Risk stratification tools in prostate cancer, where do we stand?
Pre-treatment risk stratification tools—the end of an era?
In an attempt to predict outcomes and individualize care for prostate cancer (PCa) patients, parallels are drawn between a patient’s current health status and similar cases in the past. To adopt this in clinical practice, risk stratification tools are frequently used. These are, in essence, equations relating multiple factors for a particular individual to the probability or risk for future occurrence of particular outcomes in a certain time period. The terms prognostic and predictive are frequently misunderstood, especially in the field of biomarkers and precision medicine. When a possible interaction (e.g., an intervention) is taken into account, a measurement can be called predictive because it is associated with the impact of a specific therapy. A control group is always needed to evaluate the interaction between treatment benefit and a biomarker or clinical factor (1). Prognostic factors on the other hand provide information on outcomes regardless of any therapeutic intervention (1,2). A biomarker panel can, for example, assign a tumor’s aggressiveness regardless of the treatment that will be provided, where a predictive test could identify which patients will benefit from radiotherapy after biochemical recurrence post-surgery.
The main goal of risk stratification tools is to answer how lethal prostate cancer is, with and without therapy, and how different therapies influence survival outcomes. Most models use similar predictor variables as originally used by D’Amico to differentiate between risk groups: prostate-specific antigen (PSA) level, clinical T-stage, and biopsy Gleason score (3). Adding more variables generally provides more granularity but also increases complexity and impairs usability, as seen in more recent risk scores and nomograms (4-7).
Due to the abundancy of available pre-treatment tools, it can be hard for clinicians and patients to select the appropriate instrument. Zelic et al. recently reported on a head-to-head comparison of nine widely used pretreatment risk stratification tools predicting PCa death and measured their individual performance within the prospectively collected Swedish prostate cancer database (PCBaSe) (8). They found that the Memorial Sloan Kettering Cancer Center (MSKCC) nomogram, Cancer of the Prostate Risk Assessment (CAPRA) score and Cambridge Prognostic Groups (CPG) showed better performance in predicting prostate cancer death than D’Amico risk stratification system and derived tool. However, a great effort is done by the team of Zelic, there are some additional key factors to consider when comparing risk models.
Study population and origin of data
Ideally, the development of risk stratification tools is based on data of a prospective longitudinal cohort study. Information on received diagnostic and staging examinations and treatment modalities should be readily available and indicates generalizability and usefulness of the model. The medical field is continuously evolving and patients recruited a long time ago may have undergone different treatments than recommended by current guidelines. For example, primary androgen deprivation therapy (ADT) monotherapy for high-risk PCa is no longer considered as a contemporary treatment strategy and nowadays magnetic resonance imaging prior to biopsies is advised. In the Swedish database, the cohort was stratified by treatment and year of diagnosis to tackle this issue when calculating concordance indices (9).
The tools themselves, however, are developed in selected cohorts treated in different time frames. The D’Amico risk groups are based on localized PCa patients who underwent definitive local therapy prior to 1998, thus without accounting for deferred treatment strategies such as active surveillance or watchful waiting (3). This points out the need for exhaustive external validation in different cohorts to confirm the generalizability of the predicted risks.
Predicted outcome
The principal aim is to predict outcomes that are clinically relevant for patients such as survival, morbidity or quality of life measurements. When developing prediction models, ideally the outcome should be assessed while blinded to information about the predictor variables. Otherwise, this knowledge may influence outcome assessment which can lead to a biased estimation of the association between predictor and outcome. This is particularly important for outcomes requiring interpretation, such as PCa specific mortality, radiologic imaging or pathology (5). Pathologists, for example, should be blinded for PIRADS scores when scoring biopsies to assess the predictive power of pre-biopsy MRI.
Given the high 5-year survival rate for localized PCa, without substantial evidence for superiority of one treatment over another, the CEASAR study group opted to investigate post-treatment functional outcomes based on patient reported outcomes (10). Recently they published a web-based tool to predict functional outcomes after treatment, based on pretreatment characteristics (11,12). Within the online PREDICT prostate tool, findings from this study are also incorporated, however without adapting for pretreatment functional scores (13).
D’Amico risk groups and other derived tools were developed to predict surrogate endpoints such as biochemical recurrence, which do not always have a clear causal relation to relevant patient outcomes (3,6). The CAPRA score predict probability of disease-free survival (which is based on observed disease recurrence rates, i.e., confirmed PSA raise >0.2 ng/mL or additional treatment), only in patients for whom a radical prostatectomy is planned (14). However, even though not developed for this outcome, D’Amico model and the CAPRA score have later been correlated with Cancer Specific Survival (CSS) (15,16).
Crucially, in prediction studies, the effective sample size is determined by the observed outcome events. In the Swedish database, median follow-up time was only 5.8 years, which is relatively short to evaluate PCa specific death (8). A large sample size may be inadequate if only few outcome events are observed.
Predictors
Candidate predictors are typically obtained from patient demographics, medical history, physical examination, disease characteristics and test results (14). Registries contain a large number of potential predictors, but this leads to a higher probability of including weak or useless predictors in the model, overfitting and less user-friendly models (17).
Since the availability and adoption of multi-parametric magnetic resonance imaging (mpMRI) in PCa evaluation, a new generation of risk calculators incorporating mpMRI data have been introduced. Recently, Mortezavi compared conventional, image and biomarker-based risk calculators and concluded that MRI-based predictions outperform conventional models with clinical parameters only in terms of performance (discrimination and calibration) and net benefits (summing up true-positive biopsies and subtracting false-positive biopsies weighted by a factor related to the relative harm of a missed cancer versus an unnecessary biopsy) to distinguish clinical significant PCa (9).
Moreover, prognostic models may focus on a cohort of patients who are yet to receive any kind of treatment and where treatment can be included as a potential prognostic factor, as seen with the PREDICT prostate model (5). However, caution is needed when including treatment as a prognostic factor because administration of treatment should be standardized to prevent bias of confounding by indication and the predictive impact of treatment is generally small compared to other predictive variables (14).
In Table 1 a subset of the criteria for Transparent reporting of a multivariable prediction model for individual prognosis or diagnosis (TRIPOD) (17) is used to summarize key characteristics of the three best performing tools according to Zelic et al., compared with the more recent PREDICT prostate and D’Amico risk classification. Each of these tools use at least the same predictor variables as described by D’Amico, but additionally there are some differences. All, except for D’Amico differentiate between primary and secondary Gleason grade thus discriminating between 3+4 versus 4+3. CAPRA, MSKCC and PREDICT prostate also account for percentage of positive biopsies as surrogate for tumor volume. This variable is optional for the latter two models, but it is necessary to calculate CAPRA scores. Therefore, CAPRA scores can only be calculated when 6 or more biopsies have been obtained, which was contemporary practice before the introduction of targeted biopsies (5,6). Consequently, caution is advised when calculating CAPRA scores with modern biopsy techniques.
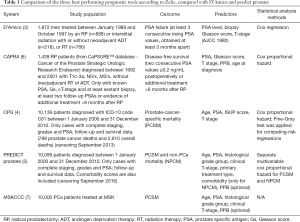
Full table
PREDICT prostate is the only model that includes comorbidities as a predictive variable, but the predictive effect was only seen in relation to non-prostate cancer specific death (18). A model that incorporates a holistic view of the patients and predicts OS instead of CSS is obviously of greater interest for patients and clinicians.
Model specification
Deciding the appropriate treatment modality is often influenced by survival predictions. Guidelines recommend radical prostatectomy only in patients with few comorbidities and a >10 years life expectancy (19). This implies low PCa specific mortality and consequently, other causes of death may occur prior to death of prostate cancer (e.g., death of heart failure can precede prostate cancer specific mortality). These competing risk events can influence the supposed benefit of active treatment and consequently influence the decision process (20). A standard Cox proportional hazard model leads to an increased risk prediction compared to a Fine and Gray model due to the lack of accounting for competing risks, which is especially important in an elderly population (21). Thus, it is important to acknowledge the effect of a standard Cox model developed within a radical prostatectomy population, in which patients de facto have a better health status, when generalizing it to a newly diagnosed PCa population with their typical competing risks.
Machine learning (ML) predictions requires a paradigm shift
When using prediction tools, clinicians often fill out all the necessary variables in an online calculator or paper based nomogram or they select the corresponding risk group. Crucial to widespread adoption is the usability. Overall, compact models with few variables are more frequently used (17). Besides usability, utility plays an important role and can be achieved when predictions can be adequately interpreted and translated into clinical practice.
A multivariable approach also enables researchers to investigate and quantify whether new (bio)markers or investigations have an added predictive value over traditionally used clinic-pathological variables (14). Notice that a large dataset is necessary to provide evidence for significant added value of a test. But if this new test is not incorporated in prognostic models, its adoption might be hampered because the perception might live that there is not enough predictive value in it and consequently no evidence of significant added predictive value can be generated.
A prognostic model might be used to provide insight into causality of the predictor variable and the studied outcome, however this is not equivalent to etiological research. The latter has a goal to explain, whether an outcome can be attributed to a risk factor, with adjustment for confounding factors (14). In a prognostic study, all variables which are potentially associated with the outcome, not necessarily causally, can be considered. All causal factors are predictors but not every predictor is a cause (14). PSA, for example is an example of a predictive but non-causal factor for disease recurrence. This is of importance since both prognostic and etiological research are based on similar design and analysis. Conversely, etiological research, uses statistical inference to characterize the relations between the data and the outcome variable. Often, these two go hand-in-hand since causality and the understanding of underlying drivers of disease are of specific interest to develop new therapeutic strategies. Figure 1 shows the relationship between inferential and predictive statistics.
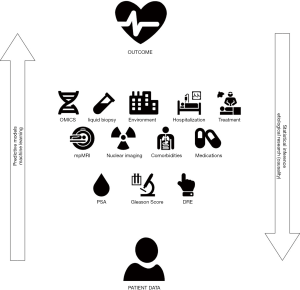
In a visionary commentary by Vickers the question is raised “why can’t nomograms be more like Netflix?” (22). They describe four major advantages of the online streaming platform Netflix-like algorithms compared to traditional nomograms and make the bold statement that medical prediction can be done using technologically superior methods.
Traditional risk prediction tools are based on regression analyses of manually acquired data. ML on the other hand is a form of artificial intelligence (AI) and refers to computer systems that learn from raw data with some degree of autonomy (23). The weaknesses of traditional approaches may be overcome by directly incorporating electronic health record (EHR) data into ML models to augment decision making trough different phases (23).
Multiple challenges, some similar as seen in traditional prediction model development, are blocking today’s adoption of ML techniques. Data standardization, model interpretability, implementation and monitoring as well as ethical issues must be carefully considered (23).
A real paradigm shift would happen if we move away from predictions based on relatively small data obtained by “manual” observations to large amounts of data produced by high-throughput instruments analyzed by ML “black box” models that might produce more accurate predictions. ML models are often perceived as black boxes since the algorithm implicitly learned the features, possibly unknown for physicians, that were most predictive based on the data (24).
Regardless of how the model is built, it should be externally validated the clinical effectiveness must be assessed (25). The TRIPOD steering group together with a large group of experts is developing guidelines for prediction models that are based on AI or ML which will contribute to improvements in the design and reporting of such studies (26).
Conclusions
A holistic view of PCa patients together with an integrated approach of the tumor combining advanced diagnostics and treatment strategies will enable more accurate and relevant predictions. Newer models, such as PREDICT prostate, incorporate already comorbidities and calculate the effect of radical versus conservative treatment, and enable the adoption of additional factors if proven prognostic. However, it is arguable that complex concepts as comorbidities can hardly be summarized in one binary variable. Further, the settings in which therapy will be delivered cannot be underestimated. Surgeon’s experience, hospital volume and a dedicated team are already shown to have an impact on surgical patient’s outcomes (27). It becomes clear that the abundance of data generated combined with ongoing insights and novel technologies calls for a change in the methodology of predicting outcomes that matters for PCa patients, with overall survival as a top priority.
As long as ML models are still under development, the uro-oncological community may be helped by analyses as done by Zelic et al. to choose a suitable model for predicting PCa patient’s survival.
Acknowledgments
Funding: None.
Footnote
Provenance and Peer Review: This article was commissioned by the editorial office, Translational Andrology and Urology. The article did not undergo external peer review.
Conflicts of Interest: All authors have completed the ICMJE uniform disclosure form (available at http://dx.doi.org/10.21037/tau-20-1211). The authors have no conflicts of interest to declare.
Ethical Statement: The authors are accountable for all aspects of the work in ensuring that questions related to the accuracy or integrity of any part of the work are appropriately investigated and resolved.
Open Access Statement: This is an Open Access article distributed in accordance with the Creative Commons Attribution-NonCommercial-NoDerivs 4.0 International License (CC BY-NC-ND 4.0), which permits the non-commercial replication and distribution of the article with the strict proviso that no changes or edits are made and the original work is properly cited (including links to both the formal publication through the relevant DOI and the license). See: https://creativecommons.org/licenses/by-nc-nd/4.0/.
References
- Clark GM. Prognostic factors versus predictive factors: Examples from a clinical trial of erlotinib. Mol Oncol 2008;1:406-12. [Crossref] [PubMed]
- McKay RR, Feng FY, Wang AY, et al. Recent Advances in the Management of High-Risk Localized Prostate Cancer: Local Therapy, Systemic Therapy, and Biomarkers to Guide Treatment Decisions. Am Soc Clin Oncol Educ B 2020;(40):1-12.
- D’Amico AV, Whittington R, Bruce Malkowicz S, et al. Biochemical outcome after radical prostatectomy, external beam radiation therapy, or interstitial radiation therapy for clinically localized prostate cancer. JAMA 1998;280:969-74. [Crossref] [PubMed]
- Gnanapragasam VJ, Lophatananon A, Wright KA, et al. Improving Clinical Risk Stratification at Diagnosis in Primary Prostate Cancer: A Prognostic Modelling Study. Beck AH, editor. PLoS Med 2016;13:e1002063.
- Thurtle DR, Greenberg DC, Lee LS, et al. Individual prognosis at diagnosis in nonmetastatic prostate cancer: Development and external validation of the PREDICT Prostate multivariable model. Johnstone RW, editor. PLOS Med 2019;16:e1002758.
- Cooperberg MR, Pasta DJ, Elkin EP, et al. The University of California, San Francisco Cancer of the Prostate Risk Assessment score: A straightforward and reliable preoperative predictor of disease recurrence after radical prostatectomy. J Urol 2005;173:1938-42. [Crossref] [PubMed]
- Prostate Cancer Nomograms | Memorial Sloan Kettering Cancer Center [Internet]. [cited 2020 Jul 29]. Available online: https://www.mskcc.org/nomograms/prostate
- Zelic R, Garmo H, Zugna D, et al. Predicting Prostate Cancer Death with Different Pretreatment Risk Stratification Tools: A Head-to-head Comparison in a Nationwide Cohort Study. Eur Urol 2020;77:180-8. [Crossref] [PubMed]
- Mortezavi A, Palsdottir T, Eklund M, et al. Head-to-head Comparison of Conventional, and Image- and Biomarker-based Prostate Cancer Risk Calculators. Eur Urol Focus 2020. Available online: http://www.eu-focus.europeanurology.com/article/S2405456920301139/fulltext
- Barocas DA, Chen V, Cooperberg M, et al. Using a population-based observational cohort study to address difficult comparative effectiveness research questions: The CEASAR study. J Comp Eff Res 2013;2:445-60. [Crossref] [PubMed]
- Hoffman KE, Penson DF, Zhao Z, et al. Patient-Reported Outcomes Through 5 Years for Active Surveillance, Surgery, Brachytherapy, or External Beam Radiation With or Without Androgen Deprivation Therapy for Localized Prostate Cancer. JAMA 2020;323:149. [Crossref] [PubMed]
- Laviana AA, Zhao Z, Huang LC, et al. Development and Internal Validation of a Web-based Tool to Predict Sexual, Urinary, and Bowel Function Longitudinally After Radiation Therapy, Surgery, or Observation. Eur Urol 2020;78:248-55. [Crossref] [PubMed]
- Predict Prostate [Internet]. [cited 2020 Jul 30]. Available online: https://prostate.predict.nhs.uk/tool
- Moons KGM, Royston P, Vergouwe Y, et al. Prognosis and prognostic research: what, why, and how? BMJ 2009;338:b375. [Crossref] [PubMed]
- Boorjian SA, Karnes RJ, Rangel LJ, et al. Mayo Clinic Validation of the D’Amico Risk Group Classification for Predicting Survival Following Radical Prostatectomy. J Urol 2008;179:1354-60. [Crossref] [PubMed]
- Vainshtein JM, Schipper M, Vance S, et al. Limitations of the cancer of the prostate risk assessment (CAPRA) prognostic tool for prediction of metastases and prostate cancer-specific mortality in patients treated with external beam radiation therapy. Am J Clin Oncol 2016;39:173-80. [Crossref] [PubMed]
- Moons KGM, Altman DG, Reitsma JB, et al. Transparent reporting of a multivariable prediction model for individual prognosis or diagnosis (TRIPOD): Explanation and elaboration. Ann Intern Med 2015;162:W1-73. [Crossref] [PubMed]
- Thurtle D, Bratt O, Stattin P, et al. External validation of the PREDICT Prostate tool for prognostication in non-metastatic prostate cancer: A study in 69,206 men from prostate cancer data base Sweden. Eur Urol Suppl 2019;18:e221. [Crossref]
- Mottet N, Bellmunt J, Bolla M, et al. EAU-ESTRO-SIOG Guidelines on Prostate Cancer. Part 1: Screening, Diagnosis, and Local Treatment with Curative Intent. Eur Urol 2017;71:618-29. [Crossref] [PubMed]
- Austin PC, Lee DS, Fine JP. Introduction to the Analysis of Survival Data in the Presence of Competing Risks. Circulation 2016;133:601-9. [Crossref] [PubMed]
- Wolbers M, Koller MT, Witteman JCM, et al. Prognostic models with competing risks methods and application to coronary risk prediction. Epidemiology 2009;20:555-61. [Crossref] [PubMed]
- Vickers AJ, Fearn P, Scardino PT, et al. Why Can’t Nomograms Be More Like Netflix? Urology 2010;75:511-3. [Crossref] [PubMed]
- Loftus TJ, Tighe PJ, Filiberto AC, et al. Artificial Intelligence and Surgical Decision-making. JAMA Surg 2020;155:148. [Crossref] [PubMed]
- Bihorac A, Ozrazgat-Baslanti T, Ebadi A, et al. MySurgeryRisk: Development and Validation of a Machine-learning Risk Algorithm for Major Complications and Death After Surgery. Ann Surg 2019;269:652-62. [Crossref] [PubMed]
- Liu Y, Chen PC, Krause J, et al. How to Read Articles That Use Machine Learning: Users' Guides to the Medical Literature. JAMA 2019;322:1806-16. [Crossref] [PubMed]
- Collins GS, Moons KGM. Reporting of artificial intelligence prediction models. Lancet 2019;393:1577-9. [Crossref] [PubMed]
- Williams SB, Ray-Zack MD, Hudgins HK, et al. Impact of Centralizing Care for Genitourinary Malignancies to High-volume Providers: A Systematic Review. Eur Urol Oncol 2019;2:265-73. [Crossref] [PubMed]