An 11-gene glycosyltransferases-related model for the prognosis of patients with bladder urothelial carcinoma: development and validation based on TCGA and GEO datasets
Highlight box
Key findings
• The novel prognostic model based on 11 glycosyltransferase (GT)-related genes identified were associated with overall survival (OS) in patients with bladder urothelial carcinoma (BLCA), suggesting that the model had potential predictive value.
What is known and what is new?
• BLCA had a wide range of prognoses ranging from low-grade non-muscle-invasive BLCA, which has a good prognosis but a high recurrence rate, to high-grade muscle-invasive BLCA, which has a poor prognosis. There is currently no model for predicting the prognosis of patients with BLCA.
• This knowledge gap prompted our study, where we identified 11 GT genes that are associated with the prognosis of BLCA. These genes have been integrated into a novel prognostic model, which has demonstrated potential in predicting OS.
What is the implication, and what should change now?
• The new model has the potential to predict the prognosis of BLCA patients, allowing high-risk patients with a poor prognosis to get aggressive treatment options. At the same time, the model's efficacy needs to be validated in clinical settings.
Introduction
Bladder cancer is the most prevalent malignant tumor of the urinary system, with over 90% of cases being bladder urothelial carcinoma (BLCA) (1). BLCA is a highly heterogeneous cancer with varied prognoses ranging from low-grade non-muscle-invasive bladder cancer (NMIBC), which has a high recurrence rate despite a good prognosis, to high-grade muscle-invasive bladder cancer (MIBC), which has a poor prognosis (2,3). Treatment options depend on the disease type, with transurethral resection of bladder tumor (TURBT) being the primary treatment for NMIBC, followed by intravesical chemotherapy or immunotherapy, whereas MIBC is treated with cystectomy combined with chemotherapeutic drugs (4). Despite these treatments, BLCA mortality rates remain high due to the recurrence rate post-TURBT, the risk of progression to more invasive and metastatic forms, and the lack of reliable biomarkers (5).
Glycosylation, a major posttranslational modification in eukaryotic cells, occurs in the endoplasmic reticulum and Golgi apparatus and is driven by glycosyltransferases (GT) and glycosidases (6-8). It contributes to tumor growth by contributes to tumor growth by enabling cancer cells to bypass cell cycle checkpoints, evade apoptosis, and resist immune surveillance during metastasis (9,10). Glycosylation dysregulation is an early event in carcinogenesis and significantly influences cancer development and progression (6), contributing to tumor heterogeneity (2,11), and determining the stage, direction, and fate of tumor development (8,11). Altered glycosylation patterns, which lead to the production of abnormal tumor-associated polysaccharides or glycoproteins, are cancer hallmarks. These molecules, secreted or shed into the bloodstream, serve as tumor-associated biomarkers (12). The currently published model, which is dominated by a single tumor marker, therefore, lacks diagnostic and predictive efficacy (1,13). Comprehensive glycomic analysis is essential for evaluating cancer progression, as demonstrated by the accurate identification of indolent and metastatic prostate cancer using glycomic analysis of biopsy samples, with an accuracy of 91% compared to the conventional evaluation accuracy of 72% (14). Glycosylation dysregulation contributes to the immunosuppressive microenvironment in gliomas (15) and ovarian cancer heterogeneity, with specific glycoforms associated with disease development and severity (16). Therefore, detecting multiple biomarkers is a more practical and comprehensive approach to studying cancer glycosylation patterns (12).
We utilized bioinformatics tools and public databases to identify prognostic GT-related genes in BLCA and developed predictive models. We also analyzed the immune microenvironment differences between high- and low-risk BLCA groups, providing novel insights for BLCA treatment. We present this article in accordance with the TRIPOD reporting checklist (17) (available at https://tau.amegroups.com/article/view/10.21037/tau-2024-632/rc).
Methods
The study was conducted in accordance with the Declaration of Helsinki (as revised in 2013). The study flowchart is presented in Figure S1.
Data source
The messenger RNA (mRNA) expression dataset of BLCA from The Cancer Genome Atlas (TCGA-BLCA) was downloaded (https://portal.gdc.cancer.gov/), including 411 BLCA tumor samples (406 samples with survival information) and 19 normal samples. The GSE13507 dataset from the Gene Expression Omnibus (GEO) database (http://www.ncbi.nlm.nih.gov/geo/) was used as a validation set, including 165 primary bladder cancer samples with survival information. The clinical information of the relevant samples of the dataset is shown in Table S1. Additionally, 169 GT-related genes were obtained from the GlycoGene database (GGDB; http://jcggdb.jp/rcmg/ggdb/).
Identification of differentially expressed GT-related genes in BLCA
In TCGA-BLCA, differentially expressed genes (DEGs) between the BLCA (n=411) and control (n=19) groups were screened using the “DESeq2” package in R (The R Foundation for Statistical Computing) (18). The cutoff criteria for statistical significance were absolute log2 fold change (logFC) >1 and adjusted P value (adj. P) <0.05. The DEGs’ volcano plot and heatmap were drawn using the “ggplot2” and “pheatmap” packages in R (19). The intersections of the DEGs and GT-related genes were considered the differentially expressed GT-related genes (DEGRGs) in BLCA.
Functional enrichment of DEGRGs
The DEGRGs were used to conduct the Gene Ontology (GO) annotation and Kyoto Encyclopedia of Genes and Genomes (KEGG) pathway enrichment analyses via the R package “clusterProfiler” (20). The GO terms comprised the following three divisions: biological process (BP), cellular component (CC), and molecular function (MF). Adj. P<0.05 was considered to indicate statistical significance.
Identification of survival-related DEGRGs and establishment of the risk score model
In the training set, the DEGRGs associated with the OS of patients with BLCA were identified with a univariate Cox proportional hazards (PH) regression model. DEGRGs with hazard ratio (HR) ≠1 and P<0.05 were considered statistically significant and were included in subsequent analyses. The least absolute shrinkage and selection operator (LASSO) regression analysis was performed to minimize the risk of overfitting via 10-fold cross-validation based on the “glmnet” package in R (21). A PH hypothesis test was performed on the genes screened with LASSO regression. Following this, a multivariate Cox regression analysis was performed on the genes that successfully passed the PH hypothesis test. The risk score of patients with BLCA was calculated based on the regression coefficients from the multivariate Cox regression model. Patients in the training set were divided into high-risk and low-risk groups according to the median risk score, which was used as the cutoff value. Kaplan-Meier (KM) survival analysis and the log-rank test were used to analyze and compare the difference in OS between the high- and low-risk groups. Subsequently, receiver operating characteristic (ROC) curve and area under the curve (AUC) analyses were applied to test the predictive power of the prognostic risk score model. AUC value greater than 0.6 was considered to have predictive value. Furthermore, the risk score model was tested in the GEO validation set.
Gene expression analysis of different cisplatin chemotherapy responses and molecular subtypes
According to the response of different cisplatin chemotherapy regimens, the patients with BLCA in GSE169455 could be classified as pathologic complete response (pCR; y-pathological (yp) T0N0), pathologic partial response (pPR; ypT1N0, ypTaN0, or ypTisN0), or no response (yp ≥ T2 or ypN+). The five main LundTax molecular subtype proportions were identified via RNA-based classification and included urothelial-like (Uro; further subdivided into UroA, UroB, and UroC), genomically unstable (GU), basal/squamous (Ba/Sq), mesenchymal-like (Mes-like), and small cell/neuroendocrine-like (Sc/NE). The expressions of the model genes in different cisplatin response groups and molecular subtypes were analyzed.
Tumor-immune microenvironment analysis in the high- and low-risk groups
The ESTIMATE algorithm was used to evaluate the ratio of the immune to stromal components in the tumor microenvironment via the “estimate” R package (22), which generated four scores, including the immune score (reflecting the level of immune cell infiltrations), stromal score (reflecting the presence of stroma), ESTIMATE score (reflecting the sum of both), and tumor purity (percentage of tumor cells). Infiltrating immune cells are critical to effective tumor immunotherapies. The CIBERSORT algorithm (23) was adopted to calculate the abundance of 22 immune cell types in patients with BLCA, with RNA-sequencing data being used to compare the infiltrating immune cells in samples with different risk scores. We also analyzed expression differences of immune checkpoints and human leukocyte antigen (HLA) family genes between the high- and low-risk groups.
Predictive nomogram construction and evaluation
The nomogram, integrating multiple risk factors, was an excellent tool in the assessment and quantification of risk for individuals in a clinical setting. The independent predictive factors identified by univariate Cox regression (P<0.05) were integrated to construct a predictive nomogram via multivariable Cox regression analysis, and the corresponding calibration curve was built using the “rms” R package. The closer the calibration curve was to the 45° line, which represented the best prediction, the better the prognostic prediction performance of the nomogram.
The tumor immune dysfunction and exclusion (TIDE), immunophenoscore, cell stemness, and functional enrichment analyses
In TCGA-BLCA, the TIDE indicators were calculated via the TIDE database (http://tide.dfci.harvard.edu), and their differences between high- and low-risk groups were analyzed separately. To further extrapolate the response of patients with BLCA to immunotherapy, the immune prognostic signature data including immunophenoscore (IPS), IPS-CTLA4- and PD1/PD-L1/PD-L2 blocker, IPS-CTLA4 blocker, and IPS-PD1/PD-L1/PD-L2 blocker were accessed via The Cancer Immunome Atlas website (https://tcia.at/home), and their differences between high- and low-risk groups were compared. Next, Spearman correlation analysis was carried out for the risk score and 32 angiogenesis-related genes. The one-class logistic regression (OCLR) algorithm was used to calculate the mRNA expression-based stemness index (mRNAsi) for each patient with BLCA, and the stemness difference in the two risk groups was compared. Additionally, the Spearman correlation between the risk and mRNAsi scores was analyzed. The KEGG pathway enrichment analysis for the high- and low-risk groups was also applied to identify the related biological functions and pathways.
Statistical analysis
Differential analysis was performed using the DESeq2 package (v1.34.0) (18) in R. Functional enrichment analysis was performed using ClusterProfiler (v4.0.5) (20). LASSO regression analysis was conducted using the glmnet package (21). Additionally, the estimate package (v1.0.13) (22) was used to evaluate the immune stromal components of the tumor microenvironment. The CIBERSORT algorithm (23) was employed to calculate the abundance of 22 immune cell types in BLCA patients. A P value of <0.05 was considered statistically significant.
Results
Identification of DEGRGs in BLCA
A total of 8,864 DEGs (BLCA vs. control) were identified with a threshold of adj. P <0.05 and |logFC| >1, including 5,651 upregulated genes and 3,213 downregulated genes (Figure 1A). The expressions of the top 10 upregulated genes and top 10 downregulated genes (sorted by logFC) are shown in Figure 1B. The Venn diagram showed that 33 genes crossed among 8,864 DEGs and 169 GT-related genes, and these were used as the DEGRGs (Figure 1C).
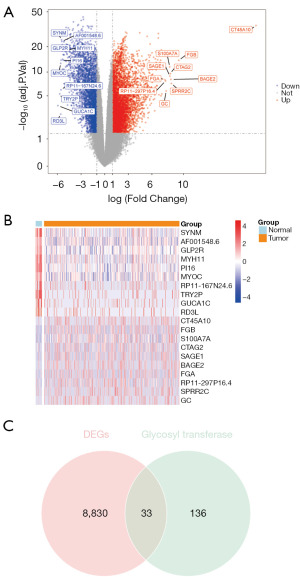
Functional enrichment of DEGRGs
The DEGRGs were significantly enriched into 79 BPs, 6 CCs, 22 MFs, and 20 KEGG pathways (table available at https://cdn.amegroups.cn/static/public/tau-2024-632-1.xlsx, Table S2). The significantly enriched GO-BP terms included “glycoprotein biosynthetic process”, “protein glycosylation”, “macromolecule glycosylation”, “glycosylation”, and “glycoprotein metabolic process”. For the GO-CC analysis, the significantly enriched terms were “Golgi cisterna”, “Golgi stack”, “Golgi cisterna membrane”, “Golgi apparatus subcompartment”, and “integral component of Golgi membrane”. Finally, for the GO-MF analysis, the significantly enriched terms were “glycosyltransferase activity”, “hexosyltransferase activity”, “UDP-glycosyltransferase activity”, “acetylgalactosaminyltransferase activity”, and “sialyltransferase activity” (Figure 2A). In addition, the markedly enriched KEGG pathways for these DEGRGs were “other types of O-glycan biosynthesis”, “mucin-type O-glycan biosynthesis”, “glycosphingolipid biosynthesis-lacto and neolacto series”, “glycosphingolipid biosynthesis-ganglia series”, and “various types of N-glycan biosynthesis” (Figure 2B).
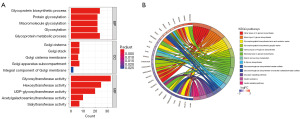
Prognostic prediction model of BLCA based on the DEGRGs
Using the univariate Cox regression model, we analyzed the prognostic value of DEGRGs in 406 BLCA patents with prognostic information. Among them, 12 survival-related DEGRGs were identified (Figure 3A). The LASSO regression algorithm was used for a more precise prediction of BLCA prognosis using DEGRGs, and the optimal model with 12 genes could be attained at lambda.min =0.001187783 (Figure 3B,3C). The PH hypothesis test was performed on the 12 genes selected by LASSO, and 11 genes passed the test. After further implementation of stepwise multivariate Cox regression analysis, these 11 genes were ultimately used to construct the prognostic model. The risk score of each patient was calculated with the gene expression value of the 11 genes and the corresponding regression coefficient (Table 1). Among the genes, GALNTL6, GLT8D2, and GYS2 were low-risk genes (HR <1), while ALG9, B3GALNT2, B3GALT2, CHPF, GALNT15, PYGB, ST6GALNAC3, and ST8SIA6 were high-risk genes (HR >1, Figure 3D). The correlation between the risk score and each clinical characteristic was investigated. Figure 3A shows that the risk score significantly differed among subgroups divided by age and stage (Figure 3E). In GSE169455, the expression data of only 7 model genes (ALG9, B3GALNT2, B4GALNT2, CHPF, GALNTL6, GLT8D2, PYGB) could be extracted. In both the cisplatin response groups and molecular subtypes, CHPF expression was the highest, while GLT8D2 expression was the lowest (Figure 3F).
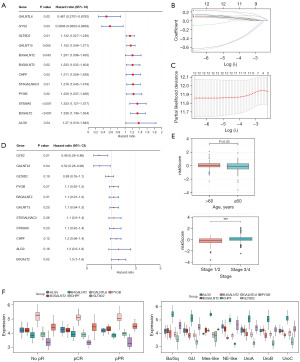
Table 1
Gene | coef |
---|---|
CHPF | 0.15754823 |
PYGB | 0.10346106 |
GLT8D2 | −0.11193863 |
B3GALNT2 | 0.10101936 |
ALG9 | 0.24668539 |
GALNT15 | 0.09162525 |
ST6GALNAC3 | 0.09646491 |
B3GALT2 | 0.24225244 |
ST8SLA6 | 0.13149067 |
GYS2 | −0.71745428 |
GALNTL6 | −0.65704759 |
Evaluation of the prognostic value of risk score and validation of an external independent cohort
We categorized patients into high- and low-risk groups based on their median risk score. The risk score distribution and associated survival status indicated that as risk score increases, so does the likelihood of death (Figure 4A). Next, we evaluated this model using the KM and ROC curves. The KM curve showed that patients in the high-risk group had significantly shorter OS times than did those in the low-risk group (Figure 4B). The ROC curve analysis showed that the 3-, 5-, and 7-year AUC values were 0.65, 0.67, and 0.68 based on the risk score alone (Figure 4C). To confirm the risk signature independently, we applied an 11-gene prognostic signature to an independent GEO cohort. The risk score increase was correlated with higher patient mortality rates (Figure 4D). The low-risk group had a considerably better chance of survival as compared to the high-risk group (Figure 4E). In the ROC curve analysis of risk score alone, the AUC values of the validation set for 3 years, 5 years, and 7 years were 0.64, 0.65 and 0.63, respectively (Figure 4F), and the results were all greater than 0.6, indicating that the model had better prediction accuracy.
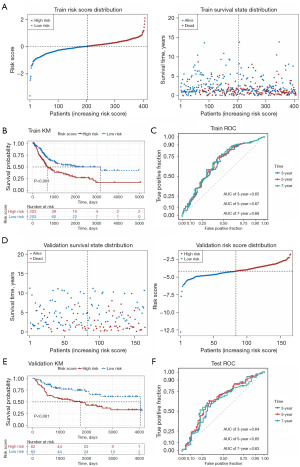
Tumor immune microenvironment analysis of risk score
We proceeded to investigate the immune-stromal components present in the tumor microenvironment (Figure 5A). Immune, stromal, and ESTIMATE scores positively correlated with risk scores and were significantly higher in the high-risk group. The tumor purity was negatively correlated with risk score and was considerably lower in the high-risk group. To further characterize the immune microenvironment of tumors, we estimated the abundance of 22 immune cells in patients with BLCA (Figure 5B), and 11 cell types were significantly different between the high- and low-risk groups (Figure 5C). We also compared the expressions of immune checkpoints and HLA family genes between the high- and low-risk groups. We found that 33 immune checkpoints and 18 HLA family genes significantly differed in expression between the two groups (Figure 5D,5E).
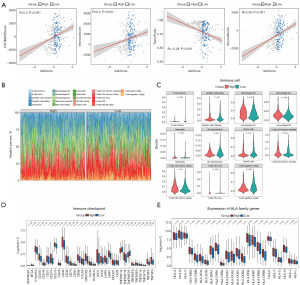
Predictive nomogram construction and evaluation
Based on the results of the univariate Cox regression and multivariate Cox regression analyses (Figure 6A,6B), we further constructed a nomogram based on four independent prognostic factors, including age, stage, T stage, and risk score, to provide a quantitative method for clinicians to predict the probability of 3-, 5- and 7-year OS for patients with BLCA (Figure 6C). Moreover, the calibration curve indicated that in comparison with an ideal model, the nomogram had a similar performance (Figure 6D).
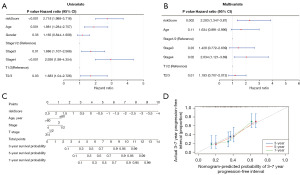
High-risk group patients had less effective immunotherapy, and the glycan synthesis-related pathways were upregulated in the high-risk group
Both the exclusion and TIDE scores were significantly higher in the high-risk group than in the low-risk group, implying that patients in the high-risk group may have a more severe rejection of T cells, resulting in poorer outcomes from receiving immunotherapy (Figure 7A). The box plot showed a significant difference in IPS scores between the high- and low-risk groups, with a lower expression in the high-risk group, further illustrating the poorer immunotherapy outcome of high-risk group patients (Figure 7B). In addition, the risk score was significantly positively correlated with most angiogenesis-related genes, such as FSTL1, COL5A2, and COL3A, and was significantly negatively correlated with VEGFA, TNFRSF21, S100A4, and LRPAP1 (Figure 7C). There was a notable stemness difference between the high- and low-risk groups, and as anticipated, mRNAsi was negatively correlated with risk score (Figure 7D). Additionally, the O-glycan biosynthesis, glycosphingolipid biosynthesis ganglia series, glycosaminoglycan biosynthesis chondroitin sulfate, and other KEGG pathways were upregulated in the high-risk group. Meanwhile, insulin signaling, bladder cancer, and snare interactions in vesicular transport pathways, among others, were downregulated in the low-risk group (Figure 7E).
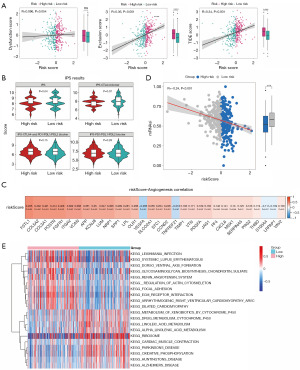
Discussion
BLCA is the most prevalent malignant tumor in the urinary system and is a highly heterogeneous tumor with multiple pathologic types and varied prognoses (1,5), such as the nested variant of urothelial carcinoma is a variant of urothelial carcinoma with aggressive behavior and poor prognosis (24). Moreover, due to the high recurrence rate, the risk of redevelopment to more invasive and metastatic malignancies, and the lack of sensitive biomarkers, existing treatment approaches do not reduce mortality rates in BLCA; the survival rate has remained poor (5). Furthermore, while recently published tumor markers such as FLG (25) and TWIST1 (26) have potential prognostic value, a single biomarker is insufficient for diagnostic and prognostic purposes. Therefore, it is vital to discover novel biomarkers. The study developed a model and evaluated its performance by measuring the AUC for OS at 3, 5, and 7 years, which yielded AUCs of 0.65, 0.67, and 0.68, respectively. These results indicate that the model has good predictive ability. Additionally, KM curves demonstrated that patients placed in the high-risk group had high tumor grades and worse prognoses.
Glycosylation dysregulation contributes to tumor heterogeneity. Changes in glycosylation have been associated with cancer progression and poor prognosis in a variety of malignancies and are now generally acknowledged to be one of the hallmarks of cancer. However, the role of GT-related genes in the outcome and progression of BLCA is unknown. Therefore, we aimed to develop a GT-related predictive model to improve BLCA prognosis prediction.
In this study, we found 11 GT-related genes associated with BLCA: GYS2, GALNTL6, GLT8D2, PYGB, B3GALNT2, GALNT15, ST6GALNAC3, ST8SIA6, CHPF, ALG9, and B3GALT2. CHPF (chondroitin polymerizing factor) with dual GlcAT-II and GalNAcT-II activity participates in energy metabolism and may be associated with glycolysis (27). CHPF regulates TGF-β1 expression, a significant regulator of cancer cell contact with the tumor microenvironment. CHPF stimulates SMAD3 and JNK via activating TGF-β1, enhancing breast cancer cell proliferation, migration, and invasion (28). GYS2 is an isoform of GYS (glycogen synthase) that directly controls glycogen synthesis and is primarily expressed in the liver (29). Low GYS2 expression in intrahepatic cholangiocarcinoma implies a poor prognosis, but GYS2 overexpression dramatically reduces cholangiocarcinoma cell proliferation, migration, and invasion through activating the p53 pathway (30). PYGB (glycogen phosphorylase) is the rate-limiting enzyme in glycogen degradation (29) and is highly expressed in lung cancer (31), prostate cancer (32), breast cancer (33), gastric cancer (34), and ovarian cancer (35,36). PYGB can serve as a diagnostic molecular marker for gastric cancer (34), and its overexpression is associated with poor prognosis in patients with ovarian cancer (35). PYGB influences cancer cell proliferation via multiple routes, including the Wnt/β-catenin pathway (34), the PI3K/AKT pathway (31), and the NF-κB/Nrf2 signaling network (32). GLT8D2 (glycosyltransferase 8 domain containing 2) has been linked to cisplatin resistance, and its overexpression in ovarian cancer cells can promote resistance. Inhibiting the FGFR and PI3K signaling pathways can considerably reduce GLT8D2-induced cisplatin resistance and improve cisplatin’s therapeutic efficacy in ovarian cancer. Targeting the GLT8D2–FGFR–PI3K/AKT axis may improve platinum therapy response in patients with chemotherapy-resistant ovarian cancer (37). B3GALNT2 (β-1,3-N-acetylgalactosaminyltransferase II) is a B3GT (β-1,3-glycosyltransferases) family member (38) that affects normal and malignant tissue development (39). B3GALNT2 lowers acetate secretion, inhibits macrophage inhibitory factor activity, increases macrophage recruitment, and promotes tumor growth in hepatocellular carcinoma, consequently performing metabolic reprogramming and microenvironment remodeling tasks (40). ALG9 (alpha-1,2-mannosyltransferase) mutations can develop in polycystic kidney and liver (41). ST6GALNAC3 (ST6(alpha-N-acetyl-neuraminyl-2,3-beta-galactosyl-1,3)-N-acetylgalactosaminide alpha-2,6-sialyltransferase 3) catalyzes the transfer of sialic acid to GalNAc and is a member of the sialyltransferase enzyme family implicated in the synthesis of sialylated glycoproteins and lipids (42,43). ST6GALNAC3 expression is elevated in several cancers (42,44,45) and plays a central role in cancer biology and immunity (42). According to Haldrup et al., extremely cancer-specific hypermethylation of the ST6GALNAC3 and ZNF660 promoters may be biomarkers for prostate cancer (44). GALNTL6 (polypeptide N-acetylgalactosaminyltransferase-like 6) overexpression is linked to a decreased survival time in individuals with thyroid cancer (46). ST8SIA6 (ST8 alpha-N-acetyl-neuraminide alpha-2,8-sialyltransferase 6) modulates immune cell infiltration and immunotherapy response in colon cancer (47). Except for those of B3GALT2 (UDP-Gal:betaGlcNAc beta 1,3-galactosyltransferase, polypeptide 2), which is a reliable predictor of BLCA (48), the functions of the remaining 10 GT-related genes in BLCA are unknown.
The high-risk and low-risk groups also exhibited differences in the abundance of immune cells, including memory B cells, resting dendritic cells, M1 and M2 macrophages, neutrophils, activated natural killer cells, plasma cells, resting and activated memory CD4 T cells, naive T cells, and regulatory T cells, and immune status is an essential factor in determining the prognosis of BLCA (49-51). The immune status of BLCA is determined by the types and proportions of immune cells and matrix cells in tumor tissue (49). Notably, the 11-gene GT-related model can be used to determine whether a patient can respond to immunotherapy. The exclusion score and TIDE score were significantly higher in the high-risk group, indicating that patients in the high-risk group had greater T-cell rejection and did not respond to immunotherapy, which was further confirmed by IPS analysis. In addition, there were significant differences in mRNAsi between the high-risk and low-risk groups. Cancer stem cells (CSCs) are significantly correlated with tumorigenesis, prognosis, clinical stage of American Joint Committee on Cancer (AJCC), and bone metastasis in patients with BLCA. CSC is essential to tumor recurrence and chemotherapy resistance (52). The characteristics of CSC can be identified by the mRNAsi DNA methylation-based stemness index (53). mRNAsi was significantly upregulated in BLCA and increased with higher tumor stage, with T3 having the profile with greatest cell stemness. mRNAsi is considered a repredictor of BLCA occurrence, metastasis, and patient prognosis (54). The lower the mRNAsi score is, the better the OS and treatment outcome (53).
We discovered a novel set of molecular indicators that reliably predict clinical outcomes in patients with BLCA. According to KM analysis, patients with high-risk scores have a relatively short OS. These findings emphasize the critical predictive impact of risk scores for the 11 GT-associated genes. This model not only provides a new means to predict the prognosis of patients with BLCA but may also generate therapeutic alternatives in clinical practice. Furthermore, assessing multiple biomarkers simultaneously may be a more feasible and thorough means of diagnosing BLCA.
However, certain limitations of our research should be acknowledged. This study depended on database analysis, and the results were not confirmed in vitro or in vivo. Moreover, we employed a retrospective design; further validation through a prospective study is required to support our findings. Finally, additional study with experimental confirmation is required to understand the relationship between GT-related genes and BLCA prognosis.
Conclusions
In conclusion, we identified 11 GT-related genes that are associated with OS in patients with BLCA, which may be useful for prognostic prediction. However, further validation and experimental studies are required to fully comprehend the function of these genes and their effect on BLCA.
Acknowledgments
Funding: The study was supported by
Footnote
Reporting Checklist: The authors have completed the TRIPOD reporting checklist. Available at https://tau.amegroups.com/article/view/10.21037/tau-2024-632/rc
Peer Review File: Available at https://tau.amegroups.com/article/view/10.21037/tau-2024-632/prf
Conflicts of Interest: All authors have completed the ICMJE uniform disclosure form (available at https://tau.amegroups.com/article/view/10.21037/tau-2024-632/coif). The authors have no conflicts of interest to declare.
Ethical Statement: The authors are accountable for all aspects of the work in ensuring that questions related to the accuracy or integrity of any part of the work are appropriately investigated and resolved. The study was conducted in accordance with the Declaration of Helsinki (as revised in 2013).
Open Access Statement: This is an Open Access article distributed in accordance with the Creative Commons Attribution-NonCommercial-NoDerivs 4.0 International License (CC BY-NC-ND 4.0), which permits the non-commercial replication and distribution of the article with the strict proviso that no changes or edits are made and the original work is properly cited (including links to both the formal publication through the relevant DOI and the license). See: https://creativecommons.org/licenses/by-nc-nd/4.0/.
References
- Sung H, Ferlay J, Siegel RL, et al. Global Cancer Statistics 2020: GLOBOCAN Estimates of Incidence and Mortality Worldwide for 36 Cancers in 185 Countries. CA Cancer J Clin 2021;71:209-49. [Crossref] [PubMed]
- Meeks JJ, Al-Ahmadie H, Faltas BM, et al. Genomic heterogeneity in bladder cancer: challenges and possible solutions to improve outcomes. Nat Rev Urol 2020;17:259-70. [Crossref] [PubMed]
- Lopez-Beltran A, Cookson MS, Guercio BJ, et al. Advances in diagnosis and treatment of bladder cancer. BMJ 2024;384:e076743. [Crossref] [PubMed]
- Lenis AT, Lec PM, Chamie K, et al. Bladder Cancer: A Review. JAMA 2020;324:1980-91. [Crossref] [PubMed]
- Berdik C. Unlocking bladder cancer. Nature 2017;551:S34-5. [Crossref] [PubMed]
- Grzesik K, Janik M, Hoja-Łukowicz D. The hidden potential of glycomarkers: Glycosylation studies in the service of cancer diagnosis and treatment. Biochim Biophys Acta Rev Cancer 2023;1878:188889. [Crossref] [PubMed]
- Reily C, Stewart TJ, Renfrow MB, et al. Glycosylation in health and disease. Nat Rev Nephrol 2019;15:346-66. [Crossref] [PubMed]
- Varki A, Kannagi R, Toole B, et al. Glycosylation Changes in Cancer. In: Varki A, Cummings RD, Esko JD, et al. editors. Essentials of Glycobiology. Cold Spring Harbor (NY): Cold Spring Harbor Laboratory Press; 2015:597-609.
- Ferreira IG, Pucci M, Venturi G, et al. Glycosylation as a Main Regulator of Growth and Death Factor Receptors Signaling. Int J Mol Sci 2018;19:580. [Crossref] [PubMed]
- He M, Zhou X, Wang X. Glycosylation: mechanisms, biological functions and clinical implications. Signal Transduct Target Ther 2024;9:194. [Crossref] [PubMed]
- Pinho SS, Reis CA. Glycosylation in cancer: mechanisms and clinical implications. Nat Rev Cancer 2015;15:540-55. [Crossref] [PubMed]
- Silsirivanit A. Glycosylation markers in cancer. Adv Clin Chem 2019;89:189-213. [Crossref] [PubMed]
- Guo CC, Czerniak B. Bladder Cancer in the Genomic Era. Arch Pathol Lab Med 2019;143:695-704. [Crossref] [PubMed]
- Murphy K, Murphy BT, Boyce S, et al. Integrating biomarkers across omic platforms: an approach to improve stratification of patients with indolent and aggressive prostate cancer. Mol Oncol 2018;12:1513-25. [Crossref] [PubMed]
- Chang X, Pan J, Zhao R, et al. DDOST Correlated with Malignancies and Immune Microenvironment in Gliomas. Front Immunol 2022;13:917014. [Crossref] [PubMed]
- Pan J, Hu Y, Sun S, et al. Glycoproteomics-based signatures for tumor subtyping and clinical outcome prediction of high-grade serous ovarian cancer. Nat Commun 2020;11:6139. [Crossref] [PubMed]
- Collins GS, Reitsma JB, Altman DG, et al. Transparent reporting of a multivariable prediction model for individual prognosis or diagnosis (TRIPOD): the TRIPOD statement. BMJ 2015;350:g7594. [Crossref] [PubMed]
- Kang K, Xie F, Mao J, et al. Significance of Tumor Mutation Burden in Immune Infiltration and Prognosis in Cutaneous Melanoma. Front Oncol 2020;10:573141. [Crossref] [PubMed]
- Zhang MY, Huo C, Liu JY, et al. Identification of a Five Autophagy Subtype-Related Gene Expression Pattern for Improving the Prognosis of Lung Adenocarcinoma. Front Cell Dev Biol 2021;9:756911. [Crossref] [PubMed]
- Yu G, Wang LG, Han Y, et al. clusterProfiler: an R package for comparing biological themes among gene clusters. OMICS 2012;16:284-7. [Crossref] [PubMed]
- Zhang M, Zhu K, Pu H, et al. An Immune-Related Signature Predicts Survival in Patients With Lung Adenocarcinoma. Front Oncol 2019;9:1314. [Crossref] [PubMed]
- Yoshihara K, Shahmoradgoli M, Martínez E, et al. Inferring tumour purity and stromal and immune cell admixture from expression data. Nat Commun 2013;4:2612. [Crossref] [PubMed]
- Newman AM, Liu CL, Green MR, et al. Robust enumeration of cell subsets from tissue expression profiles. Nat Methods 2015;12:453-7. [Crossref] [PubMed]
- Yang Y, Li J, Qi R, et al. A case of bladder urothelial carcinoma nested variant with invasion to the rectum treated with surgery and intraoperative radiotherapy. Quant Imaging Med Surg 2023;13:5409-16. [Crossref] [PubMed]
- Chen L, Huang X, Xiong L, et al. Analysis of prognostic oncogene filaggrin (FLG) wild-type subtype and its implications for immune checkpoint blockade therapy in bladder urothelial carcinoma. Transl Androl Urol 2022;11:1419-32. [Crossref] [PubMed]
- Wan M, Meng H, Li H. Potential role of TWIST1 and its methylation in bladder urothelial carcinoma. Transl Cancer Res 2024;13:6070-86. [Crossref] [PubMed]
- Liu G, Wu X, Chen J. Identification and validation of a glycolysis-related gene signature for depicting clinical characteristics and its relationship with tumor immunity in patients with colon cancer. Aging (Albany NY) 2022;14:8700-18. [Crossref] [PubMed]
- Pan QF, Ouyang WW, Zhang MQ, et al. Chondroitin polymerizing factor predicts a poor prognosis and promotes breast cancer progression via the upstream TGF-β1/SMAD3 and JNK axis activation. J Cell Commun Signal 2023;17:89-102. [Crossref] [PubMed]
- Zois CE, Harris AL. Glycogen metabolism has a key role in the cancer microenvironment and provides new targets for cancer therapy. J Mol Med (Berl) 2016;94:137-54. [Crossref] [PubMed]
- A S, Wu H, Wang X, et al. Value of glycogen synthase 2 in intrahepatic cholangiocarcinoma prognosis assessment and its influence on the activity of cancer cells. Bioengineered 2021;12:12167-78.
- Zhan Y, Chen R, Wang T, et al. Glycogen phosphorylase B promotes cell proliferation and migration through PI3K/AKT pathway in non-small cell lung cancer. Exp Lung Res 2021;47:111-20. [PubMed]
- Wang Z, Han G, Liu Q, et al. Silencing of PYGB suppresses growth and promotes the apoptosis of prostate cancer cells via the NF-κB/Nrf2 signaling pathway. Mol Med Rep 2018;18:3800-8. [Crossref] [PubMed]
- Altemus MA, Goo LE, Little AC, et al. Breast cancers utilize hypoxic glycogen stores via PYGB, the brain isoform of glycogen phosphorylase, to promote metastatic phenotypes. PLoS One 2019;14:e0220973. [Crossref] [PubMed]
- Xia B, Zhang K, Liu C. PYGB Promoted Tumor Progression by Regulating Wnt/β-Catenin Pathway in Gastric Cancer. Technol Cancer Res Treat 2020;19:1533033820926592. [Crossref] [PubMed]
- Zhang QF, Li YK, Chen CY, et al. Identification and validation of a prognostic index based on a metabolic-genomic landscape analysis of ovarian cancer. Biosci Rep 2020;40:BSR20201937. [Crossref] [PubMed]
- Zhou Y, Jin Z, Wang C. Glycogen phosphorylase B promotes ovarian cancer progression via Wnt/β-catenin signaling and is regulated by miR-133a-3p. Biomed Pharmacother 2019;120:109449. [Crossref] [PubMed]
- Huang S, Liang S, Chen G, et al. Overexpression of glycosyltransferase 8 domain containing 2 confers ovarian cancer to CDDP resistance by activating FGFR/PI3K signalling axis. Oncogenesis 2021;10:55. [Crossref] [PubMed]
- Hiruma T, Togayachi A, Okamura K, et al. A novel human beta1,3-N-acetylgalactosaminyltransferase that synthesizes a unique carbohydrate structure, GalNAcbeta1-3GlcNAc. J Biol Chem 2004;279:14087-95. [Crossref] [PubMed]
- Stevens E, Carss KJ, Cirak S, et al. Mutations in B3GALNT2 cause congenital muscular dystrophy and hypoglycosylation of α-dystroglycan. Am J Hum Genet 2013;92:354-65. [Crossref] [PubMed]
- Yang T, Wang Y, Dai W, et al. Increased B3GALNT2 in hepatocellular carcinoma promotes macrophage recruitment via reducing acetoacetate secretion and elevating MIF activity. J Hematol Oncol 2018;11:50. [Crossref] [PubMed]
- Besse W, Chang AR, Luo JZ, et al. ALG9 Mutation Carriers Develop Kidney and Liver Cysts. J Am Soc Nephrol 2019;30:2091-102. [Crossref] [PubMed]
- Pearce OM, Läubli H. Sialic acids in cancer biology and immunity. Glycobiology 2016;26:111-28. [Crossref] [PubMed]
- Tsuchida A, Ogiso M, Nakamura Y, et al. Molecular cloning and expression of human ST6GalNAc III: restricted tissue distribution and substrate specificity. J Biochem 2005;138:237-43. [Crossref] [PubMed]
- Haldrup C, Pedersen AL, Øgaard N, et al. Biomarker potential of ST6GALNAC3 and ZNF660 promoter hypermethylation in prostate cancer tissue and liquid biopsies. Mol Oncol 2018;12:545-60. [Crossref] [PubMed]
- Hatano K, Miyamoto Y, Nonomura N, et al. Expression of gangliosides, GD1a, and sialyl paragloboside is regulated by NF-κB-dependent transcriptional control of α2,3-sialyltransferase I, II, and VI in human castration-resistant prostate cancer cells. Int J Cancer 2011;129:1838-47. [Crossref] [PubMed]
- Ren S, Cao W, Ma J, et al. Correlation evaluation between cancer microenvironment related genes and prognosis based on intelligent medical internet of things. Front Genet 2023;14:1132242. [Crossref] [PubMed]
- Ko CY, Chu TH, Hsu CC, et al. Bioinformatics Analyses Identify the Therapeutic Potential of ST8SIA6 for Colon Cancer. J Pers Med 2022;12:401. [Crossref] [PubMed]
- Xu C, Song L, Peng H, et al. Clinical Eosinophil-Associated Genes can Serve as a Reliable Predictor of Bladder Urothelial Cancer. Front Mol Biosci 2022;9:963455. [Crossref] [PubMed]
- Pan S, Li S, Zhan Y, et al. Immune status for monitoring and treatment of bladder cancer. Front Immunol 2022;13:963877. [Crossref] [PubMed]
- Ghosh RK, Pandey T, Dey P. Liquid biopsy: A new avenue in pathology. Cytopathology 2019;30:138-43. [Crossref] [PubMed]
- Birkenkamp-Demtröder K, Christensen E, Nordentoft I, et al. Monitoring Treatment Response and Metastatic Relapse in Advanced Bladder Cancer by Liquid Biopsy Analysis. Eur Urol 2018;73:535-40. [Crossref] [PubMed]
- Kang Y, Zhu X, Wang X, et al. Identification and Validation of the Prognostic Stemness Biomarkers in Bladder Cancer Bone Metastasis. Front Oncol 2021;11:641184. [Crossref] [PubMed]
- Yang Z, Li C, Fan Z, et al. Single-cell Sequencing Reveals Variants in ARID1A, GPRC5A and MLL2 Driving Self-renewal of Human Bladder Cancer Stem Cells. Eur Urol 2017;71:8-12. [Crossref] [PubMed]
- Pan S, Zhan Y, Chen X, et al. Identification of Biomarkers for Controlling Cancer Stem Cell Characteristics in Bladder Cancer by Network Analysis of Transcriptome Data Stemness Indices. Front Oncol 2019;9:613. [Crossref] [PubMed]
(English Language Editor: J. Gray)