Molecular signature of immune-related new survival predictions for subtype of renal cell carcinomas
Highlight box
Key findings
• This study identified five key survival-related genes (ANK3, FREM2, KIF13B, MPP7 and SOX6) in renal clear cell carcinoma (KIRC) that are associated with favorable prognosis.
• Higher expression levels of these genes are associated with better survival outcomes, whereas downregulation is associated with immune regulation and infiltration of various immune cells.
• This study highlighted these genes as potential novel prognostic biomarkers for KIRC.
What is known and what is new?
• Renal cell carcinoma (RCC) encompasses various subtypes, including KIRC, kidney renal papillary cell carcinoma, and kidney chromophobe, and is influenced by multiple genetic factors. Previous studies have identified various genes associated with RCC prognosis and immune response.
• Despite recent advances in renal cell carcinoma, patients with KIRC have a poor prognosis, and new prognostic biomarkers and treatment strategies are urgently needed.
• This paper systematically evaluated the gene expression profiles of RCC subtypes and identified specific differentially expressed genes associated with survival in KIRC.
• It provides new insights into the relationship between the expression of the identified DEGs and the immune response, suggesting their role as prognostic biomarkers.
What is the implication, and what should change now?
• ANK3, FREM2, KIF13B, MPP7 and SOX6 can be used as biomarkers for the prognosis of KIRC, which may affect treatment decisions and patient management.
• Future research should focus on understanding the mechanisms behind the downregulation and functional inactivation of these genes to explore their therapeutic potential.
Introduction
Kidney cancer stands among the leading ten malignancies worldwide, constituting 2% of the total global cancer incidence, with an increasing number observed each year. Renal cell carcinoma (RCC) is the predominant type of kidney cancer, comprising 2–3% of all non-cutaneous malignant neoplasms in adults (1). RCC are classified into three main subtypes, including kidney renal papillary cell carcinoma (KIRP), kidney chromophobe (KICH), and kidney renal clear cell carcinoma (KIRC). The most common subtype is KIRC, which accounts for ~70–75% of RCC (2). Despite significant advances in understanding the molecular mechanisms and therapy approaches for RCC in recent years, the prognosis for KIRC patients remains discouraging (3). Consequently, it is urgent to explore promising novel prognostic biomarkers and treatment strategies. These endeavors will help clinicians in selecting more effective treatment strategies, accurately predicting long-term prognosis, and ultimately benefiting individuals diagnosed with KIRC.
The molecular pathogenesis of cancer is complex, which is related to the inactivation and mutation of antioncogenes and the activation of oncogenes (4). RCC exhibits molecular diversity, so capturing relevant molecular features may improve outcome prediction (5). The application of gene sequencing technology has facilitated the identification of numerous mRNA molecules in RCC. Zhao et al. identified 259 genes correlated with survival in conventional renal cell carcinoma (cRCC) using DNA microarrays (5). Numerous studies have shown that multiple genes are associated with overall survival, such as genes related to cuproptosis, apoptosis, glycolysis, ferroptosis and phytanoyl-CoA 2-hydroxylase (PHYH) family genes, etc. (6-10). The molecular mechanisms affecting RCC development are still not well understood, due to the discontinuity of most studies. Recently, immunotherapy as a hot topic has been shown to be an appreciated treatment strategy for cancers including RCC (11,12). Programmed cell death protein 1/programmed cell death protein ligand 1 (PD-1/PD-L1) blockade showed modest anti-tumor efficacy in non-clear cell RCC, and exhibited a considerably higher response rate compared to patients with other RCC types (11). Nevertheless, the availability of viable immune-related biomarkers for predicting patient survival in these other RCC types remains scarce. Furthermore, the identification of potential novel immunotherapeutic targets is also lacking in this context.
Fortunately, with the advance of modern bioinformatics technologies, our understanding of the molecular basis of cancer has significantly improved. In our present study, we used multiple databases to explore the difference among three subtypes of RCC, and investigate key genes and mechanism related to survival in KIRC. In conclusion, five immune-related genes, including ANK3, FREM2, KIF13B, MPP7 and SOX6, were identified to be as immune-related biomarkers for KIRC, and these genes may be feasible for predicting prognosis and immunotherapy efficacy. Our findings improve our understanding of the molecular basis of RCC, and provide the indication of targeting immune-related genes in RCC therapy. We present this article in accordance with the TRIPOD reporting checklist (available at https://tau.amegroups.com/article/view/10.21037/tau-24-225/rc).
Methods
Identification of differentially expressed genes (DEGs)
GEPIA2 website was used to analyze tumor and normal gene expression based on The Cancer Genome Atlas (TCGA) and Genotype-Tissue Expression data (13). The cut-off value of log2FC was set as 1, and q-value cutoff was set to 0.01. Next, a volcano plot and Venn diagram of DEGs were created by the Genescloud (https://www.genescloud.cn). The study was conducted in accordance with the Declaration of Helsinki (as revised in 2013).
Functional enrichment analysis
FLAME provides a combined approach through merging and visualizing results from extensively used functional enrichment analysis following a variety of input options (14). In our present study, FLAME was used to perform Kyoto Encyclopedia of Genes and Genomes (KEGG) pathway analysis, and the heatmap of KEGG pathways enriched in DEGs was also analyzed using FLAME database. GeneMANIA is an available, user-friendly website, which can provide a large amount of functional association data (15). GeneMANIA was used to establish gene networks and predict the gene enriched in KICH, KIRC and KIRP. In our study, those identified key genes were submitted to the GeneMANIA to illustrate the functional association network. The weighing method used was automatically selected.
Survival analysis
Survival analysis and the differential survival genes were obtained from GEPIA2 (http://gepia2.cancer-pku.cn/#survival). These genes were downloaded with the top 500 genes most relevant to overall survival in KIRP, KICH and KIRC, separately. The cutoff was defined based on the median. The survival analysis of five key genes screened by GEPIA2 and PrognoScan databases was further confirmed using UALCAN database. A value of P<0.05 was considered significant.
PrognoScan (http://dna00.bio.kyutech.ac.jp/PrognoScan/index.html) was used to further identify the key genes associated with KIRC prognosis, and evaluate the relationships between these key genes and patient outcomes in KIRC (16). The threshold of Cox P value was set as <0.05.
UALCAN is a comprehensive and interactive website for analyzing tumor data according to the TCGA project (17). UALCAN was applied to analyze the protein expression of key genes and validate the survival value of these genes.
Analysis of metastasis potential
The Cancer Dependency Map offers an available online database based on large-scale multi-omics screening projects, including Cancer Cell Line Encyclopedia (CCLE) (18). We used DepMap to analyze the dependencies between the key genes associated with survival and cancer cell lines. In the Correlation analysis of relative metastatic potential, we use Depmap (https://depmap.org/portal/data_explorer_2), in the X Axis module of plot Configuration, Expression was selected as Data Type, and MPP7, SOX6, ANK3, FREM2 and KIF13B were selected as Feature.
Immune cell infiltration analysis
To investigate the association between the levels of ANK3, FREM2, KIF13B, MPP7, SOX6 and immune infiltration in KIRC patients, the immune cell infiltration profile was identified via the Tumor Immune Estimation Resource (TIMER) and Translational Medicine Integrated Database (TISIDB) web portal resources. TISIDB incorporates numerous heterogeneous data types (19), and TIMER offers comprehensive analysis and visualization of cancer-immune system interactions (20).
Statistical analysis
All data were presented as the mean and standard deviation (SD). The Student’s t-test was used for comparing the difference between two groups. The log-rank test was used to analyze the survival curve. GraphPad Prism 8 (GraphPad Software) was used to create chart and common data analysis. The P value <0.05 was regarded as the level of statistically significant.
Results
Screening of DEGs
The GEPIA2 database was used to characterize DEGs profile in RCC and normal tissues. In total, 4,204, 2,408 and 2,955 DEGs were obtained for KICH, KIRP and KIRC, respectively (Figure 1A-1C). Result showed that KICH may be the most major subtype of RCC with the largest number of DEGs. Based on the DEGs for different RCC subtypes in the GEPIA2 dataset separately, we found that the intersection of the results provided 678 overlapping DEGs using Venn tool (Figure 1D). Among these DEGs, Venn diagram revealed that 533 DEGs were sole for KIRP, 1,105 DEGs were sole for KIRC, while 2,421 DEGs were solely for KICH (Figure 1D).
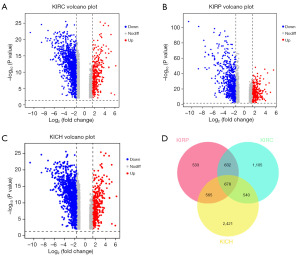
Visualization of KEGG enrichment analysis
KEGG pathway analysis was applied to display functions of DEGs. The DEGs in three RCC subtypes were involved in different pathways. Results showed that 4,204 DEGs for KICH were predominantly involved in tumor necrosis factor (TNF) signaling pathway, Rap1 signaling pathway and metabolic pathway (Figure 2A), and 2,408 DEGs for KIRP were mainly involved in Rap1 signaling pathway, calcium signaling pathway, metabolic pathway and phosphatidylinositol-3-kinase-protein kinase B (PI3K-Akt) pathway (Figure 2B), and 2,955 DEGs for KIRC were mainly involved in Rap1 and HIF-1 signaling pathway and PI3K-Akt pathway (Figure 2C). While 678 overlapping DEGs, among three different RCC subtypes, were mainly involved in metabolic pathway and PI3K-Akt pathway (Figure 2D). In addition, we generated a KEGG pathway heatmap based on the 678 overlapping DEGs. This analysis revealed that these DEGs significantly intersected with ten main pathways, including retinol metabolism, mineral absorption, metabolism of xenobiotics by cytochrome P450, PI3K-Akt signaling pathway, metabolic pathways, drug metabolism by cytochrome P450, complement and coagulation cascades, collecting duct acid secretion, cell adhesion molecules (CAMs), and arachidonic acid metabolism (Figure 2E). These results showed that tumors raised in the same organ had poor gene consistency due to transcription difference. These results indicated that transcriptomic profiling may contribute to identifying different RCC subtypes.
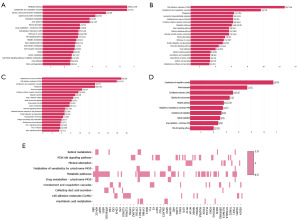
Correlation of DEGs with survival
Differential survival genes were obtained from the “Expression Analysis-Survival Analysis” module of GEPIA2. Furthermore, we intersected DEGs of transcriptome and overall survival (OS)-related differential survival genes. A total of 81 overlapping differential genes were identified between KIRC DEGs and KIRC OS (Figure 3A, and Table S1), while 68 overlapping differential genes were identified between DEGs and OS both in KICH and KIRP (Figure 3B,3C, and Tables S2,S3). Moreover, the correlation was analyzed based on the survival P value and |log2 fold change| of these overlapping differential genes. As shown in Figure 3, there was no direct relationship between the fold change of DEGs and the survival associations (Figure 3D-3F).
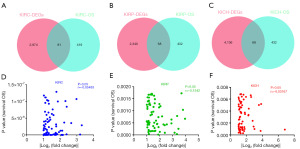
Protein-protein interactions (PPI) and prediction of gene function
A query gene list including all those overlapping genes identified above is shown in Figure 3. GeneMANIA was used to analyze the interactions between protein and predict gene function. The network showed co-expression 77.06%, co-localization 19.45%, shared protein domains 2.53%, and genetic interactions 0.96% based on the 81 overlapping genes in KIRC group (Figure 4A). The networks represented co-expression 81.92%, physical interactions 15.66%, co-localization 2.06% and pathways 0.07% based on the 68 overlapping genes in KIRP group (Figure 4B). While the networks represented only two tasks including co-expression 91.64% and co-localization 8.36% based on the 68 overlapping genes in KICH group (Figure 4C). KIRC is the most typical subtype of RCC, and accounts for 75% of all renal cancers (21). Thus, we next performed a more in-depth analysis focusing on KIRC. According to GEPIA2 database, 81 overlapping genes were obtained and further filtrated according to p value of overall survival, and the top 20 overlapping genes were further crossed with PrognoScan database. Finally, five key genes (ANK3, FREM2, KIF13B, MPP7 and SOX6) were confirmed to be strongly associated with prognosis in KIRC, according to the results of two interactive databases (Table S4). The survival value of these key genes was also validated using the UALCAN database (Figure 5A-5E), and we also utilized the Kaplan-Meier plotter dataset to evaluate the prognostic relevance of 5 key genes in KIRC based on their expression levels, revealing that their increase was all significantly linked with a greater overall survival in KIRC (Figure 5F-5J). Our analysis indicated that the low-expression group exhibited a shorter survival time.
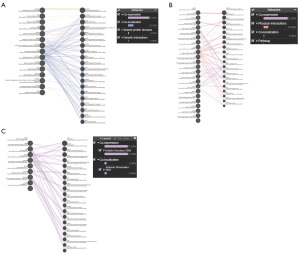
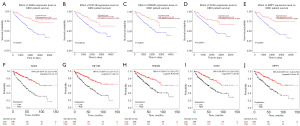
Validation and analysis of key genes expression
To obtain a comprehensive understanding of the expression levels of these key genes, we used UALCAN database to analyze the protein levels in KIRC. The results obtained from UALCAN showed that ANK3, FREM2, KIF13B, MPP7 and SOX6 were all significantly downregulated in KIRC (n=110), compared with the normal control (n=84) (Figure 6A-6E).
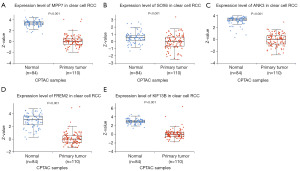
Key genes expression in different RCC and correlation with metastasis potential
We then analyzed the 5 key genes level in different renal cancer cell lines, and investigated the correlation between gene expression and renal cancer metastasis potential using Depmap database. The data for all renal cancer cell lines available in Depmap showed that the expression of 5 key genes were different in various renal cancer cell lines (Figure 7), although with lower expression in tumor tissue in KIRC (Figure 6). Metastasis potential results showed that there was no significant correlation with ANK3, FREM2, KIF13B, MPP7 and SOX6 expression in renal cancer cell lines (Figure 7).
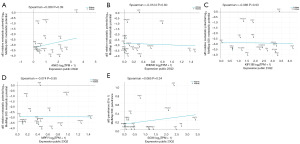
Correlation between downregulated key genes expression and chemokines receptors in KIRC patients
The correlation of the expression levels of ANK3, FREM2, KIF13B, MPP7 and SOX6 with chemokines receptors in KIRC was demonstrated in this study. Results implicated that the 5 key genes were interrelated with different chemokines receptors in KIRC (P<5.12E−12). ANK3 was mainly correlated with CCR10 (rho =−0.359) (Figure 8A), KIF13B (rho =−0.353), MPP7 (rho =−0.346) and SOX6 (rho =−0.369) were all significantly correlated with CXCR4, while FREM2 was significantly connected with CXCR5 (rho =−0.294) (Figure 8). These findings further revealed that all these 5 key genes may serve as immunoregulatory elements in KIRC.
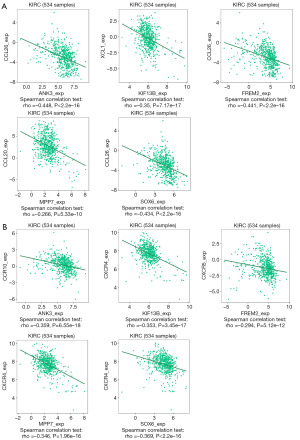
Correlation between downregulated key genes expression and immune infiltration in KIRC
Immune infiltration around tumors is a crucial factor correlated with tumor progression. Therefore, 5 key genes were submitted to the TIMER database to explore their associations with 6 tumor-infiltrating immune cells (CD8+ T cells, B cells, CD4+ T cells, neutrophil, macrophage and dendritic cells), and tumor purity. Our analysis results found that ANK3 was positively associated with B cells (Figure 9A), while KIF13B was positively correlated with CD4+ T cells (Figure 9B). FREM2 was positively correlated with macrophages, as was MPP7 and SOX6 (Figure 9C-9E). These results showed that 5 key genes expression were all correlated with immune infiltration in KIRC, which might be a potential mechanism to exert the effects on prognosis.
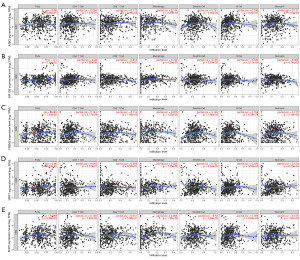
Discussion
Bioinformatics, as an interdisciplinary field of science, provides more significant insights and data related to molecular mechanisms of cancer progression. The identification of potential key genes and understanding their interactions contribute significantly to the discovery of promising prognostic, predictive biomarkers and novel clues for therapies in RCC. In our study, to explore the unique and shared genes signature in different subtype of RCC, we first performed RNA-seq analysis among KIRC, KIRP and KICH samples from gepia2 database. In our present study, 4,204, 2,408 and 2,955 DEGs were identified in KICH, KIRP and KIRC, respectively. Guo et al. identified 5,699, 4,896 and 5,759 DEGs in KIRC, KIRP and KICH from TCGA cohort, respectively, and found that cell migration and immune response process were mainly enriched in KIRC and KIRP, but more synaptic plasticity and kidney morphogenesis were involved in KICH (22). These results indicated that RCC patients of different subtypes with different originating cell types had different transcript response, although tumors occurred in the same organ.
The most typical subtype of RCC is KIRC, which accounts for about 80–90% of RCC with a poor prognosis (23). In the meantime, the pathogenesis of KIRC remains unclear, and no sensitive biomarkers have been discovered. Therefore, we paid more attention to KIRC in our research. We further screened 5 key genes associated with KIRC survival through cross-database analysis. Our results showed that 5 key genes had a similar trend in KIRC, compared to normal control. FREM2, MPP7 and SOX6 were suggested to be potential candidates for KIRC prognosis prediction (24-27), which is consistent with our present results. Although ANK3 was reported to be only associated with RCC in previous publication (28), our study further proved that ANK3 was a novel survival-associated gene in KIRC. Previous studies have reported that low FREM2 expression in KIRC patients showed a high propensity for metastasis and poor prognosis (29). MPP7 and SOX6 were also identified to be associated with breast cancer and liver metastasis (30,31). These results showed that five key genes associated with metastasis are potential immune-related biomarkers in multiple cancers. Furthermore, our present study systematically explored the relationships between these key genes expression and KIRC in bioinformatics manner, and our results showed that all these 5 key genes expressions were related with different chemokines (receptors) (Figure 8) and infiltration of different immune cells (Figure 9).
Numerous studies have proved that chemokines and their receptors modulate tumor microenvironment (TME) and affect the development of cancers and the therapeutic outcomes (32-34). In our present study, we found that all 5 key genes were correlated with different chemokines and chemokines receptors (Figure 8). Enhanced immune infiltration in tumors has been proved to be typically associated with good prognosis in tumor patients, including KIRC (26,35). Furthermore, we found that these 5 key genes were closely correlated with different immune cells (Figure 9). In addition, we found no correlations between these genes and metastasis potential (Figure 7). These results revealed that ANK3, FREM2, KIF13B, SOX6 and MPP7 may serve as crucial immunoregulatory elements in KIRC patients, and we speculated that these novel survival-related genes may be involved in the development of KIRC through immunomodulation.
Undeniably, our present study has certain limitations. Although we conducted integrated bioinformatics analysis on multiple datasets to explore the differences among three subtypes of RCC, and mainly focused on analysis of key genes and mechanisms related to survival in KIRC, we did not carry out corresponding experimental verification in vitro or in vivo, which will be improved in future research. In conclusion, our present findings provide valuable clues for future studies, and foundation for our further validation of the present findings, and a deeper understanding of the mechanisms.
Conclusions
In summary, we identified five key genes that were correlated with prognosis and immunotherapy for KIRC. Our findings improve our understanding of the function of these immune-related novel prognostic biomarkers in KIRC, while the crucial factors that lead to the downregulation and functional inactivation of these five key new immune-related prognostic biomarkers need to be explored in future studies.
Acknowledgments
We would like to thank Nana Wei for her help in polishing our paper.
Funding: None.
Footnote
Reporting Checklist: The authors have completed the TRIPOD reporting checklist. Available at https://tau.amegroups.com/article/view/10.21037/tau-24-225/rc
Peer Review File: Available at https://tau.amegroups.com/article/view/10.21037/tau-24-225/prf
Conflicts of Interest: All authors have completed the ICMJE uniform disclosure form (available at https://tau.amegroups.com/article/view/10.21037/tau-24-225/coif). The authors have no conflicts of interest to declare.
Ethical Statement: The authors are accountable for all aspects of the work in ensuring that questions related to the accuracy or integrity of any part of the work are appropriately investigated and resolved. The study was conducted in accordance with the Declaration of Helsinki (as revised in 2013).
Open Access Statement: This is an Open Access article distributed in accordance with the Creative Commons Attribution-NonCommercial-NoDerivs 4.0 International License (CC BY-NC-ND 4.0), which permits the non-commercial replication and distribution of the article with the strict proviso that no changes or edits are made and the original work is properly cited (including links to both the formal publication through the relevant DOI and the license). See: https://creativecommons.org/licenses/by-nc-nd/4.0/.
References
- Zheng Z, Yang T, Li Y, et al. A future directions of renal cell carcinoma treatment: combination of immune checkpoint inhibition and carbon ion radiotherapy. Front Immunol 2024;15:1428584. [Crossref] [PubMed]
- Li Y, Lih TM, Dhanasekaran SM, et al. Histopathologic and proteogenomic heterogeneity reveals features of clear cell renal cell carcinoma aggressiveness. Cancer Cell 2023;41:139-163.e17. [Crossref] [PubMed]
- Sharma R, Kadife E, Myers M, et al. Determinants of resistance to VEGF-TKI and immune checkpoint inhibitors in metastatic renal cell carcinoma. J Exp Clin Cancer Res 2021;40:186. [Crossref] [PubMed]
- Linehan WM, Ricketts CJ. The Cancer Genome Atlas of renal cell carcinoma: findings and clinical implications. Nat Rev Urol 2019;16:539-52. [Crossref] [PubMed]
- Zhao H, Ljungberg B, Grankvist K, et al. Gene expression profiling predicts survival in conventional renal cell carcinoma. PLoS Med 2006;3:e13. [Crossref] [PubMed]
- Chang K, Yuan C, Liu X. Ferroptosis-Related Gene Signature Accurately Predicts Survival Outcomes in Patients With Clear-Cell Renal Cell Carcinoma. Front Oncol 2021;11:649347. [Crossref] [PubMed]
- Wang Y, Chen Y, Zhu B, et al. A Novel Nine Apoptosis-Related Genes Signature Predicting Overall Survival for Kidney Renal Clear Cell Carcinoma and its Associations with Immune Infiltration. Front Mol Biosci 2021;8:567730. [Crossref] [PubMed]
- Xing Q, Zeng T, Liu S, et al. A novel 10 glycolysis-related genes signature could predict overall survival for clear cell renal cell carcinoma. BMC Cancer 2021;21:381. [Crossref] [PubMed]
- Zhengqi Q, Zezhi G, Lei J, et al. Prognostic role of PHYH for overall survival (OS) in clear cell renal cell carcinoma (ccRCC). Eur J Med Res 2021;26:9. [Crossref] [PubMed]
- Yuan H, Qin X, Wang J, et al. The cuproptosis-associated 13 gene signature as a robust predictor for outcome and response to immune- and targeted-therapies in clear cell renal cell carcinoma. Front Immunol 2022;13:971142. [Crossref] [PubMed]
- McKay RR, Bossé D, Xie W, et al. The Clinical Activity of PD-1/PD-L1 Inhibitors in Metastatic Non-Clear Cell Renal Cell Carcinoma. Cancer Immunol Res 2018;6:758-65. [Crossref] [PubMed]
- Gupta R, Ornstein MC, Li H, et al. Clinical Activity of Ipilimumab Plus Nivolumab in Patients With Metastatic Non-Clear Cell Renal Cell Carcinoma. Clin Genitourin Cancer 2020;18:429-35. [Crossref] [PubMed]
- Tang Z, Li C, Kang B, et al. GEPIA: a web server for cancer and normal gene expression profiling and interactive analyses. Nucleic Acids Res 2017;45:W98-W102. [Crossref] [PubMed]
- Karatzas E, Baltoumas FA, Aplakidou E, et al. Flame (v2.0): advanced integration and interpretation of functional enrichment results from multiple sources. Bioinformatics 2023;39:btad490. [Crossref] [PubMed]
- Franz M, Rodriguez H, Lopes C, et al. GeneMANIA update 2018. Nucleic Acids Res 2018;46:W60-4. [Crossref] [PubMed]
- Mizuno H, Kitada K, Nakai K, et al. PrognoScan: a new database for meta-analysis of the prognostic value of genes. BMC Med Genomics 2009;2:18. [Crossref] [PubMed]
- Chandrashekar DS, Karthikeyan SK, Korla PK, et al. UALCAN: An update to the integrated cancer data analysis platform. Neoplasia 2022;25:18-27. [Crossref] [PubMed]
- Ghandi M, Huang FW, Jané-Valbuena J, et al. Next-generation characterization of the Cancer Cell Line Encyclopedia. Nature 2019;569:503-8. [Crossref] [PubMed]
- Ru B, Wong CN, Tong Y, et al. TISIDB: an integrated repository portal for tumor-immune system interactions. Bioinformatics 2019;35:4200-2. [Crossref] [PubMed]
- Li T, Fan J, Wang B, et al. TIMER: A Web Server for Comprehensive Analysis of Tumor-Infiltrating Immune Cells. Cancer Res 2017;77:e108-10. [Crossref] [PubMed]
- Zhang D, Zeng S, Hu X. Identification of a three-long noncoding RNA prognostic model involved competitive endogenous RNA in kidney renal clear cell carcinoma. Cancer Cell Int 2020;20:319. [Crossref] [PubMed]
- Guo J, Lv X, He B, et al. Molecular Signature of Subtypes of Renal Cell Carcinomas and Immunotherapy Strategy. SSRN Electronic Journal 2021.
10.21203/rs.3.rs-847344/v1 10.21203/rs.3.rs-847344/v1 - Miller KD, Nogueira L, Devasia T, et al. Cancer treatment and survivorship statistics, 2022. CA Cancer J Clin 2022;72:409-36. [Crossref] [PubMed]
- Huang W, Lu Y, Huang X, et al. FREM2 is an independent predictor of poor survival in clear cell renal cell carcinoma-evidence from the cancer genome atlas (TCGA). Int J Clin Exp Med 2019;12:13741-8.
- Chen L, Xie Y, Ma X, et al. SOX6 represses tumor growth of clear cell renal cell carcinoma by HMG domain-dependent regulation of Wnt/β-catenin signaling. Mol Carcinog 2020;59:1159-73. [Crossref] [PubMed]
- Xiong X, Chen C, Yang J, et al. Characterization of the basement membrane in kidney renal clear cell carcinoma to guide clinical therapy. Front Oncol 2022;12:1024956. [Crossref] [PubMed]
- Cheng X, Sun D, Li H, et al. MPP7 is a potential prognostic marker and is associated with cancer metabolism and immune infiltration in clear cell renal cell carcinoma: a bioinformatics analysis based on the TCGA database. Transl Androl Urol 2023;12:642-58. [Crossref] [PubMed]
- Tan Y, Meng W, Jiang Z, et al. A comprehensive analysis of the prognostic and immunological role of ANK3 in pan-cancer. Transl Cancer Res 2024;13:1091-113. [Crossref] [PubMed]
- Wang G, Wang Z, Lu H, et al. Comprehensive analysis of FRAS1/FREM family as potential biomarkers and therapeutic targets in renal clear cell carcinoma. Front Pharmacol 2022;13:972934. [Crossref] [PubMed]
- Jiang W, Yuan Q, Jiang Y, et al. Identification of Sox6 as a regulator of pancreatic cancer development. J Cell Mol Med 2018;22:1864-72. [Crossref] [PubMed]
- Liao W, Fan L, Li M, et al. MPP7 promotes the migration and invasion of breast cancer cells via EGFR/AKT signaling. Cell Biol Int 2021;45:948-56. [Crossref] [PubMed]
- Huang CG, Liu Q, Zheng ST, et al. Chemokines and Their Receptors: Predictors of Therapeutic Potential in Tumor Microenvironment on Esophageal Cancer. Dig Dis Sci 2024;69:1562-70. [Crossref] [PubMed]
- Mehraj U, Mushtaq U, Mir MA, et al. Chemokines in triple-negative breast cancer heterogeneity: New challenges for clinical implications. Semin Cancer Biol 2022;86:769-83. [Crossref] [PubMed]
- Saxena S, Singh RK. Chemokines orchestrate tumor cells and the microenvironment to achieve metastatic heterogeneity. Cancer Metastasis Rev 2021;40:447-76. [Crossref] [PubMed]
- Xiong TF, Pan FQ, Liang Q, et al. Prognostic value of the expression of chemokines and their receptors in regional lymph nodes of melanoma patients. J Cell Mol Med 2020;24:3407-18. [Crossref] [PubMed]