Identification of significant genes with prognostic influence in clear cell renal cell carcinoma via bioinformatics analysis
Introduction
There were about 403,262 new cases of kidney cancer that were diagnosed worldwide in 2018, and 175,098 patients died of this disease (1). Renal cell carcinoma (RCC) is a kind of malignant tumor originating from renal tubular epithelium, which denotes more than 90% of cancers in kidneys (2). RCC accounts for 2% to 3% of adult cancers, ranking the third cause of cancerous death in urologic cancers. And there is a trend of increasing incidence and mortality during these years. RCC consists of a broad spectrum of histopathological types, clear cell renal cell carcinoma (ccRCC) accounts for 80% to 90% of RCC (3,4). Patients with ccRCC have worse prognosis than chromophobe RCC and papillary RCC in a multicenter experience (5). ccRCC has very complicated pathogenesis and various biological features. Surgical treatment is thought to achieve cure outcome effectively in localized RCC currently (3). While 20% to 40% of these patients may develop recurrence with poor prognosis after surgery (6). Advanced or metastatic RCC is not sensitive to chemotherapy and conventional radiotherapy, and the median survival of patients with metastatic disease is about 13 months (7). Researches on this important disease are still urgent. In addition to developing new ways of treatment for ccRCC, it is of great importance to find novel prognostic biomarkers and therapeutic targets.
High throughput sequencing makes it possible to analyze transcriptome and genome quickly and cheaply (8). A huge amount of information is detected and stored in public database. Researchers can make use of existing data to detect differentially expressed genes and dig out valuable information via bioinformatics analysis. Bioinformatics analysis could help us to explore the underlying findings and guide further study. Up to now, few reliable prognostic biomarkers have been identified in ccRCC. In this study, we combined the integrated bioinformatics analysis with expression profiling techniques to solve the issue. We discovered 6 significant genes with prognostic influence in ccRCC, namely ANGPTL4, CA9, CXCR4, LOX, EGF and HRG. High expression of HRG denoted a better prognosis in ccRCC patients, which had not been reported previously. HRG was down-regulated in ccRCC tissues compared with normal kidney tissues via bioinformatics analysis. Our findings may provide a novel prognostic predictor and potential therapeutic target for ccRCC.
Methods
Data information and database analysis
Gene expression profiles of GSE11151, GSE12606, GSE46699 and GSE53757 were obtained from the free public database of Gene Expression Omnibus (GEO, https://www.ncbi.nlm.nih.gov/gds/). All the datasets came from GPL570 platform [(HG-U133_Plus_2) Affymetrix Human Genome U133 Plus 2.0 Array]. There were 26 ccRCC tissues and 5 normal kidney tissues, 3 ccRCC tissues and 3 normal kidney tissues, 67 ccRCC tissues and 63 normal kidney tissues, 72 ccRCC tissues and 72 normal kidney tissues, respectively. DEGs between ccRCC samples and normal kidney samples were identified via GEO2R online according to the standards of |log FC| >2 and adjust P value <0.05. DEGs with log FC >2 were considered as up-regulated genes, and DEGs with log FC <−2 were categorized as down-regulated genes. The raw data was rearranged and analyzed to acquire common DEGs with Venny online software (https://bioinfogp.cnb.csic.es/tools/venny/index.html).
Gene ontology (GO) functional enrichment analysis and signaling pathway analysis
GO is established to construct a standard vocabulary system including biological process (BP), molecular function (MF) and cellular component (CC) (9). DAVID bioinformatics resources website (https://david.ncifcrf.gov/) is a free resource providing a comprehensive set of functional annotation to understand biological meaning behind a large number of genes. We applied DAVID website to dealing with GO functional enrichment analysis and Kyoto Encyclopedia of Genes and Genomes (KEGG) pathway analysis.
Protein-protein interaction (PPI) network construction and cluster analysis
The Search Tool for the Retrieval of Interacting Genes/Proteins (STRING, http://string-db.org/) was logged to perform PPI network construction. We selected “Multiple proteins” and submitted the common DEGs list, then we got a PPI network. We could observe the state of gene enrichment clusters previously. Then we used the open access software platform of Cytoscape to visualize the PPI network. The main clusters and core genes were identified by the Molecular Complex Detection (MCODE) plugin.
Kaplan-Meier Plotter analysis and validation of gene expression
We applied Kaplan-Meier Plotter Database (https://kmplot.com/analysis/) to assessing the effect of core genes on overall survival (OS). Kaplan-Meier Plotter is a website tool with relapse free and OS information based on GEO, European Genome-phenome Archive (EGA) and The Cancer Genome Atlas (TCGA) database, which helps us to analyze the prognostic value of a particular gene. We got the log rank P value and hazard ratio (HR) with 95% confidence intervals in the presented figures. Then we compared the gene expression profiles between ccRCC tissues and normal tissues by the Gene Expression Profiling Interactive Analysis (GEPIA, http://gepia.cancer-pku.cn/) to confirm the significant correlation. GEPIA website contains information of hundreds of ccRCC samples from the Genotype-Tissue Expression (GTEx) project and TCGA database.
Results
Screening of common DEGs
We searched and extracted GSE11151, GSE12606, GSE46699 and GSE53757 from GEO database. The four series were all from GPL570 platform. There were totally 168 ccRCC tissues and 143 normal kidney tissues were included in this study. One hundred and one common DEGs were identified, including 31 up-regulated genes and 70 down-regulated genes (adjust P value <0.05, |log FC| >2) (Figure 1). However, the up-regulated gene item LOC101928916///NNMT and the down-regulated gene item CLCNKB///CLCNKA display one probe corresponding to two genes, we removed them and eventually got 99 common DEGs (Table 1).
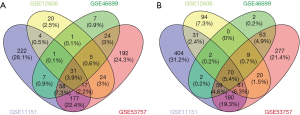
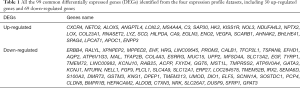
Full table
GO functional enrichment analysis and KEGG pathway analysis
All the 99 common DEGs were input into DAVID website for GO enrichment analysis. The GO functional enrichment analysis showed that for BP the down-regulated DEGs were enriched in excretion, negative regulation of cell proliferation, ion transmembrane transport, positive regulation of extrinsic apoptotic signaling pathway via death domain receptors, cellular response to BMP stimulus, positive regulation of transcription, metanephric distal convoluted tubule development, potassium ion homeostasis, metanephric collecting duct development and regulation of phosphatidylinositol 3-kinase signaling, etc. For CC, down-regulated DEGs were enriched in extracellular exosome, basolateral plasma membrane, apical plasma membrane, integral component of membrane, plasma membrane, integral component of plasma membrane, platelet alpha granule lumen, apical part of cell, vacuolar proton-transporting V-type ATPase complex and extracellular region. For MF, down-regulated DEGs were significantly enriched in ATPase binding, sequence-specific DNA binding, ATP-activated inward rectifier potassium channel activity, heparin binding, phosphatidylinositol-4,5-bisphosphate 3-kinase activity, RNA polymerase II regulatory region sequence-specific DNA binding, transcriptional activator activity, RNA polymerase II transcription regulatory region sequence-specific binding and identical protein binding (Table 2). However, our data showed no significant GO enrichment in up-regulated genes.
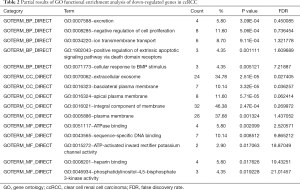
Full table
KEGG pathway analysis indicated that the common DEGs are particularly enriched in Hypoxia-inducible factor-1 (HIF-1) signaling pathway and aldosterone-regulated sodium reabsorption pathway (P<0.05) (Table 3).

Full table
Identification of core genes by PPI network and cluster analysis
The 99 common DEGs were imported into the STRING multiple proteins catalogue, we got a PPI network including 95 nodes and 108 edges (Figure 2). The PPI enrichment P value <1.0e-16, which had significantly more interactions than expected. We could also observe two clusters roughly. Then we made further study with Cytoscape MCODE to analyze the interaction and we got 7 core genes from the main cluster. The 7 core genes were ANGPTL4, CA9, CXCR4, EGF, HRG, LOX, and VEGFA.
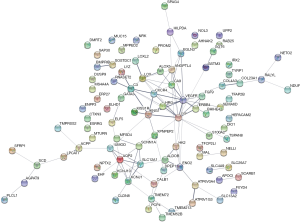
Prognostic analysis of core genes and confirmation of gene expression
Of the 7 core genes, we found that 6 genes have significant effect on prognosis via Kaplan-Meier analysis, namely ANGPTL4, CA9, CXCR4, LOX, EGF and HRG. High expressions of CXCR4 and LOX were significantly correlated with worse survival (Figure 3). Patients had better prognosis when ANGPTL4, CA9, EGF and HRG were highly expressed (P<0.05). Then we applied GEPIA to validating the differential expression level of the 6 genes. ANGPTL4, CA9, CXCR4 and LOX were highly expressed in ccRCC tissues compared with normal kidney tissues, while EGF and HRG were low expressed in ccRCC tissues (Figure 4). Moreover, GO functional enrichment analysis showed that HRG played important roles in negative regulation of cell proliferation, extracellular exosome, platelet alpha granule lumen and heparin binding.
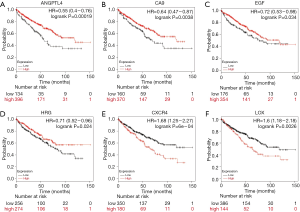
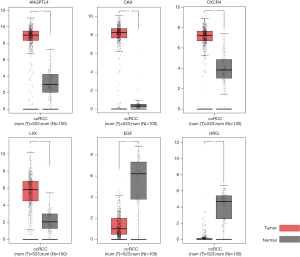
Discussion
ccRCC is the most prevalent RCC in the world. Patients with ccRCC have worse prognosis compared with other RCC subtypes such as papillary RCC and chromophobe RCC. The cancer specific survival rates at 5 years of ccRCC patients range from 44% to 69% (10,11). In spite of the increasing utilization of imaging techniques making more and more patients diagnosed in early stages, there are still about 20% to 30% of all patients diagnosed with advanced stages (12). More than 30% of patients who undergo nephrectomy will relapse. Median survival of patients with advanced RCC is 18 to 24 months (13). The vast majority of cancer associated death (about 90%) is owning to metastatic disease rather than primary tumor (14). ccRCC has a variety of biological behaviors. It is notorious for the ability to metastasize to various unusual sites and characteristic late recurrence, which confuse doctors and exacerbate the poor outcome of patients (15). VHL, PBRM1, TCEB1 and PIK3CA mutations are related to HIF pathway and PI3K pathway which proved to be very important in the oncogenesis of ccRCC (13). However, there is no uniform and definite biomarkers to predict prognosis of ccRCC.
In this study, we applied bioinformatics methods to data analysis on the basis of four profile datasets (GSE11151, GSE12606, GSE46699 and GSE53757) from GEO database. 168 ccRCC specimens and 143 normal kidney specimens were involved in this study. We screened out a total of 99 common DEGs (adjust P value <0.05 and |log FC| >2), including 30 up-regulated (log FC >2) and 69 down-regulated DEGs (log FC <−2). Then we used DAVID website tools to perform GO enrichment analysis and KEGG pathway analysis. The outcome showed that the down-regulated DEGs were enriched in BP, CC and MF such as excretion, negative regulation of cell proliferation, positive regulation of extrinsic apoptotic signaling pathway via death domain receptors, cellular response to BMP stimulus, positive regulation of transcription, exosome, basolateral plasma membrane, sequence-specific DNA binding, heparin binding, identical protein binding and so on. Roles of common DEGs in carcinogenesis and tumor suppression of ccRCC could be investigated according to our results. For KEGG pathway analysis, DEGs were particularly enriched in HIF-1 signaling pathway and aldosterone-regulated sodium reabsorption (P<0.05). HIF-1 is a kind of oxygen-sensitive transcriptional activator. The outcome is in agreement with former researches about HIF target genes playing important roles in driving renal carcinogenesis (13).
We constructed PPI network consisting of 95 nodes and 108 edges via the STRING online database. Next, we got 7 core genes through clusters analysis by MCODE plugin of Cytoscape software. Furthermore, we screened out 6 genes from the 7 core genes through Kaplan-Meier plotter analysis. Differential expression of the 6 genes showed significant difference in patients’ prognosis. All the 6 genes were validated to be differentially expressed in ccRCC samples compared with normal kidney samples by GEPIA analysis (P<0.05). Of which several genes have been demonstrated to be of great importance in oncogenesis or tumor suppression. Interestingly, existing researches have proved the importance of ANGPTL4, CA9, CXCR4, LOX and EGF in ccRCC (16-20). But few attentions have been paid to that increased level of HRG expression can impact the prognosis of patients who have ccRCC indicating that HRG could be used as a predictor of prognosis. The roles of HRG in ccRCC have not been investigated.
Histidine-rich glycoprotein (HRG) is a plasma protein consisting of 507 amino acid residues (21). It shows a multidomain structure binding to immunoglobulins, heparin, heparan sulfates, thrombospondin, fibrinogen, plasminogen, divalent metal ions and other complement proteins, which enables HRG to interacting with a variety of cells (22). But the definite cell surface receptors are still unclear. HRG is relatively abundant in healthy liver and is less prominent in vessels and macrophages (23). It has been investigated in mice and tumor cell lines that HRG inhibits the tumor growth of T241 fibrosarcomas, orthotopic pancreatic tumors and breast tumor lines. HRG may reduce metastasis and improve chemotherapy through promoting vessel normalization. HRG also affects tumor associated macrophage polarization and other antitumor immune response (24). Another research on mice indicated that HRG may be a potential tumor suppressor, which regulated platelet activity to attenuate the angiogenic switch, tumor growth, epithelial-mesenchymal transition and subsequent metastatic spread (25). According to present researches, HRG takes part in several physiological and pathological processes by modulating the immune, vascular and coagulation systems (21,26,27). Our GO functional enrichment analysis showed that HRG is enriched in negative regulation of cell proliferation, extracellular exosome, platelet alpha granule lumen and heparin binding. HRG seemed to be an important component during the process of negative regulation of cell proliferation to inhibit cancer progression.
In previous studies, researchers tried to discover a perfect biomarker for ccRCC. For example, Hansson et al. found that ccRCC expressed very high transcript level of the dopamine transporter SLC6A3 and was influenced by HIF-2a. They recommended SLC6A3 as a novel biomarker for diagnosis and therapy (28). Xu et al. reported that CXCL13 was highly expressed in ccRCC tissues and the high expression could predict poor survival (29). Another research drew a conclusion that high expression of SLC1A5 is associated with poor prognosis in ccRCC (30). As sequencing databases expand quickly, numerous molecular markers have been identified and investigated via bioinformatics analysis. Song et al. also identified PTPRC, TGFB1, EGF, MYC, ITGB2, CTSS, FN1, CCL5, KNG1, and CD86 as potential crucial genes associated with carcinogenesis of ccRCC (31). Wu et al. viewed VEGFA, PPARA, CCND1, FLT1, CXCL12, FN1, DCN and ERBB4 as biomarkers of ccRCC (32). Wang et al. found EGFR may be a potential prognostic biomarker for ccRCC (33). Chen et al. studied FCER1G and thought it was in association with ccRCC progression and prognosis (34). Xiao et al. screened out three hub genes (EHHADH, ACADM and AGXT2) as novel tumor suppressors in ccRCC (35). Some biomarkers are common among different researches such as TOP2A, VEGFA and EGFR (36,37). However, there is not an optimal prognostic predictor that has been recommended for patients with ccRCC in clinical practice (3). As one prognostic factor is not accurate enough, multivariable prognostic models is put forward to predict prognosis. And prognostic models and nomograms provide better predictive accuracy than a single independent predictor (38). A novel biomarker can be utilized as not only an independent predictor, but also a part of new prognostic models to improve predictive accuracy. The investigation of the mechanism of a biomarker may provide potential therapeutic targets to treat ccRCC.
Considering the lack of experimental research on HRG in ccRCC, this study is limited. However, we totally identified 99 common DEGs of ccRCC. And importantly, we found that HRG may play important roles in ccRCC. Our study can be considered as a preliminary study for future related researches.
Conclusions
In summary, our bioinformatics analysis identified ANGPTL4, CA9, CXCR4, LOX, EGF and HRG that were expressed differentially between ccRCC tissues and normal kidney tissues on the basis of GEO database. And all of the 6 genes showed significant prognostic influence in patients with ccRCC. HRG was associated with ccRCC that had not been previously reported. In our study, HRG was regarded as an important common differentially expressed gene of ccRCC and could be a novel prognostic predictor. HRG could play an important role in tumor suppression, the underlying mechanism would be verified by further study.
Acknowledgments
Funding: None.
Footnote
Conflicts of Interest: All authors have completed the ICMJE uniform disclosure form (available at http://dx.doi.org/10.21037/tau.2020.02.11). The authors have no conflicts of interest to declare.
Ethical Statement: The authors are accountable for all aspects of the work in ensuring that questions related to the accuracy or integrity of any part of the work are appropriately investigated and resolved.
Open Access Statement: This is an Open Access article distributed in accordance with the Creative Commons Attribution-NonCommercial-NoDerivs 4.0 International License (CC BY-NC-ND 4.0), which permits the non-commercial replication and distribution of the article with the strict proviso that no changes or edits are made and the original work is properly cited (including links to both the formal publication through the relevant DOI and the license). See: https://creativecommons.org/licenses/by-nc-nd/4.0/.
References
- Bray F, Ferlay J, Soerjomataram I, et al. Global cancer statistics 2018: GLOBOCAN estimates of incidence and mortality worldwide for 36 cancers in 185 countries. CA Cancer J Clin 2018;68:394-424. [Crossref] [PubMed]
- Hsieh JJ, Purdue MP, Signoretti S, et al. Renal cell carcinoma. Nature reviews Disease primers 2017;3:17009. [Crossref] [PubMed]
- Ljungberg B, Albiges L, Abu-Ghanem Y, et al. European Association of Urology Guidelines on Renal Cell Carcinoma: The 2019 Update. Eur Urol 2019;75:799-810. [Crossref] [PubMed]
- Moch H, Cubilla AL, Humphrey PA, et al. The 2016 WHO Classification of Tumours of the Urinary System and Male Genital Organs—Part A: Renal, Penile, and Testicular Tumours. Eur Urol 2016;70:93-105. [Crossref] [PubMed]
- Patard JJ, Leray E, Rioux-Leclercq N, et al. Prognostic value of histologic subtypes in renal cell carcinoma: a multicenter experience. J Clin Oncol 2005;23:2763-71. [Crossref] [PubMed]
- Brookman-May SD, May M, Shariat SF, et al. Time to recurrence is a significant predictor of cancer-specific survival after recurrence in patients with recurrent renal cell carcinoma--results from a comprehensive multi-centre database (CORONA/SATURN-Project). BJU Int 2013;112:909-16. [PubMed]
- Cohen HT, McGovern FJ. Renal-cell carcinoma. N Engl J Med 2005;353:2477-90. [Crossref] [PubMed]
- Metzker ML. Sequencing technologies — the next generation. Nat Rev Genet 2010;11:31. [Crossref] [PubMed]
- Ashburner M, Ball CA, Blake JA, et al. Gene Ontology: tool for the unification of biology. Nature Genetics 2000;25:25-9. [Crossref] [PubMed]
- Young JR, Coy H, Douek M, et al. Clear cell renal cell carcinoma: identifying the gain of chromosome 12 on multiphasic MDCT. Abdom Radiol (NY) 2017;42:236-41. [Crossref] [PubMed]
- Cheville JC, Lohse CM, Zincke H, et al. Comparisons of outcome and prognostic features among histologic subtypes of renal cell carcinoma. Am J Surg Pathol 2003;27:612-24. [Crossref] [PubMed]
- Ljungberg B, Campbell SC, Cho HY, et al. The Epidemiology of Renal Cell Carcinoma. Eur Urol 2011;60:615-21. [Crossref] [PubMed]
- Ricketts CJ, Crooks DR, Sourbier C, et al. SnapShot: Renal Cell Carcinoma. Cancer Cell 2016;29:610-.e1.
- Lambert AW, Pattabiraman DR, Weinberg RA. Emerging Biological Principles of Metastasis. Cell 2017;168:670-91. [Crossref] [PubMed]
- Zhang F, Zhao G, Wu P, et al. Asynchronous abdominal wall and sigmoid metastases in clear cell renal cell carcinoma: A case report and literature review. Asian Journal of Urology 2019;6:210-4. [Crossref] [PubMed]
- Verine J, Lehmann-Che J, Soliman H, et al. Determination of angptl4 mRNA as a diagnostic marker of primary and metastatic clear cell renal-cell carcinoma. PLoS One 2010;5:e10421. [Crossref] [PubMed]
- de Martino M, Klatte T, Seligson DB, et al. CA9 gene: single nucleotide polymorphism predicts metastatic renal cell carcinoma prognosis. J Urol 2009;182:728-34. [Crossref] [PubMed]
- Ieranò C, Santagata S, Napolitano M, et al. CXCR4 and CXCR7 transduce through mTOR in human renal cancer cells. Cell Death Dis 2014;5:e1310. [Crossref] [PubMed]
- Matsuyama M, Yoshimura R, Tsuchida K, et al. Lipoxygenase inhibitors prevent urological cancer cell growth. Int J Mol Med 2004;13:665-8. [PubMed]
- Dorđević G, Matusan Ilijas K, Hadzisejdic I, et al. EGFR protein overexpression correlates with chromosome 7 polysomy and poor prognostic parameters in clear cell renal cell carcinoma. J Biomed Sci 2012;19:40. [Crossref] [PubMed]
- Blank M, Shoenfeld Y. Histidine-rich glycoprotein modulation of immune/autoimmune, vascular, and coagulation systems. Clin Rev Allergy Immunol 2008;34:307-12. [Crossref] [PubMed]
- Jones AL, Hulett MD, Parish CR. Histidine-rich glycoprotein: A novel adaptor protein in plasma that modulates the immune, vascular and coagulation systems. Immunol Cell Biol 2005;83:106-18. [Crossref] [PubMed]
- Hulett MD, Parish CR. Murine histidine-rich glycoprotein: cloning, characterization and cellular origin. Immunol Cell Biol 2000;78:280-7. [Crossref] [PubMed]
- Rolny C, Mazzone M, Tugues S, et al. HRG inhibits tumor growth and metastasis by inducing macrophage polarization and vessel normalization through downregulation of PlGF. Cancer Cell 2011;19:31-44. [Crossref] [PubMed]
- Cedervall J, Zhang Y, Ringvall M, et al. HRG regulates tumor progression, epithelial to mesenchymal transition and metastasis via platelet-induced signaling in the pre-tumorigenic microenvironment. Angiogenesis 2013;16:889-902. [Crossref] [PubMed]
- Dixelius J, Olsson AK, Thulin A, et al. Minimal active domain and mechanism of action of the angiogenesis inhibitor histidine-rich glycoprotein. Cancer Res 2006;66:2089-97. [Crossref] [PubMed]
- Klenotic PA, Huang P, Palomo J, et al. Histidine-rich glycoprotein modulates the anti-angiogenic effects of vasculostatin. Am J Pathol 2010;176:2039-50. [Crossref] [PubMed]
- Hansson J, Lindgren D, Nilsson H, et al. Overexpression of Functional SLC6A3 in Clear Cell Renal Cell Carcinoma. Clin Cancer Res 2017;23:2105-15. [Crossref] [PubMed]
- Xu T, Ruan H, Song Z, et al. Identification of CXCL13 as a potential biomarker in clear cell renal cell carcinoma via comprehensive bioinformatics analysis. Biomed Pharmacother 2019;118:109264. [Crossref] [PubMed]
- Liu Y, Yang L, An H, et al. High expression of Solute Carrier Family 1, member 5 (SLC1A5) is associated with poor prognosis in clear-cell renal cell carcinoma. Sci Rep 2015;5:16954. [Crossref] [PubMed]
- Song E, Song W, Ren M, et al. Identification of potential crucial genes associated with carcinogenesis of clear cell renal cell carcinoma. Journal of cellular biochemistry 2018;119:5163-74. [Crossref] [PubMed]
- Wu F, Wu S, Gou X. Identification of biomarkers and potential molecular mechanisms of clear cell renal cell carcinoma. Neoplasma 2018;65:242-52. [Crossref] [PubMed]
- Wang S, Yu Z-H, Chai K-Q. Identification of EGFR as a Novel Key Gene in Clear Cell Renal Cell Carcinoma (ccRCC) through Bioinformatics Analysis and Meta-Analysis. Biomed Res Int 2019;2019:6480865. [PubMed]
- Chen L, Yuan L, Wang Y, et al. Co-expression network analysis identified FCER1G in association with progression and prognosis in human clear cell renal cell carcinoma. Int J Biol Sci 2017;13:1361-72. [Crossref] [PubMed]
- Xiao H, Chen P, Zeng G, et al. Three novel hub genes and their clinical significance in clear cell renal cell carcinoma. J Cancer 2019;10:6779-91. [Crossref] [PubMed]
- Xiong Y, Yuan L, Chen L, et al. Identifying a Novel Biomarker TOP2A of Clear Cell Renal Cell Carcinoma (ccRCC) Associated with Smoking by Co-Expression Network Analysis. J Cancer 2018;9:3912-22. [Crossref] [PubMed]
- Yuan L, Zeng G, Chen L, et al. Identification of key genes and pathways in human clear cell renal cell carcinoma (ccRCC) by co-expression analysis. Int J Biol Sci 2018;14:266-79. [Crossref] [PubMed]
- Klatte T, Rossi SH, Stewart GD. Prognostic factors and prognostic models for renal cell carcinoma: a literature review. World J Urol 2018;36:1943-52. [Crossref] [PubMed]