Developing and validating risk predicting models to assess venous thromboembolism risk after radical cystectomy
Highlight box
Key findings
• This study created new prediction models that were superior to the Caprini risk assessment model for predicting the risk of venous thromboembolism (VTE) after radical cystectomy (RC).
What is known and what is new?
• The Caprini risk score has long been regarded as a universal tool for assessing the risk of thrombotic events in hospitalized patients.
• We constructed the new nomograms for patients after RC, including body mass index, tumor diameter, tumor grade, preoperative fibrinogen, and postoperative platelet, etc. to perfect the Caprini risk score.
What is the implication, and what should change now?
• Now Caprini risk score with our nomograms provides a more accurate assessment of the risk of VTE in patients after RC.
Introduction
Globally, bladder cancer ranks among the top ten most common cancer types, with an estimated 550,000 new cases being reported each year (1). Radical cystectomy (RC) remains the gold standard for treating muscle-invasive bladder cancer (MIBC) and some high-risk non-MIBC (NMIBC) (2). As one of the most extensive surgeries in urology, RC is often plagued by postoperative complications of various levels of severity, which inevitably increase the total hospitalization costs, and in the worst scenario, even become life-threatening (3).
One potentially severe complication after RC is venous thromboembolism (VTE), which has been well-described and clinically consists of deep vein thrombosis (DVT) and pulmonary embolism (PE). It is true that the overall morbidity rate after urologic surgery is relatively low, compared with other specialties (4). However, VTE incidence is highest in patients undergoing RC among major urologic procedures, and this is made worse by recent research showing that incidents of VTE following RC are well under-reported (5). VTE occurrence not only prolongs length of stay and aggravates the economic burden of patients and strains the healthcare system (6), but is also associated with increased mortality in patients with bladder cancer (7).
The application of pharmacologic thromboprophylaxis in urologic surgery may reduce the risks of VTE, however the European Association of Urology (EAU) guidelines specify that the benefits should be weighed against the increased risks of bleeding (8). Therefore, a balance between the risks of perioperative bleeding vs. thromboembolic events must be carefully considered in order to minimize the occurrence of potentially catastrophic side effects and maximize the benefits for patients.
While the risk of hemorrhage is quite individualized among patients, the risk of VTE could be standardized. In fact, there are several predictive tools for estimating the risk of VTE, including the widely adopted Caprini risk score. However, those tools are generalized tools for inpatients to evaluate the risk of thrombotic events, and not specifically designed for surgical patients with malignancy, much less than those after RC. At the same time, different tumors carry different risks for VTE (9). Therefore, it is critical to introduce a model specially targeting bladder cancer to precisely evaluate the risk of VTE and therefore guide clinical decision-making regarding anticoagulant therapy.
Nomograms represent the probability of occurrence of predicted events in order to guide clinical decisions. Nomograms have been shown to be effective in predicting post-surgical survival in patients with malignant tumors in previous studies (10-12). We aimed to develop a model that would predict the risk of VTE in bladder cancer patients after RC. The proposed nomogram highlights patients with increased risks for thrombosis and will aid urologists to make more targeted clinical decisions, which may help mitigate the risk of post-RC thrombosis without increasing bleeding risk. We present this article in accordance with the TRIPOD reporting checklist (available at https://tau.amegroups.com/article/view/10.21037/tau-24-194/rc).
Methods
Study design and patient selection
Study participants included 272 patients receiving RC at the Peking University People’s Hospital from January 1, 2010 to December 31, 2019. The inclusion criteria were as follows: (I) patients pathologically diagnosed with bladder cancer and underwent RC in Peking University People’s Hospital; and (II) patients with complete clinical data. The exclusion criteria included the following: (I) patients diagnosed with VTE before RC; (II) patients with concurrent cancer besides bladder cancer; (III) patients with previous hematological diseases; and (IV) patients with primary or secondary coagulation disorders. Cystoscopic biopsy histopathology was used to diagnose bladder cancer in all patients included in this study. The diagnosis of VTE was based on color duplex ultrasound and computerized tomography pulmonary angiography (CTPA). A lower limb ultrasound was administered to those with VTE-related symptoms or continually increased D-dimer (D-dimer levels were tested the day following surgery; if the D-dimer value exceeds three times the upper limit of normal, further daily retesting and monitoring will be conducted) and CTPA was further administered to patients suspected of PE. All patients received early mechanical prophylaxis right after RC until ambulation during the postoperative period. The application of pharmacological anticoagulation was at the discretion of attending surgeons, usually initiated without potential contraindication such as postoperative hemorrhage and terminated at discharge.
Using computer-generated random numbers, patients were randomly assigned in a ratio of 4:1 to training cohorts (n=220) and testing cohorts (n=52). Additionally, patients were followed up for 1 month after surgery and categorized into VTE and non-VTE groups based on whether a VTE event occurred during the follow-up period.
The study was conducted in accordance with the Declaration of Helsinki (as revised in 2013) and approved by the ethical committee of Peking University People’s Hospital (No. 2023PHB246-001). As the data are anonymous, informed consent was not required.
Data collection
The selection of prospective clinical variables was based on existing literature, ease of access, as well as clinical experience (13,14). After a comprehensive review of the medical records, the following data were collected retrospectively: the demographic information and medical history [including age, sex, body mass index (BMI), smoking, comorbidities including hypertension, diabetes mellitus, hyperlipidemia, coronary artery disease (CAD), chronic obstructive pulmonary disease (COPD), etc.]; laboratory examination [coagulation index, platelet (PLT) count, D-dimer, etc.]; surgical information (including operation approach, urinary diversion approach, lymph node dissection, duration of operation, intraoperative blood loss, blood transfusion, etc.); pathological characteristics of tumor [including T stage, N stage, grade, tumor diameter, tumor classification (primary, secondary), amount of tumor (single and multiple), etc.]. Tumor diameter was evaluated by imaging (mainly pelvic computed tomography) and describes the maximum diameter of the tumor, whereas if multiple lesions were present, the maximum diameter of the largest one. Additionally, the Caprini risk assessment (CRA) was performed for all patients after surgery to assess the risk of postoperative VTE.
Model building
We began by performing univariate logistic regression on potential variables. Significant variables were subjected to multivariate logistic regression to identify independent risk factors. These factors were used to create a nomogram model. In parallel, we built an optimal model using stepwise logistic regression from the ‘MASS’ package and obtained clinical variables for model construction.
For the two new models constructed either by univariate and multivariate logistic regression analysis (Model A) or stepwise logistic regression analysis (Model B), their calibration and discrimination were calculated in the training dataset. To compare discrimination, a receiver operating characteristic (ROC) curve and the area under the curves (AUCs) were constructed, and the Brier score was calculated in order to assess calibration (15). Then two new models were weighed against the CRA model with regard to AUC, net reclassification improvement (NRI), and integrated discrimination improvement (IDI) in order to evaluate its predictive capabilities, and this was analyzed using “pROC”, “nricens”, and “PredictABEL” packages. Validation was performed using the Bootstrap method with 1,000 resamples in addition to an internal validation using the testing cohort. The RMS package was used for computing the concordance index (C-index) (16). Lastly, two nomograms were constructed for each of the regression methods described above.
Statistical analysis
Only variables with over 70% intact values were included in the study and predictive mean matching was used to fill in missing data. Measurements are presented as mean and standard deviation or median and interquartile range, while counts are represented by numbers (percentages). Statistical comparisons involved the two-tailed unpaired samples t-test for measurement data and the Chi-squared test or Fisher’s exact test for count data. A two-sided P value of less than 0.05 was considered statistically significant. All analyses were performed using SPSS (version 26.0) and R software (version 4.1.0).
Results
Baseline and clinical characteristics of patients enrolled
Table 1 outlines the baseline characteristics through a univariate analysis of the entire cohort. Among the patients, 36 (13.2%) experienced VTE, while the remaining 236 (86.8%) were grouped as non-VTE cases. The detailed procedure for patient selection is presented in Figure 1. In comparison with the non-VTE group, the VTE group presented with a higher BMI (25.55 vs. 24.05 kg/m2, P=0.001) and higher rates of hypertension (69.4% vs. 42.8%, P=0.003). With regard to laboratory test, the VTE group had a lower preoperative fibrinogen (pre-Fib) level (290.5 vs. 315 mg/dL, P=0.01), a higher postoperative D-dimer level (1,454 vs. 676.58 ng/mL, P<0.001) and a lower postoperative PLT (post-PLT) count [142.5 vs. 166 (×109/L), P=0.03], which usually indicated a state of coagulation disorder and increased likelihood to incur postoperative thrombotic events. In addition, the VTE group presented more carcinoma in situ (CIS) (27.8% vs. 13.6%, P=0.03), and less variant histology (0.0% vs. 24.2%, P<0.001) (Table 1).
Table 1
Characteristics | No VTE (n=236) | VTE (n=36) | Z/t/χ2 | P |
---|---|---|---|---|
Age (years) | 67.5 [61, 74] | 70 [67, 77] | −1.905 | 0.057 |
Sex (male) | 194 (82.2) | 25 (69.4) | 3.241 | 0.07 |
BMI (kg/m2) | 24.05 [22.2, 26.25] | 25.55 [23.83, 27.25] | −3.185 | 0.001 |
Smoking | 104 (44.1) | 11 (30.6) | 2.337 | 0.13 |
Hypertension | 101 (42.8) | 25 (69.4) | 8.920 | 0.003 |
Diabetic | 43 (18.2) | 11 (30.6) | 2.987 | 0.08 |
Hyperlipidemia | 114 (48.3) | 17 (47.2) | 0.015 | 0.90 |
CAD | 30 (12.7) | 7 (19.4) | – | 0.30† |
COPD | 74 (31.4) | 12 (33.3) | 0.056 | 0.81 |
NAC | 17 (7.2) | 3 (8.3) | – | 0.81 |
Preoperative | ||||
LDH (U/L) | 165 [146, 187] | 167.5 [156.25, 180.75] | −0.388 | 0.70 |
BUN (mmol/L) | 6.03 [4.98, 7.5] | 7.01 [4.87, 8.67] | −1.191 | 0.23 |
Creatinine (μmol/L) | 82.5 [69, 99] | 81.5 [71.25, 97.75] | −0.224 | 0.82 |
FBG (mmol/L) | 5.4 [4.86, 6.16] | 5.57 [5.14, 6.49] | −1.678 | 0.09 |
PT (s) | 11.10±0.87 | 11.24±0.91 | −0.892 | 0.37 |
APTT (s) | 30.4 [28.4, 31.88] | 29.3 [27.7, 30.9] | −1.942 | 0.052 |
Fib (mg/dL) | 315 [269, 375.75] | 290.5 [238.25, 342] | −2.549 | 0.01 |
Surgical information | ||||
Duration (h) | 6 [5, 7.5] | 6.75 [5.5, 8] | −1.406 | 0.16 |
Blood loss (mL) | 400 [250, 700] | 400 [262.5, 875] | −0.328 | 0.74 |
ASA | 3.654 | 0.30 | ||
I | 16 (6.8) | 1 (2.8) | ||
II | 168 (71.2) | 31 (86.1) | ||
III | 50 (21.2) | 4 (11.1) | ||
IV | 2 (0.8) | 0 (0.0) | ||
Blood transfusion | 57 (24.2) | 11 (30.6) | 0.683 | 0.41 |
Plasma (mL) | 0 [0, 0] | 0 [0, 200] | −1.214 | 0.23 |
RBC (mL) | 0 [0, 0] | 0 [0, 260] | −1.011 | 0.31 |
Surgical approach | 0.403 | 0.84† | ||
Open | 17 (7.2) | 3 (8.3) | ||
Laparoscopy | 190 (80.5) | 28 (77.8) | ||
Robot-assisted | 29 (12.3) | 5 (13.9) | ||
Urinary diversion | 1.079 | 0.58 | ||
Uretero-cutaneous diversion | 107 (45.3) | 13 (36.1) | ||
Ileum conduit urinary diversion | 84 (35.6) | 15 (41.7) | ||
Neobladder | 45 (19.1) | 8 (22.2) | ||
Postoperative | ||||
PLT (×109/L) | 166 [131.25, 201.75] | 142.5 [114, 191.5] | −2.249 | 0.03 |
D-dimer (ng/mL) | 676.58 [411.25, 1,113.75] | 1,454 [740.25, 2,692.75] | −4.342 | <0.001 |
Hospital duration (days) | 15.90 | 16.39 | – | 0.05 |
Clavien-Dindo complication | ||||
≥ grade 3 | 19 (8.1) | 5 (13.9) | – | 0.25 |
≤ grade 2 | 85 (36.0) | 15 (41.6) | – | 0.51 |
Anticoagulant therapy | 219 (92.8) | 36 (100.0) | – | 0.14 |
Tumor characteristics | ||||
Diameter (cm) | 2.97 [1.5, 4] | 2.97 [2, 4.38] | −0.852 | 0.39 |
Abnormal differentiation | 57 (24.2) | 0 (0.0) | – | <0.001† |
Origin | 0.415 | 0.81 | ||
Primary | 158 (66.9) | 26 (72.2) | ||
Secondary | 78 (33.1) | 10 (27.8) | ||
Amount | 1.079 | 0.30 | ||
Single | 107 (45.3) | 13 (36.1) | ||
Multiple | 129 (54.7) | 23 (63.9) | ||
Grade | – | 0.79 | ||
Low | 30 (12.7) | 5 (13.9) | ||
High | 206 (87.3) | 31 (86.1) | ||
T stage | 1.428 | 0.49 | ||
T0/T1/Ta | 94 (39.8) | 18 (50.0) | ||
T2 | 81 (34.3) | 11 (30.6) | ||
T3/T4 | 61 (25.8) | 7 (19.4) | ||
N stage | 5.298 | 0.15† | ||
0 | 215 (91.1) | 31 (86.1) | ||
1 | 7 (3.0) | 4 (11.1) | ||
2 | 13 (5.5) | 1 (2.8) | ||
3 | 1 (0.4) | 0 (0.0) | ||
BVI and LVI | 45 (19.1) | 7 (19.4) | 0.003 | 0.96 |
With CIS | 32 (13.6) | 10 (27.8) | 4.836 | 0.03 |
Data are presented as median [IQR], n (%), mean ± SD, or mean. †, Fisher’s exact test. RC, radical cystectomy; VTE, venous thromboembolism; BMI, body mass index; CAD, coronary artery disease; COPD, chronic obstructive pulmonary disease; NAC, neoadjuvant chemotherapy; LDH, lactate dehydrogenase; BUN, blood urea nitrogen; FBG, fasting blood glucose; PT, prothrombin time; APTT, activated partial thromboplastin time; Fib, fibrinogen; ASA, American Society of Anesthesiologists; RBC, red blood cell; PLT, platelet; BVI, blood vessel invasion; LVI, lymphovascular invasion; CIS, carcinoma in situ.
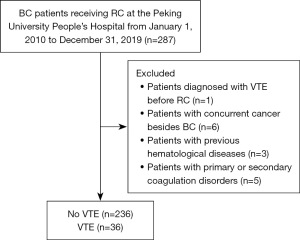
The risk factors for modeling
To prevent overfitting, only factors with P<0.05 were included in multivariate regression analyses and two different models were developed. The multivariate analysis identified BMI (P=0.03), hypertension (P=0.005), pre-Fib (P<0.001), and post-PLT (P=0.02) as independent predictors of VTE following RC (Table 2).
Table 2
Variables | Model A | Model B | |||||
---|---|---|---|---|---|---|---|
Estimate | SE | P | Estimate | SE | P | ||
Sex | |||||||
Female | 1.554 | 0.747 | 0.04 | ||||
Male | 0 | ||||||
BMI | 0.159 | 0.074 | 0.03 | 0.302 | 0.09 | 0.001 | |
Hypertension | 1.472 | 0.524 | 0.005 | 1.615 | 0.628 | 0.01 | |
Pre-Fib | −0.013 | 0.004 | <0.001 | −0.02 | 0.005 | <0.001 | |
Blood loss | 0.000164 | 0.000463 | 0.72 | ||||
RBC | 0.001 | 0.001 | 0.09 | ||||
Plasma | 0.001 | 0.002 | 0.36 | ||||
Post-PLT | −0.011 | 0.005 | 0.02 | −0.011 | 0.008 | 0.15 | |
Post-D-dimer | 0.00227 | 0.000145 | 0.12 | <0.001 | <0.001 | 0.07 | |
Tumor diameter | 0.48 | 0.187 | 0.01 | ||||
T stage | |||||||
T2 | −1.209 | 0.685 | 0.08 | ||||
T3/T4 | −1.543 | 0.791 | 0.051 | ||||
T0/T1/Ta | 0 | ||||||
Amount | |||||||
Multiple | −1.05 | 0.65 | 0.11 | ||||
Single | 0 | ||||||
Grade | |||||||
High | −1.776 | 0.85 | 0.04 | ||||
Low | 0 | ||||||
With CIS | |||||||
Yes | 1.499 | 0.758 | 0.048 | ||||
No | 0 |
Model A, univariate and multivariate logistic regression model; Model B, stepwise logistic regression. SE, standard error; BMI, body mass index; pre-Fib, preoperative fibrinogen; RBC, red blood cell; post-PLT, postoperative platelet; CIS, carcinoma in situ.
From a starting set of 16 variables, the Akaike Information Criterion (AIC) was utilized to identify significant predictors. Ultimately, sex, BMI, hypertension, tumor grade, tumor diameter, concurrent CIS, pre-Fib, and post-PLT were chosen for model development. A significance level of P<0.05 was applied to all these factors (Table 2).
Development and assessment of new models
Discrimination performance of the established models was compared using ROC curve analysis. Model A had an AUC of 0.806 [95% confidence interval (CI): 0.748–0.856], in contrast to Model B with a comparable AUC of 0.833 (95% CI: 0.777–0.880). It was surprising to find that the discrimination of both Models A and B were far superior than that of the CRA model which had an AUC of 0.508 (95% CI: 0.440–0.576), which was also further confirmed by the testing cohort with AUCs of 0.762 (95% CI: 0.624–0.869), 0.710 (95% CI: 0.567–0.827), and 0.681 (95% CI: 0.537–0.803), respectively (Figure 2).
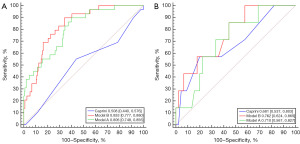
We also used the training cohort to evaluate the calibration of Models A and B (Figure 3), which yielded the Brier scores of 0.084 (range, 0.001–0.915) and 0.092 (range, 0.001–0.933) for Models A and B, respectively, indicating that both models demonstrate consistent and acceptable predictive accuracy.
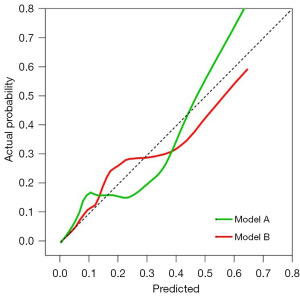
By using R programming language to verify 1,000 times with Bootstrap, Models A and B yielded C-index values of 0.806 and 0.833, respectively. Consequently, both new models had high prediction accuracy, with prediction probabilities that were consistent with actual probabilities.
Nomogram visualization
For practical use, both models were translated into nomograms (Figure 4). Nomogram A (Figure 4A) with fewer variables includes BMI, hypertension, pre-Fib, and post-PLT. Nomogram B (Figure 4B) includes seven variables: sex, BMI, hypertension, tumor grade, tumor diameter, concurrent CIS, and pre-Fib. In both nomograms, a higher total score signifies an increased VTE likelihood.
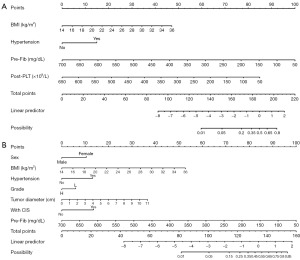
Improved performance of new models over the CRA model
In addition, the two new models’ predictive accuracy were compared to that of the CRA model based on NRI and IDI. The cutoff values of 0.2 and 0.4 were set for VTE risk. We found that the two new models significantly (P<0.01; Table 3) outperformed the CRA model (NRI >0, IDI >0) in terms of both prediction and discrimination.
Table 3
Index | Model A | P1 | Model B | P2 |
---|---|---|---|---|
NRI (categorical) (95% CI) | 0.2706 (0.1076–0.4336) | 0.001 | 0.1964 (0.0483–0.3446) | 0.009 |
NRI (continuous) (95% CI) | 0.724 (0.3547–1.0933) | <0.001 | 1.0356 (0.7013–1.3698) | <0.001 |
IDI (95% CI) | 0.2107 (0.1217–0.2997) | <0.001 | 0.2061 (0.1236–0.2887) | <0.001 |
Model A, univariate and multivariate logistic regression model; Model B, stepwise logistic regression. P1, P value for Model A; P2, P value for Model B. CRA, Caprini risk assessment; NRI, net reclassification improvement; CI, confidence interval; IDI, integrated discrimination improvement.
Decision curve analysis (DCA) for clinical application
We employed DCA to assess the clinical utility of the two prediction nomograms. In the training cohort, both models exhibited a net benefit within a threshold probability range of 0.02 to 0.84 (Figure 5A). Despite the small sample size, the new models displayed superior net benefit, evident by their higher lines on the decision curve (Figure 5B), indicating potential clinical value. Furthermore, both new models demonstrated better predictive accuracy than the CRA model as shown by the clinical impact curve (Figure 6). In comparison to the CRA model, the red solid lines (indicating estimated high-risk VTE cases) of both models more closely paralleled the blue dotted lines (indicating true VTE cases), thus signifying enhanced prediction across various threshold probabilities for future VTE events.
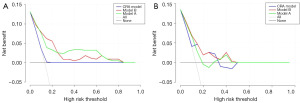
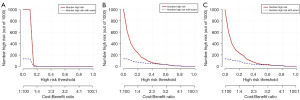
Discussion
VTE remains a non-negligible complication of urologic surgery, with PE being one of the leading causes of postoperative mortality in patients that have undergone RC (17). In addition, long-term complications following less fatal VTE plague many patients, aggravating economic burden and lowering quality of life (18-20). However, as a major urologic surgery with high bleeding risk, willingness to use anticoagulant pharmacological prophylaxis exhibited large variation among urologists, often for fear of hemorrhage (21). It is thus critical to recognize patients with higher risk of VTE and take preventive measures to prevent its occurrence at an early stage following RC.
In our cohort, the VTE incidence is 13.2%, higher than previous reports (22,23). The reason of this difference may be that we adopted a more active screening strategy and detected many subclinical asymptomatic thrombi. Asymptomatic and subclinical thrombosis, usually located in calf, hold certain significance (24,25). Several researches indicate that asymptomatic DVT could also progress to symptomatic thrombosis, or, even lead to PE (24,25). However, it is obviously beyond the scope of our study. Nonetheless, according to our follow-up, all patients in this cohort who were screened for VTE were treated with timely therapeutic doses of anticoagulant medication, and none suffered PE or long-term complications. Future research could focus on the selection of timing for anticoagulation after RC. This approach could potentially reduce the incidence of postoperative VTE formation while minimizing the risk of postoperative bleeding.
Our findings show that factors including sex, BMI, hypertension, pre-Fib, post-PLT, tumor grade, tumor diameter, and concurrent CIS are associated with risks of post-RC VTE, some of which are consistent with other studies of postoperative thrombosis (13,26,27).
Among them, BMI is a widely acknowledged clinical index associated with VTE. Based on Eichinger et al.’s findings, a higher BMI increases the risk of VTE at both the biochemical and anatomical levels (28). The biochemical effects of excessive visceral adipose tissue may damage the endothelium and trigger systemic coagulation due to generation of reactive oxygen species along with increased transport of free fatty acids into the liver as well as pro-inflammatory adipokines (29). Although not included in the well-established Caprini risk model, hypertension which is a risk factor for thrombotic disease has previously been found to be associated with venous thrombus in cancer patients (30) and was studied in the context of myeloproliferative neoplasms (31). Our study confirms again the relation between hypertension and VTE occurrence, which may be explained by induced vascular dysfunction under elevated blood pressure.
Also, we found for the first time, the relationship between bladder cancer-specific parameters and VTE. Larger tumor is associated with thrombus, which could be explained by more severe local venous compression or heavier tumor burden. Interestingly, we also confirm the concurrence of CIS as a risk factor for postoperative VTE, which represents a relatively high malignant state and deserves further research and validation. Low grade is found to be associated with thrombus occurrence, contrary to our hypothesis. The reason may be that low-grade tumors tend to be larger than high-grade ones, with the average maximum diameter being 3.64 and 2.00 cm, respectively.
Although age is potentially a strong independent predictor, it was not finally included in the model (P>0.05). The risk of VTE increases with age for women over 55 years and men over 65 years, according to previous data (32). Furthermore, elderly patients tend to experience slower recovery from illness and remain bedridden for longer periods of time, resulting in a longer time of immobilization and subsequent blood stasis. Operation time also tended to be longer in the VTE group (6.75 h) than the non-VTE group (6 h), although without statistical significance, which often suggests more extensive surgery and more severe trauma.
The most important conclusion of our study, however, is the inability of the widely-used Caprini risk score to stratify the VTE risk in post-RC patients. The combination of radical surgery and bladder cancer incurs two elements of the famous Virchow’s thrombogenic triad (blood stasis, hypercoagulability, and endothelial dysfunction). As a result, while RC patients have significantly different risk profiles, almost all are categorized as being at high-risk for VTE, thus limiting its actual value as a risk assessment tool. In fact, McAlpine found VTE risk after RC to be independent of Caprini risk category (22).
Nonetheless, our study has limitations. It is a single-center, retrospective analysis with a limited sample size, leading to some inconsistencies between the training and testing cohorts. To establish robust conclusions, validation in prospective cohorts from other institutions is essential. Enhanced statistical power and better representation can be achieved through large-scale multicenter investigations. Moreover, our study employed low-molecular-weight heparin sodium as the standard anticoagulation therapy. However, the landscape of anticoagulation treatment has progressed significantly with the emergence of novel oral anticoagulants (NOACs) encompassing direct thrombin and FXa inhibitors. Optimizing thromboprophylaxis strategies to maximize benefits warrants further exploration.
Conclusions
We retrospectively analyzed bladder cancer data from Peking University People’s Hospital to create two improved prediction models, which outperform the CRA model. This easy-to-use nomogram could aid urologists in making better-informed clinical decisions and aid in the early detection of high-risk cases, thus benefiting patients. Nevertheless, owing to limited sample size and a retrospective approach, it is not flawless in optimizing thrombus risk assessment for post-RC patients. These nomograms require validation and adjustments through future prospective, multicenter studies.
Acknowledgments
Funding: None.
Footnote
Reporting Checklist: The authors have completed the TRIPOD reporting checklist. Available at https://tau.amegroups.com/article/view/10.21037/tau-24-194/rc
Data Sharing Statement: Available at https://tau.amegroups.com/article/view/10.21037/tau-24-194/dss
Peer Review File: Available at https://tau.amegroups.com/article/view/10.21037/tau-24-194/prf
Conflicts of Interest: All authors have completed the ICMJE uniform disclosure form (available at https://tau.amegroups.com/article/view/10.21037/tau-24-194/coif). The authors have no conflicts of interest to declare.
Ethical Statement: The authors are accountable for all aspects of the work in ensuring that questions related to the accuracy or integrity of any part of the work are appropriately investigated and resolved. The study was conducted in accordance with the Declaration of Helsinki (as revised in 2013) and approved by the ethical committee of Peking University People’s Hospital (No. 2023PHB246-001). As the data are anonymous, informed consent was not required.
Open Access Statement: This is an Open Access article distributed in accordance with the Creative Commons Attribution-NonCommercial-NoDerivs 4.0 International License (CC BY-NC-ND 4.0), which permits the non-commercial replication and distribution of the article with the strict proviso that no changes or edits are made and the original work is properly cited (including links to both the formal publication through the relevant DOI and the license). See: https://creativecommons.org/licenses/by-nc-nd/4.0/.
References
- Babjuk M, Burger M, Capoun O, et al. European Association of Urology Guidelines on Non-muscle-invasive Bladder Cancer (Ta, T1, and Carcinoma in Situ). Eur Urol 2022;81:75-94. [Crossref] [PubMed]
- Domínguez Escrig JL. Muscle invasive bladder cancer and bladder preservation protocols. Where are we?. Arch Esp Urol 2020;73:986-95. [PubMed]
- Kim SP, Shah ND, Karnes RJ, et al. The implications of hospital acquired adverse events on mortality, length of stay and costs for patients undergoing radical cystectomy for bladder cancer. J Urol 2012;187:2011-7. [Crossref] [PubMed]
- Scarpa RM, Carrieri G, Gussoni G, et al. Clinically overt venous thromboembolism after urologic cancer surgery: results from the @RISTOS Study. Eur Urol 2007;51:130-5; discussion 136. [Crossref] [PubMed]
- Bagrodia A, Sukhu R, Winer AG, et al. Incidence and Effect of Thromboembolic Events in Radical Cystectomy Patients Undergoing Preoperative Chemotherapy for Muscle-invasive Bladder Cancer. Clin Genitourin Cancer 2017; [Crossref] [PubMed]
- Elting LS, Escalante CP, Cooksley C, et al. Outcomes and cost of deep venous thrombosis among patients with cancer. Arch Intern Med 2004;164:1653-61. [Crossref] [PubMed]
- Alberts BD, Woldu SL, Weinberg AC, et al. Venous thromboembolism after major urologic oncology surgery: a focus on the incidence and timing of thromboembolic events after 27,455 operations. Urology 2014;84:799-806. [Crossref] [PubMed]
- Ostrowski A, Skrudlik P, Kowalski F, et al. Current thromboprophylaxis in urological cancer patients during COVID-19 pandemic. Cent European J Urol 2022;75:128-34. [PubMed]
- Khorana AA, Mackman N, Falanga A, et al. Cancer-associated venous thromboembolism. Nat Rev Dis Primers 2022;8:11. [Crossref] [PubMed]
- Cheng X, Zhou L, Liu W, et al. Construction and Verification of Risk Predicting Models to Evaluate the Possibility of Venous Thromboembolism After Robot-Assisted Radical Prostatectomy. Ann Surg Oncol 2022;29:5297-306. [Crossref] [PubMed]
- Leong CH, Ranjan SR, Javed A, et al. Predictive accuracy of boosted regression model in estimating risk of venous thromboembolism following minimally invasive radical surgery in pharmacological prophylaxis-naïve men with prostate cancer. World J Surg Oncol 2024;22:67. [Crossref] [PubMed]
- Cai Y, Dong H, Li X, et al. Development and validation of a nomogram to assess postoperative venous thromboembolism risk in patients with stage IA non-small cell lung cancer. Cancer Med 2023;12:1217-27. [Crossref] [PubMed]
- Abdullah O, Parashar D, Mustafa IJ, et al. Venous Thromboembolism Rate in Patients With Bladder Cancer According to the Type of Treatment: A Systematic Review. Cureus 2022;14:e22945. [Crossref] [PubMed]
- Elsayed AS, Ozair S, Iqbal U, et al. Prevalence and Predictors of Venous Thromboembolism After Robot-Assisted Radical Cystectomy. Urology 2021;149:146-53. [Crossref] [PubMed]
- Umakanthan S, Sahu P, Ranade AV, et al. Origin, transmission, diagnosis and management of coronavirus disease 2019 (COVID-19). Postgrad Med J 2020;96:753-8. [PubMed]
- Schomaker M, Heumann C. Bootstrap inference when using multiple imputation. Stat Med 2018;37:2252-66. [Crossref] [PubMed]
- Quek ML, Stein JP, Daneshmand S, et al. A critical analysis of perioperative mortality from radical cystectomy. J Urol 2006;175:886-9; discussion 889-90. [Crossref] [PubMed]
- Franzeck UK, Schalch I, Jäger KA, et al. Prospective 12-year follow-up study of clinical and hemodynamic sequelae after deep vein thrombosis in low-risk patients (Zürich study). Circulation 1996;93:74-9. [Crossref] [PubMed]
- Prandoni P, Bernardi E, Marchiori A, et al. The long term clinical course of acute deep vein thrombosis of the arm: prospective cohort study. BMJ 2004;329:484-5. [Crossref] [PubMed]
- Bergqvist D, Jendteg S, Johansen L, et al. Cost of long-term complications of deep venous thrombosis of the lower extremities: an analysis of a defined patient population in Sweden. Ann Intern Med 1997;126:454-7. [Crossref] [PubMed]
- Violette PD, Vernooij RWM, Aoki Y, et al. An International Survey on the Use of Thromboprophylaxis in Urological Surgery. Eur Urol Focus 2021;7:653-8. [Crossref] [PubMed]
- McAlpine K, Breau RH, Mallick R, et al. Current guidelines do not sufficiently discriminate venous thromboembolism risk in urology. Urol Oncol 2017;35:457.e1-8. [Crossref] [PubMed]
- Klaassen Z, Arora K, Goldberg H, et al. Extended Venous Thromboembolism Prophylaxis after Radical Cystectomy: A Call for Adherence to Current Guidelines. J Urol 2018;199:906-14. [Crossref] [PubMed]
- Heidrich H, Konau E, Hesse P. Asymptomatic venous thrombosis in cancer patients--a problem often overlooked. Results of a retrospective and prospective study. Vasa 2009;38:160-6. [Crossref] [PubMed]
- Lohr JM, James KV, Deshmukh RM, et al. Calf vein thrombi are not a benign finding. Am J Surg 1995;170:86-90. [Crossref] [PubMed]
- Liu X, Xu A, Huang J, et al. Effective prediction model for preventing postoperative deep vein thrombosis during bladder cancer treatment. J Int Med Res 2022;50:3000605211067688. [Crossref] [PubMed]
- Doiron RC, Booth CM, Wei X, et al. Risk factors and timing of venous thromboembolism after radical cystectomy in routine clinical practice: a population-based study. BJU Int 2016;118:714-22. [Crossref] [PubMed]
- Eichinger S, Hron G, Bialonczyk C, et al. Overweight, obesity, and the risk of recurrent venous thromboembolism. Arch Intern Med 2008;168:1678-83. [Crossref] [PubMed]
- Görlach A. Redox regulation of the coagulation cascade. Antioxid Redox Signal 2005;7:1398-404. [Crossref] [PubMed]
- Zhao H, Liang F, Ling Y, et al. Prevalence and treatment of venous thromboembolism in patients with solid tumors. Exp Ther Med 2022;24:743. [Crossref] [PubMed]
- Arachchillage DR, Laffan M. Pathogenesis and Management of Thrombotic Disease in Myeloproliferative Neoplasms. Semin Thromb Hemost 2019;45:604-11. [Crossref] [PubMed]
- Chopard R, Albertsen IE, Piazza G. Diagnosis and Treatment of Lower Extremity Venous Thromboembolism: A Review. JAMA 2020;324:1765-76. [Crossref] [PubMed]