Identifying CDCA3 as a pivotal biomarker for predicting outcomes and immunotherapy efficacy in pan-renal cell carcinoma
Highlight box
Key findings
• This study investigates cell division cycle-associated 3 (CDCA3) as a novel biomarker for prognostic assessment and immunotherapy response in renal cell carcinoma (RCC).
What is known and what is new?
• The gene encoding CDCA3 is situated on chromosome 12p12, and it translates into a protein composed of 268 amino acids. CDCA3 takes part in both physiological and pathological processes in humans by modulating a broad array of downstream cytokines. Although there have been reports of CDCA3’s involvement in kidney renal clear cell carcinoma (KIRC) and kidney renal papillary cell carcinoma (KIRP), comprehensive comprehension of its function across RCC remains incomplete.
• This study integrates multi-omics data from the three most prevalent types of RCC, KIRC, KIRP, and kidney chromophobe (KICH), along with in-vitro experiments and clinical samples to thoroughly analyze the role of CDCA3 as a potential biomarker in pan-RCC.
What is the implication, and what should change now?
• Our research highlights CDCA3’s role as a prognostic and immunotherapy biomarker for RCC. These findings have significant implications for prognostic risk assessments and inform the development of targeted therapies and immunomodulatory strategies for RCC.
Introduction
In the year 2023, it was projected that renal cancers would constitute 5% of all new cancer diagnoses among both genders in the United States, with renal cell carcinoma (RCC) being the predominant form. Pathological classification of renal tumors is based on their cellular origin and behavioral characteristics, and each histological subtype is distinguished by distinct patterns in terms of incidence and clinical outcomes (1). This investigation focuses on three widespread types of RCC: kidney chromophobe (KICH), kidney renal papillary cell carcinoma (KIRP), and kidney renal clear cell carcinoma (KIRC) (2). Due to ongoing enhancements in diagnostic procedures and therapeutic approaches for RCC, the 5-year survival rate for patients has increased to at least 74%. Nonetheless, 12% of individuals experience progression to advanced metastatic disease (3). When surgical removal of advanced renal malignancies is unfeasible, life expectancy may be extended through molecularly targeted therapies, as the combination of chemotherapy and targeted therapy can achieve a robust objective response rate of 71% (4). Therefore, it is crucial to further investigate the pathogenesis of RCC to identify new biomarkers that can improve disease management and prognostic evaluation.
The cell division cycle-associated (CDCA) gene family, comprising eight members (CDCA1–8), is known for its critical role in regulating mitosis, particularly in processes such as chromosome segregation and cell division that are closely associated with cancer. Certain members of this family, such as CDCA1, CDCA5, and CDCA8, have been implicated in tumorigenesis and poor prognosis in various cancers due to their influence on cell proliferation. These findings suggest the potential significance of CDCA genes in cancer biology (5-9).
Among these genes, CDCA3 has garnered particular attention in the context of RCC. The gene encoding CDCA3 is located on chromosome 12p12 and encodes a protein involved in regulating the cell cycle through its role in the Skp1-Cullin-F-box (SCF) ubiquitin ligase complex (10). Although CDCA3’s role has been studied in some RCC subtypes, a comprehensive understanding of its impact across different RCC subtypes remains incomplete (11,12) In this study, we integrate multi-omics data from the three most prevalent types of RCC (KIRC, KIRP, and KICH), alongside in-vitro experiments and clinical samples, to thoroughly analyze the role of CDCA3 as a potential biomarker in pan-RCC. By focusing on CDCA3, we aim to elucidate its potential as a prognostic marker and its implications in the management and therapeutic response of RCC. We present this article in accordance with the MDAR reporting checklist (available at https://tau.amegroups.com/article/view/10.21037/tau-24-233/rc).
Methods
Flowchart of this study
Figure 1 illustrates the integration of multi-omics data from The Cancer Genome Atlas (TCGA) for RCC, encompassing KIRC, KIRP, and KICH. Our analysis revealed the potential of CDCA3 as a biomarker in RCC. Subsequent assessments using Cox regression and Kaplan-Meier survival analyses were performed to investigate the association of CDCA3 with adverse outcomes in RCC. Further investigations into CDCA3’s function in RCC were undertaken through gene set enrichment analysis (GSEA), immune infiltration, immunohistochemistry (IHC), responses to immunotherapy, and mutation analysis. The study was conducted in accordance with the Declaration of Helsinki (as revised in 2013).
Data collection and processing
To initiate this study, we downloaded RNA sequencing (RNA-seq) data, corresponding mutation data, and clinical information for RCC, specifically KIRC, KIRP, and KICH, from the UCSC Xena platform (https://xenabrowser.net/datapages/) (13). We employed the R packages “clusterProfiler” (version 4.8.1) and “org.Hs.eg.db” to convert RNA-seq Ensembl IDs to SYMBOL IDs (14). Dataset summary is provided in Tables S1,S2.
Prognostic analysis
We utilized the R package “survival” (version 3.5-5) to perform univariate Cox regression and Kaplan-Meier analysis to evaluate the prognostic impact of gene expressions in RCC.
Functional enrichment
We assessed the correlation between CDCA3 expression and all other messenger RNA (mRNA) expressions using the Spearman correlation method. The ranked list of genes was then analyzed using the “clusterProfiler” package (version 4.8.1) to conduct GSEA. This analysis aimed to identify enrichment related to Gene Ontology (GO), Kyoto Encyclopedia of Genes and Genomes (KEGG) pathways, and Hallmark gene sets (MSigDB v7.0).
IHC
The RCC tissue microarrays utilized in our study were procured from Shanghai Outdo Biotech Company (Shanghai, China). The methodologies employed in our study are briefly summarized below, with further details available in our previously published work (15). The following procedure was employed for the tissue section processing: Sections were initially treated with a 1% H2O2 solution and subsequently blocked using non-immunogenic goat serum. The sections were incubated overnight with primary antibodies at 4 ℃. This was followed by a 30-minute incubation at room temperature with biotinylated secondary antibodies, which were used to facilitate binding to the primary antibodies. The primary antibody applied was an anti-CDCA3 antibody (YT0819; ImmunoWay, Plano, TX, USA).
Western blot
Cell lysates were prepared by collecting cells and lysing them in radioimmunoprecipitation assay (RIPA) buffer containing protease inhibitors. The extracted protein samples were then separated via sodium dodecyl sulfate-polyacrylamide gel electrophoresis (SDS-PAGE) and electrotransferred onto polyvinylidene fluoride (PVDF) membranes, which were subsequently blocked with 5% non-fat milk. Membranes were incubated with anti-CDCA3 antibody (YT0819; ImmunoWay) at a dilution of 1:2,000 and anti-β-actin antibody (ImmunoWay) at 1:5,000. Afterward, membranes were washed three times with PBST for 10 minutes each, before detection. β-actin was used as a normalization control. Protein band intensities were quantified using ImageJ software. Here is the ImageJ protocol for calculating Western blot band intensity: (I) open the file; (II) convert the image to grayscale: go to “Image > Type” and select “8-bit”; (III) remove background: go to “Process > Subtract Background”, and set the rolling ball radius to 50; (IV) set measurement parameters: go to “Analyze > Set Measurements”, and check “Area”, “Mean Gray Value”, and “Integrated Density”; (V) set the unit: go to “Analyze > Set Scale”, and enter “pixels” in the “Unit of length” box; (VI) invert the image: go to “Edit > Invert”; (VII) use the “Freehand Selection” tool to outline the band, then press “m” on the keyboard to get the “Integrated Density” value; (VIII) select the next band with the “Freehand Selection” tool and press “m” to measure; and (IX) after measuring all bands, go to “Results”, select “Edit > Select All”, and copy the “Integrated Density” data into an Excel sheet for analysis.
Cell transient transfection
In this study, two human RCC cell lines, Caki-1, and 786-O were purchased from Wuhan Pricella Biotechnology Co., Ltd. (Wuhan, China), and were cultured in McCoy’s 5A or RPMI-1640 medium (Bio-Channel, Nanjing, China) supplemented with 10% fetal bovine serum (ThermoFisher) and maintained in a humidified incubator at 37 ℃ with 5% CO2. Transfections were performed using GP-transfect-Mate (GenePharma, Suzhou, China) according to the manufacturer’s instructions, with both negative control (NC) and CDCA3 small interfering RNA (siRNA) (RiboBio, Guangzhou, China) introduced into RCC cells.
The sequence details are provided in Table S3.
In vitro assays
Caki-1 and 786-O cell lines were used to examine the functional role of CDCA3, with the si-NC group serving as the NC. The methodologies employed in our study are briefly summarized below, with further details available in our previously published work (16).
Cell Counting Kit-8 (CCK-8) assay
In a 96-well plate, each well was prepared with 100 µL of medium containing approximately 4×103 transiently transfected cells distributed across five replicate wells. After incubation periods of 24, 48, 72, and 96 hours, 100 µL of CCK-8 solution diluted 1:9 in medium was added to each well. After a further 2-hour incubation, optical density (OD) at 450 nm was measured using a spectrophotometer.
Colony formation assay
Cells were seeded at a density of 1,000 cells per well in a 6-well plate and cultured at 37 ℃ in a 5% CO2 incubator for 2 weeks. At the endpoint, colonies were washed twice with cold phosphate-buffered saline (PBS), fixed with 4% paraformaldehyde for 15 minutes, and stained with 1% crystal violet for 20 minutes at room temperature. Visible colonies were then counted by ImageJ. Here is a detailed protocol: (I) open the image colony formation assay image in ImageJ; (II) go to “Image > Type”, and select “8-bit”; (III) go to “Image > Adjust > Threshold”, and adjust the sliders to highlight the colonies, ensuring only the colonies are selected; (IV) go to “Analyze > Set Measurements”, Check “Area”, “Mean Gray Value”, and “Integrated Density”, then click “OK”; (V) go to “Analyze > Analyze Particles”, set the size and circularity parameters to exclude noise and select colonies, check “Display results”, “Clear results”, “Summarize”, and “Add to Manager”, then click “OK”; and (VI) inspect the results table that appears, which shows the measurements for each colony.
Transwell assay
To assess cell migration, approximately 4×104 transiently transfected cells were seeded in the upper chamber of a Transwell apparatus in serum-free medium, with complete medium added to the lower chamber. Cells were maintained at 37 ℃ in a 5% CO2 atmosphere for 48 hours, followed by washing with saline and fixation with paraformaldehyde. Cells were subsequently stained with 0.1% crystal violet, and stained cells were imaged and counted under a microscope.
Mutation landscape
The differential genomic mutations between high and low expression groups divided by the median expression of CDCA3 were assessed using the “maftools” R package (version 2.16) (17). The differences were evaluated using the Wilcoxon rank-sum test to ascertain statistical significance.
Immune infiltration
Immune infiltration in RCC (KIRC, KIRP, and KICH) from TCGA was analyzed using the xCell algorithm within the “IOBR” R package (version 0.99.9) (18). The correlation between CDCA3 expression and immune cell infiltration was subsequently evaluated using Spearman’s correlation coefficient.
Statistical analysis
Statistical analyses and graphical representations were conducted using R software version 4.3.1. Visualizations of certain datasets were facilitated by the Sanger Box bioinformatics analysis online tool (http://sangerbox.com/home.html). Experimental data were analyzed and plotted using GraphPad Prism version 8.0. The Wilcoxon rank-sum test (for non-normal distributions) or Student’s t-test (for normal distributions) was used to assess differences between the two groups. For comparisons among multiple groups, the Kruskal-Wallis test (for non-normal distributions) or one-way analysis of variance (ANOVA) (for normal distributions) was employed. A P value of less than 0.05 was considered indicative of a significant difference in medians between the two samples, or that the median of one sample is significantly different from the others.
Results
The expression landscape of CDCA3
Within normal human tissues, an analysis using data from the Genotype-Tissue Expression (GTEx) and Human Protein Atlas (HPA) cohorts indicated that CDCA3 exhibited higher RNA expression levels in the testis and small intestine, whereas its expression in the kidney was lower than in most other organs (Figure 2A). In the pan-cancer cell line RNA expression profiles from the Cancer Cell Line Encyclopedia (CCLE), CDCA3 showed moderate expression levels in renal cancers (Figure 2B). Furthermore, differential expression analysis of matched tumor samples from TCGA, excluding kidney KICH, showed elevated expression of CDCA3 in both KIRC and KIRP, as well as higher expression in most other cancer types (Figure 2C). Similar patterns were also observed in unmatched tissue differential analysis within TCGA (Figure 2D). Moreover, CDCA3 expression tended to increase with tumor-node-metastasis (TNM) stage in RCC (Figure S1). These findings suggested that CDCA3 possesses potential as a biomarker for RCC.
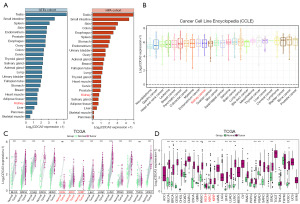
CDCA3’s prognostic value
We subsequently conducted a prognostic value analysis of CDCA3 within the pan-RCC cohort from TCGA, using overall survival (OS) and progression-free interval (PFI) as prognostic endpoints. The results revealed that in KIRC, KICH, and KIRP, Kaplan-Meier survival curves consistently demonstrated higher mortality rates in groups with elevated CDCA3 expression (Figure 3A-3C). Additionally, receiver operating characteristic (ROC) analyses at three distinct time points predominantly showed CDCA3’s area under the curve (AUC) values exceeding 0.7, with only slightly lower values in KIRC (Figure 3D-3F). Subsequently, we included gender, age, pathologic stage, overall stage, and CDCA3 expression for univariate and multivariate Cox regression analysis. The results indicated that CDCA3 as an independent risk factor for OS across different types of RCC (Figure 3G, Table S4). Similarly, this association was observed at the additional prognostic endpoint of PFI (Figure 3G). Collectively, these findings established CDCA3 as a prognostic risk factor in pan-RCC.
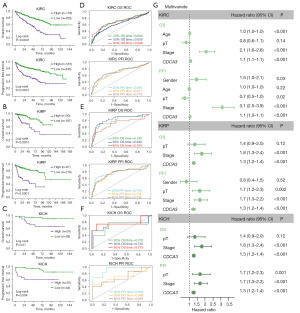
IHC of CDCA3 in clinical tissues
The initial findings, predominantly derived from RNA expression levels in public datasets, prompted further investigation into the detectability of CDCA3 at the protein level in clinical samples. Results indicated that CDCA3 was primarily localized in the cytoplasm with occasional staining in the nucleus across KIRC, KIRP, and KICH (Figure 4 and Figure S2). Generally, the expression of CDCA3 was higher in RCC than in adjacent non-cancerous tissues. This expression pattern supported the potential of CDCA3 as a biomarker for RCC.
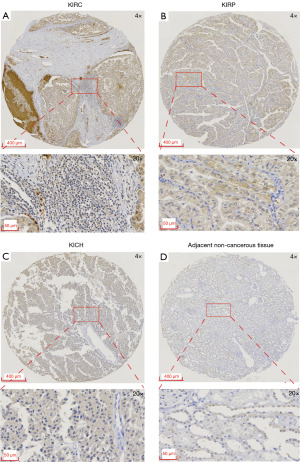
Oncogene effect of CDCA3 in vitro
In the following study, we explored the impact of CDCA3 on the phenotypic characteristics of RCC cell lines through in vitro assays. Utilizing loss-of-function assays, we validated the functional role of CDCA3 in RCC cells. As depicted in Figure 5A, siRNA1 and siRNA2 effectively reduced the expression of CDCA3 in Caki-1 and 786-O cell lines. Subsequent CCK-8 assays demonstrated that downregulation of CDCA3 inhibited the proliferative activity of Caki-1 and 786-O (Figure 5B). Clone formation assays further indicated that CDCA3 downregulation significantly reduced the clonal formation of Caki-1 and 786-O cells (Figure 5C). Additionally, Transwell invasion assays revealed that knockdown of CDCA3 markedly inhibited the invasive capabilities of RCC cells (Figure 5D). Collectively, these results suggested that CDCA3 acted as an oncogene in RCC.
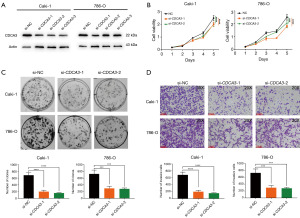
Functional enrichment analysis
To explore the biological functions of CDCA3, we employed GSEA to analyze different pathways in KEGG, GO, and Hallmark. We completed enrichment analyses in separate datasets for KIRC, KIRP, and KICH. The results were presented as the top 25 pathways based on the average absolute values of normalized enrichment scores (NESs) for each dataset, aiming to identify common mechanistic themes of CDCA3 in RCC. As depicted in Figure 6, the top five activated pathways included E2F targets, single-stranded DNA helicase activity, negative regulation of metaphase-anaphase transition of cell cycle, regulation of mitotic sister chromatid segregation, and negative regulation of nuclear division. The top five inhibited pathways were ammonium ion metabolic process, proximal tubule bicarbonate reclamation, major histocompatibility complex (MHC) class II protein complex, MHC protein complex, and short-chain fatty acid metabolic process. These enriched pathways aligned with the prevailing understanding of CDCA3 as a regulator of the cell cycle.
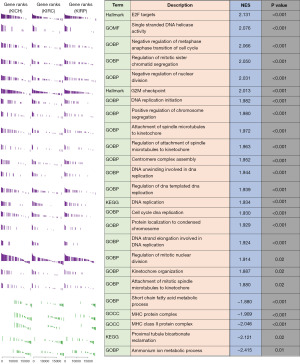
CDCA3 was a biomarker for immunotherapy in RCC
Based on the results of functional enrichment analysis, we observed that CDCA3 suppressed several immune pathways, such as the MHC class II protein complex and MHC protein complex. This led us to hypothesize that CDCA3 might play a crucial role in the immune microenvironment of RCC. A previous study revealed a positive correlation between CDCA3 expression and CD8+ T cell infiltration, and this correlation was also observed in our results (11) (Figure S3). However, we interestingly found a significant positive correlation between CDCA3 expression and Th2 cells in KIRC, KIRP, and KICH (Figure 7A). Previous studies suggest that Th2 cells may hinder antitumor immunity, prompting us to investigate potential differences in immune therapy responses between high and low CDCA3 expression groups using Submap analysis. Our findings suggest that patients with low CDCA3 expression in the KIRC and KIRP cohorts responded better to programmed cell death protein 1 (PD-1) therapy, although this trend was not observed in the KICH cohort (Figure 7B). To validate these observations, we conducted analyses in two advanced RCC immunotherapy cohorts. In the nivolumab cohort, the high CDCA3 expression group exhibited lower OS and progression-free survival (PFS) rates (Figure 7C). Similarly, in the avelumab cohort (avelumab + axitinib), the high CDCA3 expression group showed a lower PFS rate (Figure 7C). These cohort data suggested that high CDCA3 expression correlated with poor immune therapy outcomes, highlighting its potential as a biomarker for immunotherapy in RCC.
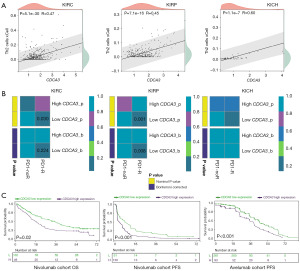
CDCA3 and genetic mutations
In our subsequent analysis, we explored the role of CDCA3 from the perspective of genetic mutations. We divided the samples into two groups based on the median expression of CDCA3 in KIRC, KIRP, and KICH. Our findings indicated that in KIRC, VHL and PBRM1 exhibited the highest mutation frequencies, at 44% and 40%, respectively (Figure 8A). However, the group with higher CDCA3 expression showed increased mutation frequencies of SETD2 and BAP1 (Figure 8A). In KICH, TP53 displayed the highest mutation frequency at 31%, with the high CDCA3 expression group showing a higher mutation frequency of PTEN (Figure 8B). In KIRP, the most frequently mutated gene was TTN, at 14%, although there was no significant difference in mutation frequency between the CDCA3 expression groups (Figure 8C). Overall, in RCC, higher expression of CDCA3 is associated with a more unfavorable mutational landscape.
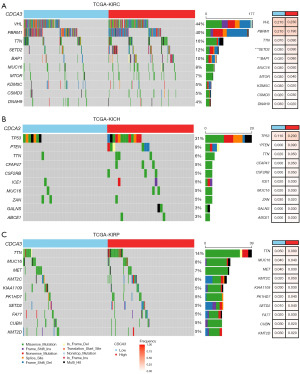
Discussion
RCC represents the predominant malignancy of the upper urinary tract within the urinary system, comprising 2% of adult malignancies. Nonetheless, the majority of RCC variants exhibit resistance to conventional radiotherapy and chemotherapy. Beyond surgical interventions, achieving effective therapeutic results with current treatment approaches remains challenging. Consequently, identifying sensitive biomarkers and specific therapeutic targets for personalized treatment strategies holds significant clinical relevance in elevating survival rates for RCC patients.
The expression levels of CDCA mRNA vary significantly across different cancer types compared to normal tissues, highlighting the role of CDCA family genes in cell proliferation—a fundamental characteristic of cancer progression. These genes are implicated in recurrence and poorer prognosis across various cancers. Notably, CDCA3 functions within the SCF ubiquitin ligase complex as a key regulator of the cell cycle (19). Our functional enrichment analysis further supports this role, showing that E2F targets, the G2M checkpoint, and DNA replication processes are activated in groups with high CDCA3 expression. Specifically, CDCA3 is essential for the appropriate activation of CDK1/cyclin B and the initiation of mitosis. Its degradation during the G1 phase facilitates the accumulation of WEE1 in interphase, linking the anaphase-promoting complex to SCF pathways (20). This interaction is crucial for regulating CDK1/cyclin B activity, thereby coordinating mitotic entry and exit, and influencing both physiological and pathological cellular processes through the regulation of downstream cytokines, hormones, and proteins (21).
As depicted in Figure 2C,2D, CDCA3 expression is elevated in various tumor types. Previous research has consistently demonstrated that CDCA3 is critically involved in the initiation and progression of multiple cancers, including gastric, colorectal, non-small cell lung, bladder, breast, prostate cancer, and leukemia. In gastric cancer, increased levels of CDCA3 correlate with poor patient outcomes. Furthermore, both in vivo and in vitro studies indicate that CDCA3 overexpression enhances the proliferative and colony-forming capacities of gastric cancer cells (22). In colorectal cancer, upregulation of CDCA3 boosts cell proliferation, whereas downregulation diminishes it in both experimental and clinical settings (23). For non-small cell lung cancer, elevated CDCA3 expression is significantly associated with adverse prognostic outcomes (24). In bladder cancer, CDCA3 overexpression is prognostically significant (25), and its high levels are similarly linked with decreased survival rates in breast cancer (26). Additionally, in prostate cancer, patients exhibiting higher CDCA3 levels tend to experience poorer clinical results (27). It functions by repressing CDCA3 expression through the downregulation of HOXB3, subsequently inhibiting cell proliferation (28).
In our analysis of public datasets, CDCA3 exhibits lower expression at the RNA level in benign tissues, whereas its expression is elevated in RCC. Similarly, IHC of our clinical samples demonstrates that CDCA3 is also expressed at higher levels in RCC at the protein level. The distinct expression profile of CDCA3 ensures its detectability as a biomarker in clinical tissues.
Immunotherapy has emerged in recent years as a novel treatment modality, offering new therapeutic options for patients with various solid tumors. Among these, some patients with RCC have benefited, although it does not prove effective for all individuals. Identifying patients who are more likely to respond to immunotherapy is critical. Recent advances in tumor immunology have further highlighted the significance of exhausted T cells and tertiary lymphoid structures (TLSs) in influencing immunotherapy outcomes. Exhausted T cells, characterized by reduced functionality due to prolonged antigen exposure, can significantly limit the efficacy of immune checkpoint blockade (ICB), particularly in RCC (29). In parallel, the formation of TLS, which serve as specialized sites for antigen presentation and T cell activation, has been associated with improved responses to immunotherapy (30).
Interestingly, in our functional enrichment analysis, we found that CDCA3 suppresses several immune pathways including MHC class II protein complex. MHC class II was primarily expressed by dendritic cells, macrophages, and B cells. They mainly present exogenous antigens to CD4+ T cells, thereby activating the immune response (31). High expression of CDCA3 in tumors may indicate immune evasion. Additionally, immune cell infiltration analysis revealed a strong positive correlation between CDCA3 and Th2 cells in RCC. Th2 cells play a pivotal role in humoral immunity by upregulating antibody production against extracellular pathogens. Evidence suggests that Th2 cell infiltration in the tumor microenvironment (TME) can promote tumor growth (32). For example, inhibition of Th2 cytokines was found to increase the infiltration and proliferation of cytotoxic CD8+ T cells, as well as enhancing their cytotoxic cytokine secretion, thereby activating antitumor T cell immunity (33). Similarly, in our analysis of advanced RCC immunotherapy cohorts, we observed that high CDCA3 expression correlates with poor immune therapy outcomes. This finding leads us to suspect that high Th2 cell infiltration associated with CDCA3 may contribute to reduced immune therapy responses. The balance and cytokine secretion of Th1 and Th2 cells may shift after ICB therapy in a cancer-dependent manner (34,35).
While our study provides valuable insights into the role of CDCA3 in RCC, several limitations must be acknowledged. First, the expression of CDCA3 may vary significantly across different RCC subtypes. The exclusive use of clear cell RCC (ccRCC) cell lines and patient cohorts in this study may limit the generalizability of our findings. Moreover, genetic and environmental factors could further modulate CDCA3 expression, influencing its role in RCC progression and therapy response. For example, the relationship between CDCA3 and Th2 cells, as suggested by our findings, warrants further investigation to fully delineate these effects. A recent study has highlighted the importance of certain biomarkers and immune signatures in predicting the efficacy of ICB in various cancers (36). Additionally, the use of novel imaging technologies may improve our capacity to monitor immune-related changes in vivo, offering a more precise assessment of therapy response (37). Thus, future research should focus on integrating these advanced biomarkers and imaging techniques into the study of CDCA3 in RCC, potentially providing a more comprehensive framework for personalized treatment strategies and improving patient outcomes.
Conclusions
In conclusion, our research highlights CDCA3’s role as a prognostic and immunotherapeutic biomarker for RCC. These findings have significant implications for prognostic risk assessments and inform the development of targeted therapies and immunomodulatory strategies for RCC.
Acknowledgments
Funding: The project was supported by grants from
Footnote
Reporting Checklist: The authors have completed the MDAR reporting checklist. Available at https://tau.amegroups.com/article/view/10.21037/tau-24-233/rc
Data Sharing Statement: Available at https://tau.amegroups.com/article/view/10.21037/tau-24-233/dss
Peer Review File: Available at https://tau.amegroups.com/article/view/10.21037/tau-24-233/prf
Conflicts of Interest: All authors have completed the ICMJE uniform disclosure form (available at https://tau.amegroups.com/article/view/10.21037/tau-24-233/coif). The authors have no conflicts of interest to declare.
Ethical Statement: The authors are accountable for all aspects of the work in ensuring that questions related to the accuracy or integrity of any part of the work are appropriately investigated and resolved. The study was conducted in accordance with the Declaration of Helsinki (as revised in 2013).
Open Access Statement: This is an Open Access article distributed in accordance with the Creative Commons Attribution-NonCommercial-NoDerivs 4.0 International License (CC BY-NC-ND 4.0), which permits the non-commercial replication and distribution of the article with the strict proviso that no changes or edits are made and the original work is properly cited (including links to both the formal publication through the relevant DOI and the license). See: https://creativecommons.org/licenses/by-nc-nd/4.0/.
References
- Ljungberg B, Albiges L, Abu-Ghanem Y, et al. European Association of Urology Guidelines on Renal Cell Carcinoma: The 2019 Update. Eur Urol 2019;75:799-810. [Crossref] [PubMed]
- Shuch B, Amin A, Armstrong AJ, et al. Understanding pathologic variants of renal cell carcinoma: distilling therapeutic opportunities from biologic complexity. Eur Urol 2015;67:85-97. [Crossref] [PubMed]
- Siegel RL, Miller KD, Wagle NS, et al. Cancer statistics, 2023. CA Cancer J Clin 2023;73:17-48. [Crossref] [PubMed]
- Motzer RJ, Choueiri TK, Hutson T, et al. Characterization of Responses to Lenvatinib plus Pembrolizumab in Patients with Advanced Renal Cell Carcinoma at the Final Prespecified Survival Analysis of the Phase 3 CLEAR Study. Eur Urol 2024;86:4-9. [Crossref] [PubMed]
- DeLuca JG, Moree B, Hickey JM, et al. hNuf2 inhibition blocks stable kinetochore-microtubule attachment and induces mitotic cell death in HeLa cells. J Cell Biol 2002;159:549-55. [Crossref] [PubMed]
- DeLuca JG, Howell BJ, Canman JC, et al. Nuf2 and Hec1 are required for retention of the checkpoint proteins Mad1 and Mad2 to kinetochores. Curr Biol 2003;13:2103-9. [Crossref] [PubMed]
- Ladurner R, Kreidl E, Ivanov MP, et al. Sororin actively maintains sister chromatid cohesion. EMBO J 2016;35:635-53. [Crossref] [PubMed]
- Watrin E, Demidova M, Watrin T, et al. Sororin pre-mRNA splicing is required for proper sister chromatid cohesion in human cells. EMBO Rep 2014;15:948-55. [Crossref] [PubMed]
- Hindriksen S, Meppelink A, Lens SM. Functionality of the chromosomal passenger complex in cancer. Biochem Soc Trans 2015;43:23-32. [Crossref] [PubMed]
- Li H, Li M, Yang C, et al. Prognostic value of CDCA3 in kidney renal papillary cell carcinoma. Aging (Albany NY) 2021;13:25466-83. [Crossref] [PubMed]
- Bai Y, Liao S, Yin Z, et al. CDCA3 Predicts Poor Prognosis and Affects CD8(+) T Cell Infiltration in Renal Cell Carcinoma. J Oncol 2022;2022:6343760. [Crossref] [PubMed]
- Li F, Wu Z, Du Z, et al. Comprehensive molecular analyses and experimental validation of CDCAs with potential implications in kidney renal papillary cell carcinoma prognosis. Heliyon 2024;10:e33045. [Crossref] [PubMed]
- Goldman MJ, Craft B, Hastie M, et al. Visualizing and interpreting cancer genomics data via the Xena platform. Nat Biotechnol 2020;38:675-8. [Crossref] [PubMed]
- Yu G, Wang LG, Han Y, et al. clusterProfiler: an R package for comparing biological themes among gene clusters. OMICS 2012;16:284-7. [Crossref] [PubMed]
- Zhong C, Long Z, Yang T, et al. M6A-modified circRBM33 promotes prostate cancer progression via PDHA1-mediated mitochondrial respiration regulation and presents a potential target for ARSI therapy. Int J Biol Sci 2023;19:1543-63. [Crossref] [PubMed]
- Lu J, Zhong C, Luo J, et al. HnRNP-L-regulated circCSPP1/miR-520h/EGR1 axis modulates autophagy and promotes progression in prostate cancer. Mol Ther Nucleic Acids 2021;26:927-44. [Crossref] [PubMed]
- Mayakonda A, Lin DC, Assenov Y, et al. Maftools: efficient and comprehensive analysis of somatic variants in cancer. Genome Res 2018;28:1747-56. [Crossref] [PubMed]
- Zeng D, Ye Z, Shen R, et al. IOBR: Multi-Omics Immuno-Oncology Biological Research to Decode Tumor Microenvironment and Signatures. Front Immunol 2021;12:687975. [Crossref] [PubMed]
- Tsunekawa Y, Hasegawa T, Nadai M, et al. Interspecies differences and scaling for the pharmacokinetics of xanthine derivatives. J Pharm Pharmacol 1992;44:594-9. [Crossref] [PubMed]
- Li R, Chen Y, Yang B, et al. Integrated bioinformatics analysis and experimental validation identified CDCA families as prognostic biomarkers and sensitive indicators for rapamycin treatment of glioma. PLoS One 2024;19:e0295346. [Crossref] [PubMed]
- Ayad NG, Rankin S, Murakami M, et al. Tome-1, a trigger of mitotic entry, is degraded during G1 via the APC. Cell 2003;113:101-13. [Crossref] [PubMed]
- Zhang Y, Yin W, Cao W, et al. CDCA3 is a potential prognostic marker that promotes cell proliferation in gastric cancer. Oncol Rep 2019;41:2471-81. [Crossref] [PubMed]
- Qian W, Zhang Z, Peng W, et al. CDCA3 mediates p21-dependent proliferation by regulating E2F1 expression in colorectal cancer. Int J Oncol 2018;53:2021-33. [Crossref] [PubMed]
- Adams MN, Burgess JT, He Y, et al. Expression of CDCA3 Is a Prognostic Biomarker and Potential Therapeutic Target in Non-Small Cell Lung Cancer. J Thorac Oncol 2017;12:1071-84. [Crossref] [PubMed]
- Li S, Liu X, Liu T, et al. Identification of Biomarkers Correlated with the TNM Staging and Overall Survival of Patients with Bladder Cancer. Front Physiol 2017;8:947. [Crossref] [PubMed]
- Phan NN, Wang CY, Li KL, et al. Distinct expression of CDCA3, CDCA5, and CDCA8 leads to shorter relapse free survival in breast cancer patient. Oncotarget 2018;9:6977-92. [Crossref] [PubMed]
- Gu P, Zhang M, Chen X, et al. Prognostic value of cell division cycle-associated protein-3 in prostate cancer. Medicine (Baltimore) 2023;102:e34655. [Crossref] [PubMed]
- Bi L, Zhou B, Li H, et al. A novel miR-375-HOXB3-CDCA3/DNMT3B regulatory circuitry contributes to leukemogenesis in acute myeloid leukemia. BMC Cancer 2018;18:182. [Crossref] [PubMed]
- Ficial M, Jegede OA, Sant'Angelo M, et al. Expression of T-Cell Exhaustion Molecules and Human Endogenous Retroviruses as Predictive Biomarkers for Response to Nivolumab in Metastatic Clear Cell Renal Cell Carcinoma. Clin Cancer Res 2021;27:1371-80. [Crossref] [PubMed]
- Xu W, Lu J, Liu WR, et al. Heterogeneity in tertiary lymphoid structures predicts distinct prognosis and immune microenvironment characterizations of clear cell renal cell carcinoma. J Immunother Cancer 2023;11:e006667. [Crossref] [PubMed]
- Steier Z, Kim EJY, Aylard DA, et al. The CD4 Versus CD8 T Cell Fate Decision: A Multiomics-Informed Perspective. Annu Rev Immunol 2024;42:235-58. [Crossref] [PubMed]
- Koyasu S, Moro K. Type 2 innate immune responses and the natural helper cell. Immunology 2011;132:475-81. [Crossref] [PubMed]
- Loeuillard E, Yang J, Buckarma E, et al. Targeting tumor-associated macrophages and granulocytic myeloid-derived suppressor cells augments PD-1 blockade in cholangiocarcinoma. J Clin Invest 2020;130:5380-96. [Crossref] [PubMed]
- Protti MP, De Monte L. Cross-talk within the tumor microenvironment mediates Th2-type inflammation in pancreatic cancer. Oncoimmunology 2012;1:89-91. [Crossref] [PubMed]
- Saulite I, Ignatova D, Chang YT, et al. Blockade of programmed cell death protein 1 (PD-1) in Sézary syndrome reduces Th2 phenotype of non-tumoral T lymphocytes but may enhance tumor proliferation. Oncoimmunology 2020;9:1738797. [Crossref] [PubMed]
- Crocetto F, Ferro M, Buonerba C, et al. Comparing cardiovascular adverse events in cancer patients: A meta-analysis of combination therapy with angiogenesis inhibitors and immune checkpoint inhibitors versus angiogenesis inhibitors alone. Crit Rev Oncol Hematol 2023;188:104059. [Crossref] [PubMed]
- Tataru OS, Marchioni M, Crocetto F, et al. Molecular Imaging Diagnosis of Renal Cancer Using (99m)Tc-Sestamibi SPECT/CT and Girentuximab PET-CT-Current Evidence and Future Development of Novel Techniques. Diagnostics (Basel) 2023;13:593. [Crossref] [PubMed]