Identification and validation of glycosyltransferase-related gene signatures to predict prognosis and immunological characteristics of renal clear cell carcinoma
Highlight box
Key findings
• A prognostic model for clear cell renal cell carcinoma (ccRCC) was developed based on four glycosyltransferase (GT)-related signature genes (ST8SIA4, GCNT4, LARGE2, and GALNT14).
• This model showed high predictive performance for patient prognosis and was validated using external datasets, and quantitative real-time polymerase chain reaction experiments validated the expression levels of the four signature genes, confirming their reliability as biomarkers.
What is known and what is new?
• GTs play a key role in tumor progression, immune evasion, and glycosylation modification. Previous studies have linked glycosylation-related genes to cancer, but their prognostic value in ccRCC has not been fully explored.
• This study identifies and validates four GT-related genes as independent prognostic biomarkers for ccRCC. A novel risk stratification model was established, demonstrating its clinical utility in predicting prognosis, immune characteristics, and drug sensitivity.
What is the implication, and what should change now?
• This study provides a novel GT-based prognostic model, which can help stratify ccRCC patients and predict immunotherapy response. The four signature genes could serve as potential therapeutic targets for ccRCC.
• Future studies should focus on elucidating the molecular mechanisms of ST8SIA4, GCNT4, LARGE2, and GALNT14 in ccRCC. Additionally, large-scale clinical trials are required to validate the generalizability of this model across diverse populations.
Introduction
In 2022, there were 434,419 new cases of kidney cancer globally, constituting 2.2% of all cancer incidences, and 155,702 deaths, representing 1.6% of total cancer fatalities. The incidence and mortality rates of kidney cancer are influenced by regional factors and are generally higher in countries with a high Human Development Index (HDI) (1,2). This disease predominantly affects males, with primary risk factors including smoking, obesity, hypertension, and a familial history of kidney disease (3). Kidney cancer classification primarily depends on the cytological and structural characteristics of the tumor, as well as its immunohistochemical expression profile. The World Health Organization (WHO) recognizes 14 subtypes of kidney cancer, with clear cell renal cell carcinoma (ccRCC) being the most common (4). The metastasis rate of ccRCC is closely associated with the disease stage at diagnosis (5). Although surgery significantly improves survival rates, approximately 30% of patients still experience metastasis post-operation, and they exhibit a worse prognosis compared to those with other subtypes (6). Recently, immunotherapy, including treatments with immune checkpoint inhibitors such as nivolumab and pembrolizumab [programmed death 1 (PD-1) inhibitors] (7,8), and ipilimumab (cytotoxic T-lymphocyte associated antigen inhibitor) (9), has been widely adopted for ccRCC patients. Nevertheless, due to the immunological diversity among patients, devising an optimal treatment strategy remains a major challenge (10).
Against this backdrop, multiple studies have provided crucial evidence for clinical practice and revealed issues that urgently need to be addressed. A real-world study (11) compared the efficacy of two immunotherapy combinations, immune oncology (IO) + tyrosine kinase inhibitor (TKI) and IO + IO, in patients with advanced renal cell carcinoma (RCC). It was found that in patients with moderate-risk RCC, overall survival (OS) and progression-free survival (PFS) were significantly better with IO + TKI treatment than with IO + IO. However, in high-risk patients, there was no difference in efficacy between the two treatment regimens. This result not only indicates that risk stratification is an important basis for choosing combination treatment regimens but also reflects the complexity of RCC treatment decisions. A recent meta-analysis (12) showed that an increase in the Royal Marsden Hospital (RMH) score was significantly associated with a poor prognosis. This study once again demonstrates the importance of risk stratification and provides a standardized tool for RCC risk stratification. However, the clinical application of immunotherapy still faces two core issues. The first is the mechanism of drug resistance. A review study (13) pointed out that the dynamics of immunotherapy resistance in patients with advanced RCC involve multiple mechanisms, such as tumor microenvironment remodeling and activation of immune escape pathways. The second is treatment-related toxicity. Immunotherapy may cause various organ-specific side effects (14). In addition, significant progress has been made in the research of prognostic biomarkers. For the neutrophil-to-eosinophil ratio (NER), an increase in the pre-treatment NER level was significantly associated with a shortening of OS/PFS in cancer patients (15), suggesting that it can be used as a dynamic monitoring indicator. In conclusion, the treatment and prognosis assessment of advanced RCC require comprehensive consideration of multiple factors. Future research should further explore individualized treatment strategies to improve patient prognosis.
The role of glycosyltransferases (GTs) in cancer progression has gained increasing attention. These enzymes catalyze glycosyl transfer reactions that modify proteins, lipids, and polysaccharides, thereby influencing glycosylation patterns. These modifications are crucial for processes such as cell signaling, cell adhesion, immune evasion, and the modulation of the tumor microenvironment (16). For example, the GT ST6Gal-I mediates the interaction between programmed death-ligand 1 (PD-L1) and PD-1 through glycosylation, subsequently inhibiting T-cell activation (17). Similarly, GT GnT-V enhances vascular endothelial growth factor (VEGF) receptor activity via glycosylation, facilitating the development of tumor vasculature and enhancing the supply of nutrients and oxygen to tumor cells (18). Moreover, tumor-associated glycosylation modifications serve as biomarkers for early cancer diagnosis. For example, CA19-9 is a widely recognized serum marker for pancreatic cancer (19). Importantly, in addition to serving as biomarkers, monoclonal antibodies targeting glycosylation modifications, as well as CAR-T cell therapy, have also shown potential in tumor immunotherapy (20,21). Although GTs play a significant role in tumor progression and treatment, they have not yet been utilized to predict prognosis and immunotherapy response in ccRCC.
The Gene Expression Omnibus Database (GEO) is a public repository for genomic data, featuring high-throughput gene expression datasets submitted by research institutions worldwide (22). The Cancer Genome Atlas (TCGA) compiles comprehensive clinical, pathological, and biological information from cancer patients (23). Researchers can leverage this data, along with various bioinformatics approaches, to identify and predict new diagnostic and prognostic biomarkers across different cancer types. Machine learning, a branch of artificial intelligence, has numerous applications in the medical field. It aids in developing informative models and predicting biological phenomena, thereby significantly influencing the field of biology.
In this study, we employed univariate Cox, least absolute shrinkage and selection operator (LASSO), and multivariate Cox regression analyses to develop a prognostic model based on GTs-related genes. The benefits of immunotherapy in the low- and high-risk groups were predicted, and chemotherapy agents more suitable for patients in the high-risk group were further identified. The prognostic model highlights four signature genes (ST8SIA4, GCNT4, LARGE2, and GALNT14) as potential biomarkers for ccRCC. Overall, our prognostic model based on GTs-related genes provides a valuable tool for predicting ccRCC patient outcomes and supports the development of personalized treatment strategies. We present this article in accordance with the TRIPOD reporting checklist (available at https://tau.amegroups.com/article/view/10.21037/tau-2025-21/rc).
Methods
Research population and data collection
The study was conducted in accordance with the Declaration of Helsinki and its subsequent amendments. Transcriptome messenger ribonucleic acid (mRNA) data from 59 normal samples and 535 ccRCC patient samples were retrieved from TCGA database (https://portal.gdc.cancer.gov/) (24). This dataset included additional information on mutations, copy number variations (CNVs), and corresponding clinical data. The 594 datasets were randomly divided into a training set (70%) and a validation set (30%). To further validate the model, microarray data (GSE29609) with 39 samples were obtained from the GEO database (https://www.ncbi.nlm.nih.gov/geo/) as an additional validation set. GTs-related genes were obtained from related research (Table S1) (25).
Analysis of differential genes associated with GTs
The edgeR package was employed to conduct differential expression analysis between normal and tumor samples in ccRCC [|log fold change (FC)| >0.585 and false discovery rate (FDR) <0.05]. Differentially expressed genes (DEGs) in ccRCC were cross-referenced with GTs-related genes to identify differentially GTs-related genes (DGRGs). The chi-square test was applied to compare CNVs between normal and ccRCC tumor samples, specifically focusing on the CNVs of DGRGs. The “RCircos” package was utilized to illustrate the chromosomal locations of DGRGs. Tumor mutational burden (TMB) data were analyzed to evaluate the TMB status of these genes. Finally, Gene Ontology (GO) and Kyoto Encyclopedia of Genes and Genomes (KEGG) enrichment analyses were performed on DGRGs to identify associated biological processes and functions.
Construction of prognostic markers in ccRCC based on DGRGs
Tumor patient samples with a survival duration exceeding 30 days were selected from clinical datasets. Univariate Cox regression analysis of DGRGs was performed using the “survival” package (P<0.05). To mitigate the risk of model overfitting and enhance robustness, LASSO regression analysis was subsequently applied using the “glmnet” package. The penalty parameter lambda was optimized through cross-validation to simplify the model and eliminate redundant genes. The “survival” package was then used again to conduct multivariate Cox regression analysis on the candidate genes selected by the LASSO method, aiming to identify the final signature genes and construct a prognostic model. The formula for the risk score is as follows (The “Coef” represents non-zero regression coefficients, and “ExpGene” denotes the expression value of a gene derived from the prognostic risk score model):
The Kaplan-Meier method was used to assess the prognostic efficacy of the model. The timeROC package was used to generate the receiver operating characteristic (ROC) curves and calculate the area under the curve (AUC) for 1-, 3-, and 5-year survival predictions. To evaluate the model’s robustness and generalizability, independent validation was performed using an external dataset, including plotting survival and ROC curves.
Gene set enrichment analysis (GSEA)
The samples were divided into high- and low-risk groups based on the median risk score, and GSEA was used for pathway enrichment in each group. Subsequently, GO, KEGG pathway enrichment analyses were carried out for differential genes in high- and low-risk groups, respectively.
Nomogram construction and evaluation of ccRCC patients
Clinical data and prognostic model risk scores were utilized to generate forest plots. The “rms” package was applied to construct nomograms for predicting 1-, 3-, and 5-year patient survival rates, while calibration curves and decision curve analysis (DCA) were employed to assess the predictive accuracy of these nomograms and to determine whether the model functions as an independent prognostic factor.
Immune infiltration analysis
Single-sample gene set enrichment analysis (ssGSEA) in gene set variation analysis (GSVA) package was used for immune enrichment analysis. The ESTIMATE algorithm was used to estimate the immune scores in tumor tissues. The CIBERSORT algorithm was used to assess the correlation between risk scores and the levels of immune cell infiltration (26). TIDE (Tumor Immune Dysfunction and Exclusion, http://tide.dfci.harvard.edu/) was used to calculate tumor immune dysfunction and exclusion scores. Differences in the expression of immune-related genes in high- and low-risk groups were also calculated.
TMB and drug sensitivity analysis
The mutation data of ccRCC were used to calculate the TMB score of each sample. The mutation data of the top 30 genes was organized and statistically analyzed for the high- and low-risk groups, visualized using the GenVisR package. The CellMiner database (https://discover.nci.nih.gov/cellminer/) was employed to identify antitumor drugs significantly associated with prognostic genes. The pRRophetic package was used to predict the half-maximal inhibitory concentration (IC50) of various drugs.
Quantitative real-time polymerase chain reaction (qRT-PCR) validation of the feature genes
Human renal epithelial cells (HK-2) and renal clear cell adenocarcinoma cells (786-O) were obtained from the Cell Bank of the Chinese Academy of Sciences (Shanghai, China). Cells were cultured in RPMI-1640 medium for the gene expression analysis. Total RNA was extracted using TRIzol reagent, and cDNA synthesis was performed with the PrimerScript RT Reagent Kit (Ugbio, Hangzhou). RT-PCR was conducted using SYBR Green and the RT-PCR System. Cycle thresholds (CT) were determined for each sample, and the expression levels of ST8SIA4, GALNT14, GCNT4, and LARGE2 were calculated using the 2-ΔΔCT method. Primer sequences are listed in Table 1.
Table 1
Primer name | Sequence (5'-3') |
---|---|
GAPDH-F | ACATCGCTCAGACACCATG |
GAPDH-R | TGTAGTTGAGGTCAATGAAGGG |
GALNT14-F | ACCACTCTCTCCCTACCAAA |
GALNT14-R | CAGCAGTGTGCATCTGACTTG |
GCNT4-F | TCACGTAGGAAACGGTGCTA |
GCNT4-R | ATGGCGTGACTGGGACAGTG |
LARGE2-F | ACTGCTAGCCAGCAGCATCT |
LARGE2-R | AGCGACCGACCGAGTACGAC |
ST8SIA4-F | AGACCTGTGCAGTTGTTGGA |
ST8SIA4-R | CCACAGGAGCTAGATTACACCTTA |
Statistical analysis
Data analysis was performed using the statistical software R (version 4.4.1) and related packages. Survival analysis was conducted using the Kaplan-Meier method, with statistical significance assessed through the log-rank test. The predictive accuracy of this model was assessed via time-dependent ROC curve analysis. The Wilcoxon test was applied to compare statistical differences between groups. Data visualization was primarily performed using the “ggplot2” package. Statistical significance was determined with P values of less than 0.05 and FDR below 0.05.
Results
Function and pathway enrichment analysis of DGRGs
We utilized the edgeR package to identify 8,505 DEGs in ccRCC sourced from the TCGA database. DGRGs were defined as the intersection of ccRCC differential genes and GTs-related genes (Figure 1A). Ultimately, we identified a total of 104 DGRGs. Abnormal gene mutations significantly contribute to cancer development. To identify the genetic alterations in ccRCC, we performed TMB analysis, and the five most frequently mutated genes were ARFGF1, TP63, DPY19L1, GALNT5, and GCNT4 (Figure 1B). To explore the biological roles and pathways associated with DGRGs, we conducted GO and KEGG enrichment analyses (Figure 1C,1D). GO enrichment analysis demonstrated that these genes predominantly involved in GT activities, including hexosyltransferase, UDP-GT, and acetylglucosaminyl transferase activities, as well as carbohydrate binding (Figure 1C). These enzymatic functions are crucial for glycosylation processes and are implicated in cell recognition, signal transduction, immune responses, and other cellular processes (27). Additionally, KEGG pathway enrichment analysis showed significant involvement of these genes in pathways such as Mucin type O-glycan biosynthesis, other types of O-glycan biosynthesis, Glycosphingolipid biosynthesis-lacto and neolacto series (Figure 1D).
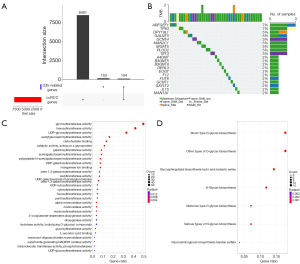
Establishment of ccRCC prognostic model based on signature genes.
To build a prognostic model for ccRCC, we performed univariate regression analysis on 104 differential genes associated with clinical survival. This approach allowed us to identify 21 candidate genes that significantly correlate with survival (Figure 2A, Table S2). To enhance the accuracy of these candidates, we applied LASSO regression analysis alongside multiple regression analysis (Figure 2B-2D). Through LASSO analysis, we identified seven candidate genes from a pool of 21 that showed a significant correlation with survival (Figure 2B,2C, Table S3). Subsequently, we performed multiple regression analysis on these seven candidate genes and recognized four distinct signature genes (Figure 2D). Moreover, we obtained the following formula: Riskscore = −0.00014*ST8SIA4 − 0.00077*GCNT4 − 0.00061*LARGE2 − 0.000049*GALNT14 (Table S4). This formula enables the computation of risk scores across various datasets. To understand the correlation among the four signature genes, we performed a correlation analysis on these genes. Correlation analysis among the 4 signature genes demonstrated positive correlations between GALNT14 and ST8SIA4 (r=0.221), and between LARGE2 and GCNT4 (r=0.372). In contrast, the remaining gene pairs exhibited negative correlations (Figure 2E). To assess the expression levels of four signature genes in both tumor and normal samples, we conducted expression profiling analysis for each gene. The results indicated that these four signature genes function as protective factors, with ST8SIA4 and GALNT14 significantly upregulated, while GCNT4 and LARGE2 significantly downregulated in tumor samples compared to normal samples (Figure 2F).
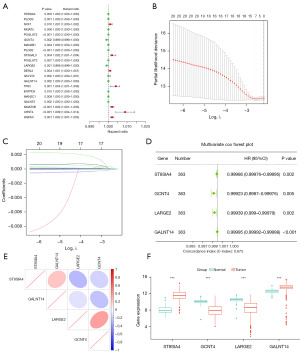
Utilizing the risk formula derived from the aforementioned multiple regression analysis, we calculated the risk scores of the TCGA training set. Based on the median risk score, the samples were categorized into high- and low-risk groups. Kaplan-Meier survival analysis revealed that the high-risk group exhibited lower survival probabilities than the low-risk group (Figure 3A). The model’s reliability was validated using GSE29609 validation set (Figure 3B) and TCGA test set (Figure 3C), both showing lower survival probabilities for patients in the high-risk group. We next sought to verify the risk scoring on clinical samples in the TCGA training set. The AUC values for 1-, 3-, and 5-year predictions in the TCGA cohort were 0.711, 0.692, and 0.719, respectively (Figure 3D). To validate the predictive performance of the model, AUC values in TCGA validation set were 0.737, 0.772, and 0.906 (Figure 3E). Similarly, the GSE29609 validation set was used to verify the prediction effect, and the AUC values were 0.717, 0.72 and 0.761, respectively (Figure 3F).
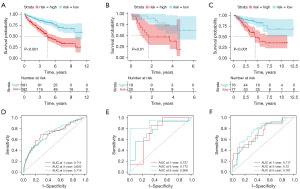
Furthermore, risk scores, survival status, and expression patterns were analyzed in the TCGA training set, GEO validation set, and TCGA test set. The survival status analysis revealed that patients with higher risk scores exhibited a higher mortality rate (Figure 4A). The heatmap demonstrated significant differences in the expression levels of the four signature genes between the high- and low-risk groups (Figure 4B). Similarly, the same trend was observed in the GSE29609 validation set (Figure 4C,4D) and was further confirmed in the TCGA test set (Figure 4E,4F). Overall, the results suggested that the prognostic model for ccRCC, derived from four signature genes, demonstrated strong predictive capability.
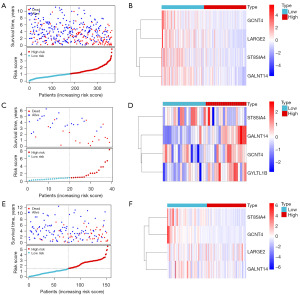
Independent prognostic analysis of ccRCC signature genes
Using the previously detailed risk formula: Riskscore = −0.00014*ST8SIA4 − 0.00077*GCNT4 − 0.00061*LARGE2 − 0.000049*GALNT14, we can evaluate the risk values for the TCGA training set based on age, TNM stage (T: Tumor, N: Node, M: Metastasis), and cancer stage. Patients aged 65 years old and over had significantly lower risk scores than those under 65 (P=0.02, Figure 5A). The risk scores for patients in T3+T4 stages significantly higher than those in T1+T2 stages (P<0.001, Figure 5A). Similarly, the risk scores for N1 stages were greater than those for N0 stages (P<0.001, Figure 5A), and M1 stages showed significantly higher scores compared to M0 stages (P=0.003, Figure 5A). Patients in stages III and IV had significantly higher risk scores than those in stages I and II (P<0.001, Figure 5A). Survival curves based on signature genes expression revealed that high expression of all four genes was associated with significantly better OS compared to low expression (Figure 5B).
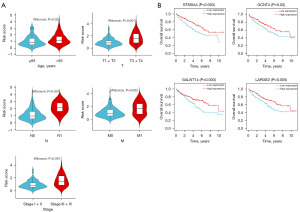
To assess the relationship between five clinical variables (age, T stage, N stage, M stage, and cancer stage), the TCGA training set risk score, and survival rates, we performed a univariate regression analysis. Our findings indicated that TNM stage, cancer stage, and risk score were significantly correlated with survival (Figure 6A). Subsequently, multiple regression analysis revealed that both M stage and risk score were notably associated with survival outcomes (Figure 6B). Next, five clinical variables (age, T stage, N stage, M stage, and cancer stage), along with TCGA training set risk scores, were used to predict patients’ 1-, 3-, and 5-year survival, constructing the following nomogram (Figure 6C). Calibration curves demonstrated a close alignment between the model’s predicted and actual survival rates (Figure 6D). The DCA curves showed that our prognostic model exhibited good net benefit for 1-, 3- and 5-year (Figure 6E). These findings reaffirm the prognostic model, based on the four signature genes, as an independent prognostic factor.
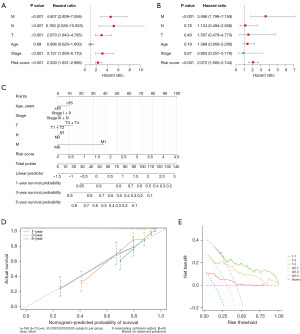
The analysis of immune microenvironment
In recent decades, significant breakthroughs have been achieved in tumor immunotherapy. Immune infiltration within the tumor microenvironment is a crucial factor influencing tumor progression and clinical prognosis in cancer patients (28). Accordingly, we examined the relationship between immune infiltration and risk scores to predict the therapeutic effects of immunotherapy across different risk groups. Figure 7A displays a heatmap of immune cell abundance derived from the ssGSEA algorithm, showing that the high-risk group generally exhibits a higher relative abundance of immune cells compared to the low-risk group. We assessed the scores of the high- and low-risk groups using the ESTIMATE algorithms. The results demonstrate that the immune score, Stromal score, and ESTIMATE score are significantly lower in the low-risk group compared to the high-risk group (Figure 7B-7D). In contrast, the tumor purity score is significantly higher in the low-risk group than in the high-risk group (Figure 7E, P<0.001). Statistical analysis of TIDE scores reveals that the low-risk group has a lower TIDE score (Figure 7F). CIBERSORT analysis indicates a significantly higher proportion of plasma cells and resting memory CD4 T cells in the high-risk group than in the low-risk group (Figure 7G), whereas activated NK cells and resting mast cells are more prevalent in the low-risk group (Figure 7G). Immunological analyses indicate a significant increase in plasma cells and resting memory CD4 T cells in the high-risk group. These findings, along with TIDE results, suggest a heightened likelihood of tumor immune evasion in these patients, indicating that high-risk ccRCC patients may derive less benefit from immune checkpoint blockade (ICB) therapy. Differences in the expression of immune-related genes between the high- and low-risk groups were evaluated, showing that all immune-related genes exhibit higher expression levels in the high-risk group (Figure 7H). These findings suggest that patients in the low-risk group may experience better immunotherapy outcomes.
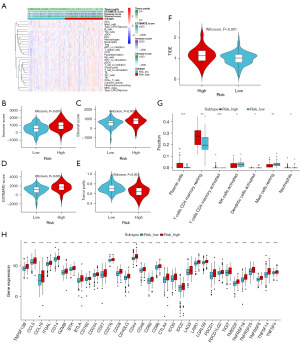
TMB analysis and drug sensitivity
Gene mutation analysis showed a higher frequency of gene mutations in the high-risk group than in the low-risk group. The results revealed that VHL, PBRM1, and TTN were the three most frequently mutated genes in both risk groups, with mutations primarily observed as frameshift and missense variations (Figure 8A,8B). Given that the risk score of signature genes was associated with poor prognosis in ccRCC patients, we further explored the difference in sensitivity to anticancer drugs between the high- and low-risk groups. Sensitivity predictions for anticancer drugs showed that the high-risk group had significantly lower half-maximal inhibitory concentration (IC50) values for cisplatin, dasatinib and axitinib than the low-risk group (Figure 8C-8E). These findings suggested that cisplatin, dasatinib and axitinib may be more effective in treating high-risk patients. Additionally, drug screening based on gene expression levels in the prognostic model, using the CellMiner database identified a significant positive correlation between IC50 values for cisplatin and the expression of ST8SIA4 (Figure 8F, P=0.01, r=0.315). In contrast, the expression of ST8SIA4 had a significantly negative correlation with the IC50 of dasatinib (Figure 8G, P=0.03, r=−0.277). Furthermore, the expression of GALNT14 was significantly positive correlation with the IC50 of gemcitabine (Figure 8H, P=0.003, r=0.383). Similarly, the expression of ST8SIA4 showed a significantly positive correlation with IC50 on etoposide (Figure 8I, P=0.001, r=0.409). These results hold substantial significance for the drug selection in the clinical management of ccRCC.
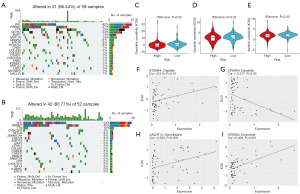
Validation of feature genes by qRT-PCR
We conducted qRT-PCR assays to assess the expression levels of ST8SIA4, GCNT4, GALNT14, and LARGE2 in normal human kidney cells (HK-2) and renal clear cell carcinoma (786-O) cells. The results demonstrated a significant upregulation of ST8SIA4 and GALNT14 expression in 786-O cells compared to HK-2 cells. However, the expression of GCNT4 and LARGE2 was significantly reduced in 786-O cells (Figure 9). These findings were consistent with our bioinformatics analysis. ST8SIA4, GCNT4, GALNT14, and LARGE2 show promise as reliable prognostic biomarkers with considerable research potential.
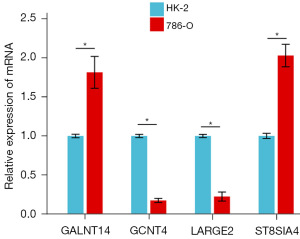
Discussion
ccRCC is the most aggressive and prevalent histological subtype of RCC, with its incidence having doubled over the past two decades (29,30). Due to the absence of distinct early clinical symptoms, approximately 40% of ccRCC cases are diagnosed at advanced stages, necessitating surgical intervention to improve the 5-year survival rate. However, postoperative complications remain a significant challenge (31). Consequently, it is essential to develop accurate prognostic models for ccRCC to predict patient outcomes and treatment responses. GTs are key enzymes in the glycosylation process, and research has demonstrated a significant negative correlation between GT expression and CD8+ T cell infiltration, which is associated with poor prognosis in tumors (32). We identified four signature genes (ST8SIA4, GCNT4, LARGE2, and GALNT14) associated with GTs as prognostic biomarkers for ccRCC.
The α-2,8-sialyltransferase (ST8SIA4) predominantly catalyzes the addition of sialic acids to glycan chains through α-2,8 linkages, a process known as sialylation, which is implicated in cancer progression (33). Ma et al. found that the expression of ST8SIA4 was increased 14.81-fold in aggressive breast cancer cells compared to non-tumorigenic cells, and silencing ST8SIA4 in these cell lines significantly reduced malignant behaviors such as cell proliferation and invasion (34). However, Soukhtehzari et al. found that high ST8SIA4 expression in breast tumor cells correlates with poor patient outcomes, while its expression in infiltrating stromal cells was linked to improved prognosis (35). Furthermore, Li et al. found that increased ST8SIA4 expression in gastric cancer was associated with significantly lower survival rates (36). GALNT14 belongs to the peptide N-acetylgalactosaminotransferase (PPGALacT-TS) family, whose glycosylation modification can significantly affect the stability and activity of the protein (37). Additionally, GALNT14 is involved in regulating the proliferation, migration and invasion of cancer cells. Kwon et al. found that GALNT14 may reduce patient survival by promoting lung cancer metastasis (38). Chiang et al. found that the expression level of GALNT14 was also associated with OS in pancreatic ductal carcinoma and cholangiocarcinoma (39). Liang et al. found that different genotypes of GALNT14 had different effects on the prognosis of cholangiocarcinoma, with the “TT” genotype being unfavorable for OS following cholangiocarcinoma resection (40). Both Song et al. and Sheta et al. found that GALNT14 may be a potential therapeutic target for breast cancer (41,42). Similar to the results of this study, low expression of GALNT14 implies that Gemcitabine has a lower IC50. LARGE2, a bifunctional GT, plays a crucial role in the maturation of alpha-dystroglycan (α-DG) (43). Dietinger et al. found that in the context of colon cancer, the expression of LARGE2 and the O-glycosylation of α-DG may have a dual impact on cancer progression. On one hand, these processes may restrict the migration and invasion of early tumor cells; on the other hand, they may facilitate liver colonization by enhancing the adhesion of circulating tumor cells to blood vessels (44). In the prognostic model of this study, LARGE2 is identified as a protective factor for ccRCC, with high expression levels correlating with improved OS. Glucosaminyl (N-Acetyl) transferase 4 (GCNT4) is a GT that plays a critical role in the biosynthesis of glycoproteins (45). GCNT4 is regulated by miR-615 (46), which has been reported to act as a tumor suppressor in various cancers (47,48). Milde-Langosch et al. found that bone metastasis in breast cancer is often accompanied by increased GCNT4 expression (49). In gastric cancer, patients with lower GCNT4 expression exhibit poorer prognoses, while overexpression of GCNT4 inhibits gastric cancer cell proliferation and cell cycle progression (45). Our findings also suggest that high expression of GCNT4 is associated with improved prognosis in ccRCC patients.
CIBERSORT analysis showed that plasma cells and resting memory CD4 T cell were significantly enriched in the high-risk group. Plasma cells are fully differentiated B cells that specialize in antibodies production, capable of surviving long-term as an important part of humoral immune memory after initial differentiation in secondary lymphoid organs or inflammatory tissues (50). Patil et al. studied the transcriptomes of tumor samples from non-small cell lung cancer (NSCLC) patients before and after treatment with different regimens in POPLAR and OAK. The results showed that the overexpressed genes in the long-lived group were enriched in pathways related to B cells and plasma cells (51). Li et al. found that, compared with normal tissues, kidney cancer tissues exhibited the decreased number of B cells, the results are consistent with those of this study (52). CD4+ T cells promote RCC proliferation, and significantly associated with lymph node metastasis, which may lead to poor prognosis in high-risk patients (53). Activated natural killer (NK) cells and resting mast cells were significantly enriched in the low-risk group. NK cells recognize and eliminate virus-infected cells and tumor cells (54). After identifying CISH (cytokine induced SH2-containing protein) as a key negative regulator of NK cell function, Zhu et al. designed chimeric antigen receptor-natural killer (CAR-NK) cells lacking this intracellular cytokine checkpoint, demonstrating significantly improved metabolic adaptability and anti-tumor activity (55). Mast cells can combat pathogens and regulate the inflammatory response of the immune system (56). But high density of mast cells is associated with poor prognosis in liver cancer, gastric adenocarcinoma, and direct bowel cancer (57-59).
Enrichment analysis revealed that the high-risk group was significantly associated with pathways including Natural Killer Cell-Mediated Cytotoxicity, Hematopoietic Cell Lineage, the Cytosolic DNA Sensing Pathway, and Cytokine-Cytokine Receptor Interaction. Furthermore, activation of the cytosolic DNA sensing pathway in ccRCC may promote tumor cell proliferation, angiogenesis, and immune evasion, thereby impacting the immunogenicity of tumor cells and the surrounding microenvironment (60,61). Drug sensitivity analysis demonstrated that individuals in the high-risk group exhibited increased sensitivity to cisplatin and dasatinib. Markova et al. investigated a targeted drug combining anti-tumor cisplatin and dasatinib to prevent the spread and metastasis of esophageal squamous cell carcinoma (62). Dasatinib can decrease the expression of MDR1 and Mcl-1, thus reducing cisplatin resistance (63). Notably, in this study, ST8SIA4 was more suitable as a biomarker for etoposide, dasatini, and cisplatin. Gemcitabine is a commonly used drug for the treatment of ccRCC (64). Yao et al. found that the patients with PIMREG overexpression are more suitable for gemcitabine (65). In our study, GALNT14 may serve as a new biomarker for gemcitabine, more suitable for patients with low expression of GALNT14. Although this study did not directly evaluate KIM1 expression, recent research highlights its significance in the prognosis of RCC and its impact on response to immunotherapy (66-68). Studies have shown that KIM1 regulates T-cell proliferation and macrophage polarization (69,70). Notably, our findings revealed a significant increase in plasma cells and resting memory CD4 T cells in the high-risk group, suggesting a potential link to KIM1-mediated immunomodulation. KIM1 promotes macrophage polarization, potentially triggering an inflammatory response. Local inflammation can induce PD-L1 expression (71), a process termed “adaptive immune resistance” in cancer (72). This suggests a possible association between KIM1 expression and PD-L1 treatment resistance. Additionally, high ST8SIA4 expression has been linked to M1 macrophage infiltration in diseases such as atherosclerosis and ankylosing spondylitis (73). This mechanism may synergize with ST8SIA4, identified as a signature gene in this study, indicating its potential as a combined biomarker. In renal cancer, KIM1 is associated with poor prognosis (74), implying that it may share molecular mechanisms or signaling pathways with the signature genes identified in this study, influencing ccRCC prognosis. Future research integrating KIM1 expression data could refine prognostic models, enhance predictions of immune checkpoint blockade responses, and provide deeper insights into tumor immunotherapy strategies.
The prognostic model for ccRCC developed in this study, based on GTs-related genes, provides a valuable tool for clinical prediction. This model allows for personalized treatment plans tailored to patient risk stratification. Scientifically, the four signature genes (ST8SIA4, GCNT4, LARGE2, and GALNT14) identified in the model are pivotal for investigating the role of GTs in the progression of ccRCC. These findings offer insights for the development of novel targeted therapies. However, knowledge gaps remain in understanding the molecular mechanisms underlying the involvement of these genes in ccRCC. Although their association with prognosis is established, the regulatory pathways of these genes, particularly ST8SIA4, are not fully understood. Future research using multi-omics technologies is needed to explore these regulatory networks, alongside in vitro and in vivo studies to validate gene functions. Moreover, the model’s reliance on existing databases highlights the need for verification through large-scale, prospective clinical studies to confirm its clinical applicability. Looking ahead, technological advancements are expected to accelerate ccRCC research. Single-cell sequencing will enable detailed analysis of tumor heterogeneity, improving immunotherapy precision. Additionally, machine learning and AI can integrate multi-omics data to create more accurate prediction models, enhancing personalized medicine. Further investigation into GTs and their associated genes may lead to novel therapies that regulate glycosylation, ultimately improving patient outcomes and quality of life.
This study developed a prognostic model for ccRCC based on four signature genes associated with GTs. Despite these findings, there are limitations in this study. While we utilized extensive sample data from the TCGA database and validated it with GEO data, these datasets are not without their constraints. The samples originate from diverse regions and ethnic groups, with varying clinical characteristics and treatment methods, which could introduce data heterogeneity. This variability may impact the model’s accuracy and generalizability across different populations. Furthermore, although the four signature genes (ST8SIA4, GCNT4, LARGE2, and GALNT14) have been identified, their upstream and downstream regulatory networks remain unclear. Therefore, the regulatory roles of the four pivotal genes in ccRCC progression need to be elucidated through integrated multi-omics and molecular biology approaches.
Conclusions
We developed a prognostic model for ccRCC based on GTs-related genes, which can serve as an independent prognostic factor with high prognostic accuracy. The four signature genes in this model provide a foundation for studying the potential mechanism of GTs involvement in the progression of ccRCC.
Acknowledgments
None.
Footnote
Reporting Checklist: The authors have completed the TRIPOD reporting checklist. Available at https://tau.amegroups.com/article/view/10.21037/tau-2025-21/rc
Peer Review File: Available at https://tau.amegroups.com/article/view/10.21037/tau-2025-21/prf
Funding: None.
Conflicts of Interest: All authors have completed the ICMJE uniform disclosure form (available at https://tau.amegroups.com/article/view/10.21037/tau-2025-21/coif). The authors have no conflicts of interest to declare.
Ethical Statement: The authors are accountable for all aspects of the work in ensuring that questions related to the accuracy or integrity of any part of the work are appropriately investigated and resolved. The study was conducted in accordance with the Declaration of Helsinki and its subsequent amendments.
Open Access Statement: This is an Open Access article distributed in accordance with the Creative Commons Attribution-NonCommercial-NoDerivs 4.0 International License (CC BY-NC-ND 4.0), which permits the non-commercial replication and distribution of the article with the strict proviso that no changes or edits are made and the original work is properly cited (including links to both the formal publication through the relevant DOI and the license). See: https://creativecommons.org/licenses/by-nc-nd/4.0/.
References
- Han S, Zhao S, Zhong R, et al. Global burden, trends, and disparities in kidney cancer attributable to smoking from 1990 to 2021. Front Public Health 2024;12:1506542. [Crossref] [PubMed]
- Wong MCS, Goggins WB, Yip BHK, et al. Incidence and mortality of kidney cancer: temporal patterns and global trends in 39 countries. Sci Rep 2017;7:15698. [Crossref] [PubMed]
- Scelo G, Larose TL. Epidemiology and Risk Factors for Kidney Cancer. J Clin Oncol 2018; Epub ahead of print. [Crossref] [PubMed]
- Sung H, Ferlay J, Siegel RL, et al. Global Cancer Statistics 2020: GLOBOCAN Estimates of Incidence and Mortality Worldwide for 36 Cancers in 185 Countries. CA Cancer J Clin 2021;71:209-49. [Crossref] [PubMed]
- Liu Y, Qi L, Ye B, et al. MOICS, a novel classier deciphering immune heterogeneity and aid precise management of clear cell renal cell carcinoma at multiomics level. Cancer Biol Ther 2024;25:2345977. [Crossref] [PubMed]
- Kim HY, Ha Thi HT, Hong S. IMP2 and IMP3 cooperate to promote the metastasis of triple-negative breast cancer through destabilization of progesterone receptor. Cancer Lett 2018;415:30-9. [Crossref] [PubMed]
- McKay RR, Leucht K, Xie W, et al. A Pooled Analysis of 3 Phase II Trials of Salvage Nivolumab/Ipilimumab After Nivolumab in Renal Cell Carcinoma. Oncologist 2024;29:324-31. [Crossref] [PubMed]
- Campi R, Pecoraro A, Roussel E, et al. Could a Risk-adapted Approach Support Shared Decision-making Regarding Eligibility for Adjuvant Pembrolizumab for Patients with Clear Cell Renal Cell Carcinoma at High Risk of Recurrence? A Multicentre Cohort Study. Eur Urol Oncol 2024;7:323-7. [Crossref] [PubMed]
- Montemagno C, Jacquel A, Pandiani C, et al. Unveiling CXCR2 as a promising therapeutic target in renal cell carcinoma: exploring the immunotherapeutic paradigm shift through its inhibition by RCT001. J Exp Clin Cancer Res 2024;43:86. [Crossref] [PubMed]
- Sharma P, Siddiqui BA, Anandhan S, et al. The Next Decade of Immune Checkpoint Therapy. Cancer Discov 2021;11:838-57. [Crossref] [PubMed]
- Santoni M, Buti S, Myint ZW, et al. Real-world Outcome of Patients with Advanced Renal Cell Carcinoma and Intermediate- or Poor-risk International Metastatic Renal Cell Carcinoma Database Consortium Criteria Treated by Immune-oncology Combinations: Differential Effectiveness by Risk Group? Eur Urol Oncol 2024;7:102-11. [Crossref] [PubMed]
- Sahin TK, Rizzo A, Aksoy S, et al. Prognostic Significance of the Royal Marsden Hospital (RMH) Score in Patients with Cancer: A Systematic Review and Meta-Analysis. Cancers (Basel) 2024;16:1835. [Crossref] [PubMed]
- Guven DC, Erul E, Kaygusuz Y, et al. Immune checkpoint inhibitor-related hearing loss: a systematic review and analysis of individual patient data. Support Care Cancer 2023;31:624. [Crossref] [PubMed]
- Santoni M, Mollica V, Rizzo A, et al. Dynamics of resistance to immunotherapy and TKI in patients with advanced renal cell carcinoma. Cancer Treat Rev 2025;133:102881. [Crossref] [PubMed]
- Sahin TK, Ayasun R, Rizzo A, et al. Prognostic Value of Neutrophil-to-Eosinophil Ratio (NER) in Cancer: A Systematic Review and Meta-Analysis. Cancers (Basel) 2024;16:3689. [Crossref] [PubMed]
- He M, Zhou X, Wang X. Glycosylation: mechanisms, biological functions and clinical implications. Signal Transduct Target Ther 2024;9:194. [Crossref] [PubMed]
- Bangarh R, Khatana C, Kaur S, et al. Aberrant protein glycosylation: Implications on diagnosis and Immunotherapy. Biotechnol Adv 2023;66:108149. [Crossref] [PubMed]
- Xi X, Yang Y, Chen Q, et al. GnT-V-mediated aberrant N-glycosylation of TIMP-1 promotes diabetic retinopathy progression. Mol Biol Rep 2024;51:428. [Crossref] [PubMed]
- Kim J, Bamlet WR, Oberg AL, et al. Detection of early pancreatic ductal adenocarcinoma with thrombospondin-2 and CA19-9 blood markers. Sci Transl Med 2017;9:eaah5583. [Crossref] [PubMed]
- Xiao H, Woods EC, Vukojicic P, et al. Precision glycocalyx editing as a strategy for cancer immunotherapy. Proc Natl Acad Sci U S A 2016;113:10304-9. [Crossref] [PubMed]
- Jennewein MF, Alter G. The Immunoregulatory Roles of Antibody Glycosylation. Trends Immunol 2017;38:358-72. [Crossref] [PubMed]
- Clough E, Barrett T. The Gene Expression Omnibus Database. Methods Mol Biol 2016;1418:93-110. [Crossref] [PubMed]
- Wang Z, Jensen MA, Zenklusen JC. A Practical Guide to The Cancer Genome Atlas (TCGA). Methods Mol Biol 2016;1418:111-41. [Crossref] [PubMed]
- Liu J, Lichtenberg T, Hoadley KA, et al. An Integrated TCGA Pan-Cancer Clinical Data Resource to Drive High-Quality Survival Outcome Analytics. Cell 2018;173:400-416.e11. [Crossref] [PubMed]
- Bian C, Sun X, Huang J, et al. A novel glycosyltransferase-related lncRNA signature correlates with lung adenocarcinoma prognosis. Front Oncol 2022;12:950783. [Crossref] [PubMed]
- Chen B, Khodadoust MS, Liu CL, et al. Profiling Tumor Infiltrating Immune Cells with CIBERSORT. Methods Mol Biol 2018;1711:243-59. [Crossref] [PubMed]
- Kawahara R, Saad J, Angeli CB, et al. Site-specific characterization of N-linked glycosylation in human urinary glycoproteins and endogenous glycopeptides. Glycoconj J 2016;33:937-51. [Crossref] [PubMed]
- Zhang Y, Zhang Z. The history and advances in cancer immunotherapy: understanding the characteristics of tumor-infiltrating immune cells and their therapeutic implications. Cell Mol Immunol 2020;17:807-21. [Crossref] [PubMed]
- Deng J, Tu S, Li L, et al. Diagnostic, predictive and prognostic molecular biomarkers in clear cell renal cell carcinoma: A retrospective study. Cancer Rep (Hoboken) 2024;7:e2116. [Crossref] [PubMed]
- Zhou Q, Qi Y, Wang Z, et al. CCR5 blockade inflames antitumor immunity in BAP1-mutant clear cell renal cell carcinoma. J Immunother Cancer 2020;8:e000228. [Crossref] [PubMed]
- Wang XM, Lu Y, Song YM, et al. Integrative genomic study of Chinese clear cell renal cell carcinoma reveals features associated with thrombus. Nat Commun 2020;11:739. [Crossref] [PubMed]
- Zhu Q, Wang H, Chai S, et al. O-GlcNAcylation promotes tumor immune evasion by inhibiting PD-L1 lysosomal degradation. Proc Natl Acad Sci U S A 2023;120:e2216796120. [Crossref] [PubMed]
- Purushothaman A, Mohajeri M, Lele TP. The role of glycans in the mechanobiology of cancer. J Biol Chem 2023;299:102935. [Crossref] [PubMed]
- Ma X, Dong W, Su Z, et al. Functional roles of sialylation in breast cancer progression through miR-26a/26b targeting ST8SIA4. Cell Death Dis 2016;7:e2561. [Crossref] [PubMed]
- Soukhtehzari S, Berish RB, Fazli L, et al. The different prognostic significance of polysialic acid and CD56 expression in tumor cells and lymphocytes identified in breast cancer. NPJ Breast Cancer 2022;8:78. [Crossref] [PubMed]
- Li X, Liu D, Wu Z, et al. Diffuse tumors: Molecular determinants shared by different cancer types. Comput Biol Med 2024;178:108703. [Crossref] [PubMed]
- Bennett EP, Mandel U, Clausen H, et al. Control of mucin-type O-glycosylation: a classification of the polypeptide GalNAc-transferase gene family. Glycobiology 2012;22:736-56. [Crossref] [PubMed]
- Kwon OS, Lee H, Kong HJ, et al. Connectivity map-based drug repositioning of bortezomib to reverse the metastatic effect of GALNT14 in lung cancer. Oncogene 2020;39:4567-80. [Crossref] [PubMed]
- Chiang CC, Yeh CT, Hwang TL, et al. The GALNT14 Genotype Predicts Postoperative Outcome of Pancreatic Ductal Adenocarcinoma. J Clin Med 2019;8:2225. [Crossref] [PubMed]
- Liang KH, Yeh TS, Wu RC, et al. GALNT14 genotype is associated with perineural invasion, lymph node metastasis and overall survival in resected cholangiocarcinoma. Oncol Lett 2017;13:4215-23. [Crossref] [PubMed]
- Song KH, Park MS, Nandu TS, et al. GALNT14 promotes lung-specific breast cancer metastasis by modulating self-renewal and interaction with the lung microenvironment. Nat Commun 2016;7:13796. [Crossref] [PubMed]
- Sheta R, Bachvarova M, Plante M, et al. Altered expression of different GalNAc transferases is associated with disease progression and poor prognosis in women with high-grade serous ovarian cancer. Int J Oncol 2017;51:1887-97. [Crossref] [PubMed]
- Brockington M, Torelli S, Prandini P, et al. Localization and functional analysis of the LARGE family of glycosyltransferases: significance for muscular dystrophy. Hum Mol Genet 2005;14:657-65. [Crossref] [PubMed]
- Dietinger V, García de Durango CR, Wiechmann S, et al. Wnt-driven LARGE2 mediates laminin-adhesive O-glycosylation in human colonic epithelial cells and colorectal cancer. Cell Commun Signal 2020;18:102. [Crossref] [PubMed]
- Sun H, Chang J, Ye M, et al. GCNT4 is Associated with Prognosis and Suppress Cell Proliferation in Gastric Cancer. Onco Targets Ther 2020;13:8601-13. [Crossref] [PubMed]
- Wu F, Yuan G, Chen J, et al. Network analysis based on TCGA reveals hub genes in colon cancer. Contemp Oncol (Pozn) 2017;21:136-44. [Crossref] [PubMed]
- Gao W, Gu Y, Li Z, et al. miR-615-5p is epigenetically inactivated and functions as a tumor suppressor in pancreatic ductal adenocarcinoma. Oncogene 2015;34:1629-40. [Crossref] [PubMed]
- Sun Y, Zhang T, Wang C, et al. MiRNA-615-5p functions as a tumor suppressor in pancreatic ductal adenocarcinoma by targeting AKT2. PLoS One 2015;10:e0119783. [Crossref] [PubMed]
- Milde-Langosch K, Karn T, Schmidt M, et al. Prognostic relevance of glycosylation-associated genes in breast cancer. Breast Cancer Res Treat 2014;145:295-305. [Crossref] [PubMed]
- Liu X, Yao J, Zhao Y, et al. Heterogeneous plasma cells and long-lived subsets in response to immunization, autoantigen and microbiota. Nat Immunol 2022;23:1564-76. [Crossref] [PubMed]
- Patil NS, Nabet BY, Müller S, et al. Intratumoral plasma cells predict outcomes to PD-L1 blockade in non-small cell lung cancer. Cancer Cell 2022;40:289-300.e4. [Crossref] [PubMed]
- Li S, Wang X, Wang Q, et al. Effects and Prognostic Values of Circadian Genes CSNK1E/GNA11/KLF9/THRAP3 in Kidney Renal Clear Cell Carcinoma via a Comprehensive Analysis. Bioengineering (Basel) 2022;9:306. [Crossref] [PubMed]
- Wang Y, Wang Y, Xu L, et al. CD4 + T cells promote renal cell carcinoma proliferation via modulating YBX1. Exp Cell Res 2018;363:95-101. [Crossref] [PubMed]
- Han B, Sun Y, Yang D, et al. USP22 promotes development of lung adenocarcinoma through ubiquitination and immunosuppression. Aging (Albany NY) 2020;12:6990-7005. [Crossref] [PubMed]
- Zhu H, Blum RH, Bernareggi D, et al. Metabolic Reprograming via Deletion of CISH in Human iPSC-Derived NK Cells Promotes In Vivo Persistence and Enhances Anti-tumor Activity. Cell Stem Cell 2020;27:224-237.e6. [Crossref] [PubMed]
- Dudeck J, Kotrba J, Immler R, et al. Directional mast cell degranulation of tumor necrosis factor into blood vessels primes neutrophil extravasation. Immunity 2021;54:468-483.e5. [Crossref] [PubMed]
- Terada T, Matsunaga Y. Increased mast cells in hepatocellular carcinoma and intrahepatic cholangiocarcinoma. J Hepatol 2000;33:961-6. [Crossref] [PubMed]
- Ribatti D, Guidolin D, Marzullo A, et al. Mast cells and angiogenesis in gastric carcinoma. Int J Exp Pathol 2010;91:350-6. [Crossref] [PubMed]
- Gulubova M, Vlaykova T. Prognostic significance of mast cell number and microvascular density for the survival of patients with primary colorectal cancer. J Gastroenterol Hepatol 2009;24:1265-75. [Crossref] [PubMed]
- Almutairi SM, Ali AK, He W, et al. Interleukin-18 up-regulates amino acid transporters and facilitates amino acid-induced mTORC1 activation in natural killer cells. J Biol Chem 2019;294:4644-55. [Crossref] [PubMed]
- Zhang L, Jiang C, Zhong Y, et al. STING is a cell-intrinsic metabolic checkpoint restricting aerobic glycolysis by targeting HK2. Nat Cell Biol 2023;25:1208-22. [Crossref] [PubMed]
- Markova L, Maji M, Kostrhunova H, et al. Multiaction Pt(IV) Prodrugs Releasing Cisplatin and Dasatinib Are Potent Anticancer and Anti-Invasive Agents Displaying Synergism between the Two Drugs. J Med Chem 2024;67:9745-58. [Crossref] [PubMed]
- Huang XP, Li X, Situ MY, et al. Entinostat reverses cisplatin resistance in esophageal squamous cell carcinoma via down-regulation of multidrug resistance gene 1. Cancer Lett 2018;414:294-300. [Crossref] [PubMed]
- La Spina d'Anna R. Light and electron microscopic study of unfertilized egg of Ascidia malaca (Tunicata). Acta Embryol Exp (Palermo) 1974;1:3-17. [PubMed]
- Yao H, Lyu F, Ma J, et al. PIMREG is a prognostic biomarker involved in immune microenvironment of clear cell renal cell carcinoma and associated with the transition from G1 phase to S phase. Front Oncol 2023;13:1035321. [Crossref] [PubMed]
- Xu W, Gaborieau V, Niman SM, et al. Plasma Kidney Injury Molecule-1 for Preoperative Prediction of Renal Cell Carcinoma Versus Benign Renal Masses, and Association With Clinical Outcomes. J Clin Oncol 2024;42:2691-701. [Crossref] [PubMed]
- Lee JC, Yotis DM, Lee JY, et al. Kidney injury molecule-1 inhibits metastasis of renal cell carcinoma. Sci Rep 2021;11:11840. [Crossref] [PubMed]
- Tutunea-Fatan E, Arumugarajah S, Suri RS, et al. Sensing Dying Cells in Health and Disease: The Importance of Kidney Injury Molecule-1. J Am Soc Nephrol 2024;35:795-808. [Crossref] [PubMed]
- Meyers JH, Chakravarti S, Schlesinger D, et al. TIM-4 is the ligand for TIM-1, and the TIM-1-TIM-4 interaction regulates T cell proliferation. Nat Immunol 2005;6:455-64. [Crossref] [PubMed]
- Du X, Guo Y, Zhao X, et al. METTL3-mediated TIM1 promotes macrophage M1 polarization and inflammation through IGF2BP2-dependent manner. J Biochem Mol Toxicol 2024;38:e23845. [Crossref] [PubMed]
- Keir ME, Liang SC, Guleria I, et al. Tissue expression of PD-L1 mediates peripheral T cell tolerance. J Exp Med 2006;203:883-95. [Crossref] [PubMed]
- Pardoll DM. The blockade of immune checkpoints in cancer immunotherapy. Nat Rev Cancer 2012;12:252-64. [Crossref] [PubMed]
- Ma Y, Lai J, Wan Q, et al. Exploring the common mechanisms and biomarker ST8SIA4 of atherosclerosis and ankylosing spondylitis through bioinformatics analysis and machine learning. Front Cardiovasc Med 2024;11:1421071. [Crossref] [PubMed]
- Scelo G, Muller DC, Riboli E, et al. KIM-1 as a Blood-Based Marker for Early Detection of Kidney Cancer: A Prospective Nested Case-Control Study. Clin Cancer Res 2018;24:5594-601. [Crossref] [PubMed]