Relative fat mass is a valuable predictor of lower urinary tract symptoms suggestive of benign prostatic hyperplasia in aging males: clinical implications
Highlight box
Key findings
• Positive cross-sectional and longitudinal associations between relative fat mass (RFM) and lower urinary tract symptoms suggestive of benign prostate hyperplasia (LUTS/BPH) were observed.
What is known and what is new?
• Obesity independently increases the prevalence of LUTS/BPH, with prior research suggesting that several anthropometric indices related to lipid accumulation, such as the cardiometabolic index and waist-to-height ratio, possess prognostic potential for BPH risk. Meanwhile, RFM, an innovative metric derived from height to waist circumference ratio, has been introduced and validated as a straightforward yet superior method for estimating body fat percentage in adults compared with conventional indicators including body mass index and waist circumference.
• This study explored the cross-sectional and longitudinal associations of RFM with the prevalence and incidence of LUTS/BPH in aging males.
What is the implication, and what should change now?
• Employing RFM as a simple predictive tool could facilitate prompt detection and management strategies for LUTS/BPH in aging males.
Introduction
Benign prostatic hyperplasia (BPH), a precursor to lower urinary tract symptoms (LUTS), is predominantly diagnosed in aging males, with the incidence escalating as age advances (1). In 2019, it was estimated that there were 94.0 million cases of BPH globally, accounting for a global prevalence of 2.48%, predominantly among individuals aged 65–74 years. The same study reported prevalence rates of 2.22% in China, 2.25% in Western Europe, and 1.80% in the USA. Moreover, the prevalence of BPH across all age groups significantly increased from 2000 to 2019 (2). Moreover, the probability of experiencing associated LUTS, characterized by urgent and frequent urination, urinary retention, and other symptoms, progressively increases with age (1). LUTS/BPH imposes a considerable burden on the health of aging males (3) and contributes to increased healthcare costs (4).
An extensive array of modifiable and nonmodifiable risk factors influences the progression of LUTS/BPH. Apart from non-modifiable risk factors including genetics and age, the primary modifiable risk factors include obesity, glucose intolerance and diabetes, dyslipidemia, physical activity, consumption of cigarettes and alcohol, hypertension, diet (5,6), levels of sex hormones (7,8), chronic inflammation (9), sleep duration (10), depression (11), the gut microbiota (12), educational level, use of antidepressants, erectile function or dysfunction, and treatments for cardiac diseases (13). Furthermore, some studies have also revealed an association between LUTS/BPH and conditions such as asthma (14) or severe acute respiratory syndrome coronavirus 2 infection (15). Impressively, metabolic syndrome (MetS) accelerates the development of LUTS/BPH by the activation of inflammatory pathways in the prostate (16,17). It is reported that obesity, a component of MetS or the recently proposed circadian syndrome (CircS), independently increases the prevalence of LUTS/BPH (18-20). Various anthropometric measures including body mass index (BMI), visceral adiposity index, and waist-to-hip ratio are employed to characterize obesity and assess its impact on different diseases. Relative fat mass (RFM), an innovative metric derived from height to waist circumference ratio, has been introduced and validated as a simple yet superior method for estimating body fat percentage in adults compared with conventional indicators including BMI and waist circumference (21-25). RFM has demonstrated enhanced predictive capabilities for conditions including type 2 diabetes (26,27), hypertension, and arthritis (25). Research by Huang et al. also indicated that several anthropometric indices related to lipid accumulation, including the cardiometabolic index and waist-to-height ratio, hold predictive potential for BPH risk in southern China (28), suggesting that RFM may also serve as a simple yet accurate predictor for LUTS/BPH. However, the link between RFM and LUTS/BPH remains relatively uninvestigated.
This study aims to explore both the cross-sectional and longitudinal associations of RFM with the prevalence and incidence of LUTS/BPH in elderly males, utilizing the China Health and Retirement Longitudinal Study (CHARLS) data. Establishing a robust association between RFM and LUTS/BPH could facilitate prompt detection and therefore corresponding management strategies for LUTS/BPH within this demographic, with the use of RFM as a simple and effective predictive tool. Intervention and treatment for LUTS, including prostate surgery, should not be delayed in these patients. We present this article in accordance with the STROBE reporting checklist (available at https://tau.amegroups.com/article/view/10.21037/tau-24-446/rc).
Methods
Data sources and study populations
All data used in this study were sourced from CHARLS. CHARLS is a national long-term program launched in 2011, with follow-ups every 2–3 years, focusing on the Chinese aging population. It has conducted four waves of data collection respectively in 2011, 2013, 2015, and most recently, 2018. To secure a typical aging population, a multistage stratified sampling method weighted according to population size was employed by CHARLS. Comprehensive details on CHARLS are available in the official publication or online (http://charls.pku.edu.cn/). CHARLS was thoroughly reviewed and approved by the ethics review board of Peking University (Beijing, China; IRB No. 00001052-11014). Informed consent, both written and oral, was obtained from all respondents prior to their involvement in the CHARLS.
In this study, a 2011 baseline survey in which 17,705 participants from 28 provinces were sampled was employed to investigate the cross-sectional association of RFM with prevalence of LUTS/BPH. We excluded females, participants under 40 years of age, missing values, and height and waist circumference outliers, ultimately accounting for 6,153 males (Figure 1). Among them, 4,321 males were followed up until 2018 to search for a longitudinal relationship between RFM and the occurrence of LUTS/BPH. In addition, 2011–2013 and 2011–2015 retrospective cohorts were established for sensitivity tests. The study was conducted in accordance with the Declaration of Helsinki (as revised in 2013).
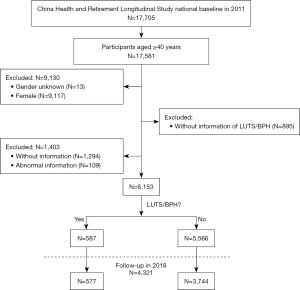
Evaluation of RFM and LUTS/BPH
RFM was computed as in males (21,25,29,30). The waist circumference was measured by measuring with a tape measure placed over clothing. The measurements were documented in centimeters. The height was assessed with a vertical height gauge. The participants were instructed to stand barefoot, maintaining an erect posture, with their heads aligned with their eye-ear level. The recordings were documented in metric units.
In this study, LUTS/BPH was identified using self-reports of doctor-diagnosed prostatic conditions instead of pathological dissection and ultrasonographic examination, to simplify procedures for large-scale national cohorts. All the male participants were asked “Have you previously received a diagnosis of any prostate-related condition, such as benign prostatic hyperplasia, with the exclusion of prostate cancer?” Symptoms of LUTS, comprising nocturia, frequent urination, and urinary retention, were explained to all the participants. Participants who responded with a “yes” were defined as having LUTS/BPH. The same way to determine LUTS/BPH has been employed in prior studies (11,31).
Venous blood collection and blood biomarkers measurements
With all the participants instructed to maintain an uninterrupted state of fasting overnight, trained medical personnel meticulously collected venous blood samples during the morning. Collected samples were subsequently spun in a centrifuge to isolate plasma, which was promptly saved at −20 °C and later conveyed to Peking University for storage at −80 °C and further examination of blood biomarkers. A more comprehensive procedure for the determination of blood biomarkers was delineated in a prior study (32).
Measurements of covariates
The covariates adopted by this study can be divided into four parts: demographic variables, medical history, lifestyle variables, and blood biomarkers. Demographic variables included age (years), educational level (literate or illiterate), and sex (male or female). The term “illiterate” referred to participants whose education did not exceed elementary schooling. Lifestyle factors were defined by afternoon napping (yes or no), sleep duration (≤6, >6 and ≤8, and >8 hours) (11), and smoking (recent smoker, nonsmoker, or former smoker) and drinking (more than monthly, less than monthly, or not at all). The medical history consisted of conditions such as hyperuricemia, hypertension, and depression. Depression was identified via the questionnaire of Center for Epidemiological Studies Depression Scale-10 (CESD-10), with a score ≥10 indicating the presence of depression (11). Hypertension was classified by a systolic pressure exceeding 140 mmHg, a diastolic pressure exceeding 90 mmHg, or the use of antihypertensive medication. Hyperuricemia was characterized by a blood uric acid concentration exceeding 420 µmol/L for males (33). Blood biomarkers measured consisted of levels of triglycerides (mg/dL), blood glucose (mg/dL), high-density lipoprotein (HDL) (mg/dL), low-density lipoprotein (LDL) (mg/dL), and total cholesterol (mg/dL).
Statistical analyses
The RFM was categorized into tertiles, ranging from the minimum (T1) to the maximum (T3). Data were demonstrated as mean (lower quantile, upper quantile) for continuous measures with negative normality test, mean ± standard deviation (SD) for continuous variables with positive normality test, and n (%) for categorical variables. The differences of continuous and categorical variables across the tertiles were assessed with one-way analysis of variance for the former and Chi-squared test for the latter. Given the quantities of missing values of covariates which threatened to deplete our sample size, we initially conducted interpolation employing multivariate imputation using chained equations grounded on the random forest method before analyses for association between RFM and LUTS/BPH. We subsequently used binary logistic regression targeting the cross-sectional association of RFM with LUTS/BPH. We established a series of logistic regression models to evaluate both the cross-sectional and longitudinal associations of RFM with LUTS/BPH based on the 2011 baseline datasets, incorporating subsequent data from 2018. Model 1, the crude unadjusted model; Model 2, refined by adjustments for demographic attributes comprising age and educational levels; Model 3, additionally refined by adjustments for sleep length, cigarette and alcohol use, hypertension, and depression; Model 4, expanded to include adjustments for a suite of blood biomarkers consisting of glucose, LDL, triglycerides, HDL, total cholesterol, and uric acid.
Furthermore, a range of sensitivity tests were performed to validate the integrity and robustness of our results. Firstly, RFM was treated as a continuous variable across four models to ascertain the significance of its relationship with LUTS/BPH. Additionally, we employed restricted cubic spline (RCS) regression, featuring three knots, targeting the dose-response relationship between them. Secondly, subgroup and interactive analyses were carried out targeting the RFM-LUTS/BPH relationship in specific subgroups and examining whether interactive effects of covariates potentially exist. Thirdly, recognizing the potential methodological flaw in categorizing RFM into three groups based on tertiles, we opted instead to segment RFM into two groups using the median and into four groups based on quartiles, thereby reevaluating the aforementioned models. Fourthly, the cross-sectional relationship was verified after participants aged ≤60 years were excluded to mitigate age-related bias. Finally, we employ the 2011–2013 and 2011–2015 cohorts as supplements to check the longitudinal association between RFM and LUTS/BPH.
All the statistical analyses were executed with R 4.4.1 software (R Foundation for Statistical Computing, Vienna, Austria). A two-tailed P<0.05 denotes the significance of statistics.
Results
Participant characteristics from the 2011 CHARLS baseline survey
Following data cleaning, a total of 6253 males were successfully enrolled in the 2011 baseline survey, as detailed in Table 1. The participants in the T3 group were inclined to be literate, smoke less, sleep longer, have afternoon naps, have depression and reduced blood pressure, and have LUTS/BPH, with statistical significance indicated by P<0.05. With respect to blood biomarkers, these individuals exhibited reduced levels of HDL cholesterol as well as elevated levels of uric acid, LDL cholesterol, total cholesterol, glucose, and triglycerides, all of which were highly significantly different (P<0.001). A comparable distribution of participant characteristics post-interpolation can be found in Table S1.
Table 1
Characteristics | Total (n=6,153) | Tertiles of RFM | P value | ||
---|---|---|---|---|---|
T1 (n=2,050) | T2 (n=2,051) | T3 (n=2,052) | |||
Age (years) | 59.00 (53.00, 66.00) | 59.00 (53.00, 66.00) | 59.00 (53.00, 66.00) | 59.00 (52.00, 66.00) | 0.62 |
Educational levels | <0.001 | ||||
Literate | 4,163 (67.66) | 1,307 (63.76) | 1,365 (66.55) | 1,491 (72.66) | |
Illiterate | 1,990 (32.34) | 743 (36.24) | 686 (33.45) | 561 (27.34) | |
Cigarette consumption | <0.001 | ||||
Current smoker | 3,591 (58.36) | 1,383 (67.46) | 1,219 (59.43) | 989 (48.20) | |
Non-smoker | 1,540 (25.03) | 422 (20.59) | 507 (24.72) | 611 (29.78) | |
Ex-smoker | 1,022 (16.61) | 245 (11.95) | 325 (15.85) | 452 (22.03) | |
Alcohol consumption | 0.14 | ||||
Drink more than once a month | 2,757 (44.81) | 924 (45.07) | 944 (46.03) | 889 (43.32) | |
Drink but less than once a month | 666 (10.82) | 229 (11.17) | 230 (11.21) | 207 (10.09) | |
None of these | 2,730 (44.37) | 897 (43.76) | 877 (42.76) | 956 (46.59) | |
Depression | n=5,912 | n=1,958 | n=1,972 | n=1,982 | <0.001 |
No | 4,392 (74.29) | 1,380 (70.48) | 1,461 (74.09) | 1,551 (78.25) | |
Yes | 1,520 (25.71) | 578 (29.52) | 511 (25.91) | 431 (21.75) | |
Hypertension | n=6,113 | n=2,037 | n=2,039 | n=2,037 | <0.001 |
No | 2,256 (36.90) | 503 (24.69) | 684 (33.55) | 1,069 (52.48) | |
Yes | 3,857 (63.10) | 1,534 (75.31) | 1,355 (66.45) | 968 (47.52) | |
Sleep duration (hours) | n=6,127 | n=2,036 | n=2,046 | n=2,045 | 0.04 |
≤6 | 2,956 (48.25) | 1,021 (50.15) | 996 (48.68) | 939 (45.92) | |
>6 and ≤8 | 2,671 (43.59) | 843 (41.40) | 898 (43.89) | 930 (45.48) | |
>8 | 500 (8.16) | 172 (8.45) | 152 (7.43) | 176 (8.61) | |
Afternoon nap | <0.001 | ||||
No | 2,460 (39.98) | 902 (44.00) | 841 (41.00) | 717 (34.94) | |
Yes | 3,693 (60.02) | 1,148 (56.00) | 1,210 (59.00) | 1,335 (65.06) | |
Uric acid (mg/dL) | 4.97±1.28 | 4.72±1.18 | 4.93±1.25 | 5.24±1.34 | <0.001 |
LDL cholesterol (mg/dL) | 110.18 (90.08, 131.83) | 105.54 (86.21, 125.84) | 109.41 (90.85, 131.06) | 115.21 (93.36, 138.02) | <0.001 |
Total cholesterol (mg/dL) | 184.41 (162.37, 209.15) | 178.61 (157.73, 204.12) | 185.18 (163.53, 207.99) | 189.82 (167.40, 215.72) | <0.001 |
HDL cholesterol (mg/dL) | 48.33 (39.05, 59.54) | 54.12 (44.85, 64.18) | 49.87 (39.82, 60.70) | 42.14 (34.41, 50.26) | <0.001 |
Glucose (mg/dL) | 102.60 (94.32, 114.48) | 100.08 (92.52, 109.08) | 102.06 (93.78, 113.58) | 106.20 (97.38, 121.86) | <0.001 |
Triglycerides (mg/dL) | 97.35 (69.92, 145.14) | 80.54 (61.95, 110.62) | 94.69 (68.14, 139.83) | 122.13 (87.61, 186.74) | <0.001 |
LUTS/BPH | <0.001 | ||||
No | 5,566 (90.46) | 1,894 (92.39) | 1,859 (90.64) | 1,813 (88.35) | |
Yes | 587 (9.54) | 156 (7.61) | 192 (9.36) | 239 (11.65) |
Data are demonstrated as mean (lower quantile, upper quantile) for continuous variables with negative normality test, mean ± SD for continuous variables with positive normality test, and n (%) for categorical data. . RFM was categorized into tertiles, designated as T1, T2, and T3, representing groups from lower to higher respectively. The differences of covariates across tertiles were tested using one-way analysis of variance for continuous variables and Chi-squared test for categorical variables. CHARLS, China Health and Retirement Longitudinal Study; RFM, relative fat mass; LDL, low-density lipoprotein; HDL, high-density lipoprotein; LUTS/BPH, lower urinary tract symptoms suggestive of benign prostate hyperplasia; SD, standard deviation.
The cross-sectional association of RFM with prevalent LUTS/BPH
High RFM in the T3 group was significantly correlated with a greater incidence of LUTS/BPH (Figure 2). According to the crude model, the prevalent LUTS/BPH increased by a factor of 1.25 [95% confidence interval (CI): 1.01–1.56; P=0.045] in the T2 group as well as 1.60 (95% CI: 1.30–1.98; P<0.001) in the T3 group. In the final model, after adjusting multiple demographic and lifestyle covariates, hypertension, depression, and blood biomarkers, the risk within the T2 and T3 cohorts was discerned to be 1.23 times (95% CI: 0.97–1.55; P=0.07) and 1.47 times higher than the reference (95% CI: 1.16–1.87; P=0.001). The quartet of regression models uniformly confirmed the escalated risk of LUTS/BPH concomitant with increased RFM, with P of Models 1 and 2 being less than 0.001 and P values of Models 3 and 4 being equal to 0.001.
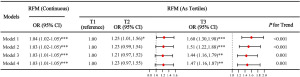
When treated as a continuous variable across the regression analyses, RFM consistently demonstrated a positive correlation with LUTS/BPH, yielding odds ratios (ORs) of 1.04 (95% CI: 1.02–1.05) and 1.03 (95% CI: 1.01–1.05) with both P<0.001 in the final analysis per unit increment in RFM, thereby substantiating the heightened risk of LUTS/BPH with increasing RFM.
Given the absence of a rationale to stratify RFM by tertiles, we bifurcated RFM using the median and further divided it into quartiles for reevaluation as a part of sensitivity assessments. As Figure 3 exhibits, group 2 presented 1.32 times (95% CI: 1.11–1.57; P=0.002) elevation of prevalence in the unadjusted model and 1.23 times (95% CI: 1.02–1.48; P=0.03) elevation of prevalence in the final model when the median RFM was used to partition participants. When the data were stratified into four groups by quantiles, the Q3 manifested 1.33 times risk (95% CI: 1.03–1.71; P=0.03) in the unadjusted model and 1.30 times risk (95% CI: 0.99–1.71; P=0.055) in the final model, whereas the Q4 presented 1.65 times risk (95% CI: 1.29–2.11; P<0.001) in the unadjusted model and 1.54 times risk (95% CI: 1.17–2.03; P=0.001) in the final model. The findings further endorsed the elevated prevalent LUTS/BPH for high RFM, all P for trend <0.01.

Upon the exclusion of 3,455 males aged ≤60 years, the results continued to underscore the robust association in line with our previously demonstrated findings with all P values for trend <0.01 for tertiles of RFM and all the P<0.001 for continuous RFM (Table S2).
All the sensitivity analyses above further substantiate the robustness of our models.
Association of tertiles of RFM with prevalence of LUTS/BPH in subgroup and interactive analyses
In most subgroups delineated in Figure 4, a significant elevation in prevalent LUTS/BPH with higher RFM was demonstrated. However, the ORs at T3 were 1.34 (95% CI: 0.72–2.48; P=0.35) for respondents aged ≤50 years, 1.53 (95% CI: 0.98–2.37; P=0.06) for nonsmokers, 1.24 (95% CI: 0.79–1.93; P=0.35) for former smokers, 1.47 (95% CI: 0.77–2.80; P=0.25) for respondents who drank less than monthly, 1.26 (95% CI: 0.95–1.69; P=0.11) for respondents with normotension, 2.20 (95% CI: 0.97–5.02; P=0.06) for respondents sleeping exceeding 8 hours and 1.35 (95% CI: 0.94–1.95; P=0.11) for respondents without afternoon naps. The insignificance could be attributed to the small cohort sizes of the subgroups (Table 1). No significant interaction effects of covariates were observed in the association of RFM with LUTS/BPH (all P values for interaction >0.05), thus underscoring the robustness of our analytical model.
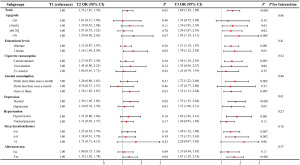
The dose-response association of RFM with prevalence of LUTS/BPH
To explore the dose-response association of RFM with the risk of LUTS/BPH, we executed RCS regression. As shown in Figure 5, the nonlinear association between RFM and LUTS/BPH was insignificant. However, the overall positive association between these variables was significant, thus further corroborating the cross-sectional association.
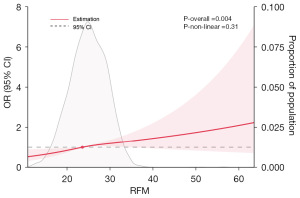
We further investigated the dose-response relationship across distinct age brackets (Figure S1) and varying levels of cigarette consumption (Figure S2). A significant linear relationship was noted in respondents aged 60 years and older with P<0.001 (Figure S1A) and the smoking subgroup with P=0.001 (Figure S2A). Conversely, the remaining groups exhibited an association that lacked statistical significance, potentially attributable to the limited incidence of LUTS/BPH within these subgroups, as indicated in Table 1.
The longitudinal relationship between RFM and incidence of LUTS/BPH
Aimed at discerning the longitudinal relationship between RFM and the incidence of LUTS/BPH, we established a series of cohorts, namely 2011–2013, 2011–2015, and 2011–2018. For the 2011–2018 cohort, ORs were 1.75 in the unadjusted model in the T3 group, with 95% CI: 1.40–2.17; P<0.001 (Figure 6). Upon adjustment for demographic and lifestyle covariates, hypertension, depression, and blood biomarkers, the OR for the T3 subgroup was 1.41 (95% CI: 1.11–1.80) in Model 4, with P=0.003. All four models corroborated the elevated risk of LUTS/BPH onset in high RFM (all P values for trend <0.01), mirroring what we found when RFM was treated as a continuous variable with all P<0.001.
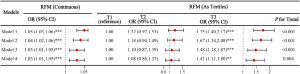
As part of our sensitivity analysis, we conducted a more detailed investigation of the longitudinal associations within the 2011–2013 and 2011–2015 cohorts. For the former one, the relationship was confirmed to be significant in both the T2 subgroup with the incidence increasing by a factor of 1.40 and the T3 subgroup with the incidence increasing by a factor of 1.85 in the unadjusted model. The relationship was also significant for the T2 subgroup with a 1.36 times augmented incidence and the T3 group with a 1.55 times augmented incidence in the fully adjusted final model (Table S3). All four models confirmed the relationship between RFM and LUTS/BPH, with all P values for trend <0.01, which aligned with continuous RFM (P<0.001). In the 2011–2015 cohort, the associations between the tertiles of RFM and LUTS/BPH were similarly substantiated by all four regression models (P values for trend <0.01), aligning with what we observed in cases where RFM was analyzed in its continuous form (P<0.001; Table S4). The T3 subgroup presented 1.63 times risk in the unadjusted model and 1.42 times risk in the final adjusted model. The aforementioned ORs were all statistically significant.
Discussion
In this national 7-year follow-up study focusing on aging males, the results revealed a positive association, both cross-sectional and longitudinal, of RFM with the prevalence and incidence of LUTS/BPH. Furthermore, a significant linear relationship was observed between RFM and prevalent LUTS/BPH within the demographic data. These findings underscore the potential for prompt detection and management strategies targeting LUTS/BPH in aging males, with the ultimate aim of enhancing their health outcomes.
In addition to the escalating global prevalence of LUTS/BPH and obesity, a burgeoning body of literature has explored their interrelationship (18,20). Despite numerous proposed mechanisms involving stem cells, cytokines, and hormones, the precise etiology of BPH remains elusive (34). Notably, obesity, particularly visceral obesity, frequently coexists with BPH (28). Numerous studies have identified some modifiable metabolic irregularities, including MetS and obesity, as key contributors to the initiation and development of BPH (16,35). Moreover, many prior studies have established a positive association of obesity with prevalent LUTS/BPH, with BMI commonly used as the obesity metric (18,20). Conversely, other studies have failed to corroborate this relationship (13,36,37). For instance, a prospective investigation encompassing males spanning the ages of 40 to 79 years from Olmsted County concluded that there is no significant correlation between certain anthropometric measures—including BMI, waist-to-hip ratio, and waist circumference—and the presence or progression of BPH (36). Another study demonstrated that, even in kidney transplant patients with LUTS, BMI was not significantly correlated with the risk of bladder outlet obstruction, indicating the questionable predictive effectiveness of BMI (38). Apart from the limitation of the relatively limited sample size, these findings indicate that the anthropometric indices traditionally employed may not be ideal predictors of LUTS/BPH. In contrast to traditional metrics, the study identified a significant association between RFM and LUTS/BPH, suggesting a clear causal direction.
As one of the novel anthropometric measures featuring simplicity, cost-effectiveness, and practicality, the RFM has promising potential for the prediction of diverse obesity-related diseases, comprising hypertension, type 2 diabetes, and arthritis, outperforming traditional indices (22,25-27). Given the positive relationship between RFM and prevalent LUTS/BPH revealed by this study, RFM could be employed for widespread recognition, enabling early management and treatment. Additionally, the result indicates that the whole-body fat percentage could be a valuable predictor of this condition, suggesting that weight reduction through methods including physical activity and reasonable drugs may serve as an adjunctive therapy. However, the feasibility of these interventions and the explicit causal relationship between weight and LUTS/BPH demand further investigation and verification.
Although the precise mechanisms are not fully understood, several plausible hypotheses can be drawn from existing research. Sex hormones, essential for the normal development of the prostate, may induce hyperplasia under the influence of environmental endocrine disruptors (34,39). Additionally, diminished levels of free testosterone may exacerbate LUTS by prohibiting nitric oxide synthase activity (16). Obesity could modify the balance and effects of sex hormones (40), contributing to hormonal imbalances which are implicated in the link between RFM and prostatic hyperplasia. Furthermore, evidence from a multicenter study involving 271 patients treated surgically for BPH suggested that lower HDL cholesterol levels and higher triglyceride levels are predominantly linked with increased intraprostatic inflammation, which can subsequently lead to BPH (41). Meanwhile, insulin resistance could directly or indirectly facilitate tissue remodeling within the prostate (42,43), whereas white adipose tissue, which is increasingly acknowledged as a significant endocrine organ, secretes numerous biologically active “adipokines” including adiponectin, which could impact insulin sensitivity through the modulation of inulin signaling and the molecules associated with the metabolism of lipid and glucose (44,45). A former study observed an association of lower serum adiponectin levels with an augmented risk of BPH (46). Furthermore, an in vitro study exploring the role of chronic inflammation in BPH pathogenesis found that BPH cells are sensitive to inflammation-related reactive oxygen species production and can be rescued by drug-induced apoptosis via reducing intracellular reactive oxygen species generation and pro-inflammatory markers (47). Therefore, we postulate that the underlying biological mechanisms may be attributed to sex steroid hormones, chronic inflammation, and insulin resistance. This hypothesis, however, still requires further substantiation through additional research.
To the best of our knowledge, this study represents the first to analyze both the cross-sectional and longitudinal associations of RFM with LUTS/BPH within a large-scale Chinese cohort. Nonetheless, several limitations must be acknowledged, warranting a cautious interpretation of the results. First, while a correlation between RFM and LUTS/BPH has been established, the observational nature of this study restricts our ability to determine causality due to potential confounding factors. Second, in light of the extensive sample size and extended follow-up duration, a streamlined diagnostic approach for LUTS/BPH was utilized, akin to methodologies used in previous studies (11,31). More objective tools such as ultrasound, and BPH treatment should be collected in future surveys, which is beneficial to a more accurate evaluation. The reliance on the self-reported approach could potentially infuse recall bias and misclassification into the statistical evaluation, which may serve as an important limitation. Third, the duration of the follow-up period was relatively brief, and the sample size was inadequate, compounded by numerous missing covariate values. Fourth, as the study participants were exclusively Chinese, the generalizability of the findings to diverse ethnic groups could be constrained due to potential ethnic variations. Consequently, further cohort studies are imperative to validate and further clarify the results.
Conclusions
Our study shows a positive relationship between RFM and BPH, with a linear association in aging males at the statistical analysis. RFM may be indicated as a novel, precise tool for widespread recognition and prompt initiation of targeted managing strategies in this demographic. Interventions to reduce excessive weight, correct insufficient physical activity, and improve early detection of clinically significant prostate enlargement, may be highly beneficial in the aging male population. Urinary obstruction treatment, including prostate surgery, when necessary, should not be delayed in these patients. Additional cohort studies are required to confirm and reinforce these findings.
Acknowledgments
The authors extend their sincere gratitude to those who shared the original dataset used in this study.
Funding: This work was supported by
Footnote
Reporting Checklist: The authors have completed the STROBE reporting checklist. Available at https://tau.amegroups.com/article/view/10.21037/tau-24-446/rc
Peer Review File: Available at https://tau.amegroups.com/article/view/10.21037/tau-24-446/prf
Conflicts of Interest: All authors have completed the ICMJE uniform disclosure form (available at https://tau.amegroups.com/article/view/10.21037/tau-24-446/coif). The authors have no conflicts of interest to declare.
Ethical Statement: The authors are accountable for all aspects of the work in ensuring that questions related to the accuracy or integrity of any part of the work are appropriately investigated and resolved. The study was conducted in accordance with the Declaration of Helsinki (as revised in 2013).
Open Access Statement: This is an Open Access article distributed in accordance with the Creative Commons Attribution-NonCommercial-NoDerivs 4.0 International License (CC BY-NC-ND 4.0), which permits the non-commercial replication and distribution of the article with the strict proviso that no changes or edits are made and the original work is properly cited (including links to both the formal publication through the relevant DOI and the license). See: https://creativecommons.org/licenses/by-nc-nd/4.0/.
References
- Platz EA, Joshu CE, Mondul AM, et al. Incidence and progression of lower urinary tract symptoms in a large prospective cohort of United States men. J Urol 2012;188:496-501. [Crossref] [PubMed]
- GBD 2019 Benign Prostatic Hyperplasia Collaborators. The global, regional, and national burden of benign prostatic hyperplasia in 204 countries and territories from 2000 to 2019: a systematic analysis for the Global Burden of Disease Study 2019. Lancet Healthy Longev 2022;3:e754-76. [Crossref] [PubMed]
- Park S, Ryu JM, Lee M. Quality of Life in Older Adults with Benign Prostatic Hyperplasia. Healthcare (Basel) 2020;8:158. [Crossref] [PubMed]
- Speakman M, Kirby R, Doyle S, et al. Burden of male lower urinary tract symptoms (LUTS) suggestive of benign prostatic hyperplasia (BPH) - focus on the UK. BJU Int 2015;115:508-19. [Crossref] [PubMed]
- Kayode OT, Owolabi AV, Kayode AAA. Biochemical and histomorphological changes in testosterone propionate-induced benign prostatic hyperplasia in male Wistar rats treated with ketogenic diet. Biomed Pharmacother 2020;132:110863. [Crossref] [PubMed]
- Russo GI, Broggi G, Cocci A, et al. Relationship between Dietary Patterns with Benign Prostatic Hyperplasia and Erectile Dysfunction: A Collaborative Review. Nutrients 2021;13:4148. [Crossref] [PubMed]
- Chughtai B, Forde JC, Thomas DD, et al. Benign prostatic hyperplasia. Nat Rev Dis Primers 2016;2:16031. [Crossref] [PubMed]
- Yang Y, Sheng J, Hu S, et al. Estrogen and G protein-coupled estrogen receptor accelerate the progression of benign prostatic hyperplasia by inducing prostatic fibrosis. Cell Death Dis 2022;13:533. [Crossref] [PubMed]
- Zlotta AR, Egawa S, Pushkar D, et al. Prevalence of inflammation and benign prostatic hyperplasia on autopsy in Asian and Caucasian men. Eur Urol 2014;66:619-22. [Crossref] [PubMed]
- Xiong Y, Zhang Y, Li X, et al. The prevalence and associated factors of lower urinary tract symptoms suggestive of benign prostatic hyperplasia in aging males. Aging Male 2020;23:1432-9. [Crossref] [PubMed]
- Xiong Y, Zhang YC, Jin T, et al. Depressive males have higher odds of lower urinary tract symptoms suggestive of benign prostatic hyperplasia: a retrospective cohort study based on propensity score matching. Asian J Androl 2021;23:633-9. [Crossref] [PubMed]
- Gu M, Liu C, Yang T, et al. High-Fat Diet Induced Gut Microbiota Alterations Associating With Ghrelin/Jak2/Stat3 Up-Regulation to Promote Benign Prostatic Hyperplasia Development. Front Cell Dev Biol 2021;9:615928. [Crossref] [PubMed]
- Kok ET, Schouten BW, Bohnen AM, et al. Risk factors for lower urinary tract symptoms suggestive of benign prostatic hyperplasia in a community based population of healthy aging men: the Krimpen Study. J Urol 2009;181:710-6. [Crossref] [PubMed]
- Peng YH, Huang CW, Chou CY, et al. Association between asthma and risk of benign prostatic hyperplasia: a retrospective population-based study. Aging Male 2020;23:599-606. [Crossref] [PubMed]
- Liu AQ, Chiu PK, Yee SC, et al. SARS-CoV-2 infection correlates with male benign prostatic hyperplasia deterioration. J Intern Med 2023;294:775-83. [Crossref] [PubMed]
- Vignozzi L, Gacci M, Maggi M. Lower urinary tract symptoms, benign prostatic hyperplasia and metabolic syndrome. Nat Rev Urol 2016;13:108-19. [Crossref] [PubMed]
- Yoo S, Oh S, Park J, et al. The impacts of metabolic syndrome and lifestyle on the prevalence of benign prostatic hyperplasia requiring treatment: historical cohort study of 130 454 men. BJU Int 2019;123:140-8. [Crossref] [PubMed]
- Penson DF, Munro HM, Signorello LB, et al. Obesity, physical activity and lower urinary tract symptoms: results from the Southern Community Cohort Study. J Urol 2011;186:2316-22. [Crossref] [PubMed]
- Parsons JK, Messer K, White M, et al. Obesity increases and physical activity decreases lower urinary tract symptom risk in older men: the Osteoporotic Fractures in Men study. Eur Urol 2011;60:1173-80. [Crossref] [PubMed]
- Wang YB, Yang L, Deng YQ, et al. Causal relationship between obesity, lifestyle factors and risk of benign prostatic hyperplasia: a univariable and multivariable Mendelian randomization study. J Transl Med 2022;20:495. [Crossref] [PubMed]
- Woolcott OO, Bergman RN. Relative fat mass (RFM) as a new estimator of whole-body fat percentage — A cross-sectional study in American adult individuals. Sci Rep 2018;8:10980. [Crossref] [PubMed]
- Kobo O, Leiba R, Avizohar O, et al. Relative fat mass is a better predictor of dyslipidemia and metabolic syndrome than body mass index. Cardiovasc Endocrinol Metab 2019;8:77-81. [Crossref] [PubMed]
- Corrêa CR, Formolo NPS, Dezanetti T, et al. Relative fat mass is a better tool to diagnose high adiposity when compared to body mass index in young male adults: A cross-section study. Clin Nutr ESPEN 2021;41:225-33. [Crossref] [PubMed]
- Suthahar N, Meems LMG, Withaar C, et al. Relative fat mass, a new index of adiposity, is strongly associated with incident heart failure: data from PREVEND. Sci Rep 2022;12:147. [Crossref] [PubMed]
- Cichosz SL, Rasmussen NH, Vestergaard P, et al. Is predicted body-composition and relative fat mass an alternative to body-mass index and waist circumference for disease risk estimation? Diabetes Metab Syndr 2022;16:102590. [Crossref] [PubMed]
- Suthahar N, Wang K, Zwartkruis VW, et al. Associations of relative fat mass, a new index of adiposity, with type-2 diabetes in the general population. Eur J Intern Med 2023;109:73-8. [Crossref] [PubMed]
- Wang D, Chen Z, Wu Y, et al. Association between two novel anthropometric measures and type 2 diabetes in a Chinese population. Diabetes Obes Metab 2024;26:3238-47. [Crossref] [PubMed]
- Huang MJ, Yang YY, Chen C, et al. Comparison of the predictive value of anthropometric indicators for the risk of benign prostatic hyperplasia in southern China. Asian J Androl 2023;25:265-70. [Crossref] [PubMed]
- Woolcott OO, Bergman RN. Defining cutoffs to diagnose obesity using the relative fat mass (RFM): Association with mortality in NHANES 1999-2014. Int J Obes (Lond) 2020;44:1301-10. [Crossref] [PubMed]
- Woolcott OO, Bergman RN. Relative Fat Mass as an estimator of whole-body fat percentage among children and adolescents: A cross-sectional study using NHANES. Sci Rep 2019;9:15279. [Crossref] [PubMed]
- Zhang W, Cao G, Sun Y, et al. Depressive symptoms in individuals diagnosed with lower urinary tract symptoms suggestive of benign prostatic hyperplasia (LUTS/BPH) in middle-aged and older Chinese individuals: Results from the China Health and Retirement Longitudinal Study. J Affect Disord 2022;296:660-6. [Crossref] [PubMed]
- Chen X, Crimmins E, Hu PP, et al. Venous Blood-Based Biomarkers in the China Health and Retirement Longitudinal Study: Rationale, Design, and Results From the 2015 Wave. Am J Epidemiol 2019;188:1871-7. [Crossref] [PubMed]
- Yang Y, Piao W, Huang K, et al. Dietary Pattern Associated with the Risk of Hyperuricemia in Chinese Elderly: Result from China Nutrition and Health Surveillance 2015-2017. Nutrients 2022;14:844. [Crossref] [PubMed]
- Devlin CM, Simms MS, Maitland NJ. Benign prostatic hyperplasia - what do we know? BJU Int 2021;127:389-99. [Crossref] [PubMed]
- Xia BW, Zhao SC, Chen ZP, et al. The underlying mechanism of metabolic syndrome on benign prostatic hyperplasia and prostate volume. Prostate 2020;80:481-90. [Crossref] [PubMed]
- Burke JP, Rhodes T, Jacobson DJ, et al. Association of anthropometric measures with the presence and progression of benign prostatic hyperplasia. Am J Epidemiol 2006;164:41-6. [Crossref] [PubMed]
- Yang HJ, Doo SW, Yang WJ, et al. Which obesity index best correlates with prostate volume, prostate-specific antigen, and lower urinary tract symptoms? Urology 2012;80:187-90. [Crossref] [PubMed]
- Righetto M, Mancini M, Modonutti D, et al. Patients with renal transplant and moderate-to-severe LUTS benefit from urodynamic evaluation and early transurethral resection of the prostate. World J Urol 2021;39:4397-404. [Crossref] [PubMed]
- Chang WH, Tsai YS, Wang JY, et al. Sex hormones and oxidative stress mediated phthalate-induced effects in prostatic enlargement. Environ Int 2019;126:184-92. [Crossref] [PubMed]
- Han YY, Forno E, Celedón JC. Sex Steroid Hormones and Asthma in a Nationwide Study of U.S. Adults. Am J Respir Crit Care Med 2020;201:158-66. [Crossref] [PubMed]
- Gacci M, Vignozzi L, Sebastianelli A, et al. Metabolic syndrome and lower urinary tract symptoms: the role of inflammation. Prostate Cancer Prostatic Dis 2013;16:101-6. [Crossref] [PubMed]
- Parsons JK, Sarma AV, McVary K, et al. Obesity and benign prostatic hyperplasia: clinical connections, emerging etiological paradigms and future directions. J Urol 2013;189:S102-6. [Crossref] [PubMed]
- Belladelli F, Montorsi F, Martini A. Metabolic syndrome, obesity and cancer risk. Curr Opin Urol 2022;32:594-7. [Crossref] [PubMed]
- Yamauchi T, Kadowaki T. Adiponectin receptor as a key player in healthy longevity and obesity-related diseases. Cell Metab 2013;17:185-96. [Crossref] [PubMed]
- Fu S, Xu H, Gu M, et al. Adiponectin deficiency contributes to the development and progression of benign prostatic hyperplasia in obesity. Sci Rep 2017;7:43771. [Crossref] [PubMed]
- Fu S, Xu H, Gu M, et al. Lack of adiponectin and adiponectin receptor 1 contributes to benign prostatic hyperplasia. Oncotarget 2017;8:88537-51. [Crossref] [PubMed]
- Saponaro M, Giacomini I, Morandin G, et al. Serenoa repens and Urtica dioica Fixed Combination: In-Vitro Validation of a Therapy for Benign Prostatic Hyperplasia (BPH). Int J Mol Sci 2020;21:9178. [Crossref] [PubMed]