Depletion of intrinsic renal macrophages with moderate-to-high expression of CD163, MRC1, PTH2R, PDE4D, and CUBN in regulating podocyte injury in diabetic nephropathy: a single-cell RNA sequencing analysis
Highlight box
Key findings
• The study identified a significant depletion of a specific subset of renal macrophages (Mφs) with a moderate-to-high expression of CD163, MRC1, PTH2R, PDE4D, and CUBN in diabetic nephropathy (DN), which is linked to increased podocyte (PODO) injury and apoptosis. This finding underscores the critical role of these Mφs in safeguarding against the progression of DN.
What is known and what is new?
• DN is a leading cause of end-stage renal disease, with PODO damage being central to its progression. Mφs contribute to renal inflammation and fibrosis, but their specific roles in DN are not fully understood.
• This study highlights the importance of a distinct subset of renal Mφs in maintaining PODO health. It reveals that their depletion in DN, alongside the overexpression of AHR and underexpression of IGF1R, provides new insights into the mechanisms driving PODO injury in DN.
What is the implication, and what should change now?
• The findings suggest that preserving or restoring this specific Mφ subset could effectively prevent or slow the progression of DN. This study introduces a novel perspective on these protective Mφs, identifying their loss as a pivotal factor in PODO damage and presenting a promising therapeutic target. Moving forward, research should prioritize strategies to restore or preserve this Mφ subset in potentially mitigating DN progression and enhancing renal function.
Introduction
Diabetic nephropathy (DN) is a common complication of diabetes, with a high incidence rate and high disability rate (1,2). According to statistics, 30–40% of diabetes patients are prone to develop DN, of whom 5–10% eventually develop end-stage renal disease (ESRD) (3,4), which is the ultimate cause of death in patients with diabetes. Therefore, the prevention and treatment of DN and the reduction of ESRD development are critical objectives in the clinical practice of diabetes.
It is currently believed that the mechanism underlying the pathogenesis and progression of DN involves the glomerulus being continuously exposed to a high-glucose environment in diabetic kidneys, which results in abnormal glucose metabolism and the production of a large number of advanced glycation end-products (AGEs) and reactive oxygen species (ROS). AGEs and ROS activate protein kinase C (PKC), Janus kinase (JAK) signal transducer and activator of transcription (STAT) pathway, and nuclear factor-kappa beta (NF-κB)-related signaling pathways in podocyte (PODO), which alter the production or degradation of extracellular matrix (ECM) components, reshape actin cytoskeleton, alter the PODO phenotype, and cause PODO hypertrophy or apoptosis (5,6). The excessive production of ECM by PODOs leads to the thickening of the glomerular basement membrane (GBM), an early symptom of DN that can lead to secondary glomerulosclerosis. The loss of PODOs leads to a decrease in the glomerular protein filtration rate and proteinuria in patients. In addition, AGEs bind to receptors for AGE (RAGE) in PODO and then induce the expression and activation of proinflammatory factors including interleukin (IL)-1β, IL-18, transforming growth factor-beta 1 (TGF-β1), tumor necrosis factor-alpha (TNF-α), and fibrotic proteins including intercellular adhesion molecule-1 (ICAM-1), and NF-κB through the inflammasome of nucleotide-binding oligomerization domain (NOD)-like receptor pyrin domain containing 3 (NLRP3) (7-10). Therefore, it is believed that a high-glucose environment induces oxidative stress damage in PODO, promotes the synthesis and secretion of inflammatory factors, and further contributes to inflammation. Persistent inflammation induces the synthesis and secretion of fibrotic proteins, which in turn leads to renal fibrosis (11,12). Diabetic kidney disease (DKD) is a chronic renal injury caused by diabetes, characterized by renal inflammation, fibrosis, and functional decline. In a hyperglycemic environment, oxidative stress and the accumulation of AGEs trigger inflammatory responses, inducing tubular epithelial cells and glomerular cells to secrete pro-inflammatory cytokines such as TNF-α, IL-6, and monocyte chemoattractant protein-1 (MCP-1) (13,14). These factors recruit monocytes into renal tissues, where they differentiate into macrophages (Mφs). The polarization of Mφs toward the M1 phenotype (pro-inflammatory) exacerbates inflammation and tissue damage, while impaired M2 phenotype (anti-inflammatory) activity diminishes tissue repair. Therapeutic targets for DKD include inhibiting the AGEs-RAGE signaling pathway, blocking pro-inflammatory cytokines like TNF-α and IL-6, modulating Mφ polarization to promote M2 anti-inflammatory responses, and targeting fibrosis-related pathways such as TGF-β/Smad signaling (15,16). These strategies show promise in alleviating inflammation and slowing the progression of renal dysfunction.
Interestingly, renal Mφs were shown to play an important role in the regulation of inflammation in DN. Renal Mφs include kidney resident Mφs (KRMs) and circulating Mφs (cirMφs). KRMs are derived from erythrocyte-myeloid progenitor (EMP) cells, fetal liver EMP cells, and hematopoietic stem cells (HSCs). In the early stage of DN, KRMs are the earliest to be activated in initiating or suppressing immune responses. After injury, PODO secretes ICAM-1, vascular cell adhesion molecule-1 (VCAM-1), and MCP-1 to recruit cirMφs. According to classical phenotype classifications, Mφs in DN include the inflammatory M1 phenotype (CD68+/iNOS+) and the M2 phenotype (CD68+/Arg-1+). The M1:M2 ratio changes dynamically in DN according to the stage of progression. An increased proportion of M1 Mφs in the glomerulus during early DN (stage I and stage IIa) induces proinflammatory factor secretion (TNF-α, IL-1β, IL-6) through the expression of iNOS, which activates apoptosis signals such as TRAIL in PODO and ultimately induces PODO damage and apoptosis. Activated M1 Mφs also activate Smad3 by secreting TGF-β1 and induce the secretion of collagen proteins (COLs) such as COL4s in PODO, which causes ECM deposition, thereby promoting renal inflammation and injury. Subsequently, the proportion of M2 Mφs increases in the late stage (DN-III), but the role and mechanism of M2 in the DN process are unclear (17-20). In addition, there are CD14+ Mφs in the outer cortex and medulla of the kidney, which according to disease models, regulate the chemotaxis and activation of neutrophils. CD206+ (MRC1+) Mφs are also present in the renal medulla, and these affect cell growth and ECM secretion by regulating the transcription of STAB1, FCRLs, and IGF1, thereby participating in tissue repair and fibrosis processes. Therefore, in addition to the classical Mφ phenotype, there are other Mφ phenotypes in the kidney, which play a role in promoting renal interstitial fibrosis, tubular atrophy, and glomerulosclerosis by regulating immune response and ECM composition at different stages of DN (21,22).
Importantly, a study on this subject has all used classical markers (CD68, CD86, iNOS, CD163, etc.) to classify the Mφ phenotype, but this cannot comprehensively identify Mφs in DN, leading to certain Mφ phenotypes which have been ignored within this context (23). In addition, the impact of the origin of renal Mφs on phenotype and function has been neglected, leading discrepant results among the studies in this field. Therefore, we analyzed the single-cell RNA sequencing (scRNA-seq) of kidney samples from patients with DN to subdivide Mφs and analyzed the regulatory effects of different subsets of Mφ on inflammation, regulation of PODO damage, and promotion of renal fibrosis (the procedure of the analysis is shown in Figure 1). We present this article in accordance with the MDAR reporting checklist (available at https://tau.amegroups.com/article/view/10.21037/tau-24-569/rc).
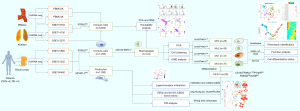
Methods
Source of renal tissue in human DN and acquisition of scRNA-seq data
We accessed the Gene Expression Omnibus (GEO) database, including data sets GSE131882 (24,25), GSE151302 (26,27), and GSE195460 (28), to download the single-nuclear RNA sequencing (snRNA-seq) data of 11 kidney samples comprising 38,654 genes and 73,784 cells. In snRNA-seq data, renal cortical tissues [control (CON) =6; DN =5] were obtained from patients with renal mass/urothelial carcinoma after nephrectomy (n=10) or deceased organ donors (n=1). The patients’ age ranged from 50 to 78 (59.091±9.332) years, and there were five women and six men. There was no significant difference in the glomerular filtration rate (GFR) between CON and DN groups (CON: 74.612±15.505 vs. DN: 78.972±17.043 mL/min/1.73 m2). Among the patients with diabetes, 80% (4/5) had proteinuria (>150 mg/24 h), glomerulosclerosis occurrence rate of 10–50%, and interstitial fibrosis and tubular atrophy (IFTA; rate >10%), while 40% (2/5) of patients had hypertension. One patient developed severe arteriosclerosis (Table S1).
In addition, in order to prevent the impact of low-quality cells on the results before data analysis, cells with a gene number of less than 500 and more than 5,000, a total number of messenger RNA (mRNA) molecules greater than 16,000, and an average mitochondrial gene proportion greater than 0.5% or that of ribosomal genes greater than 0.3% were first filtered. The resulting 51,640 cells were subjected to standardization, principal component analysis (PCA), dimensionality reduction, and cluster analysis. In addition, since the obtained data genes were chip identifiers (CHIPIDs), annotation files gencode.v37.basic.annotation.gtf and gencode.v42.annotation.gtf from GENCODE (https://www.gencodegenes.org/human/) were downloaded in order to convert CHIPIDs to gene symbols. The study was conducted in accordance with the Declaration of Helsinki (as revised in 2013).
Identification and acquisition of Mφs
As 51,640 cells (CON =37,167; DN =14,473) were from different batches of samples, there was a possibility of data bias. Thus, we used SCTransform from Seurat v. 4.3.0 (https://satijalab.org/seurat) to build a regulated negative binary expression model to standardize unique molecular identifier (UMI) counts. This could reduce the impact of sequencing depth. “RunPCA” was used to reduce the dimension of cells according to the top 3,000 most hypervariable genes. “RunHarmony” was used to linearly correct each principal component (PC) to reduce batch effects. “FindNeighbors” and “FindClusters” were used to select Pcs with cumulative contributions greater than 90% and differential contributions less than 5% (1:13) to calculate the k-nearest neighbors and sort cells into different clusters with a resolution of 0.6. “FindAllMarkers” was used to perform a Wilcoxon rank-sum test on genes in different clusters of cells, and then significantly differentially expressed genes (DEGs) with adjusted P values <0.05 and log2fold change (log2FC) >0 were selected as markers for different. Compared to other cells, those significantly overexpressing PTPRC (PTPRChig) were considered immune cells (IMCs), while those significantly overexpressing CD163 (CD163hig) and MRC1 (MRC1hig) were considered Mφs. To further identify Mφs, markers from CellMarker2.0 (http://117.50.127.228/CellMarker/) were used and then renal Mφs tha were CD163+, CD68+, FCGR3A+, FCGR1A+, C1QA+, C1QB+, MRC1+, or LYZ+ were screened. Consequently, 144 Mφs were obtained (CON =35; DN =109).
In addition, in order to determine whether there was change in the proportion of Mφs between different groups of kidneys, we calculated the proportion of Mφ relative to IMCs (n=1,295) in six CON and five DN samples and used the Wilcoxon rank-sum test to assess the differences between them.
Analysis of kidney Mφ types
As mentioned above, there are two main types of renal Mφs, KRMs, and cirMφs. To determine which of these were present in the 144 cells, we merged the scRNA-seq data from other kidney tissues [GSE118184 (29) and GSE114156 (30)—three healthy adult kidney samples, all from a discarded human donor kidney—and GSE112570 (31)—two fetal kidney tissue samples obtained from the Los Angeles Children’s Hospital for selective termination of pregnancy] with data of peripheral blood mononuclear cells [PBMCs; PBMC3K (32) and PBMC4K (33)]. Data were standardized using the same method described above, and then PCA and removal of batches were performed. Cells were dimensionally reduced and clustered based on the first Pcs. Following this, t-distributed stochastic neighbor embedding (tSNE) dimensionality reduction technique of Mφs from different sources was performed. In addition, we labeled CD14 to observe the distribution of monocytes. We used “FindAllMarkers” (v4.3.0; https://satijalab.org/seurat) to identify key DEGs in distinguishing KRMs from other Mφs.
Phenotype and function of renal Mφs
The normalized standardized contrast test (SCT) data of 144 Mφs were redimensioned and clustered using tSNE. A cluster of cells was considered a Mφ phenotype. “FindAllMarkers” (v4.3.0; https://satijalab.org/seurat) was used to obtain the marker genes for each cell cluster. The expression levels of CD163 and MRC1, along with top 3 DEGs in cell clusters, were used as markers for each Mφ subtype. The irGSEA.score in AUCell v. 1.18.1 and irGSEA v. 1.1.3 was used to calculate AUCell enrichment scores of the different phenotypes of Mφs. Additionally, the Wilcoxon rank-sum test was used to determine the significant differences in the enrichment scores of various Mφ subpopulations. The enriched gene sets with a P value less than 0.05 were considered statistically significant. In addition, the Gene Ontology (GO) and the Kyoto Encyclopedia of Genes and Genomes (KEGG) pathways that significantly enriched in the Mφ subtypes were analyzed using org.Hs.eg.db v. 3.15.0 and clusterProfiler v. 4.4.4 (Bioconductor). The hypergeometric distribution test was conducted to screen significant GO items and KEGG pathways [false discovery rate (FDR) <0.05]. The functions of each Mφ phenotype were determined based on single-cell gene pool enrichment results. In this step, the Wilcoxon rank-sum test was also employed to analyze the differences in the proportions of different Mφ phenotypes between the groups.
Differentiation status of renal Mφs
To clarify the correlation between changes in cell state among different Mφ clusters, we conducted pseudotiming analysis using Monocle v. 2.26.0. “NewCellDataSet” (https://github.com/cole-trapnell-lab) was used to build objects. The “estimateSizeFactors” and “estimateDispersion” packages (https://bioconductor.org) were used to initialize the data. “SetOrderingFilter” (http://cole-trapnell-lab.github.io) was used to label the markers of Mφs. “ReduceDimension” (https://satijalab.org/seurat) was used to reduce the dimension of Mφs under the parameter of max components =2 and method = “DDRTree”. “OrderCells” (https://bioconductor.org) was used to sort cells in pseudo time. “DifferentialGeneTest” (https://satijalab.org/seurat) was used to correct the DEGs according to pseudotiming. We then divided the cells into five-time stages based on changes in gene expression and analyzed the temporal state changes of Mφs with different phenotypes. In this step, we screened Mφs that were in the final stage and exhibited significant changes in proportions for subsequent functional analysis.
Differential gene expression and functional enrichment analysis of renal Mφs in the terminal stage
We obtained 29 key Mφs (CON =18; DN =11). The “FindMarkers” (v4.3.0; https://satijalab.org/seurat) function and Wilcoxon test were used to analyze the differential expression and significance of Mφ genes in the different groups. DEGs (P<0.05) were screened, and their significantly enriched biological processes (BPs), molecular functions (MFs), cellular components (CCs), and KEGG pathways were analyzed. We used string v. 11.5 (https://cn.string-db.org/) to analyze the interaction between DEGs. A protein-protein interaction (PPI) network was constructed using Cytoscape v. 3.9.1, and the Molecular Complex Detection (MCODE) plug-in was used to calculate and cluster the Euclidean distance (K-means) between DEGs to obtain the core PPI (k>5). cytoHubba (v.3.0; https://apps.cytoscape.org/apps/cytohubba) was used to obtain the top 10 central DEGs in PPI using the maximal clique centrality (MCC) algorithm. We further analyzed the difference between the CON and DN groups in terms of gene expression in the core PPI network for the overall tissues.
Cross-connection between renal Mφs and renal cells
In addition to Mφs, we also identified the proximal tubules (PTs), PODOs, endothelial cells (ENDOs), parietal epithelial cells (PECs), and thick ascending limbs (TALs), among others (Table S2) between renal Mφs and renal cells. To clarify the effects of Mφs on other renal tissue cells in the terminal stage of DN. We used celltalker v. 0.0.7.9000 and cellphoneDB v. 3.0 software to analyze ligand-receptor pairs (LRPs) for PODO, ENDO, TAL, PT, and PEC interactions with advanced-stage Mφs. The renal tissue cell type (PODO) with the strongest interaction with Mφs was then selected. LRPs that were significantly differentially expressed in Mφs, PODOs, and overall renal tissue were obtained.
Functional analysis of PODOs in DN
PODOs have a strong interaction with end-stage Mφs and participate in glomerulosclerosis in DN. We screened 1,392 PODOs (CON =838, DN =554) with high expression of NPHS1 (NPHS1hig) and NPHS1 (NPHS2hig) (genehig > Σlog2UMI count/1,392). Similarly, “FindMarkers” (v4.3.0; https://satijalab.org/seurat) was used to obtain the DEGs of the PODOs in DN. Subsequently GO enrichment analysis and PPI analysis of the DEGs were conducted in order to identify the significantly different signaling pathways among PODOs in DN and the relevant core gene clusters (k>3).
Statistical analysis
The analysis was conducted in R v. 4.2.1 (The R Foundation for Statistical Computing, Vienna, Austria) and Rstudio server. The differences between groups were analyzed via the Wilcoxon rank-sum test. P<0.05 indicated a statistically significant difference.
Results
The proportion of Mφ was significantly decreased in patients with DN
To determine the proportion of Mφs in DN, we first obtained the IMCs (PTPRChig, cell clusters with mean >1) in all samples. The proportion of IMCs in kidney was 2.51% (1,295/51,640; Figure 2 and Figure S1). It has been demonstrated that the number of IMCs in DN (1,127/14,473, 7.787%) was about 17 times greater than that in CON (168/37,167, 0.452%). After further clustering and annotation of IMCs, 234 Mφs were obtained (CD163higMRC1hig, cluster 1 with mean >0.5), accounting for 18.07% of PTPRChig cells (Figure 3 and Figure S2). Compared with that of the CON group (62/168; 52.11%±23.14%), the relative proportion of Mφs in the DN group (172/1,127, 14.59%±15.75%) was significantly lower (P<0.05) (Figure 3E). It should be noted that the results from the original single dataset showed no significant changes in the proportion of Mφs (5-8), and clinical and animal experimental study (21) reported a significant increase in Mφ infiltration in DN kidneys; however, our findings were inconsistent with both these sources: after integrating multiple data sets, we found that the proportion of Mφs was DN decreased. This discrepancy may be related to the heterogeneity of Mφs.
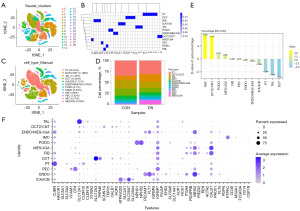
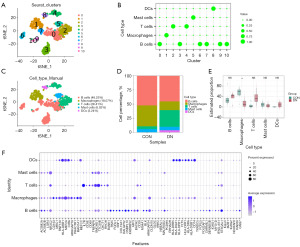
To clarify the correlation between heterogeneity and origin of Mφs (KRMs or differentiated cells after infiltration of blood monocytes), we used scRNA-seq from PBMCs, fetal kidney IMCs, and adult renal cortex IMCs and then conducted PCA and tSNE dimensionality reduction. The results showed that the transcriptional characteristics of fetal kidney IMCs and PBMCs tended to be consistent. The transcriptional characteristics of adult renal Mφs were different from those of PBMCs, with a certain difference between their Pcs and tSNE distributions. The transcription characteristics of Mφs were mainly similar to those of IMCs in the adult renal cortex (Figure 4A and Figure S3A,S3B). Thus, adult renal Mφs may be originated from KRMs.
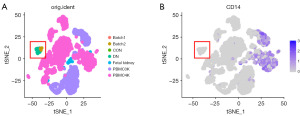
To further distinguish KRMs from cirMφs, we labeled Mφs with CD14. CD14 was found to be especially expressed in CD68+ PBMCs (34,35) (CD14+: 719/1,544, 46.567%; Figure S3C,S3D). KRMs had a lower expression of CD14 compared to PBMCs (0.204±0.470 vs. 0.097±0.316, P<0.01; Figure 4B and Figure S3A). Additionally, we obtained the markers for PBMCs and KRMs to identify both. Compared to KRMs, PBMCs had higher levels of LYZ (1.321±1.527 vs. 0.066±0.254, P<0.001) and lower levels of CD163 (0.025±0.138 vs. 1.122±0.802, P<0.001) and MRC1 (0.001±0.0190 vs. 1.085±0.994, P<0.001) (Figure S3A,S3E). The CD163higMRC1higLYZlow cells [CD163higMRC1hig: log2UMI count > mean (log2UMI count of all cells); LYZlow: log2UMI count < mean (log2UMI count of all cells)] were likely present in the Mφs of kidneys.
Identification of Mφ phenotypes in DN
Some cells in cluster 1 (90/234) annotated as Mφs did not express the typical markers of CD163, CD68, FCGR3A, FCGR1A, C1QA, C1QB, MRC1, or LYZ, although they have similar transcriptional characteristics to those of Mφs (Figure 5A). Excluding these cells, the remaining 144 Mφs were redimensionally reduced for cluster analysis. According to the characteristics of the transcriptome, they were divided into four different clusters (Mc0–3; Figure 5B), and their relative proportions from high to low were ranked as follows: Mc0 (47/144, 32.64%), Mc1 (41/144, 28.47%), Mc2 (30/144, 20.83%), and Mc3 (26/144, 18.06%). The proportions of Mc0–3 in the CON group were 51.43% (18/35), 0% (0/35), 0% (0/35) and 31.43% (11/35), respectively, while those in the DN group were 21.10% (23/109), 37.61% (41/109), 27.52% (30/109), and 13.76% (15/109), respectively. Mc1 and Mc2 were present in the DN group but not in the CON group (Figure 5C).
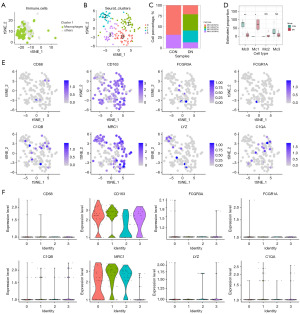
The decrease in the relative proportion of IMCs DN was mainly reflected in Mc0 and Mc1. Compared with the CON group (Mc0: 24/35, 68.49%±31.13%; Mc1: 0/35, 0%), the relative proportion of Mc0 in the DN group (23/109, 9.62%±11.54%) was significantly lower (P<0.01), while that of Mc1 (41/109, 69.78%±35.31%) was significantly higher (P<0.05). There was no significant difference in the relative proportion of Mc2 and Mc3 cells between the CON group (Mc2: 0/35, 3.66%±4.67%; Mc3: 11/35, 31.51%±31.13%) and the DN group (Mc2: 30/109, 8.24%±16.48%; Mc3: 15/109, 12.36%±18.15%) (Figure 5D).
At this point in the study, it could be discerned that there were four subtypes of KRMs in DN, with a loss Mc0 being the most common. To distinguish the KRM phenotype, we first examined the expression levels of CD163 and MRC1. The results showed that there were significant differences in the expression of CD163 and MRC1 among the four KRM types. Both Mc0 (CD163higMRC1mid) and Mc1 (CD163higMRC1hig) were CD163-overexpressing cells but had a significant difference in MRC1 expression. Mc2 showed a low level of CD163 expression (CD163low) and a high level of MRC1 expression (MRC1hig), while Mc3 was characterized as CD163higMRC1low (Figure 5E,5F and Table 1). According to further labeling of KRMs phenotypes with the top 3 DEGs, Mc0, Mc1, Mc2, and, Mc3 were respectively characterized as PTH2RhigPDE4DhigCUBNhig, CD163L1higF13A1higRGL1hig, IL1R2higJAMLhigCCDC26hig, and TANC2higPLXDC2higADGRB3hig (Figure S4 and Figure 6A). The depletion of CD163higMRC1midPTH2RhigPDE4DhigCUBNhig cells may be the key reason for the occurrence and progression of DN.
Table 1
Gene | Statistic | Mc0 (n=47) | Mc1 (n=41) | Mc2 (n=30) | Mc3 (n=26) |
---|---|---|---|---|---|
Group (n=144) | CON | 24 | 0 | 0 | 11 |
DN | 23 | 41 | 30 | 15 | |
CD163 | Mean | 1.425 | 1.485 | 0.550 | 1.126 |
SD | 0.860 | 0.727 | 0.706 | 0.770 | |
Wilcoxon | *** | *** | |||
t-test | ††† | ||||
MRC1 | Mean | 1.025 | 1.641 | 1.475 | 0.280 |
SD | 1.100 | 0.966 | 0.832 | 0.613 | |
Wilcoxon | *** | *** | |||
t-test | ‡‡†§§§ |
***, P<0.001 vs. others; ‡‡, P<0.001 vs. Mc1; † or †††, P<0.05 or P<0.001 vs. Mc2; §§§, P<0.001 vs. Mc3. Mφ, macrophage; CON, control; DN, diabetic nephropathy; SD, standard deviation.
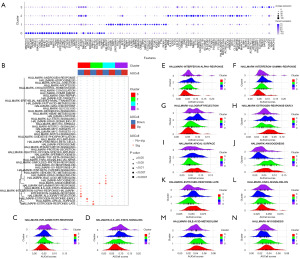
Functional analysis of different Mφ phenotypes in DN
In order to clarify the function of CD163higMRC1midPTH2RhigPDE4DhigCUBNhig cells in DN, we obtained markers and analyzed their enrichment pathways (Figure 6A,6B). The results showed that the AUCell scores for characteristic genes of CD163higMRC1midPTH2RhigPDE4DhigCUBNhig cells were increased in the inflammatory response (P<0.001; Figure 6C), IL6-JAK-STAT3 signaling (P<0.05; Figure 6B,6D), interferon-alpha response (P<0.05; Figure 6E), and interferon-gamma response (P<0.05; Figure 6F) pathways were all significantly reduced in the allograft rejection pathway (P<0.001; Figure 6G). These are typical signaling pathways of inflammatory activation (36,37), which indicates that CD163higMRC1midPTH2RhigPDE4DhigCUBNhig cells may inhibit inflammatory reactions. Moreover, we found that the AUCell score of CD163higMRC1midPTH2RhigPDE4DhigCUBNhig cells was significantly increased in early estrogen response (P<0.001; Figure 6H) and apical (P<0.05; Figure 6I) pathways. Activation of these pathways leads to increased estrogen secretion in the kidney, which maintains mitochondrial homeostasis and regulates endothelin-1 (ET-1) function through the estrogen receptor pathway, thereby inhibiting renal cell apoptosis and contributing to kidney repair and regeneration (38,39). This indicates that CD163higMRC1midPTH2RhigPDE4DhigCUBNhig cells may exert a protective effect on the kidneys, the loss of which may lead to a decrease in the self-repairing ability of the kidneys, thus exacerbating the severity of DN.
Additionally, we found that the AUcell scores of CD163higMRC1higCD163L1higF13A1higRGL1hig cells were significantly increased in the angiogenesis pathway (P<0.01; Figure 6J) but significantly decreased in the apical surface (P<0.01; Figure 6I) and late estrogen response (P<0.001; Figure 6K) pathways. The abnormal angiogenesis in DN is due to the increase of the secretion of VEGF-A, which leads to decreased glomerular endothelial connectivity, increased permeability of the glomerular ultrafiltration barrier, and the occurrence of proteinuria (40,41). We could surmise that CD163higMRC1higCD163L1higF13A1higRGL1hig cells lead to impaired angiogenesis and impaired glomerular function. In addition, CD163lowMRC1higIL1R2higJAMLhigCCDC26hig cells may also inhibit the estrogen response pathway and weaken its function in protecting the kidney, further exacerbating renal injury. CD163lowMRC1higIL1R2higJAMLhigCCDC26hig cells were significantly enriched in allograft rejection pathway (P<0.01; Figure 6G), which can enhance renal inflammation and oxidative stress damage by activating NF-κB and ATG4B pathways through allogenic inflammatory factors (AIFs) (42,43). It can also weaken KRAS signaling in DN (P<0.05; Figure 6L) and bile acid (BA) metabolism (P<0.05; Figure 6M), reduce renal Ras levels, and inhibit BA metabolism, which is helpful for blood glucose CON in patients with DN (44-47). CD163lowMRC1higIL1R2higJAMLhigCCDC26hig cells may also inhibit myogenesis (P<0.01; Figure 6N) and angiogenesis (P<0.001; Figure 6J) signaling to reduce renal cell damage. It can be concluded that CD163lowMRC1higIL1R2higJAMLhigCCDC26hig cells play a dual regulatory role, controlling blood glucose and promoting renal repair while promoting inflammation.
In view of the above, we can conclude that Mc0–3 contribute to inhibiting inflammation, promoting angiogenesis, activating inflammation and glucose metabolism, and promoting renal repair and neuronal remodeling in the kidney. This further illustrates the importance of Mc0 (CD163higMRC1midPTH2RhigPDE4DhigCUBNhig cells) in resisting the DN process.
Differentiation of Mφs in DN
As described above, we conducted phenotypic identification and functional analysis of KRMs. In order to further clarify the correlation between the differentiation status of different phenotypes of KRMs, we conducted a pseudochronological analysis (Figures 7,8). The results showed that KRMs had two differentiation time points and five differentiation states (Figure 7A). As shown in Figure 7B-7D, the branch started from Mc2 (Mc2-state1), with Mc1 (Mc1-state3) and Mc3 (Mc3-state1) being intermediate states of KRMs. There were also some cells in Mc1 that were the terminal cells of differentiation node 1 (Mc1-state2). Mc0 was mainly the terminal cell of differentiation node 2 (Mc0-state5). Differentiation state5 included cells that failed to differentiate, including some Mc0 (Mc0-state5) and Mc1 (Mc1-state5) cells.
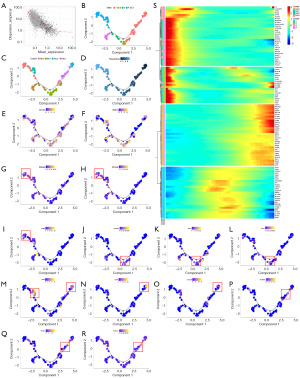
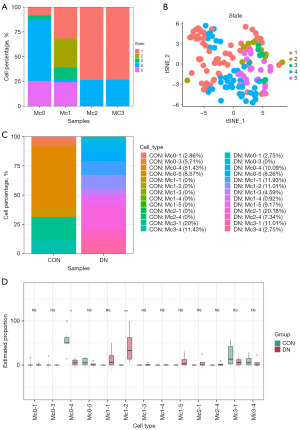
The expression trend of pseud-chronological genes in Mφs is shown in Figure 7G and was basically consistent with the phenotype-related markers of KRMs. By analyzing the expression marker genes at different stages, we found that the expression of CD163 decreased before differentiation and began to increase after differentiation. The expression of MRC1 fluctuated, with a peak at stage 2, and then began to weaken (Figure 7E,7F). The markers of Mc0 (PDE4D, PTH2R, and CUBN) were mainly overexpressed in state4 (Figure 7G-7I), those of Mc1 (CD163L1, RGL1, and F13A1) were mainly overexpressed in state2 (Figure 7J-7L), and those of Mc2 and Mc3 markers were mainly overexpressed in state1 (Figure 7M-7R). Therefore, it can be speculated that CD163higMRC1midPTH2RhigPDE4DhigCUBNhig, CD163higMRC1higCD163L1higF13A1higRGL1hig, CD163lowMRC1higIL1R2higJAMLhigCCDC26hig, and CD163higMRC1lowTANC2higPLXDC2higADGRB3hig are mainly associated with Mc0-state4, Mc1-state2, Mc2-state1, and Mc3-state1 cells, respectively. Furthermore, by analyzing the difference in the proportion of the four types of KRMs mentioned above, we found that compared with the CON group, the DN group had a significantly decreased abundance of Mc0-state4 (CD163higMRC1midPTH2RhigPDE4DhigCUBNhig) cells and a significantly increased abundance of Mc1-state2 (CD163higMRC1higCD163L1higF13A1higRGL1hig) cells (Figure 8A-8D), which were found to be key factors in the occurrence and development of DN. The decrease in the proportion of KRMs in DN mainly manifested as the loss of CD163higMRC1midPTH2RhigPDE4DhigCUBNhig cells. This Mφ phenotype may warrant further investigation in future research.
By analyzing the function of different types of KRMs, we found that the AUCell score of Mc0-state4 cells in inflammatory response pathways (including allograft rejection, inflammatory response, IL6-JAK-STAT3-signaling, interferon-gamma response, PI3K-AKT-MTO signaling, and interferon alpha response) was significantly decreased (P<0.01), consistent with results of CD163higMRC1midPTH2RhigPDE4DhigCUBNhig cells (Figure 9A). Compared to other types of cells, this subtype of cell mainly exhibited significant differential expression of genes related to biological pathways such as cell adhesion and renal system development (adjusted P<0.05; Figure 9B). In contrast to CD163higMRC1higCD163L1higF13A1higRGL1hig cells, Mc1-state2 cells did not have significantly enriched hallmark pathways, but the significantly differential expressed markers were mainly enriched in the BPs of ENDO chemotaxis and response to hepatocyte growth factor and metal ions (adjusted P<0.05; Figure 8C,9C). Mc2-state1 (CD163lowMRC1higIL1R2higJAMLhigCCDC26hig) cells and Mc3-state1 (CD163higMRC1lowTANC2higPLXDC2higADGRB3hig) cells mainly exhibited significant activation of inflammatory reactions (allograft rejection, inflammatory response, TNF-α signaling via NF-κB, etc., P<0.05). Markers of CD163lowMRC1higIL1R2higJAMLhigCCDC26hig cells were mainly enriched in BPs such as T-cell differentiation and immune receptor activation (Figure 9D), while those of CD163higMRC1lowTANC2higPLXDC2higADGRB3hig cells were enriched in the BPs of the maturation process of neuronal cells (Figure 9E).
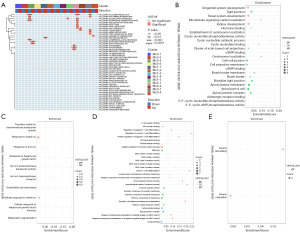
Function of CD163higMRC1midPTH2RhigPDE4DhigCUBNhig cells in DN
As we found that the loss of CD163higMRC1midPTH2RhigPDE4DhigCUBNhig cells may lead to a decrease in the ability to inhibit inflammatory reactions, which in turn may promote renal inflammatory damage, we further analyzed the mechanism of CD163higMRC1midPTH2RhigPDE4DhigCUBNhig cell loss in DN. First, in the comparison of the CON and DN groups in terms of the DEGs in CD163higMRC1midPTH2RhigPDE4DhigCUBNhig cells, 308 DEGs were found to be upregulated and 143 DEGs were downregulated in the DN group compared to CON group (Figure 10). Downregulated DEGs were enriched in KEGG pathways related tight junction; PT bicarbonate reclamation; aldosterone-regulated sodium reabsorption; and PI3K-AKT, cAMP, and MAPK signaling (FDR <0.05; Figure 10B). Upregulated DEGs were enriched immune-related signals and pathways such as T17-cell differentiation, antigen processing and presentation, megakaryocyte development, and B cell homeostasis, among others (FDR <0.05; Figure 10C). The decrease in CD163higMRC1midPTH2RhigPDE4DhigCUBNhig cells may regulate cell-matrix proteins or tight junction proteins in DN, thus contributing to an inflammatory immune activation, which is closely related to renal fibrosis.
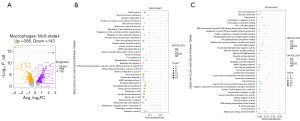
Further analysis of the interaction of DEGs revealed that the significantly upregulated HIF1A, CYBB, PRKCB, ITGAX, and AHR and significantly downregulated EGF, RHOA, PTK2, CD163, IGF1R, and PDGFB in CD163higMRC1midPTH2RhigPDE4DhigCUBNhig cells were the core of the PPI network (Figure 11A-11D). These DEGs were found to be involved in immune-cell differentiation, intracellular receptor signaling pathways, and Th17-cell differentiation (FDR <0.05), as well as the regulation of MAPK signals and PI3K-AKT signals (FDR <0.05) and the focal adhesion pathway (Figure 11E,11F). In particular, AHR and IGF1R exhibited a significantly high and low expression in DN tissues, respectively (P<0.01; Figure 12), and these are also genes related to KRM differentiation. AHR and IGF1R may regulate DN damage by inducing CD163higMRC1midPTH2RhigPDE4DhigCUBNhig cell transformation.
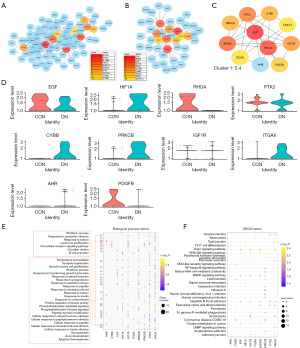
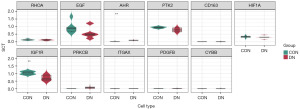
Tight communication between renal Mφs and PODOs in DN
To determine whether the imbalance of AHR and IGF1R expression leads to a decrease in DN and dysfunction of KRMs, we further analyzed their effects on renal cells. We used celltalker (version 3.0; https://github.com/ventolab/cellphonedb) to analyze the ligand and receptor interactions between KRMs and renal cells, and we used cellphoneDB (version 3.0; https://github.com/ventolab/cellphonedb) analysis to supplement the results. We discovered that KRMs in DN mainly interacted with PODOs [interaction rate (IR) =37.399] and ENDOs (IR =29.836) (P<0.05; Figure 13A and Table S3). In particular, PODOs were found to be key cell that affects the process of DN. Therefore, we mainly focused on the signal communication between KRMs and PODOs.
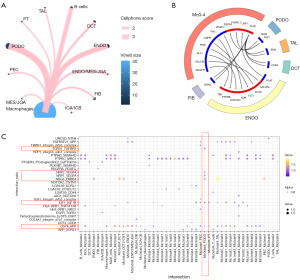
In the celltalker results, CD163higMRC1midPTH2RhigPDE4DhigCUBNhig cells mainly recognized BGN and FGF1 secreted by PODOs through FGFRL1 and FGFRL4. In addition, VEGFC, TNC, and PIGF interacted with FLT1, KDR, and PTPRB expressed by ENDOs (Figure 13B). The cellphoneDB results also showed that CD163higMRC1midPTH2RhigPDE4DhigCUBNhig recognized VEGFA secreted by PODOs through the NRP1-NRP2, NRG3-ERBB4, CD74-APP, and TNFRSF21-APP pairs (Figure 13C).
Analysis of the differential expression of receptors and ligands revealed a significant increase in the secretion of NRG3, COL4A1, and THBS1 by CD163higMRC1midPTH2RhigPDE4DhigCUBNhig cells in DN (P<0.05; Figure 14A,14B). Three ligands, ITGB1, LRP1, and SCARB1, were found to act on ENDO surfaces. However, there was no statistical difference in the expression of these three receptors between the CON and DN groups (P>0.05; Figure 14C). The expression of ERBB4 (P<0.01) and ITGB1 (P<0.001) in PODOs induced by NRG3 and COL4A1 were significantly increased in the DN group. The expression of ITGB3 (P<0.001) and ITGA3 (P<0.001) were also significantly increased, as mediated by THBS1 (Figure 14D). CD163higMRC1midPTH2RhigPDE4DhigCUBNhig cells were confirmed to mainly affect PODOs through NRG3-ERBB4, COL4A1-ITGB1, and THBS1-ITGB3/ITGA3 axis.
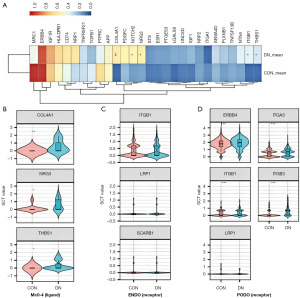
The injury mechanism of PODOs in DN
We discovered that in PODOs, CD163higMRC1midPTH2RhigPDE4DhigCUBNhig cells activate ERBB4 and surface integrins (ITGs), which are important membrane proteins on the cell surface and can cause intracellular signal and functional changes after activation. Therefore, we analyzed the impact of PODOs on the DN process after being exposed to CD163higMRC1midPTH2RhigPDE4DhigCUBNhig cells.
In this step, 227 upregulated DEGs and 101 downregulated DEGs of PODOs in DN were obtained (Figure 15A). The upregulated DEGs were significantly enriched in BPs such as second-messenger-mediated signaling, receptor-mediated endocytosis, calcium-ion transmembrane transport, regulation of protein kinase B signaling, and response to hydroperoxide, among others (adjusted P<0.05; Figure 15B). Downregulated DEGs were significantly enriched in the positive regulation of cell adhesion, urogenital system development, cell-substrate adhesion, kidney development, renal system development, cellular response to TNF-β stimulus, etc. (adjusted P<0.01; Figure 15C). It can be seen that PODO undergoes oxidative stress activation, abnormal ECM composition, and inhibition of cell development/repair.
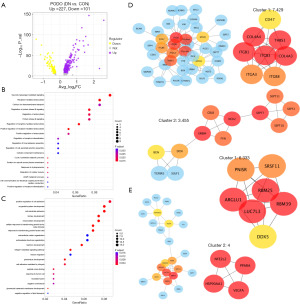
Additionally, we found that ERBB4, ITGB1, ITGB3, and ITGA3, which were significantly differentially expressed in PODO, constituted the center of the PPI network for BPs such as the progression of renal system, cellular oxidative stress reactions, and cell adhesion, among others. ERBB4, ITGB1, ITGB3, and ITGA3 were found to regulate the expression of cytoskeletal genes (NCK2) and type IV collagen genes (COL4A4, COL4A3) and to cause a series of biochemical reactions (Figure 15D,15E and Figure S5). Activation of oxidative stress in PODOs promotes cell damage and apoptosis, while secretion of type IV collagen leads to ECM deposition, which is also a typical mechanism of glomerulosclerosis and fibrosis in DN (48-50).
Discussion
In this study, we used FLT4, PDPN, PTPRC, CD3E, MS4A1, FCGR3A, CD14, and CSF1R to define IMCs and found that the abundance of IMCs in DN tissues were increased, which is consistent with previous research (28). The recruitment of IMCs in DN is related to the maintenance of inflammation (29,30). Persistent inflammatory infiltration leads to the loss of renal PODOs, renal vascular damage, excessive deposition of ECM, and even renal fibrosis. Evidence from clinical and experimental study indicates that immune activation signals such as Toll-like receptor, NOD signal, NF-κB signal pathway, and NLRP3 inflammatory body signal are activated in DN, which contributes to renal inflammation and fibrosis. Therefore, it has been proposed that anti-inflammatory therapy may have a renal protective effect against DKD (51).
In further investigating the function of IMCs in DN, we found that there was an elevated abundance of B cells, T cells, mast cells, and Mφs in kidney tissue. However, compared with normal tissue, no abnormality in the number of other types of IMCs was found except for that of Mφs. Differences in IMs in DN may be due to changes in function rather than quantity. In addition, unlike previous studies (26-30), our analysis showed a significant decrease in the number of Mφs in DN. Most related studies have reported an increase in infiltrating Mφs in the renal tissue of animals and human patients with DN, with these Mφs mainly exerting proinflammatory characteristics (M1 phenotype). M1 Mφs are involved in the initial stage of inflammation and are associated with renal tissue damage and inflammatory infiltration (52-54). The Mφs in our study were instead associated with inflammation inhibition.
We believe that the reason for the discrepancy between our and other studies’ findings may be related to the heterogeneity of Mφs. To clarify the Mφ phenotype, the expression of Mφ markers was analyzed. It was found that the main type of Mφ in the kidney was CD163+MRC1+, which mainly manifest an anti-inflammatory phenotype (M2). M2 Mφs can produce anti-inflammatory cytokines, growth factors, and angiogenic cytokines, which are involved in the wound healing process (repair stage) (55-59). In order to further clarify the function of Mφs, CD163+MRC1+ Mφs were clustered, and pseudotiming analysis was performed. We found that CD163+MRC1+ Mφs could be further divided into four clusters, thus indicating the presence of different stages in the differentiation of CD163+MRC1+ Mφs. The results indicated that there were three differentiation routes for renal Mφs. Route 1 proceeds as follows: Mc2-state1 > Mc3-state1 > Mc1-state2. The Mc1-state2 cells (CD163higMRC1higCD163L1higF13A1higRGL1hig) that ultimately differentiate by this route are related to endothelial chemotaxis, and ion reaction signals, among other functions. Route 2 proceeds as follows: Mc2-state1 > Mc3-state1 > Mc2-state3 > state5. The function of the finally differentiated stat5 cell is unclear. Route 3 (Mc2-state1 > Mc3-state1 > Mc0-state4) is a normal differentiation pathway, resulting in CD163higMRC1midPTH2RhigPDE4DhigCUBNhig cells that perform functions such as inhibition of inflammatory signals and regulation of biological responses to cell adhesion. Meanwhile, pre-differentiated cells (CD163lowMRC1higIL1R2higJAMLhigCCDC26hig) participate in promoting inflammatory response, and their proportion is higher in DN tissue. It can be surmised that the imbalance in the ratio of CD163higMRC1midPTH2RhigPDE4DhigCUBNhig to CD163lowMRC1higIL1R2higJAMLhigCCDC26hig cells can enhance the inflammatory response in kidney tissue, leading to kidney damage.
In addition, we found that the Mφs present in DN are mainly kidney resident cells rather than blood-derived monocytes that are recruited and differentiated from the kidney. It is suggested that Mφs present in the kidney are derived from bone marrow cells. These Mφs (CD14hig) infiltrate the kidneys and secrete inflammatory factors that exacerbate tissue damage. Indeed, the function of KRMs (CD14−) cannot be ignored. KRMs can be stimulated by various factors to transform into an anti-inflammatory phenotype, thereby inhibiting the damage of inflammatory factors to cells. However, the loss of KRMs leads to further accumulation of inflammatory factors, exacerbating renal damage.
Abnormal AHR and IGF1R signals may cause the loss of CD163higMRC1midPTH2RhigPDE4DhigCUBNhig cells in DN. In the DEG analysis of CD163higMRC1midPTH2RhigPDE4DhigCUBNhig cells, we found that low AHR expression was associated with CD163higMRC1midPTH2RhigPDE4DhigCUBNhig cell maturation. AHR is a transcription regulator that regulates downstream JUN, MAPK, and ERK/AKT signals to regulate cell proliferation, differentiation, and apoptosis, and it is also a regulator of IMC activation (60-62). A study has also found that AHR knockout promotes the transformation of anti-inflammatory Mφ phenotype. However, both the overall tissue and Mc0–4 cells in DN exhibit a high expression of AHR (63). In addition, we also found that IGF1R is a mature catalyst for cells with CD163higMRC1midPTH2RhigPDE4DhigCUBNhig, but its reduced expression in DN has been reported (64). This indicates that the overexpression of AHR and impairment of IGF1R function in DN leads to a reduction in CD163higMRC1midPTH2RhigPDE4DhigCUBNhig cell transformation.
CD163higMRC1midPTH2RhigPDE4DhigCUBNhig cells affect PODO injury and apoptosis. We thus analyzed the changes in renal tissue cells and found that the main cells in the kidney were the PCs (33.61%), followed by TALs (17.45%). However, CD163higMRC1midPTH2RhigPDE4DhigCUBNhig cells had stronger signal communication with PODOs (2.7%) and ENDOs (2.39%), although the proportion of these two types of cells was relatively small. The expression of NRG3, COL4A1, and THBS1 in CD163higMRC1midPTH2RhigPDE4DhigCUBNhig cells increased. COL4A1 and THBS1 form complexes with cell surface fibrinogen, collagen, and ITGs, which cause intracellular signal transduction, participate in angiogenesis, and are associated with kidney damage and functional defects. NRG3 is a neuroregulatory protein that binds to ERBB3 and ERBB4 and regulates the stimulation response of cells to growth factors, as well as cell proliferation and differentiation. Moreover, we found that ERBB4, ITGB1, ITGB3, and ITGA3, which interact with the three ligands mentioned above in PODOs, also had increased expression in DN. It has been reported that the upregulation of ERBB4 promotes PODO apoptosis (65). The expression of ITGB1, ITGB3, and ITGA3 increases the formation of ITG complexes on the surface of PODOs. The activation of ITG receptor is also a classic signal pathway of PODO apoptosis and diabetic kidney damage (66,67).
As shown in Figure 16, the high expression of AHR and the low expression of IGF1R in KRMs in DN environments lead to the failure of the CD163higMRC1midPTH2RhigPDE4DhigCUBNhig-type Mφ transformation. Moreover, CD163higMRC1midPTH2RhigPDE4DhigCUBNhig cells in DN express higher levels of NRG3, COL4A1, and THBS1, thus activating ITG and epidermal growth factor signaling pathways on PODOs. This leads to the increased expression of ECM components (including type IV collagen) and collagen deposition, ultimately resulting glomerulosclerosis and matrix fibrosis. Regulating Mφ transformation by intervening in AHR or IGF1R may be a key strategy for the treatment of DN.
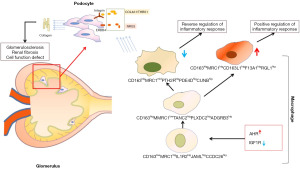
Conclusions
Through an snRNA-seq analysis of DN, we discovered that the reduction of CD163+MRC1+ KRM abundance in diabetic kidney tissue promotes renal inflammatory injury and fibrosis.
Acknowledgments
Funding: None.
Footnote
Reporting Checklist: The authors have completed the MDAR reporting checklist. Available at https://tau.amegroups.com/article/view/10.21037/tau-24-569/rc
Peer Review File: Available at https://tau.amegroups.com/article/view/10.21037/tau-24-569/prf
Conflicts of Interest: All authors have completed the ICMJE uniform disclosure form (available at https://tau.amegroups.com/article/view/10.21037/tau-24-569/coif). J.L. is an employee from Guangzhou Algae Technology. The other authors have no conflicts of interest to declare.
Ethical Statement: The authors are accountable for all aspects of the work in ensuring that questions related to the accuracy or integrity of any part of the work are appropriately investigated and resolved. The study was conducted in accordance with the Declaration of Helsinki (as revised in 2013).
Open Access Statement: This is an Open Access article distributed in accordance with the Creative Commons Attribution-NonCommercial-NoDerivs 4.0 International License (CC BY-NC-ND 4.0), which permits the non-commercial replication and distribution of the article with the strict proviso that no changes or edits are made and the original work is properly cited (including links to both the formal publication through the relevant DOI and the license). See: https://creativecommons.org/licenses/by-nc-nd/4.0/.
References
- Gupta S, Dominguez M, Golestaneh L. Diabetic Kidney Disease: An Update. Med Clin North Am 2023;107:689-705. [Crossref] [PubMed]
- Talukdar A, Basumatary M. Rodent models to study type 1 and type 2 diabetes induced human diabetic nephropathy. Mol Biol Rep 2023;50:7759-82. [Crossref] [PubMed]
- Cai SS, Zheng TY, Wang KY, et al. Clinical study of different prediction models in predicting diabetic nephropathy in patients with type 2 diabetes mellitus. World J Diabetes 2024;15:43-52. [Crossref] [PubMed]
- Navaneethan SD, Zoungas S, Caramori ML, et al. Diabetes Management in Chronic Kidney Disease: Synopsis of the KDIGO 2022 Clinical Practice Guideline Update. Ann Intern Med 2023;176:381-7. [Crossref] [PubMed]
- Giglio RV, Patti AM, Rizvi AA, et al. Advances in the Pharmacological Management of Diabetic Nephropathy: A 2022 International Update. Biomedicines 2023;11:291. [Crossref] [PubMed]
- Azhari F, Effendi I, Ali Z, et al. The Role of Podocyte Cells in Diabetic Nephropathy: A Narrative Literature Review. Biosci Med 2023;7:3808-17.
- Mohandes S, Doke T, Hu H, et al. Molecular pathways that drive diabetic kidney disease. J Clin Invest 2023;133:e165654. [Crossref] [PubMed]
- Rayego-Mateos S, Rodrigues-Diez RR, Fernandez-Fernandez B, et al. Targeting inflammation to treat diabetic kidney disease: the road to 2030. Kidney Int 2023;103:282-96. [Crossref] [PubMed]
- Yang X, Chen Z, Luo Z, et al. STING deletion alleviates podocyte injury through suppressing inflammation by targeting NLRP3 in diabetic kidney disease. Cell Signal 2023;109:110777. [Crossref] [PubMed]
- Henedak NT, El-Abhar HS, Soubh AA, et al. NLRP3 Inflammasome: A central player in renal pathologies and nephropathy. Life Sci 2024;351:122813. [Crossref] [PubMed]
- Li X, Zhang Y, Xing X, et al. Podocyte injury of diabetic nephropathy: Novel mechanism discovery and therapeutic prospects. Biomed Pharmacother 2023;168:115670. [Crossref] [PubMed]
- Issa W, Njeim R, Carrazco A, et al. Role of the Innate Immune Response in Glomerular Disease Pathogenesis: Focus on Podocytes. Cells 2024;13:1157. [Crossref] [PubMed]
- Chen X, Zhang Y, Liu Y, et al. Mechanisms underlying the progression of diabetic nephropathy: Hyperglycemia-induced oxidative stress and its impact on podocyte function. Springer Reference 2024;46:134-50.
- Wu H, Fang L, Hu Y, et al. The role of AGEs-RAGE signaling in diabetic nephropathy: A target for therapeutic intervention. Front Endocrinol 2024;15:7301-14.
- Li W, Zhou S, Zhang J, et al. Advances in targeting macrophage polarization to mitigate renal inflammation in diabetic nephropathy. Front Pharmacol 2024;14:4369-81.
- Zhao Q, Li X, Wang F, et al. Fibrosis-related pathways in diabetic nephropathy: Therapeutic potential of targeting TGF-β/Smad signaling. Front Endocrinol 2024;15:7301-20.
- Kriz W, Löwen J, Gröne HJ. The complex pathology of diabetic nephropathy in humans. Nephrol Dial Transplant 2023;38:2109-19. [Crossref] [PubMed]
- Youssef N, Noureldein MH, Riachi ME, et al. Macrophage polarization and signaling in diabetic kidney disease: a catalyst for disease progression. Am J Physiol Renal Physiol 2024;326:F301-12. [Crossref] [PubMed]
- Li G, Yang H, Zhang D, et al. The role of macrophages in fibrosis of chronic kidney disease. Biomed Pharmacother 2024;177:117079. [Crossref] [PubMed]
- Lazarov T, Juarez-Carreño S, Cox N, et al. Physiology and diseases of tissue-resident macrophages. Nature 2023;618:698-707. [Crossref] [PubMed]
- Yan J, Li X, Liu N, et al. Relationship between Macrophages and Tissue Microenvironments in Diabetic Kidneys. Biomedicines 2023;11:1889. [Crossref] [PubMed]
- Lin DW, Yang TM, Ho C, et al. Targeting Macrophages: Therapeutic Approaches in Diabetic Kidney Disease. Int J Mol Sci 2024;25:4350. [Crossref] [PubMed]
- Chen M, Menon MC, Wang W, et al. HCK induces macrophage activation to promote renal inflammation and fibrosis via suppression of autophagy. Nat Commun 2023;14:4297. [Crossref] [PubMed]
- Zhang X, Chao P, Zhang L, et al. Single-cell RNA and transcriptome sequencing profiles identify immune-associated key genes in the development of diabetic kidney disease. Front Immunol 2023;14:1030198. [Crossref] [PubMed]
- Zhang C, Li H, Wang S. Single-cell and transcriptome analysis reveals TAL cells in diabetic nephropathy. Funct Integr Genomics 2023;23:292. [Crossref] [PubMed]
- Song Y, Liu Y, Guo F, et al. Single-Cell RNA Sequencing Reveals RAC1 Involvement in Macrophages Efferocytosis in Diabetic Kidney Disease. Inflammation 2024;47:753-70. [Crossref] [PubMed]
- Quatredeniers M, Serafin AS, Benmerah A, et al. Meta-analysis of single-cell and single-nucleus transcriptomics reveals kidney cell type consensus signatures. Sci Data 2023;10:361. [Crossref] [PubMed]
- Xie R, Zhu L, Jason SKH, et al. # 3802 Role of neutrophil extracellular traps in diabetic kidney disease: data from integration of bulk RNA and single-nucleus RNA sequencing analyses. Nephrol Dial Transplant 2023;38:gfad063c_3802.
- Liu J, Wang H, Liu Q, et al. Klotho exerts protection in chronic kidney disease associated with regulating inflammatory response and lipid metabolism. Cell Biosci 2024;14:46. [Crossref] [PubMed]
- Kim N, Kang H, Jo A, et al. Perspectives on single-nucleus RNA sequencing in different cell types and tissues. J Pathol Transl Med 2023;57:52-9. [Crossref] [PubMed]
- Zhang Y, Xie Y, Lu W, et al. Identification of resident progenitors labeled with Top2a responsible for proximal tubular regeneration in ischemia reperfusion-induced acute kidney injury. Cell Signal 2023;101:110506. [Crossref] [PubMed]
- 10X-Genomics. Data from “3k PBMCs from a Healthy Donor”. 10X Genomics. 2016. Accessed 14 April 2019. Available online: https://support.10xgenomics.com/single-cell-gene-expression/datasets/1.1.0/pbmc3k
- 10X-Genomics. Data from “4k PBMCs from a Healthy Donor”. 10X Genomics. 2017. Accessed 14 April 2019. Available online: https://support.10xgenomics.com/single-cell-gene-expression/datasets/2.1.0/pbmc4k
- Wei Q, Deng Y, Yang Q, et al. The markers to delineate different phenotypes of macrophages related to metabolic disorders. Front Immunol 2023;14:1084636. [Crossref] [PubMed]
- Chew C, Brand OJ, Yamamura T, et al. Kidney resident macrophages have distinct subsets and multifunctional roles. Matrix Biol 2024;127:23-37. [Crossref] [PubMed]
- Sabry IM, Mahdy MMM, Elhalawany SHA, et al. Study of Neutrophil-Lymphocyte Ratio, Interleukin-6 (IL-6) and Tumor Necrosis Factor-Alpha (TNF-α) in Type 2 Diabetes with Diabetic Nephropathy. QJM 2023;116:hcad069. 450.
- Liu Y, Wang W, Zhang J, et al. JAK/STAT signaling in diabetic kidney disease. Front Cell Dev Biol 2023;11:1233259. [Crossref] [PubMed]
- Wang XX, Myakala K, Libby AE, et al. Estrogen-Related Receptor Agonism Reverses Mitochondrial Dysfunction and Inflammation in the Aging Kidney. Am J Pathol 2023;193:1969-87. [Crossref] [PubMed]
- Thomas W, Harvey BJ. Estrogen-induced signalling and the renal contribution to salt and water homeostasis. Steroids 2023;199:109299. [Crossref] [PubMed]
- Gacche RN. Changing landscape of anti-angiogenic therapy: Novel approaches and clinical perspectives. Biochim Biophys Acta Rev Cancer 2023;1878:189020. [Crossref] [PubMed]
- Ning Y, Zhou X, Wang G, et al. Exosome miR-30a-5p Regulates Glomerular Endothelial Cells' EndMT and Angiogenesis by Modulating Notch1/VEGF Signaling Pathway. Curr Gene Ther 2024;24:159-77. [Crossref] [PubMed]
- De Leon-Oliva D, Garcia-Montero C, Fraile-Martinez O, et al. AIF1: Function and Connection with Inflammatory Diseases. Biology (Basel) 2023;12:694. [Crossref] [PubMed]
- Fu Y, Wang X, Zhang L, et al. Allograft inflammatory factor-1 enhances inflammation and oxidative stress via the NF-κB pathway in diabetic kidney disease. Biochem Biophys Res Commun 2022;614:63-9. [Crossref] [PubMed]
- Li B, Zhao X, Xie W, et al. Integrative analyses of biomarkers and pathways for diabetic nephropathy. Front Genet 2023;14:1128136. [Crossref] [PubMed]
- Singhal A, Li BT, O'Reilly EM. Targeting KRAS in cancer. Nat Med 2024;30:969-83. [Crossref] [PubMed]
- Hou Y, Zhai X, Wang X, et al. Research progress on the relationship between bile acid metabolism and type 2 diabetes mellitus. Diabetol Metab Syndr 2023;15:235. [Crossref] [PubMed]
- Kim DH, Park JS, Choi HI, et al. The role of the farnesoid X receptor in kidney health and disease: a potential therapeutic target in kidney diseases. Exp Mol Med 2023;55:304-12. [Crossref] [PubMed]
- Hejazian SM, Ardalan M, Hosseiniyan Khatibi SM, et al. Biofactors regulating mitochondrial function and dynamics in podocytes and podocytopathies. J Cell Physiol 2023;238:2206-27. [Crossref] [PubMed]
- Loderbauer L, Schneider K, Knaup K, et al. # 3271 Expression of the collagen IV α345 molecule along the renal tubule in health and disease. Nephrol Dial Transplant 2023;38:gfad063a_3271.
- Qiu J, Chi H, Gan C, et al. A high-impact FN1 variant correlates with fibronectin-mediated glomerulopathy via decreased binding to collagen type IV. Pathology 2023;55:498-507. [Crossref] [PubMed]
- Oda Y, Nishi H, Nangaku M. Role of Inflammation in Progression of Chronic Kidney Disease in Type 2 Diabetes Mellitus: Clinical Implications. Semin Nephrol 2023;43:151431. [Crossref] [PubMed]
- Peng QY, An Y, Jiang ZZ, et al. The Role of Immune Cells in DKD: Mechanisms and Targeted Therapies. J Inflamm Res 2024;17:2103-18. [Crossref] [PubMed]
- Han X, Wei J, Zheng R, et al. Macrophage SHP2 Deficiency Alleviates Diabetic Nephropathy via Suppression of MAPK/NF-κB- Dependent Inflammation. Diabetes 2024;73:780-96. [Crossref] [PubMed]
- Fan JH, Xu HX, Qin ZZ, et al. Folic Acid Protects Against Kidney Damage in Mice with Diabetic Nephropathy by Inhibiting M1 Macrophage Polarization via Nuclear Factor-k-gene Binding Pathway. Altern Ther Health Med 2023;29:418-20.
- Yang M, Zhang C. The role of innate immunity in diabetic nephropathy and their therapeutic consequences. J Pharm Anal 2024;14:39-51. [Crossref] [PubMed]
- Li X, Zhang L, Yan C, et al. Relationship between immune cells and diabetic nephropathy: a Mendelian randomization study. Acta Diabetol 2024;61:1251-8. [Crossref] [PubMed]
- Shao X, Shi Y, Wang Y, et al. Single-Cell Sequencing Reveals the Expression of Immune-Related Genes in Macrophages of Diabetic Kidney Disease. Inflammation 2024;47:227-43. [Crossref] [PubMed]
- Wang X, Li R, Liu T, et al. CD163 in Macrophages: A Potential Biomarker for Predicting the Progression of Diabetic Nephropathy based on Bioinformatics Analysis. Endocr Metab Immune Disord Drug Targets 2023;23:294-303. [Crossref] [PubMed]
- Cao Y, Liang N, Kong K, et al. CD163 as a Potential Biomarker-associated Immune Inflammation in Diabetes Mellitus: A Systematic Review and Bioinformatics Analysis. Endocr Metab Immune Disord Drug Targets 2024;24:208-19. [Crossref] [PubMed]
- Huang W, Rui K, Wang X, et al. The aryl hydrocarbon receptor in immune regulation and autoimmune pathogenesis. J Autoimmun 2023;138:103049. [Crossref] [PubMed]
- Malany K, Li X, Vogel CFA, et al. Mechanisms underlying aryl hydrocarbon receptor-driven divergent macrophage function. Toxicol Sci 2024;200:1-10. [Crossref] [PubMed]
- Opitz CA, Holfelder P, Prentzell MT, et al. The complex biology of aryl hydrocarbon receptor activation in cancer and beyond. Biochem Pharmacol 2023;216:115798. [Crossref] [PubMed]
- Xie H, Yang N, Yu C, et al. Uremic toxins mediate kidney diseases: the role of aryl hydrocarbon receptor. Cell Mol Biol Lett 2024;29:38. [Crossref] [PubMed]
- Wang J, Han Y, Huang F, et al. Diabetic macrophage small extracellular vesicles-associated miR-503/IGF1R axis regulates endothelial cell function and affects wound healing. Front Immunol 2023;14:1104890. [Crossref] [PubMed]
- Abdelmaksoud NM, Al-Noshokaty TM, Abdelhamid R, et al. Deciphering the role of MicroRNAs in diabetic nephropathy: Regulatory mechanisms and molecular insights. Pathol Res Pract 2024;256:155237. [Crossref] [PubMed]
- Wang X, Zhang Y, Chi K, et al. IGFBP2 induces podocyte apoptosis promoted by mitochondrial damage via integrin α5/FAK in diabetic kidney disease. Apoptosis 2024;29:1109-25. [Crossref] [PubMed]
- Bukanov NO, Natoli TA, Chipashvili V, et al. Integrin α3β1 as a Potential Target for Diabetic Kidney Disease (DKD): SA-PO1006. J Am Soc Nephrol 2023;34:1011.
(English Language Editor: J. Gray)