding RNA and Prediction of Colon Cancer Patient Prognoses. J Immunol Res 2022;2022:9480628. [Crossref] [PubMed]
- Lei G, Zhuang L, Gan B. Targeting ferroptosis as a vulnerability in cancer. Nat Rev Cancer 2022;22:381-96. [Crossref] [PubMed]
- Wang Y, Fan J, Chen T, et al. A novel ferroptosis-related gene prognostic index for prognosis and response to immunotherapy in patients with prostate cancer. Front Endocrinol (Lausanne) 2022;13:975623. [Crossref] [PubMed]
- Liu H, Gao L, Xie T, et al. Identification and Validation of a Prognostic Signature for Prostate Cancer Based on Ferroptosis-Related Genes. Front Oncol 2021;11:623313. [Crossref] [PubMed]
- Ye Z, Zhuo Q, Hu Q, et al. FBW7-NRA41-SCD1 axis synchronously regulates apoptosis and ferroptosis in pancreatic cancer cells. Redox Biol 2021;38:101807. [Crossref] [PubMed]
- Tang X, Ding H, Liang M, et al. Curcumin induces ferroptosis in non-small-cell lung cancer via activating autophagy. Thorac Cancer 2021;12:1219-30. [Crossref] [PubMed]
- Xu X, Zhang X, Wei C, et al. Targeting SLC7A11 specifically suppresses the progression of colorectal cancer stem cells via inducing ferroptosis. Eur J Pharm Sci 2020;152:105450. [Crossref] [PubMed]
- Ghoochani A, Hsu EC, Aslan M, et al. Ferroptosis Inducers Are a Novel Therapeutic Approach for Advanced Prostate Cancer. Cancer Res 2021;81:1583-94. [Crossref] [PubMed]
- Lee SI, Roney MSI, Park JH, et al. Dopamine receptor antagonists induce differentiation of PC-3 human prostate cancer cell-derived cancer stem cell-like cells. Prostate 2019;79:720-31. [Crossref] [PubMed]
- Dai E, Zhang W, Cong D, et al. AIFM2 blocks ferroptosis independent of ubiquinol metabolism. Biochem Biophys Res Commun 2020;523:966-71. [Crossref] [PubMed]
- Zhou F, Chen B. Prognostic significance of ferroptosis-related genes and their methylation in AML. Hematology 2021;26:919-30. [Crossref] [PubMed]
- Sun J, Yue W, You J, et al. Identification of a Novel Ferroptosis-Related Gene Prognostic Signature in Bladder Cancer. Front Oncol 2021;11:730716. [Crossref] [PubMed]
- Wu ZH, Tang Y, Yu H, et al. The role of ferroptosis in breast cancer patients: a comprehensive analysis. Cell Death Discov 2021;7:93. [Crossref] [PubMed]
- Sun X, Niu X, Chen R, et al. Metallothionein-1G facilitates sorafenib resistance through inhibition of ferroptosis. Hepatology 2016;64:488-500. [Crossref] [PubMed]
- Meng Z, Liang H, Zhao J, et al. HMOX1 upregulation promotes ferroptosis in diabetic atherosclerosis. Life Sci 2021;284:119935. [Crossref] [PubMed]
- Lin X, Ping J, Wen Y, et al. The Mechanism of Ferroptosis and Applications in Tumor Treatment. Front Pharmacol 2020;11:1061. [Crossref] [PubMed]
- Yang F, Sun SY, Wang S, et al. Molecular regulatory mechanism of ferroptosis and its role in gastrointestinal oncology: Progress and updates. World J Gastrointest Oncol 2022;14:1-18. [Crossref] [PubMed]
- Sottnik JL, Zhang J, Macoska JA, et al. The PCa Tumor Microenvironment. Cancer Microenviron 2011;4:283-97. [Crossref] [PubMed]
(English Language Editor: L. Huleatt)