Application of proton density fat fraction imaging in risk stratification of prostate cancer
Highlight box
Key findings
• The intra-tumoral fat content in prostate cancer (PCa) is significantly associated with postoperative Gleason scores (GS), aiding in the differentiation between high-risk PCa (GS ≥4+3) and intermediate-low-risk PCa (GS ≤3+4). Furthermore, through multivariate logistic regression analysis, an increase in fat fraction (FF) within PCa is identified as an independent predictor of high-risk PCa.
What is known and what is new?
• Obesity, a recognised risk factor for various cancers including PCa, impacts both PCa incidence and prognosis.
• This study uses magnetic resonance proton density fat fraction imaging to quantitatively detect the FF of prostate lesions, which is significantly correlated with GS and is an independent predictor of high-risk prostate cancer.
What is the implication, and what should change now?
• Clinicians may pay more attention to changes in prostate fat content, especially in patients with a high accumulation of fat, and may require closer prostate cancer screening and monitoring. For diagnosed prostate cancer patients, the fat fraction of the lesion may be considered in treatment decisions.
Introduction
Prostate cancer (PCa) ranks as one of the most prevalent malignancies in men. With our society’s aging demographics, PCa exhibits the highest incidence and a rising mortality trend (1), making it the most common malignancy affecting the male genitourinary system. The Gleason score (GS) is a robust prognostic indicator for PCa progression and survival, influencing treatment decisions (2). This system histologically categorises prostate tissue based on glandular differentiation degree, highlighting tumour heterogeneity through primary and secondary scores. Patients with GS ≤3+3 and GS =3+4 show similar and notably better prognoses than those with GS ≥4+3. Distinct treatment approaches and prognoses exist between these groups (3), with disease recurrence likelihood rising with GS. Therefore, accurately identifying PCa risk levels before treatment is clinically crucial.
Obesity, a recognised risk factor for various cancers including PCa, impacts both PCa incidence and prognosis (4,5). Previous studies (4,6) largely assessed the obesity-PCa link using body mass index (BMI) as a proxy for general obesity, while some (7-9) examined periprostatic fat (visceral fat) amounts to gauge obesity’s correlation with PCa aggressiveness, with results warranting further discussion. None have specifically explored the relationship between prostate fat content and cancer aggressiveness. Magnetic resonance proton density fat fraction (PDFF) imaging offers a non-invasive, in vivo quantitative assessment of tissue fat content. It separates chemically shifted water and fat to accurately gauge tissue fat fraction (FF), correcting for factors affecting magnetic resonance signal intensity (SI) (10-12). Proven effective for non-invasively measuring liver fat content (13,14), this method is also viable for assessing fat accumulation in organs like the pancreas (15), kidneys (16), bone marrow (17), and muscle (18).
This study aims to quantitatively measure fat content within PCa using magnetic resonance imaging (MRI), assess its correlation with PCa aggressiveness, and develop a regression model to stratify pathological risk between the low-risk group (GS ≤3+4) and high-risk group (GS ≥4+3) based on PCa GS. We present this article in accordance with the STARD reporting checklist (available at https://tau.amegroups.com/article/view/10.21037/tau-24-232/rc).
Methods
Study participants
The clinicopathologic data of 467 patients with prostate disease who underwent MRI from September 2020 to March 2022 at The First Affiliated Hospital of Soochow University were retrospectively analysed. Inclusion criteria for the PCa group were: (I) MRI examination within 3 weeks of puncture biopsy or radical resection; (II) complete clinical, pathological, and imaging data; and (III) no history of prostate biopsy or PCa surgery or treatment prior to MRI examination. Exclusion criteria were: (I) patients with recurrence after PCa surgery (n=15); (II) patients with catheters in place (n=48); (III) motion artifacts or magnetic sensitive artifacts caused by gas in the rectum affect magnetic resonance image analysis (n=39); and (IV) bone metastases or metastases to other organs, as well as severe peripheral invasion, cannot undergo radical prostatectomy (RP) (n=31). Data from 143 patients with benign lesions (BLs) and 191 patients with PCa were ultimately included. BLs included benign prostatic hyperplasia (BPH) and prostatitis. The study was conducted in accordance with the Declaration of Helsinki (as revised in 2013). The study was approved by the Medical Ethics Committee of The First Affiliated Hospital of Soochow University (Suzhou, China; 2021. No. 133). Written informed consent was obtained from all patients undergoing the examination. This study was registered on Chinese Clinical Trial Registry (Registration No. ChiCTR2100046948; https://www.chictr.org.cn/).
MRI acquisitions
MRI examinations were conducted using a 3.0 T clinical magnetic resonance scanner (MAGNETOM Skyra, Siemens Healthineers, Erlangen, Germany) with a dedicated 18-channel body-phased array coil. The prostate underwent transverse axial, coronal, and sagittal 2D non-compacted fat T2-weighted imaging (T2WI) fast spin-echo scanning. The scanning parameters for transverse axial T2WI were as follows: repetition time/echo time (TR/TE): 6,980/104 ms, field of view (FOV): 200 mm × 200 mm, resolution: 0.5 mm × 0.5 mm × 3 mm, slice thickness: 3 mm, number of slices: 23, slice gap: 0 mm, and acquisition time of 3 minutes and 22 seconds. Axial plane diffusion-weighted imaging (DWI) scanning parameters were TR/TE: 5,640/60, 96 ms, FOV: 220 mm × 220 mm, resolution: 1.7 mm × 1.7 mm × 3 mm, slice thickness: 3 mm, number of slices: 25, slice gap: 0 mm, and b values of 50, 1,000, and 1,500 s/mm², with an acquisition time of 4 minutes and 12 seconds. Axial Q-DIXON scanning parameters were TR/TE: 9/1.12, 2.46, 3.69, 4.92, 6.15, 7.38 ms, FOV: 360 mm × 280 mm, matrix: 160×98, resolution: 1.1 mm × 1.1 mm × 3 mm, slice thickness: 3 mm, slice gap: 0 mm, flip angle: 15°, and acquisition time of 16 seconds. Axial DWI and Q-DIXON scans were localized to ensure, as much as possible, concordance with the level of the T2WI sequence.
Image analysis
Image analysis was conducted by two imaging physicians with 8 and 10 years of experience in diagnostic prostate imaging, respectively, using the Siemens MRI post-processing workstation syngo.via. The two radiologists discuss and negotiate the different observations to reach an agreed conclusion. Subcutaneous and periprostatic fat thickness (PPFT) was measured using sagittal T2-weighted images selected in the median sagittal plane. These measurements were taken by determining the shortest vertical distance from the pubic symphysis to the skin and from the pubic symphysis to the prostate in the median sagittal plane, respectively, as shown in Figure 1 (19). The shortest vertical distance was used to avoid overestimation of these measurements. MRI/transrectal ultrasound (TRUS) fusion puncture biopsy of the targeted lesion was correlated with the MRI image. The region of interest (ROI) was placed over the nodule or mass in the largest cross-section of the lesion to encompass as much of the targeted lesion area as possible, while excluding areas of necrosis, hemorrhage, and cystic lesions. Peripheral zone (PZ) lesions in the PCa group were outlined using apparent diffusion coefficient (ADC) maps supplemented by diffusion-weighted imaging (DWI) and T2WI sequences. Transition zone (TZ) lesions were outlined using T2WI supplemented by DWI and ADC maps. FF maps of the lesions were obtained through image fusion alignment in the Q-DIXON sequences, as illustrated in Figures 2,3. Additionally, the ADC value and normalized T2 signal intensity (T2SI) of the lesion were measured separately. For the T2WI image, we placed an ROI to measure the signal value of the lesion, and placed an additional ROI in the obturator internus muscle as a reference tissue to obtain the muscle signal. We then calculated the signal ratio of the tumor to muscle, obtaining T2SI (20). When patients had multiple lesions, only the lesion with the highest or largest GS (if the GS was the same) was evaluated. Whole gland FF scores were obtained by outlining the whole prostate gland using volume of interest (VOI) for prostates in the prostatic hyperplasia and PCa groups.
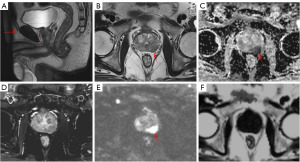
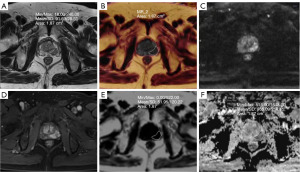
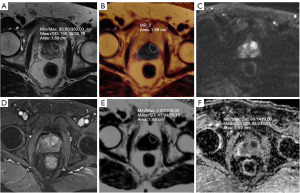
Data collection
Clinical data encompassed patient age, BMI, smoking history, alcohol consumption history, diabetes history, serum prostate-specific antigen (PSA) level, MRI-measured prostate volume (PV), ADC value, T2SI, Prostate Imaging Reporting and Data System 2.1 (PI-RADS 2.1) score, focal FF, whole gland FF, PPFT, and subcutaneous fat thickness (SFT). Patients with a PI-RADS score of ≥3 underwent TRUS/MRI fusion-targeted biopsy. Each biopsy needle was individually labeled and subjected to histopathology-based diagnosis. Patients with biopsy-proven PCa subsequently underwent radical RP, active surveillance (AS), androgen deprivation therapy (ADT), or radiation therapy (RT). Pathology specimens were analyzed using haematoxylin and eosin (HE) staining and immunohistochemistry. Two urological pathologists with over 10 years of experience observed the histological sections to determine lesion location and boundaries, and performed Gleason scoring, selecting the cancerous foci with the highest GS for analysis. This approach was in accordance with the guidelines for grading PCa established by the International Society of Urological Pathology (ISUP) Consensus Conference in 2014 (21). The GS results depended mainly on the pathology of the dissected tumor tissue during RP; in cases where RP was not performed, the results of the puncture biopsy were used instead. The GS results of the PCa group were categorized into low- and intermediate-risk groups as 3+4=7 and 3+3=6, and high-risk groups as 4+3=7 as well as GS ≥8.
Statistical analysis
The Chi-squared test or Fisher’s exact test was employed for count data, while the two independent samples t-test or Mann-Whitney U test was utilised for measurement data. Quantitative data were expressed as means ± standard deviations (SDs) or medians with IQRs according to the normality or nonnormality of distributions, respectively. Pearson or Spearman correlation coefficients were utilised to analyse the correlation between each clinicopathological variable (age, BMI, PSA, PV, PI-RADS, ADC values, FF, T2SI, PPFT, and SFT) and GS. Multifactorial binary logistic regression analysis was conducted to calculate the odds ratios (ORs) of predictors in the low- and medium-risk and high-risk groups in PCa. The Hosmer-Lemeshow goodness-of-fit test was applied to assess the model’s fit to the observed data. For better application to individual risk assessment, the area under the curve (AUC) was calculated to measure the efficacy of the model based on a multifactor binary logistic regression analysis of the receiver operating characteristic (ROC). A nomogram was constructed for the model to visualize the probability of predicting the occurrence of high-risk PCa. Column line plots were generated using the statistical software package R version 4.2.2 (R Foundation for Statistical Computing, Vienna, Austria), and other statistical tests were conducted using SPSS 25.0 (IBM Corp., Armonk, NY, USA). All P values were two-sided, and differences were considered statistically significant at P<0.05.
Results
Clinical characteristics
The differences between the BL group and the PCa group were statistically significant (P<0.05) for PV, PSA, whole gland FF, PPFT, and SFT, as shown in Table 1. A total of 191 patients were enrolled in the PCa group, of which 63 cases were in the low- and intermediate-risk group, with 5 cases having postoperative GS scores of 3+3 points and 58 cases with 3+4 points. In the high-risk group, there were 128 cases with GS scores of 4+3 in 55 cases, 4+4 in 34 cases, 4+5 in 31 cases, 5+4 in 6 cases, and 5+5 in 2 cases. 16 patients with PCa who did not receive RP after fusion targeted biopsy were assigned GSs by biopsy. In addition, 27 patients with puncture samples were at low or medium risk, and the GS was upgraded to high risk after RP. The differences between the low and medium-risk groups and the high-risk group were statistically significant (P<0.05) for patients’ BMI, PV, PSA, tumor ADC value, T2SI, PI-RADS score, lesion FF, and PPFT. There was no statistically significant difference between the two groups in terms of age, history of smoking, history of alcohol consumption, history of diabetes mellitus, and SFT (P>0.05) (Table 2).
Table 1
Variables | BL (n=143) | PCa (n=191) | t/χ2 | P |
---|---|---|---|---|
Age (years) | 70.4±7.7 | 70.8±6.8 | −0.541a | 0.59 |
BMI (kg/m2) | 23.5±2.9 | 24.1±2.9 | −1.947a | 0.052 |
Smoking history | 0.000b | 0.99 | ||
Yes | 62 (43.4) | 83 (43.5) | ||
No | 81 (56.6) | 108 (56.5) | ||
Drinking history | 0.047b | 0.83 | ||
Yes | 53 (37.1) | 73 (38.2) | ||
No | 90 (62.9) | 118 (61.8) | ||
History of diabetes | 0.143b | 0.71 | ||
Yes | 50 (35.0) | 63 (33.0) | ||
No | 93 (65.0) | 128 (67.0) | ||
PV (cm3) | 113 (84, 154) | 73 (56.3, 105.2) | −7.706c | <0.001 |
PSA (ng/mL) | 5.6 (3.2, 10.6) | 16.5 (8.9, 32.3) | −9.943c | <0.001 |
Whole gland FF (%) | 1.0±0.3 | 2.9±1.3 | −17.102a | <0.001 |
PPFT (mm) | 4 (3, 5.8) | 5.9 (4, 7.6) | −5.033c | <0.001 |
SFT (mm) | 25.5 (20.5, 31) | 32.8 (24, 38.6) | −4.830c | <0.001 |
Quantitative data are expressed as mean ± SD or median (IQR), while categorical data are presented as n (%). a, data were analyzed using independent samples t-test; b, data were analyzed using Chi-squared test; c, data were analyzed using Mann-Whitney U test. BL, benign lesion; PCa, prostate cancer; BMI, body mass index; PV, prostate volume; PSA, prostate serum-specific antigen; FF, fat fraction; PPFT, periprostatic fat thickness; SFT, subcutaneous fat thickness; SD, standard deviation; IQR, interquartile range.
Table 2
Variables | Low- and medium-risk group (n=63) | High-risk group (n=128) | t/χ2 | P |
---|---|---|---|---|
Age (years) | 69.7±6.1 | 71.4±6.3 | −1.64a | 0.10 |
BMI (kg/m2) | 24.6±2.7 | 23.7±3.0 | 2.008a | 0.046 |
Smoking history | 1.099b | 0.29 | ||
Yes | 24 (38.1) | 59 (46.1) | ||
No | 39 (61.9) | 69 (53.9) | ||
Drinking history | 0.085b | 0.77 | ||
Yes | 25 (39.7) | 48 (37.5) | ||
No | 38 (60.3) | 80 (62.5) | ||
History of diabetes | 0.159b | 0.69 | ||
Yes | 22 (34.9) | 41 (32.0) | ||
No | 41 (65.1) | 87 (68.0) | ||
PV (cm3) | 86 (58.0, 122.9) | 67.1 (52.7, 94) | −2.357c | 0.02 |
PSA (ng/mL) | 11.1 (6.5, 18.5) | 22.3 (11.3, 49.5) | −5.128c | <0.001 |
ADC (mm2/s) | 0.857±0.177 | 0.730±0.126 | 5.334a | <0.001 |
T2SI | 3.08±0.55 | 2.92±0.46 | 2.016a | 0.045 |
PI-RADS | 29.661b | <0.001 | ||
3 | 38 (60.3) | 30 (23.4) | ||
4 | 19 (30.2) | 47 (36.7) | ||
5 | 6 (9.5) | 51 (39.8) | ||
Lesion FF (%) | 2.07±0.57 | 2.97±0.88 | −7.215a | <0.001 |
PPFT (mm) | 5.0 (4.0, 6.6) | 6.1 (4.7, 8) | −2.936c | 0.003 |
SFT (mm) | 30.5 (24, 37) | 32.8 (23.6, 42.2) | −1.028c | 0.30 |
Quantitative data are expressed as mean ± SD or median (IQR), while categorical data are presented as n (%). a, data were analyzed using independent samples t-test; b, data were analyzed using chi-square test; c, data were analyzed using Mann-Whitney U test. PCa, prostate cancer; BMI, body mass index; PV, prostate volume; PSA, prostate serum-specific antigen; ADC, apparent diffusion coefficient; T2SI, T2 signal intensity; PI-RADS, Prostate Imaging Reporting and Data System; FF, fat fraction; PPFT, periprostatic fat thickness; SFT, subcutaneous fat thickness; SD, standard deviation; IQR, interquartile range.
Correlation analysis of patient data and GS in PCa group
The GS score exhibited a significant positive correlation with FF (r=0.543, P<0.001), PSA (r=0.312, P<0.001), and PI-RADS score (r=0.366, P<0.001) in the PCa group, and a significant negative correlation with the ADC value (r=−0.370, P<0.001). However, GS showed no significant correlation (P>0.001) with age, BMI, PV, T2SI, PPFT, and SFT. Additionally, there was no significant correlation between lesion FF and BMI, PPFT, SFT, and PV in the PCa group (P>0.05).
ADC values and PDFF diagnosis in low-risk PCa ROC curve analysis
The ADC value of the low-risk group in PCa was significantly higher than that of the high-risk group, with a statistically significant difference (P<0.001) (Table 2). The area under the ROC curve of the ADC value for diagnosing low-risk PCa was 0.715 (Figure 4A), with an optimal cut-off value of 0.98 mm²/s. Furthermore, the FF value of the lesion in low-risk PCa was significantly lower than that in the high-risk group, also with a statistically significant difference (P<0.001) (Table 2). The area under the ROC curve for FF values in diagnosing low and intermediate risk PCa was 0.855 (Figure 4B), with an optimal cutoff value of 1.92%.
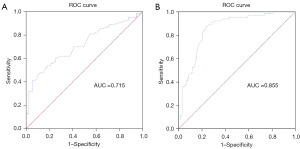
Multifactor logistic regression analysis
Introducing clinical and imaging characteristics with P<0.05 in both groups into the multifactorial logistic regression analysis revealed (Table 3) that an increased FF and a PI-RADS score of 5 were independent predictors of high-risk PCa (P<0.05). Conversely, a decreased ADC value and PV were also independent predictors of high-risk PCa (P<0.05). Models A (ADC value + PV + PI-RADS) and B (ADC value + PV + PI-RADS + FF) were constructed. The Hosmer-Lemeshow goodness-of-fit test indicated that Models A and B were well-fitted to the observed data (P=0.69, 0.91). Models A and B achieved prediction accuracies of 74.3% and 81.2%, respectively. Model A’s ROC curves showed a sensitivity of 82.8% and a specificity of 58.7%, with 0.569 as the optimal cutoff value for the logistic regression model, and an AUC of 0.777 for distinguishing high-risk PCa. On the other hand, Model B’s ROC curves showed that with 0.67 as the best cutoff value for the logistic regression model, the AUC for distinguishing high-risk PCa was 0.907, with a sensitivity of 78.1% and a specificity of 88.9%. A nomogram was constructed based on the multivariate logistic regression model to distinguish the high-risk group of PCa from the medium- and low-risk groups in the cohort, as shown in Figure 5.
Table 3
Variable | Odds ratio (95% CI) | P |
---|---|---|
FF (%) | 6.371 (2.995–13.553) | <0.001 |
ADC (mm2/s) | 0.037 (0.002–0.732) | 0.03 |
PV (cm3) | 0.989 (0.980–0.999) | 0.03 |
PI-RADS 5 | 3.782 (1.066–13.42) | 0.04 |
PCa, prostate cancer; CI, confidence interval; FF, fat fraction; ADC, apparent diffusion coefficient; PV, prostate volume; PI-RADS, Prostate Imaging Reporting and Data System.
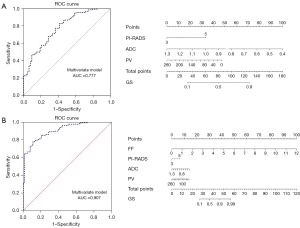
Discussion
The use of MRI for prostate disease detection is increasingly common. While routine multi-parameter magnetic resonance (Mp-MRI) examinations combined with targeted puncture biopsies can detect most clinically significant PCa, their utility is limited by the standardization of reporting and imaging techniques, as well as the invasiveness of puncture biopsies. In our study, we introduced a non-invasive quantitative measurement of intraprostatic fat content based on magnetic resonance PDFF imaging. The BL group exhibited lower fat content than the PCa group, and this difference was statistically significant. Additionally, significant differences were observed between the groups in terms of PV, PSA, PPFT, and SFT. Intra-PCa fat content significantly correlated with postoperative GS scores, aiding in the differentiation between high-risk (GS ≥4+3) PCa and intermediate- to low-risk (GS ≤3+4) PCa. Furthermore, an increase in intra-PCa fat content was identified as an independent predictor of high-risk PCa through multiple logistic regression analysis. When combined with other factors, this model significantly improved the ability to identify high-risk PCa from the medium- and low-risk groups.
There are many clinical methods to evaluate the aggressiveness of PCa, including histological grading system, imaging evaluation and biomarker detection. GS system is the most widely used histological classification of PCa, and has a good correlation with the biological behavior and prognosis of PCa. However, the GS system has significant limitations. It categorizes prognosis and treatment into three levels: 6, 7, and 8–10. While a GS of 7 can represent a majority of highly differentiated carcinomas with a small proportion of poorly differentiated carcinomas (Gleason 3+4=7) or a majority of poorly differentiated carcinomas with a small proportion of highly differentiated carcinomas (4+3=7), a simple unification of the group overlooks the fact that 3+4 and 4+3 have different prognoses in terms of therapeutic decision making. Gleason 7 tumors are heterogeneous, and patients with Gleason 4+3 tumors have a significantly higher rate of biochemical recurrence (BCR) compared to Gleason 3+4 tumors (22). Similarly, in a study by Stark et al. (23), it was found that patients with GS =3+4 and 4+3 had different biological characteristics, with PCa having GS =4+3 being three times more likely to result in a fatal outcome than those with GS =3+4. In another histological grading system, the ISUP grade group was divided into five groups with significant prognostic differences. The GS score of 3+4 is defined as level 2. A GS score of 4+3 is defined as level 3. The higher the grade group, the worse the prognosis. Therefore, in this study, we categorized PCa according to GS into low- and medium-risk group PCa (GS ≤3+4) and high-risk group (GS ≥4+3) PCa.
A multifactorial logistic regression analysis revealed that an increase in FF, along with a decrease in PI-RADS score 5, ADC value, and PV, were independent predictors of high-risk PCa. The application of PI-RADS, T2WI combined with diffusion-weighted imaging (DWI), is increasingly common in PCa detection. DWI, which characterises the microstructure of tissues by detecting changes in diffusion limitation, quantifies these changes as ADC values. PCa at different pathologic levels exhibits differences in internal cellular composition, fluid content, collagen levels, and fibromuscular matrix, among other characteristics. In a meta-analysis conducted by Shaish et al. (24), the assessment of the use of quantitative ADC values in GS risk stratification was carried out, showing moderate accuracy in predicting high-risk PCa. ADC values in this study showed a significant negative correlation with PCa GS, consistent with the results of Tian et al. (25). As the GS increased, the ADC value decreased gradually, and the DWI map signal increased gradually. These changes may result from the increasingly dense cellular arrangement and the reduction of extracellular space in high-grade tumors. The degree of decrease in ADC values may predict the progression of clinical PCa. Magnetic resonance PDFF imaging is a technique that separates water and fat based on the 3.5 ppm slower chemical shift of fat protons than the water proton incoming frequency in human tissues. It quantifies fat by plotting ROIs on the resulting FF maps. Magnetic resonance PDFF provides an accurate spectral model of fat, giving the ratio of the density of mobile protons from triglycerides to the total proton density from mobile triglycerides and mobile water, visually quantifying the change in triglycerides from 0 to 100% and calculating the concentration of mobile triglycerides in the tissue (12). The technique allows the evaluation of tissue in a short period and is easy to implement in clinical examinations. Among non-invasive imaging modalities, MRI-PDFF is considered the most accurate method for quantitatively measuring liver fat content. In a previous randomized controlled trial, Le et al. (26) used MRI-PDFF to quantify hepatic steatosis in patients with nonalcoholic steatohepatitis (NASH), cross-sectionally comparing magnetic resonance PDFF imaging, MR spectroscopy (MRS)-derived PDFF, and nontargeted liver biopsy. The study demonstrated good agreement and correlation between them and with the grading of steatosis from histologic sources. It also found that MRI-PDFF was more sensitive than biopsy for detecting changes in hepatic steatosis in longitudinal studies.
Lipid metabolism plays a crucial role in the progression of PCa and serves as an indicator of disease aggressiveness and grade (8,27). A previous study (28) suggested that PCa periglandular fat secretes more tumour drivers such as interleukin (IL)-6, IL-8, IL-10, interferon (IFN), tumor necrosis factor (TNF), and vascular endothelial growth factor (VEGF) compared to peripheral fat. Simultaneously, tumour cells regulate the secretion of aggressive cytokines by the periglandular adipose tissue, explaining the relationship between obesity and PCa. In our study, although the difference in BMI was not statistically significant in the PCa group compared to the prostatic hyperplasia group, PPFT, SFT, and whole gland FF were higher in the PCa group. This may be because changes in localised lipid metabolism around the prostate more accurately reflect the impact on PCa compared to the general surrogate index of obesity. However, our study found no correlation between PPFT, SFT, and the degree of PCa invasion, consistent with the findings of Laine-Caroff et al. (29). Standardising the definition and measurement of periprostatic fat requires further support from additional studies, indicating the need for further investigation into the role of peripheral fat in PCa progression. Intraprostatic fat content was not directly assessed by imaging in previous studies. Our study introduces a new method for non-invasively quantifying prostatic fat content. We found that whole-gland PDFF was higher in the PCa group than in the prostatic hyperplasia group and that increased PDFF within PCa was an independent predictor of high-risk PCa. PCa cells are more inclined to utilise the fatty acid oxidation pathway for metabolic reprogramming to obtain energetic substances, unlike most other tumours that use the Warburg effect generated by glycolysis for energy. This difference may result in increased lipid uptake and accumulation in PCa cells (27). A study by Randall et al. (30), using mass spectrometry imaging to analyse lipids and metabolites in prostate tissues, not only found an increase in lipid intensity in the prostate tumour region but also a similar increase in the surrounding normal tissue, with a gradient of change correlating well with GS. Disturbances and accumulation of lipid metabolism in tumour cells can further accelerate disease progression in PCa and may impede drug entry into the nucleus to exert their functional effects (31).
There are several limitations in this study. Firstly, it is a single-center study without external validation. Prospective large-sample multicenter studies are needed to establish the correlation between fat content and cancer invasiveness in the prostate. Secondly, our outlining of the whole glandular FF of the prostate may include artifacts such as hemorrhage or cystic changes, which may not fully reflect the overall signal characteristics and fat content of the prostate gland. Thirdly, when outlining the ROI of the lesion, it can be challenging to precisely match the MRI image with the pathology section, affecting the accuracy of the ROI outlining. Fourthly, while PDFF within PCa was positively correlated with GS in this study, we did not demonstrate a significant correlation between tumor PDFF and poor outcomes. Large-sample case collections and follow-up analyses are necessary in the future.
Conclusions
In the current study, a non-invasive quantitative measurement of intraprostatic fat content was introduced based on magnetic resonance PDFF imaging. A combined clinical and imaging columnar mapping model was established for risk stratification of PCa, assessing its aggressiveness, and providing an effective tool for individualized treatment of PCa patients.
Acknowledgments
Funding: This work was primarily supported by
Footnote
Reporting Checklist: The authors have completed the STARD reporting checklist. Available at https://tau.amegroups.com/article/view/10.21037/tau-24-232/rc
Data Sharing Statement: Available at https://tau.amegroups.com/article/view/10.21037/tau-24-232/dss
Peer Review File: Available at https://tau.amegroups.com/article/view/10.21037/tau-24-232/prf
Conflicts of Interest: All authors have completed the ICMJE uniform disclosure form (available at https://tau.amegroups.com/article/view/10.21037/tau-24-232/coif). The authors have no conflicts of interest to declare.
Ethical Statement: The authors are accountable for all aspects of the work in ensuring that questions related to the accuracy or integrity of any part of the work are appropriately investigated and resolved. The study was conducted in accordance with the Declaration of Helsinki (as revised in 2013). The study was approved by the Medical Ethics Committee of The First Affiliated Hospital of Soochow University (Suzhou, China; 2021. No. 133). Written informed consent was obtained from all patients undergoing the examination.
Open Access Statement: This is an Open Access article distributed in accordance with the Creative Commons Attribution-NonCommercial-NoDerivs 4.0 International License (CC BY-NC-ND 4.0), which permits the non-commercial replication and distribution of the article with the strict proviso that no changes or edits are made and the original work is properly cited (including links to both the formal publication through the relevant DOI and the license). See: https://creativecommons.org/licenses/by-nc-nd/4.0/.
References
- Xia C, Dong X, Li H, et al. Cancer statistics in China and United States, 2022: profiles, trends, and determinants. Chin Med J (Engl) 2022;135:584-90. [Crossref] [PubMed]
- Han M, Partin AW, Pound CR, et al. Long-term biochemical disease-free and cancer-specific survival following anatomic radical retropubic prostatectomy. The 15-year Johns Hopkins experience. Urol Clin North Am 2001;28:555-65. [Crossref] [PubMed]
- Epstein JI, Zelefsky MJ, Sjoberg DD, et al. A Contemporary Prostate Cancer Grading System: A Validated Alternative to the Gleason Score. Eur Urol 2016;69:428-35. [Crossref] [PubMed]
- Renehan AG, Tyson M, Egger M, et al. Body-mass index and incidence of cancer: a systematic review and meta-analysis of prospective observational studies. Lancet 2008;371:569-78. [Crossref] [PubMed]
- MacInnis RJ, English DR. Body size and composition and prostate cancer risk: systematic review and meta-regression analysis. Cancer Causes Control 2006;17:989-1003. [Crossref] [PubMed]
- Cariolou M, Markozannes G, Becerra-Tomás N, et al. Association between adiposity after diagnosis of prostate cancer and mortality: systematic review and meta-analysis. BMJ Med 2023;2:e000339. [Crossref] [PubMed]
- Zhang Q, Sun LJ, Qi J, et al. Periprostatic adiposity measured on magnetic resonance imaging correlates with prostate cancer aggressiveness. Urol J 2014;11:1793-9. [PubMed]
- Altuna-Coy A, Ruiz-Plazas X, Sánchez-Martin S, et al. The lipidomic profile of the tumoral periprostatic adipose tissue reveals alterations in tumor cell's metabolic crosstalk. BMC Med 2022;20:255. [Crossref] [PubMed]
- Chien YH, Hsieh ML, Sheng TW, et al. Body composition and pelvic fat distribution are associated with prostate cancer aggressiveness and can predict biochemical recurrence. Medicine (Baltimore) 2022;101:e31076. [Crossref] [PubMed]
- Yokoo T, Bydder M, Hamilton G, et al. Nonalcoholic fatty liver disease: diagnostic and fat-grading accuracy of low-flip-angle multiecho gradient-recalled-echo MR imaging at 1.5 T. Radiology 2009;251:67-76. [Crossref] [PubMed]
- Reeder SB, Robson PM, Yu H, et al. Quantification of hepatic steatosis with MRI: the effects of accurate fat spectral modeling. J Magn Reson Imaging 2009;29:1332-9. [Crossref] [PubMed]
- Reeder SB, Cruite I, Hamilton G, et al. Quantitative Assessment of Liver Fat with Magnetic Resonance Imaging and Spectroscopy. J Magn Reson Imaging 2011;34:729-49. [Crossref] [PubMed]
- Tang A, Desai A, Hamilton G, et al. Accuracy of MR imaging-estimated proton density fat fraction for classification of dichotomized histologic steatosis grades in nonalcoholic fatty liver disease. Radiology 2015;274:416-25. [Crossref] [PubMed]
- Caussy C, Reeder SB, Sirlin CB, et al. Noninvasive, Quantitative Assessment of Liver Fat by MRI-PDFF as an Endpoint in NASH Trials. Hepatology 2018;68:763-72. [Crossref] [PubMed]
- Schawkat K, Eshmuminov D, Lenggenhager D, et al. Preoperative Evaluation of Pancreatic Fibrosis and Lipomatosis: Correlation of Magnetic Resonance Findings With Histology Using Magnetization Transfer Imaging and Multigradient Echo Magnetic Resonance Imaging. Invest Radiol 2018;53:720-7. [Crossref] [PubMed]
- Idilman IS, Tuzun A, Savas B, et al. Quantification of liver, pancreas, kidney, and vertebral body MRI-PDFF in non-alcoholic fatty liver disease. Abdom Imaging 2015;40:1512-9. [Crossref] [PubMed]
- Martel D, Leporq B, Saxena A, et al. 3T chemical shift-encoded MRI: Detection of altered proximal femur marrow adipose tissue composition in glucocorticoid users and validation with magnetic resonance spectroscopy. J Magn Reson Imaging 2019;50:490-6. [Crossref] [PubMed]
- Schlaeger S, Inhuber S, Rohrmeier A, et al. Association of paraspinal muscle water-fat MRI-based measurements with isometric strength measurements. Eur Radiol 2019;29:599-608. [Crossref] [PubMed]
- Woo S, Cho JY, Kim SY, et al. Periprostatic fat thickness on MRI: correlation with Gleason score in prostate cancer. AJR Am J Roentgenol 2015;204:W43-7. [Crossref] [PubMed]
- Wang L, Mazaheri Y, Zhang J, et al. Assessment of biologic aggressiveness of prostate cancer: correlation of MR signal intensity with Gleason grade after radical prostatectomy. Radiology 2008;246:168-76. [Crossref] [PubMed]
- Egevad L, Delahunt B, Srigley JR, et al. International Society of Urological Pathology (ISUP) grading of prostate cancer - An ISUP consensus on contemporary grading. APMIS 2016;124:433-5. [Crossref] [PubMed]
- Lau WK, Blute ML, Bostwick DG, et al. Prognostic factors for survival of patients with pathological Gleason score 7 prostate cancer: differences in outcome between primary Gleason grades 3 and 4. J Urol 2001;166:1692-7. [Crossref] [PubMed]
- Stark JR, Perner S, Stampfer MJ, et al. Gleason score and lethal prostate cancer: does 3 + 4 = 4 + 3? J Clin Oncol 2009;27:3459-64. [Crossref] [PubMed]
- Shaish H, Kang SK, Rosenkrantz AB. The utility of quantitative ADC values for differentiating high-risk from low-risk prostate cancer: a systematic review and meta-analysis. Abdom Radiol (NY) 2017;42:260-70. [Crossref] [PubMed]
- Tian W, Zhang J, Tian F, et al. Correlation of diffusion tensor imaging parameters and Gleason scores of prostate cancer. Exp Ther Med 2018;15:351-6. [PubMed]
- Le TA, Chen J, Changchien C, et al. Effect of colesevelam on liver fat quantified by magnetic resonance in nonalcoholic steatohepatitis: a randomized controlled trial. Hepatology 2012;56:922-32. [Crossref] [PubMed]
- Watt MJ, Clark AK, Selth LA, et al. Suppressing fatty acid uptake has therapeutic effects in preclinical models of prostate cancer. Sci Transl Med 2019;11:eaau5758. [Crossref] [PubMed]
- Islami F, Goding Sauer A, Gapstur SM, et al. Proportion of Cancer Cases Attributable to Excess Body Weight by US State, 2011-2015. JAMA Oncol 2019;5:384-92. [Crossref] [PubMed]
- Laine-Caroff P, Bruyere F, Mathieu R, et al. The volume and thickness of preprostatic fat on MRIs are not associated with prostate cancer aggressiveness in men undergoing radical prostatectomy. Prog Urol 2022;32:341-53. [Crossref] [PubMed]
- Randall EC, Zadra G, Chetta P, et al. Molecular Characterization of Prostate Cancer with Associated Gleason Score Using Mass Spectrometry Imaging. Mol Cancer Res 2019;17:1155-65. [Crossref] [PubMed]
- Ahmad F, Cherukuri MK, Choyke PL. Metabolic reprogramming in prostate cancer. Br J Cancer 2021;125:1185-96. [Crossref] [PubMed]