Evaluating the impact of artificial intelligence-based assessment of body composition on primary artificial urinary sphincter placement outcomes
Highlight box
Key findings
• Sarcopenia was present in 55% of patients undergoing primary artificial urinary sphincter (AUS) placement.
• No significant difference in overall device survival was observed between sarcopenic and non-sarcopenic patients.
• An artificial intelligence (AI)-based body composition algorithm was used to automatically assess muscle mass and adiposity from computed tomography (CT) scans, providing an efficient approach for evaluating body composition.
What is known and what is new?
• Sarcopenia is commonly associated with adverse outcomes in surgical patients, and AUS placement is the standard treatment for severe post-prostatectomy incontinence.
• This is the first study to investigate the impact of body composition on AUS outcomes. This manuscript found that body composition metrics, such as sarcopenia and adiposity, were not significantly associated with overall AUS device survival, or specific device outcomes including erosion, urethral atrophy, and mechanical device failure.
What is the implication, and what should change now?
• This study highlights the potential for implementation of AI-based body composition analysis.
• The results from this study could be used to counsel patients preoperatively, suggesting that body composition in well-selected patients, including sarcopenia, does not significantly impact AUS device outcomes.
Introduction
Post-prostatectomy incontinence can be a significant procedure-related morbidity following prostate cancer treatment, for which approximately 6–9% of patients ultimately pursue surgical treatment (1). The artificial urinary sphincter (AUS) is the preferred treatment for severe stress urinary incontinence (SUI) in men following prostate cancer treatment (2). AUS implantation is associated with significant enhancement in the quality of life for patients, with high satisfaction rates, exceeding 90% (3-5). However, a substantial proportion of patients eventually require revision or removal for mechanical failure, erosion, infection, or urethral atrophy, among other factors (4). Recent studies from high-volume centers indicate an approximately 25% revision rate at the five-year timepoint (6,7). However, the literature remains conflicted on which factors lead to device failure.
Given that AUS placement occurs largely in the older adult population, factors specific to this cohort warrant further exploration (8). Sarcopenia is characterized as the progressive loss of muscle mass in older adult populations that is maladaptive and in excess of natural aging (9). Sarcopenia may be present in as many as 56% of prostatectomy patients (10). Prior studies have shown that sarcopenia is linked to post-operative outcomes in the urologic oncology and general surgery literature (11,12). Additionally, measurements of adiposity as assessed through computed tomography (CT) imaging, provide a more precise measurement of fat distribution, particularly visceral fat, which has been associated with a higher risk of metabolic complications (13). These complications may impact healing, tissue integration, and the long-term functionality of AUS devices. Thus, exploring the impact of visceral adiposity, alongside sarcopenia, on AUS outcomes could offer new insights into the likelihood of device failure, complications, or the need for revisions, potentially leading to more tailored patient management strategies.
Despite these known associations, the impact of sarcopenia and body composition on specific disease outcomes remains poorly studied. Sarcopenia has historically been difficult to assess—often operationalized through clinical questionnaires or requiring costly dual-energy X-ray absorptiometry (DEXA) scans, and manual segmentation on CT imaging is time consuming (14,15). Automated segmentation of muscle mass and adipose tissue on CT through convolutional neural networks have enabled accurate and precise determination of body composition from routine abdominal CT scans (16).
This study aimed to leverage advancements in automated body composition analysis from CT imaging to investigate sarcopenia as a potential predictor of outcomes after AUS placement. Given the potential high prevalence of sarcopenia in AUS patients, an understanding of the effect of sarcopenia on AUS outcomes could lead to the integration of body composition analysis into pre-operative risk assessment and planning. We present this article in accordance with the STROBE reporting checklist (available at https://tau.amegroups.com/article/view/10.21037/tau-24-342/rc).
Methods
The study was conducted in accordance with the Declaration of Helsinki (as revised in 2013). The study was approved by Mayo Clinic Institutional Review Board (IRB #23-008155) and individual consent for this retrospective analysis was waived. Following IRB approval, a retrospective analysis of patients undergoing primary AUS placement for post-prostatectomy incontinence from 1999 to 2023 at our institution was performed. All implanted AUS devices were an AMS 800® (Boston Scientific, Marlboro, MA, USA). The urethral cuffs were placed around the bulbar urethra via transperineal approach with cuff size at the treating surgeon’s discretion. Patients were excluded if they were undergoing secondary AUS implantation/revision surgery, had their prostate in-situ, had a history of prostate cryoablation, or did not have abdominal/pelvic CT imaging available within 12 months prior to AUS placement.
Determination of body composition
A completely automated two stage U-Net architecture model was used to perform automated abdominal CT segmentation of skeletal muscle area and adipose tissue areas (visceral, subcutaneous and intermuscular adipose tissue) as described previously (16). In the initial stage of the process, the algorithm identified the L3 vertebral level. The second stage included segmentation of skeletal muscle and adipose tissue. The output was a segmentation map identifying subcutaneous adipose tissue, skeletal muscle, visceral adipose tissue, and intermuscular adipose tissue. Figure 1 shows an example segmentation. The algorithm’s accuracy for the muscle component, indicated by the dice coefficient, was previously recorded at 0.95±0.02 (16). To account for height variations, skeletal muscle area measurements were normalized by dividing the area values by the square of the patient’s height (m2), resulting in the calculation of the skeletal muscle index (SMI). The model was written in Python 2.7.5.
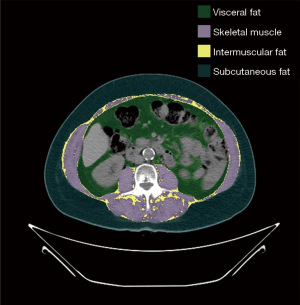
Body composition variables
We explored several body composition variables in this study. These included the continuous SMI, and the binary determination of a patient’s sarcopenic status as defined by a commonly used measure developed by Prado et al., which defines sarcopenia as a SMI <52.4 cm2/m2 (17). In addition to skeletal muscle area, our algorithm also determined subcutaneous and visceral fat area, so associations between the subcutaneous and visceral fat area to outcomes was also explored.
Outcomes
The primary outcome of interest in this study was all-cause reoperation (revision or removal), as used in recent publications (18). Secondary outcomes of interest were specific etiologies for reoperation including device infection/urethral erosion, urethral atrophy, or device malfunction. Erosion was defined as perforation of the urethral cuff into the urethral lumen on cystoscopy. Device explantation was performed due to infection if suggested by clinical presentation, without evidence of erosion on cystoscopy. Recorded non-body composition clinical variables included patient age, incontinence etiology, Charlson Comorbidity Index (CCI), radiation history, androgen deprivation therapy, coronary artery disease and history of prior incontinence procedures.
Data collection
Individual charts were reviewed to evaluate pertinent clinical and surgical comorbidities, details of the primary devices, primary device outcome including time to failure, failed component, revision management strategy (single component vs. entire device) and specific device outcomes (i.e., explantation for urethral erosion or infection, revision for device malfunction, urethral atrophy, tubing or pump complications). Patients were followed via office evaluation or periodically through mailed correspondence sent by our institution’s AUS registry. Details regarding device survival were obtained from the last office examination, any available subsequent operative report, or written or telephone correspondence.
Statistical analysis
Continuous variables were expressed as median [interquartile range (IQR)], whereas categorical variables were expressed as counts and percentages. Continuous variables were compared using the Mann-Whitney U-test and categorical variables were compared using the Chi-square and Fisher’s Exact test when cell counts were less than 5. Device survival was estimated as the time from AUS implantation for mechanical failure to subsequent repeat surgery (including explantation or device revision for any reason) or last known follow-up, using the Kaplan-Meier method and compared with the log rank test. For this analysis the patients were censored at the time of last known follow-up if no event occurred. Cox proportional hazards modelling was performed for all analyses. All statistical tests were 2-sided with P<0.05 considered statistically significant. Analyses were performed using R version 4.3.1 (19).
Results
Patient characteristics
During the study timeframe, a total of 885 primary AUS implantations for post-prostatectomy incontinence were performed. Of these, 111 (12.5%) patients had a CT scan extending down to the L3 region available for analysis within 12 months prior to surgery and were included in the study. Patients were stratified by SMI (<52.4 cm2/m2) into those with sarcopenia (n=61, 55%) and those without sarcopenia (n=50, 45%). Clinical and demographic features of the cohort are shown in Table 1. The sarcopenic group was significantly older at time of implant (71.8 vs. 68.3 years; P<0.001) and had a lower body mass index (BMI) at time of implant (29.1 vs. 32.7 kg/m2; P<0.001) compared to the non-sarcopenic group. Notably, 19 of 61 patients in the non-sarcopenic cohort had BMIs in the obese range. There were no significant differences between cohorts regarding history of pelvic radiation therapy (65.6% vs. 60.0%, P=0.68), history of ADT (27.9% vs. 18%, P=0.32), median CCI [3 (IQR 3, 4) vs. 3 (IQR 2, 4); P=0.16], or smoking status (P=0.76).
Table 1
Clinical and demographic features | Sarcopenic (n=61) | Non-sarcopenic (n=50) | P value |
---|---|---|---|
Year of surgery | 0.31 | ||
1999–2009 | 11 (18.0) | 13 (26.0) | |
2010–2023 | 50 (82.0) | 37 (74.0) | |
Patient age, years | 71.8 [67.8–76.1] | 68.3 [64.5–71.2] | <0.001 |
BMI, kg/m2 | 29.1 [26.6–31.8] | 32.7 [28.8–34.8] | <0.001 |
Charlson score | 3 [3–4] | 3 [2–4) | 0.16 |
Prior pelvic radiation | 40 (65.6) | 30 (60.0) | 0.68 |
Prior urethral bulking agent injection | 2 (3.3) | 3 (6.0) | 0.82 |
Prior androgen deprivation therapy | 17 (27.9) | 9 (18.0) | 0.32 |
Coronary artery disease | 13 (21.3) | 12 (24.0) | 0.91 |
Smoking status | 0.76 | ||
Current | 6 (9.8) | 3 (6.0) | |
Previous | 27 (44.3) | 23 (46.0) | |
Never | 28 (45.9) | 24 (48.0) | |
SMI, cm2/m2 | 46.6 [42.6–49.2] | 57.8 [54.7–61.0] | <0.001 |
Visceral to subcutaneous body fat ratio | 1.23 [0.85–1.57] | 1.02 [0.82–1.46] | 0.32 |
Subcutaneous adipose tissue area | 209 [155–280] | 238 [187–326] | 0.04 |
Visceral tissue area | 284 [171–345] | 271.5 [232–379] | 0.37 |
Follow-up duration, years | 2.11 [0.53–4.78] | 2.52 [0.36–5.80] | 0.52 |
Device failure reasons | 0.14 | ||
Infection | 3 (16.7) | 0 | |
Erosion | 3 (16.7) | 6 (33.3) | |
Malfunction | 7 (38.9) | 6 (33.3) | |
Atrophy | 4 (22.2) | 5 (27.8) | |
Pump malposition | 1 (5.6) | 1 (5.6) |
Data are presented as median [IQR] or n (%). SMI, skeletal muscle index; IQR, interquartile range; BMI, body mass index.
Device outcomes
The median postoperative follow-up among the 75 patients without device failure was 2.52 years (IQR 0.45, 5.68). Follow-up did not differ significantly by sarcopenic status (P=0.52). Overall 36 patients that underwent secondary AUS surgeries, including 12 reoperations for infection/erosion, 9 for urethral atrophy, 13 for device malfunction, and 2 for pump malposition. In each of the cohorts, there were 18 secondary operations. In the sarcopenic cohort, 16.7% (n=3) of device failures were due to infection, whereas there were no infection events in the non-sarcopenic cohort.
Association with sarcopenia
On Kaplan-Meier survival analysis, sarcopenic status was not associated with adverse overall-device survival (5-year device survival, 55.6% vs. 60%; P=0.94) (see Figure 2). When assessing specific device outcomes on univariate analysis, sarcopenic status was not significantly associated with the risk of AUS infection/erosion (P=0.86), urethral atrophy (HR: 0.79; 95% CI: 0.21–3.04; P=0.73) or device malfunction (HR 1.74; 95% CI: 0.53–5.66; P=0.36). HRs for all models are listed in Table 2.
Table 2
Parameters | All-cause device failure | Infection or erosion related device failure | Atrophy related device failure | Device malfunction | |||||||
---|---|---|---|---|---|---|---|---|---|---|---|
HR (95% CI) | P | HR (95% CI) | P | HR (95% CI) | P | HR (95% CI) | P | ||||
Subcutaneous adipose tissue | – | >0.2 (0.98) | – | >0.2 (0.52) | – | >0.2 (0.56) | – | >0.2 (0.99) | |||
Visceral adipose tissue | 1.002 (0.999–1.005) | 0.20 | – | >0.2 (0.77) | – | >0.2 (0.47) | 1.004 (1.00–1.009) | 0.13 | |||
Intermuscular adipose tissue | – | >0.2 (0.87) | – | >0.2 (0.91) | – | >0.2 (0.71) | – | >0.2 (0.65) | |||
Skeletal muscle tissue | – | >0.2 (0.30) | – | >0.2 (0.80) | – | >0.2 (0.42) | – | >0.2 (0.36) | |||
Skeletal muscle index | – | >0.2 (0.58) | – | >0.2 (0.97) | – | >0.2 (0.24) | – | >0.2 (0.96) | |||
Body mass index | 1.049 (0.99–1.11) | 0.084 | – | >0.2 (0.80) | 1.12 (1.024–1.22) | 0.012 | >0.2 (0.64) | ||||
Sarcopenic status | – | >0.2 (0.94) | – | >0.2 (0.86) | – | >0.2 (0.67) | – | >0.2 (0.57) |
HR, hazard ratio; CI, confidence interval.
Association with adiposity
On analysis of other body composition variables, subcutaneous adipose tissue area was significantly lower in sarcopenic patients [209 (IQR 155, 280) vs. 238 (IQR 187, 326) cm2; P=0.04]. Visceral adipose tissue area did not differ significantly between the two cohorts [284 (IQR 171, 345) vs. 272 (IQR 232, 379) cm2; P=0.37]. On Cox survival analysis, adiposity related parameters were not associated with device survival outcomes (see Table 2).
Discussion
We found in an assessment of body composition that SMI and sarcopenic status were not associated with all cause reoperation following AUS placement in the management of post prostatectomy incontinence. Additionally, our investigation extends to device-specific complications, such as erosion, malfunction, and urethral atrophy, presenting a comprehensive view of how sarcopenia may indirectly influence patient outcomes post-AUS placement. Those with sarcopenia did have a higher rate of infectious complications, whereas there were no device infection events in the non-sarcopenic cohorts. Also of note, within the sarcopenic cohort, 31% of patients had BMIs within the obese range, highlighting the importance of exploring the impact of differing body composition metrics on surgical outcomes outside of BMI.
Our results regarding overall device survival are like those previously reported in the literature, serving as an important background for comparison of sarcopenia status. Overall device survival in our cohort at 5 years was 72% for those with sarcopenia and 69% for those without sarcopenia, consistent with previous reports (6,7). Likewise, the median duration of follow-up is similar to other large cohorts previously reported (20). It is reassuring that, albeit with surgical selection, sarcopenia status was not significantly associated with adverse overall device survival.
One unique aspect of our study is the assessment of the association of sarcopenia status on AUS outcomes. First, our study supports the use of an automatic segmentation model using artificial intelligence to assess sarcopenia. Use of such technology represents a growing opportunity for better understanding body composition and its impact on clinical care across multiple disease states. Likewise, our finding of differential BMI by sarcopenia status highlights the potential utility of this measure, rather than relying on BMI alone. That is, some patients may have obesity with or without underlying sarcopenia status; the interaction of these two parameters on surgical outcomes may represent an area of further exploration.
Globally, sarcopenic status is thought to lead to increased perioperative complications due to decreased postoperative patient physical resilience and impaired wound healing (21). Indeed, in the sarcopenic cohort, infection constituted 16.7% of the cause of AUS explant (versus none in the non-sarcopenic cohort), although the lack of events in the non-sarcopenic cohort precluded Cox survival curve modelling. The increased infection occurrence in the sarcopenic cohort may reflect issues with wound healing in this group of patients. However, within context in the literature, AUS infection and erosion rates have a combined mean rate of 8.5%, which is similar to the combined infection and erosion rates in both cohorts within this study (22).
Qualitatively, sarcopenic patients had higher rates of infectious etiology related device failure early after surgery, however we were unable to further explore. Accordingly, there is a need for further exploration with subsequent larger cohort studies. There may be a need for higher vigilance for detection and early management of suspected infection in sarcopenic patients. Recent surgical techniques such as the “minimal-touch” technique may lead to reduced infection-related complications (23). Additionally other strategies to minimize infections in AUS patients have previously been documented (24).
This study has several limitations. Its retrospective nature and the restriction to patients with available CT scans limited the cohort size. Nevertheless, our sample of 111 patients is comparatively large within the context of previously published AUS studies and represents a more contemporary cohort (largely 2000s onwards) compared to the parent database, which dates to 1983. Selection bias may persist, as patients with severe sarcopenia might have been excluded from surgical intervention at the surgeon’s discretion. Consequently, our assessment of sarcopenia is confined to patients deemed suitable surgical candidates. Additionally, while our algorithm analyzed body composition at the L3 vertebral level, adipose tissue and muscle area can vary at different abdominal levels depending on individual anthropometrics. However, the L3 cross-section is widely accepted for determining sarcopenic status, and our model has demonstrated good generalizability across the entire abdomen in a prior validation study (16).
Conclusions
Sarcopenia was prevalent among AUS patients but did not significantly impact overall device survival. These findings suggest that AUS placement may be safe to perform in well selected sarcopenic patients, though there may be an increased risk of device infection. Future studies should explore larger cohorts and longer follow-up periods to fully understand the implications of sarcopenia on AUS outcomes.
Acknowledgments
Funding: This study was supported by
Footnote
Reporting Checklist: The authors have completed the STROBE reporting checklist. Available at https://tau.amegroups.com/article/view/10.21037/tau-24-342/rc
Data Sharing Statement: Available at https://tau.amegroups.com/article/view/10.21037/tau-24-342/dss
Peer Review File: Available at https://tau.amegroups.com/article/view/10.21037/tau-24-342/prf
Conflicts of Interest: All authors have completed the ICMJE uniform disclosure form (available at https://tau.amegroups.com/article/view/10.21037/tau-24-342/coif). The authors have no conflicts of interest to declare.
Ethical Statement: The authors are accountable for all aspects of the work in ensuring that questions related to the accuracy or integrity of any part of the work are appropriately investigated and resolved. The study was conducted in accordance with the Declaration of Helsinki (as revised in 2013). The study was approved by Mayo Clinic Institutional Review Board (IRB #23-008155) and individual consent for this retrospective analysis was waived.
Open Access Statement: This is an Open Access article distributed in accordance with the Creative Commons Attribution-NonCommercial-NoDerivs 4.0 International License (CC BY-NC-ND 4.0), which permits the non-commercial replication and distribution of the article with the strict proviso that no changes or edits are made and the original work is properly cited (including links to both the formal publication through the relevant DOI and the license). See: https://creativecommons.org/licenses/by-nc-nd/4.0/.
References
- Castellan P, Ferretti S, Litterio G, et al. Management of Urinary Incontinence Following Radical Prostatectomy: Challenges and Solutions. Ther Clin Risk Manag 2023;19:43-56. [Crossref] [PubMed]
- Sandhu JS, Breyer B, Comiter C, et al. Incontinence after Prostate Treatment: AUA/SUFU Guideline. J Urol 2019;202:369-78. [Crossref] [PubMed]
- Deruyver Y, Schillebeeckx C, Beels E, et al. Long-term outcomes and patient satisfaction after artificial urinary sphincter implantation. World J Urol 2022;40:497-503. [Crossref] [PubMed]
- Findlay BL, Jefferson FA IV, Elliott DS. Evaluation and Revision of Artificial Urinary Sphincter Failure for Male Stress Urinary Incontinence. Curr Bladder Dysfunct Rep 2023;1-7. [Crossref]
- Viers BR, Linder BJ, Rivera ME, et al. Long-Term Quality of Life and Functional Outcomes among Primary and Secondary Artificial Urinary Sphincter Implantations in Men with Stress Urinary Incontinence. J Urol 2016;196:838-43. [Crossref] [PubMed]
- Linder BJ, Rivera ME, Ziegelmann MJ, et al. Long-term Outcomes Following Artificial Urinary Sphincter Placement: An Analysis of 1082 Cases at Mayo Clinic. Urology 2015;86:602-7. [Crossref] [PubMed]
- Bentellis I, El-Akri M, Cornu JN, et al. Prevalence and Risk Factors of Artificial Urinary Sphincter Revision in Nonneurological Male Patients. J Urol 2021;206:1248-57. [Crossref] [PubMed]
- Jones CP, Shaw NM, Mena J, et al. The relationship between frailty, incontinence severity, and treatment decisions for men with post-prostatectomy stress urinary incontinence: a mixed methods analysis. Transl Androl Urol 2023;12:840-8. [Crossref] [PubMed]
- Knoedler S, Schliermann R, Knoedler L, et al. Impact of sarcopenia on outcomes in surgical patients: a systematic review and meta-analysis. Int J Surg 2023;109:4238-62. [PubMed]
- Mason RJ, Boorjian SA, Bhindi B, et al. The Association Between Sarcopenia and Oncologic Outcomes After Radical Prostatectomy. Clin Genitourin Cancer 2018;16:e629-36. [Crossref] [PubMed]
- Guo Z, Gu C, Gan S, et al. Sarcopenia as a predictor of postoperative outcomes after urologic oncology surgery: A systematic review and meta-analysis. Urol Oncol 2020;38:560-73. [Crossref] [PubMed]
- Friedman J, Lussiez A, Sullivan J, et al. Implications of sarcopenia in major surgery. Nutr Clin Pract 2015;30:175-9. [Crossref] [PubMed]
- Kim LJ, Nalls MA, Eiriksdottir G, et al. Associations of visceral and liver fat with the metabolic syndrome across the spectrum of obesity: the AGES-Reykjavik study. Obesity (Silver Spring) 2011;19:1265-71. [Crossref] [PubMed]
- Messina C, Maffi G, Vitale JA, et al. Diagnostic imaging of osteoporosis and sarcopenia: a narrative review. Quant Imaging Med Surg 2018;8:86-99. [Crossref] [PubMed]
- Cruz-Jentoft AJ, Bahat G, Bauer J, et al. Sarcopenia: revised European consensus on definition and diagnosis. Age Ageing 2019;48:16-31. [Crossref] [PubMed]
- Weston AD, Korfiatis P, Kline TL, et al. Automated Abdominal Segmentation of CT Scans for Body Composition Analysis Using Deep Learning. Radiology 2019;290:669-79. [Crossref] [PubMed]
- Prado CM, Lieffers JR, McCargar LJ, et al. Prevalence and clinical implications of sarcopenic obesity in patients with solid tumours of the respiratory and gastrointestinal tracts: a population-based study. Lancet Oncol 2008;9:629-35. [Crossref] [PubMed]
- Malshy K, Marthi S, Ortiz R, et al. Comparing the lifespan of virgin artificial urinary sphincters in radiated patients: transcorporal vs. standard placement. World J Urol 2024;42:391. [Crossref] [PubMed]
- Liu H, Yang D, Li S, et al. A Reliable Estimate of Visceral Fat Area From Simple Anthropometric Measurements in Chinese Overweight and Obese Individuals. Front Endocrinol (Lausanne) 2022;13:916124. [Crossref] [PubMed]
- Tutolo M, Cornu JN, Bauer RM, et al. Efficacy and safety of artificial urinary sphincter (AUS): Results of a large multi-institutional cohort of patients with mid-term follow-up. Neurourol Urodyn 2019;38:710-8. [Crossref] [PubMed]
- Wang H, Yang R, Xu J, et al. Sarcopenia as a predictor of postoperative risk of complications, mortality and length of stay following gastrointestinal oncological surgery. Ann R Coll Surg Engl 2021;103:630-7. [Crossref] [PubMed]
- Van der Aa F, Drake MJ, Kasyan GR, et al. The artificial urinary sphincter after a quarter of a century: a critical systematic review of its use in male non-neurogenic incontinence. Eur Urol 2013;63:681-9. [Crossref] [PubMed]
- Ziegelmann MJ, Hebert KJ, Linder BJ, et al. The "Minimal-Touch" Technique for Artificial Urinary Sphincter Placement: Description and Outcomes. Urol Res Pract 2023;49:40-7. [Crossref] [PubMed]
- Brant WO, Martins FE. Artificial urinary sphincter. Transl Androl Urol 2017;6:682-94. [Crossref] [PubMed]