Development and validation of tryptophan metabolism-related risk model and molecular subtypes for predicting postoperative biochemical recurrence in prostate cancer
Highlight box
Key findings
• The tryptophan metabolism-related risk scores and molecular subtypes could be predictors of biochemical recurrence (BCR) in prostate cancer (PCa) patients after radical prostatectomy (RP).
What is known and what is new?
• BCR following RP remains a major challenge in PCa management. Tryptophan metabolism plays a pivotal role in tumor progression and immune modulation.
• The tryptophan metabolism-related risk model and molecular subtypes effectively stratified PCa patients into low- and high-risk groups or two molecular subtypes. High-risk PCa patients and those in Cluster 1 exhibited significantly poorer biochemical recurrence-free survival (BRFS) and distinct clinicopathological features, immune infiltration profiles, and tumor microenvironment (TME) characteristics.
What is the implication, and what should change now?
• This study comprehensively analyzed the expression patterns and prognostic value of TMRGs in PCa, developing the tryptophan metabolism-related risk scores and molecular subtypes for PCa patients. The risk scores and molecular subtypes developed in this study not only effectively predicted postoperative BRFS in PCa patients but also showed strong associations with clinicopathological characteristics and the TME features. Overall, this study provides novel insights into the role of tryptophan metabolism in PCa and offers a valuable framework for stratified management and personalized treatment of PCa patients. Future studies should aim to integrate multi-omics data with clinical characteristics to construct more precise and comprehensive predictive models. Such models would enhance the generalizability and clinical applicability of these findings, offering a more robust framework for personalized PCa management.
Introduction
Prostate cancer (PCa) ranks among the most prevalent malignant tumors of the male urogenital system. According to the latest cancer statistics, it is the most frequently diagnosed malignancy in the United States and the second leading cause of cancer-related mortality (1). Over the past few decades, the widespread implementation of prostate-specific antigen (PSA) testing, along with the more recent adoption of multiparametric magnetic resonance imaging, has significantly improved the early detection of PCa (2,3). Radical prostatectomy (RP) remains a cornerstone treatment for localized PCa; however, 20–40% of patients experience biochemical recurrence (BCR) within 10 years after surgery (4,5). BCR typically reflects either local recurrence or distant metastasis of PCa (6). Previous studies have demonstrated that clinicopathological factors such as age, preoperative PSA level, postoperative Gleason score, lymph node involvement, extracapsular extension (ECE), seminal vesicle invasion (SVI), positive surgical margins, and pathological stage are significant predictors of BCR following RP (4,7-10). However, the substantial tumor heterogeneity of PCa diminishes the predictive accuracy of conventional clinicopathological parameters for BCR after RP. Therefore, identifying novel molecular biomarkers is imperative to enhance the precision of BCR prediction in PCa patients post-RP, ultimately enabling the development of personalized follow-up protocols and adjuvant treatment strategies.
During tumor development and progression, PCa cells reprogram their metabolism to meet the demands of rapid growth and proliferation. Recent studies have revealed that PCa cells promote tumor progression by remodeling various metabolic pathways, including glucose and lipid metabolism (11-15). Beyond glucose and lipid metabolism, amino acid metabolism, particularly the tryptophan metabolism pathway, also plays a pivotal role in PCa. Several studies have investigated the role and potential applications of tryptophan metabolism in PCa (16-20). However, research on the expression profiles and prognostic significance of tryptophan metabolism-related genes (TMRGs) in PCa remains limited. To date, no study has comprehensively evaluated the predictive value of TMRGs for postoperative BCR in PCa patients. In this study, we systematically examined the expression levels of TMRGs in PCa patients and assessed their prognostic significance. Additionally, we developed the tryptophan metabolism-related risk scores and molecular subtypes based on TMRGs, further exploring their associations with clinicopathological characteristics and the tumor microenvironment (TME) features in PCa. In summary, our findings underscored the critical role of tryptophan metabolism in predicting postoperative BCR in PCa, providing a theoretical basis for devising more precise and personalized follow-up and adjuvant treatment strategies. We present this article in accordance with the TRIPOD reporting checklist (available at https://tau.amegroups.com/article/view/10.21037/tau-2025-39/rc).
Methods
Acquisition and processing of PCa datasets and TMRGs
This study performed bioinformatics analyses using publicly available databases, including a training cohort and an independent validation cohort. The training cohort was derived from the Cancer Genome Atlas-Prostate Adenocarcinoma (TCGA-PRAD) dataset, comprising transcriptomic sequencing data and clinicopathological features from 421 PCa tissue samples and 52 normal prostate tissue samples (https://portal.gdc.cancer.gov). In the TCGA-PRAD dataset, all PCa patients underwent RP and were followed up under standardized protocols to record BCR status and recurrence times. To ensure the stability and reliability of the results, this study included the Memorial Sloan Kettering Cancer Center (MSKCC) dataset as an independent validation cohort (21). The MSKCC dataset, accessed through the cBioPortal database (https://www.cbioportal.org/), contains gene expression profiling data and clinical features from 131 PCa patients treated with RP (21,22). PCa patients in the validation cohort also received standardized postoperative follow-up, with clinical endpoints and times systematically documented.
The TMRGs analyzed in this study were obtained from the Molecular Signatures Database (MSigDB) and integrated from three gene sets: Kyoto Encyclopedia of Genes and Genomes (KEGG) Tryptophan Metabolism, Reactome Tryptophan Catabolism, and WP Tryptophan Metabolism. These gene sets included 40, 14, and 33 TMRGs, respectively. After integration and removal of duplicates, a total of 50 unique TMRGs were selected for further analysis. The study was conducted in accordance with the Declaration of Helsinki and its subsequent amendments.
Screening and identification of key TMRGs
Initially, differential expression analysis was conducted on PCa and normal prostate tissue samples from the TCGA-PRAD dataset to uncover the expression patterns of 50 TMRGs in PCa and normal tissues. In this study, TMRGs with a P value <0.05 were classified as differentially expressed tryptophan metabolism-related genes (DE-TMRGs). To evaluate the association between TMRGs and postoperative BCR in PCa patients, univariate Cox regression analysis was performed to determine the predictive value of the 50 TMRGs for BRFS after RP. In this study, the primary endpoint was biochemical recurrence-free survival (BRFS) following RP. Based on the univariate Cox regression analysis results, TMRGs with a P value <0.05 were selected as candidate genes with significant prognostic value. Subsequently, the least absolute shrinkage and selection operator (LASSO) regression analysis was applied to further identify key TMRGs for postoperative BRFS in PCa patients. The key TMRGs identified through screening will be used to construct a predictive model for postoperative BCR in PCa and to perform molecular subtyping in PCa.
Construction of tryptophan metabolism-related risk model based on key TMRGs
Using the key TMRGs identified through LASSO regression analysis, this study aimed to develop a risk model for predicting BRFS in PCa patients following RP. The risk model, constructed using multivariate Cox regression analysis, was applied to calculate risk scores for 421 PCa patients in the TCGA-PRAD dataset. To evaluate the performance of the risk model, the 421 patients were stratified into low- and high-risk groups based on the median risk scores. Kaplan-Meier survival curves were generated, and the log-rank test was performed to compare BRFS between the two groups, thereby assessing the prognostic utility of the risk model in predicting postoperative BCR risk in PCa patients.
Construction of tryptophan metabolism-related molecular subtypes based on key TMRGs
To further investigate the molecular characteristics of PCa patients and their relationship with postoperative BCR, this study employed an unsupervised K-means clustering approach based on key TMRGs to classify 421 PCa patients from the TCGA-PRAD dataset into molecular subtypes. First, consensus clustering analysis was performed using the expression levels of key TMRGs in these patients. The optimal number of clusters was determined by analyzing the cumulative distribution function (CDF) curve, following the principle of achieving high intra-group correlation and low inter-group correlation. Based on the clustering results, the 421 PCa patients were assigned to two molecular subtypes. To validate the accuracy and stability of the molecular subtypes, principal component analysis (PCA) was conducted to assess the clustering results, ensuring the robustness of the subtypes. Furthermore, differences in postoperative BCR between the molecular subtypes were evaluated by generating Kaplan-Meier survival curves and performing the log-rank test to compare BRFS among the subtypes. These analyses further established the prognostic significance of the molecular subtypes in distinguishing postoperative BCR risk in PCa patients.
Prognostic value analysis of the tryptophan metabolism-related risk model and molecular subtypes
To investigate prognostic factors associated with postoperative BCR, univariate and multivariate Cox regression analyses were performed using clinicopathological characteristics of 421 PCa patients from the TCGA-PRAD dataset. These factors included age, clinical T stage, pathological T stage, pathological N stage, PSA level, International Society of Urological Pathology (ISUP) grade group, surgical margin status, histological type, and tryptophan metabolism-related risk scores or molecular subtypes. The aim was to systematically evaluate the relationship between these factors and BRFS and identify independent predictors of postoperative BCR. To enhance the clinical utility of the predictive model, this study incorporated multiple clinicopathological variables, including age, pathological T stage, pathological N stage, PSA level, ISUP grade group, surgical margin status, and tryptophan metabolism-related risk scores or molecular subtypes, to construct nomograms for predicting 1-, 3-, 5-, and 10-year BRFS in PCa patients. Calibration curves and the concordance index (C-index) were employed to assess the predictive accuracy of the nomograms. Finally, decision curve analysis (DCA) was conducted to evaluate the clinical utility and patient benefit of the constructed predictive model.
Correlation analysis between tryptophan metabolism-related risk scores and molecular subtypes and clinicopathological factors
This study systematically analyzed the clinicopathological characteristics of 421 PCa patients from the TCGA-PRAD dataset, including age, clinical T stage, pathological T stage, pathological N stage, PSA level, ISUP grade group, surgical margin status, and histological type. By stratifying PCa patients into different risk score groups or molecular subtypes, the clinical relevance of tryptophan metabolism-related risk scores and molecular subtypes, as well as their associations with clinicopathological characteristics, was further investigated.
Correlation analysis between key TMRGs and TME features
This study comprehensively analyzed the relationship between key TMRGs and TME features to explore their potential mechanisms in PCa. Specifically, the “ESTIMATE” algorithm was employed to analyze the TCGA-PRAD dataset of 421 PCa patients, calculating the Stromal Score, Immune Score, ESTIMATE Score, and Tumor Purity for each patient. Subsequently, differences and correlations in these scores were evaluated between low- and high-risk PCa patients, as well as across different molecular subtypes. Furthermore, the “CIBERSORT” algorithm was applied to estimate the relative infiltration abundance of 22 immune cell types in 421 PCa patients from the TCGA-PRAD dataset. Based on immune cell infiltration profiles, this study investigated and compared immune infiltration characteristics between low- and high-risk groups, as well as among two molecular subtypes. Additionally, the correlation between tryptophan metabolism-related risk scores and immune cell infiltration abundance was assessed to further elucidate their potential roles in regulating the tumor immune microenvironment. To validate the association between key TMRGs and TME features, this study utilized the GSE176031 dataset from the Tumor Immunological Single-Cell Center (TISCH, http://tisch.comp-genomics.org/home/). The GSE176031 dataset comprises single-cell sequencing data from 11 localized PCa tissue samples obtained via transrectal prostate biopsy and RP (23). This validation provided insights into the relationship between key TMRGs and TME features at the single-cell level.
External validation of key TMRGs expression patterns, tryptophan metabolism-related risk model, and molecular subtypes
To validate the expression characteristics of key TMRGs and the robustness of the tryptophan metabolism-related risk model and molecular subtypes, this study conducted multi-level external validation analyses. First, the Human Protein Atlas (HPA) database (https://www.proteinatlas.org/) was utilized to obtain representative immunohistochemical (IHC) staining images of key TMRGs, validating their expression patterns in PCa tissues and normal prostate tissues (24). By comparing protein expression levels of TMRGs between PCa tissues and normal prostate tissues, the expression characteristics of key TMRGs in PCa were further confirmed. Furthermore, 131 PCa patients from the MSKCC dataset were employed as an independent external validation cohort to assess the stability of the tryptophan metabolism-related risk model and molecular subtypes. Risk scores for each patient in the validation cohort were calculated using the tryptophan metabolism-related risk score formula derived from the TCGA-PRAD dataset. Kaplan-Meier survival curves and log-rank tests were then applied to evaluate and validate the prognostic value of the risk scores in this independent cohort. Additionally, based on the expression levels of key TMRGs in the validation cohort, the reliability and prognostic significance of the tryptophan metabolism-related molecular subtypes in PCa patients were further established.
Statistical analysis
This study employed the Mann-Whitney U test and t-test to compare differences between the two groups. Univariate Cox regression analysis was performed to evaluate the prognostic significance of individual TMRGs. Hazard ratios (HRs) and their 95% confidence intervals (CIs) were calculated to quantitatively assess the impact of each variable on postoperative BRFS in patients. Chi-squared (χ2) tests were used to examine differences in clinicopathological characteristics between the two molecular subtypes or between low- and high-risk groups. Kaplan-Meier survival curves and Log-Rank tests were applied for survival analysis. Multivariate Cox regression analysis was conducted to identify independent predictors of postoperative BRFS in PCa patients. Spearman correlation analysis was performed to assess the relationships between variables, with correlation coefficients calculated accordingly. All statistical analyses and figure plotting were conducted using R software (version 4.2.0) and associated R packages. A P value <0.05 was considered statistically significant.
Results
Screening and identification of key TMRGs
This study initially examined the expression patterns of 50 TMRGs in PCa tissues and normal prostate tissues. The results identified 26 DE-TMRGs in PCa patients, among which 10 were upregulated and 16 were downregulated in PCa tissues (Figure 1A). To further investigate the association between TMRGs and postoperative BRFS in PCa patients, univariate Cox regression analysis was conducted to evaluate their prognostic significance. The analysis revealed that 24 TMRGs were significantly associated with postoperative BRFS. Among these, the high expression of 13 TMRGs correlated with better BRFS, whereas the high expression of 11 TMRGs was associated with poorer BRFS in PCa patients (Figure 1B). Based on differential expression and prognostic analyses, 26 DE-TMRGs and 24 prognostic-related TMRGs were identified. A Venn diagram revealed 11 overlapping TMRGs, which were selected for subsequent LASSO regression analysis (Figure 2A). LASSO regression further identified nine key TMRGs: ALDH9A1, GOT2, CAT, ALDH3A2, ALDH2, AOX1, SLC7A5, AOC1, and IL4I1 (Figure 2B,2C). Among them, the expression levels of ALDH9A1, GOT2, CAT, ALDH3A2, ALDH2, AOX1, and AOC1 were associated with a reduced probability of postoperative BCR in PCa patients, whereas the expression levels of SLC7A5 and IL4I1 were associated with an increased probability of postoperative BCR. Therefore, these nine key TMRGs not only play pivotal roles in the tryptophan metabolism pathway but also exhibit differential expression between PCa tissues and normal prostate tissues. Most importantly, they represent potential biomarkers for predicting postoperative BCR in PCa patients.
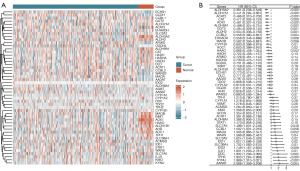
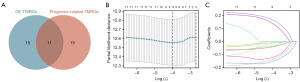
Construction of the tryptophan metabolism-related risk model
Based on the identification of nine key TMRGs, this study constructed the tryptophan metabolism-related risk model to evaluate the risk of postoperative BCR in PCa patients. Using multivariate Cox regression analysis, a risk model for PCa patients was established based on the expression levels of nine key TMRGs: ALDH9A1, GOT2, CAT, ALDH3A2, ALDH2, AOX1, SLC7A5, AOC1, and IL4I1. In this model, the risk score was calculated using weighted coefficients assigned to each gene, as shown in Figure 3A. Using this formula, risk scores were calculated for each PCa patient in the TCGA-PRAD dataset. Patients were stratified into low- and high-risk groups based on the median risk scores. The risk scores and scatter plot revealed that higher risk scores were significantly associated with an increased probability of postoperative BCR in PCa patients (Figure 3B). Additionally, a heatmap demonstrated the differential expression patterns of the nine key TMRGs between the low- and high-risk groups (Figure 3B). Survival analysis further indicated that high-risk patients had a significantly higher likelihood of BCR after RP compared to low-risk patients (HR =2.74, 95% CI: 1.79–4.17, P<0.001) (Figure 3C). These findings suggested that the tryptophan metabolism-related risk model constructed based on key TMRGs effectively predicted the risk of postoperative BCR in PCa patients and provided a potential molecular basis for clinical treatment decision-making.
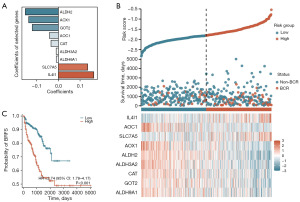
Prognostic value analysis of the tryptophan metabolism-related risk model
In this study, univariate and multivariate Cox regression analyses were performed to identify independent predictors of postoperative BRFS in PCa patients following RP. Univariate Cox regression analysis revealed that clinical T stage, pathological T stage, pathological N stage, PSA level, ISUP grade group, surgical margin status, and risk scores were significantly associated with postoperative BCR in PCa patients (Table 1). Multivariate Cox regression analysis further demonstrated that PSA level, ISUP grade group, and tryptophan metabolism-related risk scores were independent predictors of postoperative BCR (Table 1). These results indicated that, in addition to traditional clinicopathological factors, the tryptophan metabolism-related risk scores were a crucial independent predictor. Based on pathological T stage, pathological N stage, PSA level, ISUP grade group, surgical margin status, and the tryptophan metabolism-related risk scores, a prognostic nomogram was constructed to predict 1-, 3-, 5-, and 10-year BRFS in PCa patients (Figure 4A). The nomogram indicated that patients with pathological T3 or T4 stage, pathological N1 stage, higher PSA levels, higher ISUP grade group, positive surgical margins, and higher risk scores were associated with lower BRFS at 1, 3, 5, and 10 years. To validate the reliability of this nomogram, calibration curves were employed. The calibration curves demonstrated that the nomogram accurately predicted postoperative BRFS, with a C-index of 0.745, indicating moderate predictive performance (Figure 4B). Additionally, DCA analyses showed that the prediction model provided a high net benefit across 1-, 3-, 5-, and 10-year postoperative follow-up periods (Figure 4C-4F). These findings highlighted that the comprehensive prediction model, integrating clinicopathological features and the tryptophan metabolism-related risk scores, offered robust support for the postoperative management of PCa patients.
Table 1
Characteristics | Univariate analysis | Multivariate analysis | |||
---|---|---|---|---|---|
Hazard ratio (95% CI) | P | Hazard ratio (95% CI) | P | ||
Age | 1.007 (0.972–1.042) | 0.71 | – | – | |
Clinical T stage (T3 + T4 vs. T1 + T2) | 2.528 (1.478–4.322) | <0.001 | 1.609 (0.916–2.824) | 0.10 | |
Pathologic T stage (T3 + T4 vs. T2) | 4.046 (2.067–7.919) | <0.001 | 1.564 (0.721–3.394) | 0.26 | |
Pathologic N stage (N1 vs. N0) | 3.371 (2.049–5.544) | <0.001 | 1.558 (0.908–2.671) | 0.11 | |
PSA level | 1.060 (1.027–1.095) | <0.001 | 1.036 (1.001–1.073) | 0.045 | |
ISUP grade group | |||||
1+2 | Reference | Reference | |||
3 | 2.829 (1.113–7.191) | 0.03 | 2.268 (0.876–5.872) | 0.09 | |
4 | 5.008 (1.968–12.746) | <0.001 | 3.328 (1.252–8.848) | 0.02 | |
5 | 8.923 (3.984–19.984) | <0.001 | 4.027 (1.609–10.077) | 0.003 | |
Residual tumor (yes vs. no) | 2.448 (1.531–3.914) | <0.001 | 1.235 (0.717–2.129) | 0.45 | |
Histological type (acinar type vs. other subtype) | 0.415 (0.166–1.032) | 0.059 | – | – | |
Risk score | 2.718 (1.739–4.248) | <0.001 | 1.623 (1.009–2.610) | 0.046 |
BRFS, biochemical recurrence-free survival; CI, confidence interval; ISUP, International Society of Urological Pathology; PCa, prostate cancer; PSA, prostate-specific antigen.
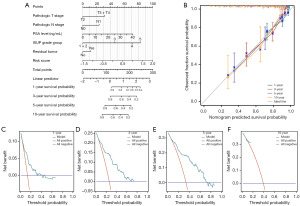
Correlation analysis of tryptophan metabolism-related risk scores and clinicopathological factors
To further investigate the relationship between risk scores and clinicopathological factors in PCa patients, a correlation analysis was conducted between tryptophan metabolism-related risk scores and various clinicopathological characteristics. The analysis revealed that PCa patients with clinical T3 or T4 stage, pathological T3 or T4 stage, pathological N1 stage, higher ISUP grade group, and positive surgical margins exhibited significantly higher risk scores (Figure 5). These findings suggested that elevated risk scores were strongly associated with adverse clinicopathological features, particularly clinical T stage, pathological T stage, pathological N stage, higher ISUP grade group, and surgical margin status. The increase in risk scores among PCa patients might reflect more aggressive tumor characteristics.
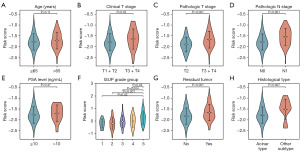
Correlation analysis of tryptophan metabolism-related risk scores and TME features
Firstly, we calculated the Stromal Score, Immune Score, ESTIMATE Score, and Tumor Purity for 421 PCa patients in the TCGA-PRAD dataset using the “ESTIMATE” algorithm. Subsequently, we analyzed the correlation between risk scores and these TME characteristic scores (Figure 6A-6H). The analysis revealed a significant positive correlation between risk scores and both the Immune Score (P<0.001) and ESTIMATE Score (P=0.01) (Figure 6B,6C). Conversely, risk scores were significantly negatively correlated with Tumor Purity (P=0.01) (Figure 6D). Further comparisons of TME characteristic scores between the high- and low-risk groups showed that high-risk patients had significantly higher Immune Scores (P=0.005) and ESTIMATE Scores (P=0.01) compared to low-risk patients (Figure 6F,6G). Additionally, Tumor Purity was significantly lower in the high-risk group (P=0.03) (Figure 6H). These findings suggested that the TME in high-risk patients was more active, characterized by a lower proportion of tumor cells and more prominent immune cell infiltration. To further investigate immune cell infiltration patterns in PCa patients, we applied the “CIBERSORT” algorithm to calculate the infiltration abundance of 22 immune cell types in 421 PCa patients from the TCGA-PRAD dataset. We also analyzed the correlation between risk scores and the infiltration abundance of these immune cell types. The results indicated that, in the high-risk group, the infiltration abundance of B cells memory (P=0.01), T cells CD4 memory activated (P=0.02), T cells regulatory (Tregs) (P<0.001), T cells gamma delta (P=0.04), and macrophages M0 (P<0.001) was significantly higher compared to the low-risk group (Figure 7A). In contrast, the infiltration abundance of plasma cells (P=0.01), T cells CD4 memory resting (P=0.008), monocytes (P=0.04), and mast cells resting (P<0.001) was significantly lower in the high-risk group (Figure 7A). Correlation analysis further revealed that risk scores positively correlated with the infiltration abundance of B cells memory (P=0.02), T cells CD4 memory activated (P=0.008), T cells follicular helper (P=0.047), Tregs (P<0.001), T cells gamma delta (P=0.01), macrophages M0 (P<0.001), and macrophages M1 (P=0.03) (Figure 7B). Conversely, risk scores negatively correlated with the infiltration abundance of plasma cells (P=0.002), T cells CD4 memory resting (P<0.001), monocytes (P<0.001), Mast cells resting (P<0.001), and Neutrophils (P=0.02) (Figure 7B).
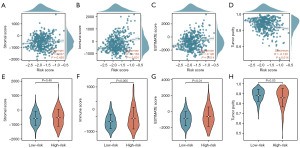
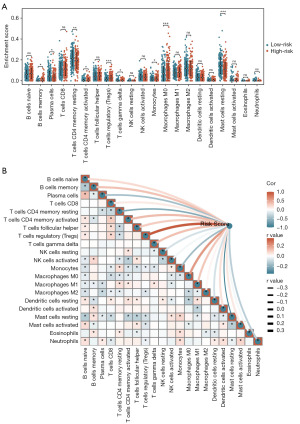
Construction of the tryptophan metabolism-related molecular subtypes based on key TMRGs
In this study, we constructed tryptophan metabolism-related molecular subtypes based on the expression levels of nine key TMRGs. Cluster analysis revealed that as the number of clusters increased from two to ten, intra-group correlation gradually decreased, while inter-group differences increased (Figure 8A,8B). Considering both intra-group correlation and inter-group differences, the optimal number of clusters was determined to be two, which exhibited the maximum intra-group correlation and minimum inter-group differences (Figure 8C). Using consensus clustering analysis, the PCa patients were classified into two molecular subtypes: Cluster 1 (n=261) and Cluster 2 (n=160). PCA analysis further validated the reliability of these molecular subtypes, demonstrating that the 421 PCa patients in the TCGA-PRAD dataset could be distinctly grouped based on the expression levels of the nine key TMRGs, thereby confirming the accuracy of the cluster analysis (Figure 8D). Additionally, a heatmap illustrated the differential expression patterns of the nine key TMRGs across the two molecular subtypes (Figure 8E). Further survival analysis revealed that PCa patients in Cluster 2 had a significantly lower probability of BCR after RP compared to those in Cluster 1 (HR =0.60, 95% CI: 0.39–0.94, P=0.03) (Figure 8F). These findings suggested that Cluster 2 represented a more favorable molecular subtype associated with better prognosis, whereas Cluster 1 corresponded to a subtype with poorer prognosis. The tryptophan metabolism-related molecular subtypes not only revealed heterogeneity in TMRG expression among different subtypes of PCa patients, but also provided a robust molecular basis for clinically predicting postoperative BCR risk.
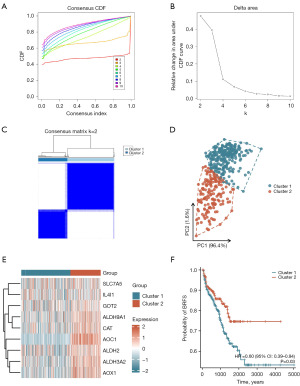
Prognostic value analysis of the tryptophan metabolism-related molecular subtypes
To further investigate the factors influencing BCR after RP in PCa patients, univariate and multivariate Cox regression analyses were conducted to identify independent predictors of postoperative BCR. Univariate Cox regression analysis revealed that clinical T stage, pathological T stage, pathological N stage, PSA level, ISUP grade group, surgical margin status, and molecular subtype were significantly associated with postoperative BRFS in PCa patients (Table 2). Multivariate Cox regression analysis further identified pathological N stage, PSA level, ISUP grade group, and molecular subtype as independent predictors of postoperative BRFS (Table 2). These findings indicated that, in addition to traditional clinicopathological factors, tryptophan metabolism-related molecular subtypes were important predictors of postoperative BCR in PCa patients. Based on pathological T stage, pathological N stage, PSA level, ISUP grade group, surgical margin status, and molecular subtypes, a prognostic nomogram was constructed to predict 1-, 3-, 5-, and 10-year BRFS in PCa patients (Figure 9A). According to the nomogram, PCa patients with pathological T3 or T4 stage, pathological N1 stage, higher PSA levels, higher ISUP grade group, positive surgical margins, and classified as Cluster 1 were predicted to have lower BRFS at 1, 3, 5, and 10 years after RP. To verify the accuracy and practicality of this prognostic nomogram, its predictive performance was assessed using calibration curves. The results demonstrated moderate predictive value for postoperative BRFS in PCa patients, with a C-index of 0.743, confirming the reliability of the nomogram (Figure 9B). Additionally, DCA analysis indicated that the predictive model provided a high net benefit at 1-, 3-, 5-, and 10-year postoperative follow-up time points (Figure 9C-9F). These findings suggested that the tryptophan metabolism-related molecular subtypes constructed in this study offered effective support for clinical decision-making and exhibited strong prognostic predictive value.
Table 2
Characteristics | Univariate analysis | Multivariate analysis | |||
---|---|---|---|---|---|
Hazard ratio (95% CI) | P | Hazard ratio (95% CI) | P | ||
Age | 1.007 (0.972–1.042) | 0.71 | – | – | |
Clinical T stage (T3 + T4 vs. T1 + T2) | 2.528 (1.478–4.322) | <0.001 | 1.572 (0.893–2.768) | 0.12 | |
Pathologic T stage (T3 + T4 vs. T2) | 4.046 (2.067–7.919) | <0.001 | 1.694 (0.787–3.649) | 0.18 | |
Pathologic N stage (N1 vs. N0) | 3.371 (2.049–5.544) | <0.001 | 1.787 (1.038–3.079) | 0.04 | |
PSA level | 1.060 (1.027–1.095) | <0.001 | 1.036 (1.000–1.074) | 0.048 | |
ISUP grade group | |||||
1+2 | Reference | Reference | |||
3 | 2.829 (1.113–7.191) | 0.03 | 2.490 (0.965–6.420) | 0.059 | |
4 | 5.008 (1.968–12.746) | <0.001 | 3.292 (1.245–8.704) | 0.02 | |
5 | 8.923 (3.984–19.984) | <0.001 | 3.880 (1.568–9.603) | 0.003 | |
Residual tumor (yes vs. no) | 2.448 (1.531–3.914) | <0.001 | 1.188 (0.688–2.051) | 0.54 | |
Histological type (acinar type vs. other subtype) | 0.415 (0.166–1.032) | 0.059 | – | – | |
Molecular subtype (cluster 2 vs. 1) | 0.565 (0.330–0.965) | 0.04 | 0.495 (0.250–0.980) | 0.04 |
BRFS, biochemical recurrence-free survival; CI, confidence interval; ISUP, International Society of Urological Pathology; PCa, prostate cancer; PSA, prostate-specific antigen.
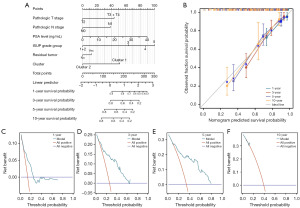
Correlation analysis of tryptophan metabolism-related molecular subtypes and clinicopathological factors
To further investigate the relationship between tryptophan metabolism-related molecular subtypes and clinicopathological factors in PCa patients, the correlation analyses were conducted between the molecular subtypes and various clinicopathological characteristics. The analysis revealed that patients classified as Cluster 2 had a significantly lower proportion of low ISUP grade group and positive surgical margins compared to those in Cluster 1 (Figure 10).
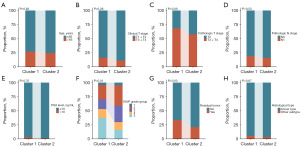
Correlation analysis of tryptophan metabolism-related molecular subtypes and TME features
Using the “CIBERSORT” algorithm, this study calculated the infiltration abundance of 22 immune cell types in 421 PCa patients from the TCGA-PRAD dataset. Additionally, the relationships between tryptophan metabolism-related molecular subtypes and immune cell infiltration abundance in PCa patients were analyzed. The results revealed significant differences in the TME features between patients in Clusters 1 and 2 (Figure 11A). Specifically, Cluster 1 patients exhibited significantly higher infiltration abundance of Tregs (P=0.04) and macrophages M2 (P<0.001) compared to Cluster 2 patients (Figure 11B). Conversely, plasma cells (P=0.008), macrophages M1 (P=0.04), dendritic cells resting (P=0.008), and neutrophils (P=0.04) demonstrated significantly higher infiltration abundance in Cluster 2 patients than in Cluster 1 patients (Figure 11B).
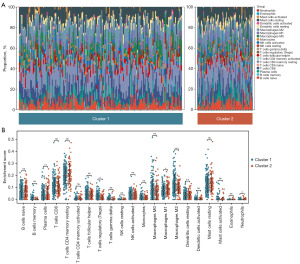
External validation of the expression levels of key TMRGs, immune characteristics, tryptophan metabolism-related risk scores, and molecular subtypes
First, the HPA database was utilized to obtain representative IHC images of nine key TMRGs, aiming to validate their expression patterns in PCa tissues compared to normal prostate tissues. The representative IHC images revealed that among the nine key TMRGs, ALDH9A1, GOT2, CAT, ALDH3A2, ALDH2, AOX1, SLC7A5, and AOC1 exhibited high expression in normal prostate tissues (Figure 12), whereas IL4I1 showed high expression in PCa tissues (Figure 12).
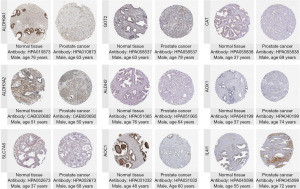
Subsequently, the TISCH database was employed to validate the correlation between key TMRGs and TME characteristics at the single-cell level (Figure 13). The analysis demonstrated that in PCa tissues, key TMRGs were primarily distributed among epithelial cells, fibroblasts, monocytes/macrophages (Mono/Macro), CD8+ T cells, and malignant cells (Figure 13). Overall, the key TMRGs were associated with the infiltration abundance of various immune cells in PCa tissues, suggesting that these genes might mediate the malignant phenotype of tumors by modulating the TME.
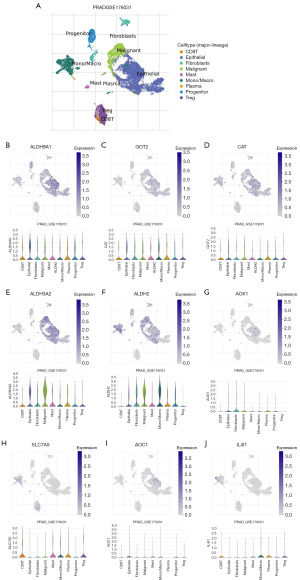
Additionally, to further validate the stability of the tryptophan metabolism-related risk scores and molecular subtypes established in this study, an independent external validation cohort of 131 PCa patients from the MSKCC dataset was utilized. Using the risk score formula derived from the TCGA-PRAD dataset, risk scores were calculated for each PCa patient in the MSKCC cohort (Figure 14A). Survival analysis revealed that high-risk PCa patients had a significantly increased probability of BCR following RP compared to low-risk patients (HR =3.20, 95% CI: 1.35–7.58, P=0.008) (Figure 14B). This result indicated that the tryptophan metabolism-related risk scores constructed in this study retained robust predictive performance in the external validation cohort, effectively predicting the risk of BCR in PCa patients after RP.
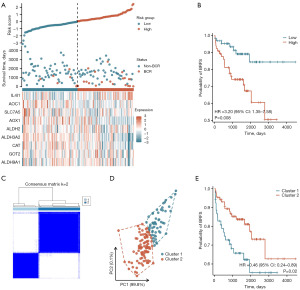
Finally, based on the expression levels of key TMRGs in the MSKCC dataset, the tryptophan metabolism-related molecular subtypes and their prognostic value were further validated. Clustering analysis showed that PCa patients in the MSKCC dataset could also be classified into two molecular subtypes, Cluster 1 (n=52) and Cluster 2 (n=79), based on the expression levels of the key TMRGs (Figure 14C). PCA analysis further confirmed the significant distinction between the two molecular subtypes, validating the accuracy of the clustering and molecular subtypes (Figure 14D). Survival analysis demonstrated that PCa patients in Cluster 2 had a significantly lower probability of BCR following RP compared to those in Cluster 1 (HR =0.46, 95% CI: 0.24–0.89, P=0.02), further corroborating the stability and prognostic value of the tryptophan metabolism-related molecular subtypes in the external validation cohort (Figure 14E).
Discussion
RP remains one of the standard treatment options for localized PCa (4). However, more than 40% of intermediate- or high-risk PCa patients experience BCR following RP (5). Although BCR does not immediately result in clinical symptoms, it often signifies local tumor recurrence or micrometastases. Early identification of BCR provides opportunities for timely clinical intervention, potentially delaying disease progression and improving prognosis (4). Currently, BCR prediction after RP primarily relies on clinicopathological features, including serum PSA levels, Gleason score, clinical stage, surgical margin status, and lymph node metastasis. Various predictive models have been developed based on these features to assess the risk of BCR post-surgery. The D’Amico risk stratification system, a widely used clinical tool, categorizes PCa patients into low-, intermediate-, and high-risk groups based on clinical stage, Gleason score, and PSA levels (4). This model has been extensively validated in clinical practice and serves as a crucial guide for evaluating postoperative BCR risk. The Cancer of the Prostate Risk Assessment (CAPRA) scoring system incorporates preoperative PSA levels, Gleason score, clinical T stage, percent positive biopsies, and patient age to construct a BCR risk prediction model (7,8). Similarly, the MSKCC developed a predictive model that integrates preoperative PSA levels, Gleason score, surgical margins, ECE, SVI, and lymph node invasion to create a nomogram predicting the 10-year risk of BCR (9,10). These three models have undergone external validation and are widely utilized in clinical practice, offering valuable reference points for clinical decision-making (25). However, prediction models based on traditional clinicopathological features have inherent limitations. First, these indicators fail to fully capture the molecular biological characteristics of PCa, limiting the precision of postoperative recurrence risk assessments. Second, current clinical prediction models do not incorporate molecular mechanisms of PCa, such as cancer-related gene expression profiles and dynamic changes in the TME. Additionally, their clinical utility is restricted, particularly in patient populations with high tumor heterogeneity, where individualized risk stratification remains challenging. In summary, while existing prediction models provide critical guidance for assessing BCR risk in PCa patients, their predictive performance requires improvement. Therefore, it is essential to develop a new generation of personalized and precise predictive models that integrate tumor molecular characteristics, TME features, and gene expression patterns to enhance the accuracy of prognosis assessment.
The progression and development of PCa are closely associated with significant metabolic reprogramming and alterations in metabolic pathways. PCa cells actively remodel multiple metabolic pathways, including glucose metabolism, lipid metabolism, and amino acid metabolism, to adapt to the pressures of the TME (11-13). Among these, amino acid metabolism, particularly the tryptophan metabolism pathway, plays a pivotal regulatory role in PCa cells. In the tryptophan metabolism pathway, tryptophan 2,3-dioxygenase (TDO) and indoleamine 2,3-dioxygenase (IDO) catalyze the conversion of tryptophan into N-formylkynurenine (NFK), which is subsequently processed by arylformamidase (AFMID) to produce kynurenine. Kynurenine is further metabolized into kynurenic acid (KYNA) by kynurenine aminotransferase (KAT) (17,26,27). Therefore, tryptophan metabolism involves three primary pathways: the kynurenine pathway, the 5-hydroxytryptamine pathway, and the indole pathway (28,29). Furthermore, tryptophan, an essential amino acid required for protein synthesis, and its metabolites, such as kynurenine, play crucial roles in regulating biological processes, including tumor immune evasion, immunosuppression, and tumor cell proliferation (28,29). Given its importance, increasing attention has been directed toward understanding the role and potential applications of tryptophan metabolism in PCa. McDunn et al. conducted a comprehensive analysis of 331 PCa tissues and 178 normal prostate tissues using gas chromatography-mass spectrometry (GC-MS) and ultra-high performance liquid chromatography-tandem mass spectrometry (UHPLC-MS/MS) to identify tissue metabolite profiles (16). The study revealed significant metabolic differences between PCa and normal prostate tissues, with 28 metabolites exhibiting a strong positive correlation with Gleason score. Notably, the tryptophan metabolism product kynurenine showed a high positive correlation with Gleason score. Based on the expression profiles of 55 metabolites, the study established a subtyping strategy for PCa patients, presenting a novel approach for metabolomics stratification studies (16). Gkotsos et al. utilized UPLC-MS/MS to analyze kynurenic acid levels in urine samples from 32 PCa patients prior to RP, 101 patients undergoing prostate biopsy (before and after prostate massage), and 15 healthy controls (17). The study revealed that urinary kynurenic acid levels were significantly lower in PCa patients compared to controls. Notably, the diagnostic efficacy of kynurenic acid was enhanced in urine samples collected after prostate massage, highlighting its potential as a diagnostic biomarker for PCa, particularly in post-massage urine samples (17). In addition to tissue and urine metabolomics studies, researchers have explored the diagnostic value of tryptophan metabolites in plasma samples. Nitusca et al. conducted untargeted metabolomic analysis on plasma samples from 48 PCa patients and 23 healthy controls using ultra-high performance liquid chromatography coupled with electrospray ionization quadrupole time-of-flight mass spectrometry (UHPLC-QTOF-[ESI+]-MS) (18). Diagnostic analysis demonstrated that L-tryptophan exhibited high diagnostic value, with an area under the curve of 0.9692. Targeted metabolomic analysis further revealed significantly lower plasma L-tryptophan concentrations in PCa patients compared to healthy controls, with L-tryptophan retaining the highest diagnostic value (18). These findings underscored the critical role of tryptophan metabolism in PCa diagnosis, providing new perspectives for the identification and validation of PCa biomarkers. Increasing attention has also been directed toward the predictive value of metabolites in postoperative outcomes for PCa patients. Clendinen et al. analyzed metabolite abundance in preoperative serum samples from 40 PCa patients with postoperative recurrence and 40 without recurrence (19). Significant alterations were identified in several metabolic pathways, including tryptophan catabolism. Using machine learning, a combined predictive model was constructed, incorporating 20 metabolites to distinguish between recurrent and non-recurrent PCa patients. The model achieved an accuracy of 92.6%, sensitivity of 94.4%, and specificity of 91.9%, providing a foundation for utilizing metabolites in predicting postoperative BCR in PCa patients (19). Additionally, studies have assessed the predictive value of tryptophan metabolites in BCR among PCa patients. Pichler et al. analyzed serum levels of tryptophan and kynurenine in 100 PCa patients both before RP and at the time of BCR (20). Survival analysis revealed that higher serum kynurenine levels were significantly associated with an increased risk of postoperative BCR (P=0.009). Moreover, univariate Cox regression analysis indicated that elevated serum kynurenine levels correlated with a higher risk of cancer-specific mortality in PCa patients (HR =2.93, 95% CI: 1.26–6.79, P=0.01). However, multivariate Cox regression analysis demonstrated that kynurenine was not an independent prognostic factor for cancer-specific survival (HR =3.466, 95% CI: 0.746–16.106, P=0.11) (20). Most of these studies focused on tryptophan metabolism in patient samples. In addition, research has explored the regulatory effects of tryptophan metabolites on PCa cells. Cellular metabolomics studies revealed significant differences in metabolic profiles between PCa cell lines (PC-3 and LNCaP) and normal prostate cell lines (RWPE-1) (26). Tryptophan metabolites were found to inhibit PCa cell proliferation in a dose-dependent manner. Further investigations demonstrated that tryptamine suppressed PC-3 cell migration and induced apoptosis through a caspase-3-dependent pathway. In vivo experiments further validated these findings, showing that intratumoral administration of tryptamine inhibited tumor growth in PC-3 xenografts in nude mice. These results highlight the potential of tryptamine as a candidate for local antitumor therapy in PCa treatment (26).
Despite this progress, studies on the expression patterns and prognostic value of TMRGs in PCa patients remain limited. To date, no comprehensive investigation has been conducted to evaluate the predictive value of TMRGs for postoperative BCR in PCa patients. In this study, we first analyzed the expression patterns of TMRGs in PCa patients using the TCGA-PRAD dataset. Among 50 TMRGs, 26 were identified as DE-TMRGs, including 10 upregulated and 16 downregulated genes. Survival analysis further revealed that 24 TMRGs were significantly associated with BRFS in PCa patients, identifying them as prognosis-related TMRGs. By overlapping the DE-TMRGs with the prognosis-related TMRGs, we identified 11 TMRGs that were both differentially expressed and closely associated with BRFS in PCa patients. Subsequently, LASSO regression analysis further refined the selection to nine key TMRGs: ALDH9A1, GOT2, CAT, ALDH3A2, ALDH2, AOX1, SLC7A5, AOC1, and IL4I1. These nine key TMRGs play critical roles in the tryptophan metabolism pathway and exhibit significant differential expression between PCa tissues and normal prostate tissues. More importantly, these nine key TMRGs hold strong potential as molecular biomarkers for predicting postoperative BCR in PCa patients. Aldehyde dehydrogenase 9 family member A1 (ALDH9A1) encodes a protein belonging to the aldehyde dehydrogenase superfamily, which primarily catalyzes the oxidation of aldehydes into their corresponding carboxylic acids (30). In clear cell renal cell carcinoma, ALDH9A1 functions as a tumor suppressor gene. Its loss promotes tumor proliferation, invasion, migration, and lipid accumulation, while facilitating tumor progression through activation of the AKT-mTOR signaling pathway (31). Glutamic-oxaloacetic transaminase 2 (GOT2) encodes a key protein in the tryptophan metabolism pathway. GOT2 catalyzes the conversion of L-kynurenine to kynurenic acid via its transaminase activity and is an essential component of the malate-aspartate shuttle (32). In PCa, GOT2 not only regulates cancer cell proliferation but also exhibits anti-cancer effects through succinylation, which inhibits aspartate synthesis and nucleotide production, potentially suppressing PCa progression (33). CAT (Catalase) encodes a vital antioxidant enzyme that catalyzes the decomposition of hydrogen peroxide, produced by peroxisomal oxidases, into water and oxygen (34). In PCa, particularly in metastatic castration-resistant PCa, catalase has gained attention as a novel therapeutic target. Multiple studies suggest that modulating CAT activity may provide therapeutic benefits in PCa treatment (34,35). Aldehyde dehydrogenase 3 family member A2 (ALDH3A2) encodes a protein in the aldehyde dehydrogenase superfamily that primarily catalyzes the oxidation of medium- and long-chain fatty aldehydes into fatty acids. In PCa, ALDH3A2 expression is significantly upregulated in androgen-independent PCa cell lines compared to androgen-dependent ones (36). Furthermore, the expression of ALDH3A2, along with ODF2, QSOX2, and microRNA-503-5p, has been identified as a predictor of recurrence in TMPRSS2-ERG-positive PCa (37). Aldehyde dehydrogenase 2 family member (ALDH2) also encodes a protein within the aldehyde dehydrogenase superfamily, which eliminates endogenous aldehydes and facilitates tryptophan metabolism (38,39). Similar to ALDH3A2, ALDH2 expression is markedly upregulated in androgen-independent PCa cell lines compared to androgen-dependent ones (36). Moreover, ALDH2 is closely associated with various urological malignancies, including PCa, and is a promising therapeutic target for malignant tumors (40,41). Aldehyde oxidase 1 (AOX1) encodes a protein that oxidizes a variety of endogenous and exogenous aldehydes. The study suggested that loss of AOX1 leads to the accumulation of tryptophan metabolites, such as kynurenine and nicotinamide adenine dinucleotide phosphate (NADP) (42). In bladder cancer, AOX1 is epigenetically silenced by EZH2-mediated methyltransferase activity, and knockdown of AOX1 in normal bladder epithelial cells reactivates the tryptophan-kynurenine pathway, promoting cellular invasion (42). In PCa, previous studies have shown that AOX1 expression is reduced, and its methylation levels are elevated in PCa tissues and cells. Furthermore, AOX1 knockdown enhances the migration and invasion capabilities of PCa cells (43). Solute carrier family 7 member 5 (SLC7A5) encodes a protein that forms a heterodimer with SLC3A2 to mediate amino acid transport across the cell membrane, directly participating in the tryptophan metabolism pathway (44). SLC7A5 is aberrantly expressed in various malignancies and is closely associated with patient prognosis (44,45). In PCa, SLC7A5 is highly expressed in drug-resistant PCa cell lines. Knockdown of SLC7A5 in LNCaP and C4-2 cells significantly inhibits cell proliferation, migration, and invasion (46). Amine oxidase copper containing 1 (AOC1) encodes a protein that catalyzes the oxidative deamination of primary amines into corresponding aldehydes, producing hydrogen peroxide and ammonia as byproducts (47). AOC1 is generally considered an oncogene in various malignancies; however, in PCa, AOC1 is downregulated. Studies have shown that reduced AOC1 expression in PCa tissues correlates positively with tumor size, lymph node metastasis, and Gleason score. Moreover, high AOC1 expression is closely associated with decreased proliferation and migration of PCa cells both in vitro and in vivo (48). Interleukin 4 induced 1 (IL4I1) encodes a secreted L-amino acid oxidase primarily involved in immune regulation (49,50). IL4I1 influences tumor progression predominantly by modulating tryptophan catabolism (49). Research has demonstrated that IL4I1 generates indole metabolites and kynurenic acid, which activate the aryl hydrocarbon receptor (AHR). AHR activation enhances tumor malignancy and suppresses anti-tumor immune responses. Consequently, blocking IL4I1 has been proposed as a novel strategy for cancer therapy (50). In addition, IL4I1 exerts immunoregulatory effects by inhibiting the proliferation of effector T lymphocytes and promoting the development of regulatory T cells (51,52). These findings highlight IL4I1 as a crucial factor in tumor immune evasion and a potential therapeutic target for PCa and other malignancies. In summary, the tryptophan metabolic pathway and its metabolite kynurenine play a crucial role in cancer progression and metastasis through immune modulation. Due to the immunosuppressive effects of tryptophan-related enzymes and kynurenine metabolites, the tryptophan pathway represents a promising therapeutic target for cancer treatment. Ongoing clinical trials are investigating various drugs and combination therapies targeting the kynurenine pathway, aiming to alter the prognosis of cancer patients (28,29).
Based on the expression levels of key TMRGs, this study developed a predictive model capable of stratifying PCa patients into low- and high-risk groups to assess the risk of BCR. High-risk PCa patients demonstrated a significantly greater likelihood of BCR after RP compared to low-risk patients. Furthermore, multivariate Cox regression analysis identified the tryptophan metabolism-related risk score as an independent predictor of postoperative BCR in PCa patients. To enhance predictive accuracy, this study constructed a nomogram incorporating tryptophan metabolism-related risk scores to estimate postoperative BRFS in PCa patients. Compared with the existing prognostic nomograms based on clinicopathological features of PCa patients, the prediction model constructed in this study also included the tryptophan metabolism-related risk scores. Therefore, the model constructed in this study is more inclined to complement and optimize the existing prognostic models. Furthermore, previous studies have demonstrated that Decipher genomic score and multiparametric magnetic resonance imaging could be used to assess the risk of postoperative BCR in patients with PCa (53,54). Therefore, compared with existing genomics-based platforms and imaging techniques, the risk score in this study is based on the TMRGs, providing a unique metabolic perspective to assess tumor progression. However, Decipher genomic score and radiographic information were not included in our dataset. Future studies could combine clinicopathological, genetic, metabolic, and radiographic features to further optimize the BCR prediction model. Analysis of the correlation between clinicopathological factors and risk scores revealed that PCa patients with higher risk scores were more likely to present with advanced clinical T stage, pathological T stage, pathological N1 stage, higher ISUP grade group, and positive surgical margins. These findings indicated a significant association between higher risk scores and adverse clinicopathological features, partially explaining why patients with higher risk scores exhibit poorer postoperative BRFS.
Subsequent analysis of TME features revealed a significant positive correlation between risk scores and both the Immune Score and ESTIMATE Score, alongside a significant negative correlation with Tumor Purity. These findings suggested that high-risk patients possessed a more active TME, characterized by a lower proportion of tumor cells and potentially more pronounced immune cell infiltration. To further investigate TME features, this study analyzed the relationship between risk scores and immune cell infiltration abundance in PCa patients. Immune infiltration analyses demonstrated that Tregs infiltration was significantly higher in high-risk patients compared to low-risk patients. Furthermore, risk scores showed a positive correlation with Tregs infiltration abundance. The TME is a complex and dynamic system where interactions between tumor cells, the extracellular matrix, vascular networks, and immune cells collectively influence tumor growth, invasion, and metastasis (55,56). Tregs play a pivotal and multifaceted role in malignancies, primarily suppressing antitumor immune responses and promoting TME formation through various immunosuppressive mechanisms. Within the TME, Tregs inhibit the activation and function of effector T cells by secreting immunosuppressive factors and expressing inhibitory molecules (57,58). Additionally, Tregs express high levels of CD25, enabling them to competitively consume IL-2, thereby limiting the proliferation and functionality of effector T cells (59). These mechanisms collectively weaken tumor immune surveillance, facilitating immune evasion by tumors. Consequently, increased Tregs infiltration is closely associated with poor prognosis in various cancers (58,60). The significantly higher postoperative BCR risk observed in high-risk PCa patients might, in part, be attributed to elevated Tregs infiltration in this group. Collectively, this study not only constructed the tryptophan metabolism-related risk scores but also developed a nomogram incorporating the risk scores to predict postoperative BRFS in PCa patients. More importantly, the study explored the correlations between risk scores, clinicopathological characteristics, and TME features in PCa patients. These findings provided a theoretical foundation for developing individualized and precise BCR prediction models for PCa patients by integrating tumor molecular characteristics, the tumor immune microenvironment, and gene expression profiles.
PCa is a highly heterogeneous malignancy, with heterogeneity manifesting at multiple levels, particularly in molecular characteristics. Current molecular subtypes of PCa primarily rely on genomic and epigenetic features, and several studies have explored the feasibility of molecular subtyping in localized PCa. The TCGA Research Network conducted a multi-omics analysis of 333 primary PCa samples, incorporating whole-exome sequencing, transcriptomics, and epigenetic profiling (61). Through comprehensive and systematic analyses, the study identified seven major molecular subtypes of PCa, each characterized by distinct genomic features and potential biological mechanisms. Based on different oncogenic drivers, 74% of PCa cases were classified into one of the following seven molecular subtypes: ERG fusion, ETV1 fusion, ETV4 fusion, FLI1 fusion, SPOP mutation, FOXA1 mutation, and IDH1 mutation. However, 26% of PCa cases could not be accurately categorized into these subtypes. Despite its comprehensiveness, this molecular subtyping approach did not establish a correlation between molecular subtypes and patient prognosis, thereby limiting its clinical applicability. In another study, Zhao et al. applied the PAM50 assay to analyze 3,782 PCa samples to establish molecular subtypes (62). The results demonstrated that PAM50 could classify PCa samples into three molecular subtypes: Luminal A, Luminal B, and Basal. Survival analysis revealed that patients with the Luminal B subtype had poorer prognosis compared to those with the Luminal A or Basal subtypes. Regarding the risk of postoperative BCR, the 10-year BCR-free rate was 29% for Luminal B patients, compared to 41% for Luminal A patients and 39% for Basal patients. Multivariate analysis, adjusting for clinicopathological features such as age, PSA levels, Gleason score, surgical margin status, ECE, SVI, and lymph node involvement, demonstrated that BRFS was significantly better for basal and luminal A subtypes compared to luminal B. Further analysis revealed that only luminal B patients exhibited a significant response to postoperative androgen deprivation therapy (ADT). Thus, this study not only established molecular subtypes for PCa patients but also correlated these subtypes with patient prognosis, providing a foundation for stratified treatment strategies. By integrating molecular subtypes with clinical outcomes, this approach offered a framework for tailoring therapeutic interventions based on molecular characteristics. Given the critical role of tryptophan metabolism in malignancies, we constructed tryptophan metabolism-related molecular subtypes for PCa patients based on key TMRGs. Through clustering analysis, PCa patients were classified into two molecular subtypes. Unlike traditional molecular subtypes, such as basal or luminal subtypes, our classification was driven by TMRGs, which introduced a unique metabolic perspective for molecular subtypes. Survival analysis revealed that PCa patients in Cluster 2 had a significantly lower risk of postoperative BCR after RP compared to those in Cluster 1. Furthermore, multivariate Cox regression analysis demonstrated that the tryptophan metabolism-related molecular subtype was an independent predictor of postoperative BCR in PCa patients. Building on these findings, this study developed a nomogram incorporating tryptophan metabolism-related molecular subtypes to more accurately predict postoperative BRFS in PCa patients. Clinicopathological correlation analysis indicated that Cluster 2 patients had a significantly lower proportion of cases with high ISUP grade group and positive surgical margins compared to Cluster 1. These findings suggested a significant association between tryptophan metabolism-related molecular subtypes and the clinicopathological characteristics of PCa patients. Additionally, they partially explained the improved postoperative BRFS observed in Cluster 2, which might be attributed to the co-occurrence of favorable clinicopathological features within this molecular subtype.
Subsequent analysis of TME characteristics revealed significant differences between PCa patients in Clusters 1 and 2. In Cluster 1, the infiltration abundance of Tregs and M2 macrophages was significantly higher compared to Cluster 2. As discussed earlier, Tregs in the TME primarily promote tumor immune evasion and shape an immunosuppressive microenvironment, driving tumor initiation, progression, and metastasis. In addition, M2 macrophages play a critical role in the TME (63). These cells suppress effector T cells by secreting immunosuppressive factors such as interleukin-10 (IL-10) and promote angiogenesis by releasing pro-angiogenic factors like vascular endothelial growth factor (VEGF) (64). Moreover, M2 macrophages facilitate the overexpression of programmed death-ligand 1 (PD-L1), inhibiting the antitumor function of cytotoxic T cells and promoting tumor cell survival, proliferation, and drug resistance (64). The enrichment of M2 macrophages is closely associated with poor prognosis in various malignancies, making them a key target in antitumor immunotherapy due to their pivotal role in tumor immune evasion (65,66). The findings of this study indicated that the significantly higher risk of postoperative BCR in Cluster 1 patients compared to cluster 2 was likely associated with the elevated infiltration of Tregs and M2 macrophages in Cluster 1. These immunosuppressive cells weaken the antitumor capability of effector T cells within the TME, contributing to the unfavorable prognosis of Cluster 1 patients. In summary, this study not only developed the tryptophan metabolism-related risk scores but also established molecular subtypes based on tryptophan metabolism. This molecular subtyping approach provided a valuable reference for stratified management and precision treatment of PCa patients. Furthermore, the findings emphasized the necessity and feasibility of integrating clinicopathological characteristics and TME features to optimize existing PCa molecular subtyping methods.
To further validate the reliability of the study results, external validation was conducted from three perspectives. First, the HPA database was utilized to examine the expression patterns of key TMRGs in PCa tissues compared to normal prostate tissues. By analyzing the protein expression levels of key TMRGs in PCa and normal tissues, the study reinforced the expression characteristics of these genes in PCa. Second, the TISCH database was employed to investigate the relationship between key TMRGs and immune cell infiltration abundance in PCa tissues at the single-cell level. This analysis demonstrated that key TMRGs were associated with the infiltration of various immune cells in PCa tissues, suggesting that these genes might mediate tumor malignant phenotypes by modulating the TME. Finally, an independent validation cohort of 131 PCa patients from the MSKCC dataset was used to validate the stability and prognostic value of the tryptophan metabolism-related risk scores and molecular subtypes. The results confirmed the robustness and predictive utility of the risk scores and molecular subtypes in this external cohort. In summary, this study employed a comprehensive external validation strategy across multiple databases and dimensions, including protein-level validation, single-cell sequencing analysis, and an independent validation cohort. This multi-dimensional approach not only enhanced the reliability of the findings but also provided a solid scientific foundation for the clinical translation of the research outcomes.
Although this study successfully developed tryptophan metabolism-related risk scores and molecular subtypes, evaluating their clinical value for the first time, several limitations should be acknowledged. First, this analysis primarily relied on public databases and datasets, which might introduce selection bias. To ensure the reliability and robustness of the findings, larger-scale prospective studies are necessary. Moreover, this study mainly focused on localized PCa treated with RP and mainly followed patients untill BCR. This restricted patient cohort limited the broader applicability of the model to patients with more advanced disease or those receiving other treatments. Second, while key TMRGs were identified using LASSO regression, alternative machine learning approaches, such as random forests and support vector machines, could also be employed to validate the roles and significance of these genes. Comparing multiple screening methods would help to consistently confirm the role and value of TMRGs in PCa. Additionally, TME features analyses revealed higher infiltration of Tregs in high-risk and Cluster 1 PCa patients, underscoring the need for further basic research to elucidate the relationship between tryptophan metabolism, Tregs infiltration, and their roles in immune regulation. Finally, given the significant heterogeneity of PCa, relying solely on molecular or clinicopathological features may not accurately predict prognosis. Future studies should aim to integrate multi-omics data with clinical characteristics to construct more precise and comprehensive predictive models. Such models would enhance the generalizability and clinical applicability of these findings, offering a more robust framework for personalized PCa management.
Conclusions
In conclusion, this study comprehensively analyzed the expression patterns and prognostic value of TMRGs in PCa, developing the tryptophan metabolism-related risk scores and molecular subtypes for PCa patients. The risk scores and molecular subtypes developed in this study not only effectively predicted postoperative BRFS in PCa patients but also showed strong associations with clinicopathological characteristics and the TME features. Overall, this study provides novel insights into the role of tryptophan metabolism in PCa and offers a valuable framework for stratified management and personalized treatment of PCa patients.
Acknowledgments
None.
Footnote
Reporting Checklist: The authors have completed the TRIPOD reporting checklist. Available at https://tau.amegroups.com/article/view/10.21037/tau-2025-39/rc
Peer Review File: Available at https://tau.amegroups.com/article/view/10.21037/tau-2025-39/prf
Funding: This work was supported by
Conflicts of Interest: All authors have completed the ICMJE uniform disclosure form (available at https://tau.amegroups.com/article/view/10.21037/tau-2025-39/coif). The authors have no conflicts of interest to declare.
Ethical Statement: The authors are accountable for all aspects of the work in ensuring that questions related to the accuracy or integrity of any part of the work are appropriately investigated and resolved. The study was conducted in accordance with the Declaration of Helsinki and its subsequent amendments.
Open Access Statement: This is an Open Access article distributed in accordance with the Creative Commons Attribution-NonCommercial-NoDerivs 4.0 International License (CC BY-NC-ND 4.0), which permits the non-commercial replication and distribution of the article with the strict proviso that no changes or edits are made and the original work is properly cited (including links to both the formal publication through the relevant DOI and the license). See: https://creativecommons.org/licenses/by-nc-nd/4.0/.
References
- Siegel RL, Giaquinto AN, Jemal A. Cancer statistics, 2024. CA Cancer J Clin 2024;74:12-49. [Crossref] [PubMed]
- Wei JT, Barocas D, Carlsson S, et al. Early Detection of Prostate Cancer: AUA/SUO Guideline Part I: Prostate Cancer Screening. J Urol 2023;210:46-53. [Crossref] [PubMed]
- Schafer EJ, Laversanne M, Sung H, et al. Recent Patterns and Trends in Global Prostate Cancer Incidence and Mortality: An Update. Eur Urol 2025;87:302-13. [Crossref] [PubMed]
- Cornford P, van den Bergh RCN, Briers E, et al. EAU-EANM-ESTRO-ESUR-ISUP-SIOG Guidelines on Prostate Cancer-2024 Update. Part I: Screening, Diagnosis, and Local Treatment with Curative Intent. Eur Urol 2024;86:148-63. [Crossref] [PubMed]
- Zaorsky NG, Calais J, Fanti S, et al. Salvage therapy for prostate cancer after radical prostatectomy. Nat Rev Urol 2021;18:643-68. [Crossref] [PubMed]
- Shore ND, Moul JW, Pienta KJ, et al. Biochemical recurrence in patients with prostate cancer after primary definitive therapy: treatment based on risk stratification. Prostate Cancer Prostatic Dis 2024;27:192-201. [Crossref] [PubMed]
- Cooperberg MR, Pasta DJ, Elkin EP, et al. The University of California, San Francisco Cancer of the Prostate Risk Assessment score: a straightforward and reliable preoperative predictor of disease recurrence after radical prostatectomy. J Urol 2005;173:1938-42. [Crossref] [PubMed]
- Meurs P, Galvin R, Fanning DM, et al. Prognostic value of the CAPRA clinical prediction rule: a systematic review and meta-analysis. BJU Int 2013;111:427-36. [Crossref] [PubMed]
- Stephenson AJ, Scardino PT, Eastham JA, et al. Preoperative nomogram predicting the 10-year probability of prostate cancer recurrence after radical prostatectomy. J Natl Cancer Inst 2006;98:715-7. [Crossref] [PubMed]
- Stephenson AJ, Scardino PT, Eastham JA, et al. Postoperative nomogram predicting the 10-year probability of prostate cancer recurrence after radical prostatectomy. J Clin Oncol 2005;23:7005-12. [Crossref] [PubMed]
- Lasorsa F, di Meo NA, Rutigliano M, et al. Emerging Hallmarks of Metabolic Reprogramming in Prostate Cancer. Int J Mol Sci 2023;24:910. [Crossref] [PubMed]
- di Meo NA, Lasorsa F, Rutigliano M, et al. The dark side of lipid metabolism in prostate and renal carcinoma: novel insights into molecular diagnostic and biomarker discovery. Expert Rev Mol Diagn 2023;23:297-313. [Crossref] [PubMed]
- Lucarelli G, Loizzo D, Ferro M, et al. Metabolomic profiling for the identification of novel diagnostic markers and therapeutic targets in prostate cancer: an update. Expert Rev Mol Diagn 2019;19:377-87. [Crossref] [PubMed]
- Cardoso HJ, Carvalho TMA, Fonseca LRS, et al. Revisiting prostate cancer metabolism: From metabolites to disease and therapy. Med Res Rev 2021;41:1499-538. [Crossref] [PubMed]
- Pujana-Vaquerizo M, Bozal-Basterra L, Carracedo A. Metabolic adaptations in prostate cancer. Br J Cancer 2024;131:1250-62. [Crossref] [PubMed]
- McDunn JE, Li Z, Adam KP, et al. Metabolomic signatures of aggressive prostate cancer. Prostate 2013;73:1547-60. [Crossref] [PubMed]
- Gkotsos G, Virgiliou C, Lagoudaki I, et al. The Role of Sarcosine, Uracil, and Kynurenic Acid Metabolism in Urine for Diagnosis and Progression Monitoring of Prostate Cancer. Metabolites 2017;7:9. [Crossref] [PubMed]
- Nitusca D, Socaciu C, Socaciu AI, et al. Potential Diagnostic Biomarker Detection for Prostate Cancer Using Untargeted and Targeted Metabolomic Profiling. Curr Issues Mol Biol 2023;45:5036-51. [Crossref] [PubMed]
- Clendinen CS, Gaul DA, Monge ME, et al. Preoperative Metabolic Signatures of Prostate Cancer Recurrence Following Radical Prostatectomy. J Proteome Res 2019;18:1316-27. [Crossref] [PubMed]
- Pichler R, Fritz J, Heidegger I, et al. Predictive and prognostic role of serum neopterin and tryptophan breakdown in prostate cancer. Cancer Sci 2017;108:663-70. [Crossref] [PubMed]
- Taylor BS, Schultz N, Hieronymus H, et al. Integrative genomic profiling of human prostate cancer. Cancer Cell 2010;18:11-22. [Crossref] [PubMed]
- Gao J, Aksoy BA, Dogrusoz U, et al. Integrative analysis of complex cancer genomics and clinical profiles using the cBioPortal. Sci Signal 2013;6:pl1. [Crossref] [PubMed]
- Song H, Weinstein HNW, Allegakoen P, et al. Single-cell analysis of human primary prostate cancer reveals the heterogeneity of tumor-associated epithelial cell states. Nat Commun 2022;13:141. [Crossref] [PubMed]
- Thul PJ, Åkesson L, Wiking M, et al. A subcellular map of the human proteome. Science 2017;356:eaal3321. [Crossref] [PubMed]
- Lughezzani G, Budäus L, Isbarn H, et al. Head-to-head comparison of the three most commonly used preoperative models for prediction of biochemical recurrence after radical prostatectomy. Eur Urol 2010;57:562-8. [Crossref] [PubMed]
- Li Z, Ding B, Ali MRK, et al. Dual Effect of Tryptamine on Prostate Cancer Cell Growth Regulation: A Pilot Study. Int J Mol Sci 2022;23:11087. [Crossref] [PubMed]
- Xue C, Li G, Zheng Q, et al. Tryptophan metabolism in health and disease. Cell Metab 2023;35:1304-26. [Crossref] [PubMed]
- Basson C, Serem JC, Hlophe YN, et al. The tryptophan-kynurenine pathway in immunomodulation and cancer metastasis. Cancer Med 2023;12:18691-701. [Crossref] [PubMed]
- Yan J, Chen D, Ye Z, et al. Molecular mechanisms and therapeutic significance of Tryptophan Metabolism and signaling in cancer. Mol Cancer 2024;23:241. [Crossref] [PubMed]
- Muzio G, Maggiora M, Paiuzzi E, et al. Aldehyde dehydrogenases and cell proliferation. Free Radic Biol Med 2012;52:735-46. [Crossref] [PubMed]
- Tan D, Miao D, Zhao C, et al. N6-methyladenosine-modified ALDH9A1 modulates lipid accumulation and tumor progression in clear cell renal cell carcinoma through the NPM1/IQGAP2/AKT signaling pathway. Cell Death Dis 2024;15:520. [Crossref] [PubMed]
- van Karnebeek CDM, Ramos RJ, Wen XY, et al. Bi-allelic GOT2 Mutations Cause a Treatable Malate-Aspartate Shuttle-Related Encephalopathy. Am J Hum Genet 2019;105:534-48. [Crossref] [PubMed]
- Jiang Y, He C, Ye H, et al. Comprehensive analysis of the lysine succinylome in fish oil-treated prostate cancer cells. Life Sci Alliance 2023;6:e202302131. [Crossref] [PubMed]
- Cao YY, Chen YY, Wang MS, et al. A catalase inhibitor: Targeting the NADPH-binding site for castration-resistant prostate cancer therapy. Redox Biol 2023;63:102751. [Crossref] [PubMed]
- Giginis F, Wang J, Chavez A, et al. Catalase as a novel drug target for metastatic castration-resistant prostate cancer. Am J Cancer Res 2023;13:2644-56. [PubMed]
- Dai X, Shi X, Luo M, et al. Integrative analysis of transcriptomic and metabolomic profiles reveals enhanced arginine metabolism in androgen-independent prostate cancer cells. BMC Cancer 2023;23:1241. [Crossref] [PubMed]
- Kobelyatskaya AA, Kudryavtsev AA, Kudryavtseva AV, et al. ALDH3A2, ODF2, QSOX2, and MicroRNA-503-5p Expression to Forecast Recurrence in TMPRSS2-ERG-Positive Prostate Cancer. Int J Mol Sci 2022;23:11695. [Crossref] [PubMed]
- Oka Y, Hamada M, Nakazawa Y, et al. Digenic mutations in ALDH2 and ADH5 impair formaldehyde clearance and cause a multisystem disorder, AMeD syndrome. Sci Adv 2020;6:eabd7197. [Crossref] [PubMed]
- Chen CH, Ferreira JC, Gross ER, et al. Targeting aldehyde dehydrogenase 2: new therapeutic opportunities. Physiol Rev 2014;94:1-34. [Crossref] [PubMed]
- Zhu W, Feng D, Shi X, et al. The Potential Role of Mitochondrial Acetaldehyde Dehydrogenase 2 in Urological Cancers From the Perspective of Ferroptosis and Cellular Senescence. Front Cell Dev Biol 2022;10:850145. [Crossref] [PubMed]
- Zhang H, Fu L. The role of ALDH2 in tumorigenesis and tumor progression: Targeting ALDH2 as a potential cancer treatment. Acta Pharm Sin B 2021;11:1400-11. [Crossref] [PubMed]
- Vantaku V, Putluri V, Bader DA, et al. Epigenetic loss of AOX1 expression via EZH2 leads to metabolic deregulations and promotes bladder cancer progression. Oncogene 2020;39:6265-85. [Crossref] [PubMed]
- Wu J, Wei Y, Li T, et al. DNA Methylation-Mediated Lowly Expressed AOX1 Promotes Cell Migration and Invasion of Prostate Cancer. Urol Int 2023;107:517-25. [Crossref] [PubMed]
- Jakobsen S, Nielsen CU. Exploring Amino Acid Transporters as Therapeutic Targets for Cancer: An Examination of Inhibitor Structures, Selectivity Issues, and Discovery Approaches. Pharmaceutics 2024;16:197. [Crossref] [PubMed]
- Kanai Y. Amino acid transporter LAT1 (SLC7A5) as a molecular target for cancer diagnosis and therapeutics. Pharmacol Ther 2022;230:107964. [Crossref] [PubMed]
- Xu M, Sakamoto S, Matsushima J, et al. Up-Regulation of LAT1 during Antiandrogen Therapy Contributes to Progression in Prostate Cancer Cells. J Urol 2016;195:1588-97. [Crossref] [PubMed]
- Lopes de Carvalho L, Bligt-Lindén E, Ramaiah A, et al. Evolution and functional classification of mammalian copper amine oxidases. Mol Phylogenet Evol 2019;139:106571. [Crossref] [PubMed]
- Ding Y, Feng Y, Huang Z, et al. SOX15 transcriptionally increases the function of AOC1 to modulate ferroptosis and progression in prostate cancer. Cell Death Dis 2022;13:673. [Crossref] [PubMed]
- Zeitler L, Murray PJ. IL4i1 and IDO1: Oxidases that control a tryptophan metabolic nexus in cancer. J Biol Chem 2023;299:104827. [Crossref] [PubMed]
- Sadik A, Somarribas Patterson LF, Öztürk S, et al. IL4I1 Is a Metabolic Immune Checkpoint that Activates the AHR and Promotes Tumor Progression. Cell 2020;182:1252-1270.e34. [Crossref] [PubMed]
- Aubatin A, Sako N, Decrouy X, et al. IL4-induced gene 1 is secreted at the immune synapse and modulates TCR activation independently of its enzymatic activity. Eur J Immunol 2018;48:106-19. [Crossref] [PubMed]
- Castellano F, Prevost-Blondel A, Cohen JL, et al. What role for AHR activation in IL4I1-mediated immunosuppression ? Oncoimmunology 2021;10:1924500. [Crossref] [PubMed]
- Akpinar C, Kuru Oz D, Oktar A, et al. Preoperative multiparametric magnetic resonance imaging based risk stratification system for predicting biochemical recurrence after radical prostatectomy. Surg Oncol 2024;57:102150. [Crossref] [PubMed]
- Leapman MS, Ho J, Liu Y, et al. Association Between the Decipher Genomic Classifier and Prostate Cancer Outcome in the Real-world Setting. Eur Urol Oncol 2024;S2588-9311(24)00183-4.
- de Visser KE, Joyce JA. The evolving tumor microenvironment: From cancer initiation to metastatic outgrowth. Cancer Cell 2023;41:374-403. [Crossref] [PubMed]
- Vilbois S, Xu Y, Ho PC. Metabolic interplay: tumor macrophages and regulatory T cells. Trends Cancer 2024;10:242-55. [Crossref] [PubMed]
- Tanaka A, Sakaguchi S. Regulatory T cells in cancer immunotherapy. Cell Res 2017;27:109-18. [Crossref] [PubMed]
- Shan F, Somasundaram A, Bruno TC, et al. Therapeutic targeting of regulatory T cells in cancer. Trends Cancer 2022;8:944-61. [Crossref] [PubMed]
- Peng Y, Tao Y, Zhang Y, et al. CD25: A potential tumor therapeutic target. Int J Cancer 2023;152:1290-303. [Crossref] [PubMed]
- Iglesias-Escudero M, Arias-González N, Martínez-Cáceres E. Regulatory cells and the effect of cancer immunotherapy. Mol Cancer 2023;22:26. [Crossref] [PubMed]
- The Molecular Taxonomy of Primary Prostate Cancer. Cell 2015;163:1011-25. [Crossref] [PubMed]
- Zhao SG, Chang SL, Erho N, et al. Associations of Luminal and Basal Subtyping of Prostate Cancer With Prognosis and Response to Androgen Deprivation Therapy. JAMA Oncol 2017;3:1663-72. [Crossref] [PubMed]
- Kzhyshkowska J, Shen J, Larionova I. Targeting of TAMs: can we be more clever than cancer cells? Cell Mol Immunol 2024;21:1376-409. [Crossref] [PubMed]
- Chen S, Saeed AFUH, Liu Q, et al. Macrophages in immunoregulation and therapeutics. Signal Transduct Target Ther 2023;8:207. [Crossref] [PubMed]
- Dussold C, Zilinger K, Turunen J, et al. Modulation of macrophage metabolism as an emerging immunotherapy strategy for cancer. J Clin Invest 2024;134:e175445. [Crossref] [PubMed]
- Wang H, Wang X, Zhang X, et al. The promising role of tumor-associated macrophages in the treatment of cancer. Drug Resist Updat 2024;73:101041. [Crossref] [PubMed]