Bioinformatic gene analysis for possible biomarkers and therapeutic targets of hypertension-related renal cell carcinoma
Introduction
Renal cell carcinoma (RCC) is a common subtype of malignant tumor in the urinary system. Its incidence rate was 11.281 per 100,000 person-years. The incidence of RCC in men is higher than that in women (1). Around the world, the incidence of RCC is higher in developed countries than in developing countries (2). According to cancer statistics 2020, in 2020 about 73,750 (4.1%) newly diagnosed kidney cancer cases are expected and around 14,830 (2.4%) will die of this cancer (3). In China, the incidence of RCC is the second highest in malignant tumors of the urinary system. Its incidence and mortality vary greatly from region to region and are higher in cities than in rural areas (4). Clear cell renal cell carcinoma (ccRCC) is the most important type in RCC. According to the statistics, it accounts for around 80–90% of RCC (5). Although the early detection of RCC has been improved in recent years, more than one third of patients have local advanced tumor or metastatic disease at the time of diagnosis (6). The treatment remains a challenge and reliable biomarkers are essential to prevent metastasis and improve the quality of patients’ life (7). Although some possible biomarkers have been found such as Ki-67, p53, VEGF, there is currently no reliable biomarker for RCC prediction (8). RCCs are usually resistant to conventional chemotherapy and almost all chemotherapeutic agents are ineffective against metastatic RCC (9). The use of targeted therapy is contributed to surgery in patients with locally advanced or metastatic RCC. Although some therapeutic targets have been applied, such as VEGFR and HIF-1α, our exploration of therapeutic targets is far from enough (7).
Hypertension is a widespread chronic disease with an increased incidence worldwide (10). Hypertension is an important risk factor for kidney cancer (11). Hypertensive nephropathy (HN) is one of the main complications of hypertension. Over the past few years, the relationship between hypertension and the risk of kidney cancer have been explored by many prospective studies (12-14). In the VITAL study, hypertension was independently associated with RCC risk (HR 1.70; 95% CI, 1.30–2.22) (15). A meta-analysis of prospective studies shows a strong positive correlation between hypertension and kidney cancer. A history of hypertension was associated with 67% increased risk of RCC. Considering heterogeneity and publication bias, each 10 mmHg increase in blood pressure was associated with 10–22% increased risk of RCC (16). However, the molecular mechanism of the relationship between kidney cancer and hypertension is not clear. For these reasons, the identification of key molecular involved in hypertension-related kidney cancer is urgent and highly demanded to improve the clinical outcome. In our research, HN-related DEGs (HN-DEGs) and RCC-related DEGs (RCC-DEGs) were identified by bioinformatic analysis. Then co-expressed DEGs (co-DEGs) of HN and RCC were found. Furthermore, further analysis and verification of DEGs and predicted targeted miRNAs were conducted for HN patients inclined to RCC to find possible molecular mechanisms. We present the following article in accordance with the MDAR reporting checklist (available at http://dx.doi.org/10.21037/tau-20-817).
Methods
Gene expression profiles data
We downloaded GSE99339, GSE99325, GSE53757 and GSE15641 datasets from GEO (http://www.ncbi.nlm.nih.gov/geo/). The expression profiling arrays of GSE99339 were generated applying GPL19109 [HG-U133_Plus_2] Affymetrix Human Genome U133 Plus 2.0 Array [CDF: Brainarray HGU133Plus2_Hs_ENTREZG_v18], including 15 Hypertensive Nephropathy specimens and 14 Tumor Nephrectomy specimens. The expression profiling arrays of GSE99325 were generated using GPL19184 [HG-U133A] Affymetrix Human Genome U133A Array [Custom Brainarray v18 ENTREZG CDF], including 20 Hypertensive Nephropathy specimens and 4 Cadaveric Donor specimens. Next, we used GPL570 [HG-U133_Plus_2] Affymetrix Human Genome U133 Plus 2.0 Array to generate the expression profiling arrays of GSE53757, including 72 ccRCC specimens and 72 normal specimens. Moreover, we also used GPL96 [HG-U133A] Affymetrix Human Genome U133A Array to generate the expression profiling arrays of GSE15641, including 49 RCC tissues and 23 normal tissues. We used the two gene expression profiles of GSE99339 and GSE99325 to filter differentially expressed genes (DEGs) of HN. Similarly, the two gene expression profiles of GSE53757 and GSE15641 were applied to filter DEGs of RCC. All procedures performed in this study were in accordance with the ethical standards of the institutional and/or national research committee and with the Helsinki Declaration (as revised in 2013).
Data processing
We used R packages of “affy”, “affyPLM”, and “limma” (http://www.bioconductor.org/packages/release/bioc/html/affy.html) to assess GSE99339, GSE99325, GSE53757 and GSE15641 RAW datasets. Background correction, probe summarization, quantile normalization, and log2-transformation were used to generate a robust multi-array average (RMA), a log-transformed mismatch, and perfect match probe methods. We applied the Benjamini-Hochberg method to adjust original P values and the false discovery rate (FDR) procedure to calculate fold-changes (FC). We used Genes expression values of the|log2 FC| >1.5 or <0.667 and adjusted P<0.05 to screen HN-DEGs. Moreover, we used the |log2 FC| >2 or <0.5 and adjusted P<0.05 to filter RCC-DEGs. Furthermore, volcano plots and Venn diagrams were made for co-DEGs of HN- and RCC-DEGs.
Analysis of protein-protein interaction (PPI) networks
We used the search tool for the retrieval of interacting genes (STRING) database (V11; http://string-db.org/) to analyze PPI networks of HN- and RCC-DEGs. Analytic data of the STRING database were downloaded with a combined score >0.4. Then we applied Cytoscape software (V3.5.1; http://cytoscape.org/) to analyze and visualize node degrees and biological networks.
Functional enrichment analysis
We conducted Gene Ontology (GO) and Kyoto Encyclopedia of Genes and Genomes (KEGG) pathway enrichment analysis of HN- and RCC-DEGs with the database for annotation, visualization and integrated discovery (DAVID) bioinformatics resources (http://david.abcc.ncifcrf.gov/).We identified the significantly enriched GO terms and KEGG pathway maps related to biofunctions based on a P<0.05. Furthermore, we identified enriched functions of HN- and RCC-DEGs in molecular functions, biological processes, and cellular components, respectively.
Afterward, we used the AmiGO database (v2.0; http://amigo.geneontology.org/amigo/) to analyze the GO consortium of chosen co-DEGs in order to check the annotate and accuracy biofunctions of confirmed co-DEGs.
Identification of co-DEGs related to hypertension or renal cancer
We used the comparative toxicogenomics database (CTD, http://ctdbase.org/) to describe chemical-gene/protein interactions and chemical-disease and gene-disease relationships in order to know their associations. These data were used to identify the genes related to hypertension or renal cancer and scored genes indirectly through the CTD.
Co-DEGs validation
We used the Gene Expression Profiling Interactive Analysis (GEPIA) database (http://gepia.cancer-pku.cn/) to verify the differential expression of these genes in ccRCC. Overall survival curve was used to analyze survival differences.
Functional and pathway enrichment related to predicted miRNAs and Co-DEGs and validation of predicted miRNAs
We applied microRNA Data Integration Portal (mirDIP) (http://ophid.utoronto.ca/mirDIP) to predict miRNAs that may be regulated by 9 genes. Five top candidate miRNAs were determined according to predicted scores for each Co-DEG. We used Diana-miRPath (v3.0; http://www.microrna.gr/miRPathv3) to conduct GO terms and pathway enrichment analysis of these predicted miRNAs. Subsequently, the online tool Starbase (http://starbase.sysu.edu.cn/) was used to identify the relationship between predicted miRNAs and corresponding genes.
Statistical analysis
Data of DEGs were analyzed using R 4.0.0. Student’s t-test was performed for comparisons between two groups, whereas ANOVA was performed for repeated measures. The χ2 test was used to analyze the statistical significance of GO and pathway. Differences with P<0.05 were considered statistically significant.
Data of Co-DEGs validation were based on GEPIA. We chose the dataset of KIRC. One-way ANOVA was used for differential analysis. The expression data were log2(TPM+1) transformed for differential analysis and the log2FC was defined as median (Tumor) – median (Normal). The screening criterion was P value <0.05. In survival analysis, we used Log-rank test for hypothesis test (P<0.05). We calculated the hazards ratio (HR) based on Cox PH Mode and added the 95% CI as dotted line.
We used Pearson correlation analysis to identify the relationship between predicted miRNAs and Corresponding genes based on Starbase. The expression data of ccRCC were from TCGA. P<0.01 was considered statistically significant.
Results
Identification of DEGs
21,592 probes in GSE99339 and GSE99325 datasets and 76,937 probes in GSE53757 and GSE15641 were identified. Then we confirmed HN- and RCC-DEGs. In GSE99325, we identified 1,147 DEGs in HN tissue specimens compared with normal tissue specimens, including 585 upregulated genes and 144 downregulated genes (Figure 1A). Similarly, we also described the DEGs of the other three datasets in the form of volcano plots (Figure 1B,C,D). The common 60 genes of GSE99339 and GSE99325 were confirmed, including 38 upregulated and 22 downregulated genes, which are the DEGs of HN (Figure 1E). In the same way, the common 679 genes of GSE53757 and GSE15641 are the DEGs of RCC (Figure 1F). Heatmaps of HN-DEGs in relation to cellular response to tumor necrosis factor, response to lipopolysaccharide, multicellular organismal homeostasis and neutrophil degranulation were conducted for genes expression (Figure 2). The value of RCC-DEGs expression concerning extracellular structure organization, leukocyte migration, neutrophil activation and response to oxygen levels has been shown in Figure 3.
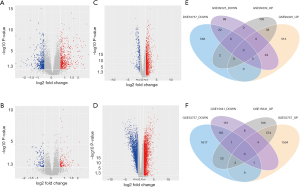
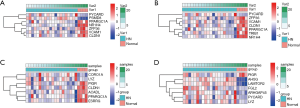
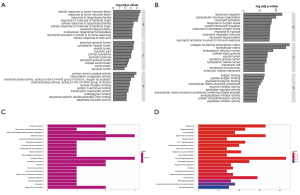
Functional enrichment in Co-DEGs
Figure 4A illustrates 9 Co-DEGs of HN- and RCC-DEGs, including Sin3A associated protein 30 (SAP30), polymerase I and transcript release factor (PTRF), lysozyme (LYZ), PYD and CARD domain containing (PYCARD), estrogen related receptor gamma (ESRRG), coronin 1A (CORO1A), fibronectin 1 (FN1), branched chain amino acid transaminase 1 (BCAT1), cysteine rich protein 1 (CRIP1). We confirmed GO term enrichment concerning biological processes, molecular functions, and cellular components with AmiGO database. We found that Co-DEGs were related to many processes (Table S1).
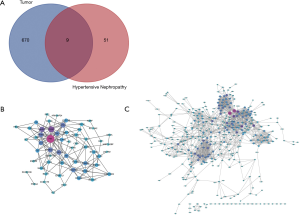
PPI network analysis, GO analysis and KEGG pathway enrichment analysis
Fifty-eight and 618 nodes have been identified from PPI network of HN- and RCC-DEGs, respectively (Figure 4B,C). The hub nodes including fibronectin 1 (FN1; degree =21), vascular cell adhesion molecule 1 (VCAM1; degree =14), alpha 2-HS glycoprotein (AHSG; degree =12), nuclear receptor subfamily 1 group H member 4 (NR1H4; degree =10), PPARG coactivator 1 alpha (PPARGC1A; degree =10), coronin 1A (CORO1A; degree =10) and activating transcription factor 3 (ATF3; degree =10) are hub genes of HN. Similarly, the hub genes including kininogen 1 (KNG1; degree =58), complement C3 (C3; degree =55), fibronectin 1 (FN1; degree =38), TIMP metallopeptidase inhibitor 1 (TIMP1; degree =36), albumin (ALB; degree =36), prolyl 4-hydroxylase subunit beta (P4HB; degree =34) are illustrated in RCC-DEGs with a high degree.
We identified the GO terms involved in biological processes among HN-DEGs by using the DAVID database. They were mainly associated with cellular response to tumor necrosis factor (Fold Enrichment: 7.88; P value: 2.82E-05), response to tumor necrosis factor (Fold Enrichment: 7.35; P value: 4.4E-05), response to lipopolysaccharide (Fold Enrichment: 6.95; P value: 6.26E-05), response to molecule of bacterial origin (Fold Enrichment: 6.68; P value: 7.98E-05) and cellular response to lipopolysaccharide (Fold Enrichment: 6.68; P value: 7.99E-05). There is evident correlation in secretory granule lumen (Fold Enrichment: 6.47; P value: 3.21E-04), cytoplasmic vesicle lumen (Fold Enrichment: 6.14; P value: 4.22E-04), vesicle lumen (Fold Enrichment: 6.12; P value: 4.29E-04) and cytosolic part (Fold Enrichment: 7.00; P value: 7.30E-04) in connection with cellular components. Furthermore, the terms concerning molecular functions were primarily referred to primary amine oxidase activity (Fold Enrichment: 107.25; P value: 1.41E-04), transcription coregulator activity (Fold Enrichment: 4.58; P value: 3.22E-04) and oxidoreductase activity, acting on the CH-NH2 group of donors, oxygen as acceptor (Fold Enrichment: 40.22; P value: 0.001). RCC was also analyzed. The biological processes terms including leukocyte migration (Fold Enrichment: 4.19; P value: 2.13E-24), extracellular structure organization (Fold Enrichment: 4.19; P value: 1.28E-20), neutrophil activation (Fold Enrichment: 3.56; P value: 4.95E-18) and response to oxygen levels (Fold Enrichment: 4.16; P value: 8.77E-20) were significantly enriched. Similarly, the terms of collagen-containing extracellular matrix (Fold Enrichment: 5.60; P value: 5.73E-29), extracellular matrix (Fold Enrichment: 4.41; P value: 9.82E-27) and endoplasmic reticulum lumen (Fold Enrichment: 4.69; P value: 7.46E-19) concerning cellular components were primarily enriched. In addition, the molecular functions terms of antigen binding (Fold Enrichment: 4.66; P value: 1.38E-13), peptide antigen binding (Fold Enrichment: 13.10; P value: 3.94E-13) and glycosaminoglycan binding (Fold Enrichment: 4.17; P value: 3.20E-12) were notably enriched (Figure 5A,B).
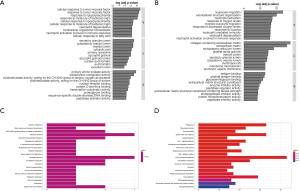
KEGG pathway analysis data is shown in Figure 5. It indicated that the HN-DEGs were greatly enriched in pathways of tyrosine metabolism (P value: 0.006) (Figure 5C). However, KEGG terms including phagosome (P value: 6.96E-13), rheumatoid arthritis (P value: 1.48E-10) and viral myocarditis (P value: 5.03E-10) were enriched in RCC-DEGs (Figure 5D).
Co-DEGs validation and detection
The CTD showed that Co-DEGs BCAT1, CORO1A, CRIP1, ESRRG, FN1, LYZ, PYCARD, and SAP30 targeted hypertension and renal cancer and these data appear in Figure 6. Compared with para cancerous normal tissues, the expression levels of 7 genes except BCAT1 were obviously higher in ccRCC in view of the GEPIA database (Figure 7A,B,C,D,E,F,G,H). Among the 8 key genes, we found that CRIP1, ESRRG, LYZ, and PYCARD were obviously associated with the overall survival of ccRCC patients (Figure 7I,J,K,L).
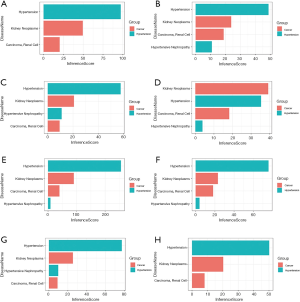
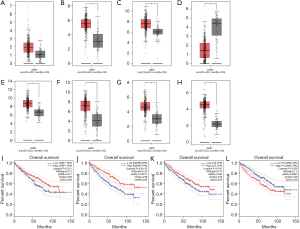
Functional and pathway enrichment related to predicted miRNAs and Co-DEGs and validation of predicted miRNAs
We used mirDIP and DIANA-MirPath database to predict the top 5 targeted miRNAs of each Co-DEG associated with HN-related RCC (Table S2). In Figure 8, we identified the relationship between predicted miRNAs and Corresponding genes in ccRCC. Hsa-miR-429 and hsa-miR-200b-3p are negatively correlated with FN1, respectively. Hsa-miR-30e-5p and hsa-miR-30b-5p are negatively correlated with SAP30, respectively. Hsa-miR-221-5p is negatively correlated with CRIP1. Hsa-miR-205-5p, hsa-miR-152-3p and hsa-miR-137 are negatively correlated with ESRRG, respectively.
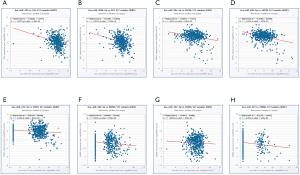
Discussion
Numerous observational studies have systematically reported an increased risk of RCC in patients with hypertension (17,18). The biological mechanism of the relationship between hypertension and RCC is indefinite. However, it is speculated that it is related to chronic kidney hypoxia and lipid peroxidation with the generation of reactive oxygen species (ROS) (19,20). Patients with hypertension may cause chronic kidney hypoxia due to the transcription of hypoxia inducible factors, which can promote tumor cell angiogenesis and proliferation (21). we identified 8 key genes of hypertension-related RCC, including BCAT1, CORO1A, CRIP1, ESRRG, FN1, LYZ, PYCARD, and SAP30. These genes will help us further explore the mechanism of hypertension-related RCC and may become biomarkers of hypertension-related RCC. And they are also important for exploring new therapeutic targets of hypertension-related RCC.
One study showed that CRIP1 has a strong connection with pressure at the population level. The effect of CRIP1 on blood pressure may be achieved by mediating SH2B3 (22). Meanwhile, Macrophages that lack SH2B3 expression are easily activated, producing more ROS (23), which can cause kidney injury. We generally believe that SH2B3 negatively inhibits proinflammatory cell signaling within the kidney in normal and pathological states (24). Long-term effects of these mechanisms may lead to the development of hypertension-related RCC. The detection of CRIP1 helps us to detect RCC early in patients with hypertension, so as to improve the survival rate of RCC. Recent studies have revealed that ESRRG is a key transcriptional regulator of mitochondrial oxidative phosphorylation (OxPhos) and fatty acid oxidation (FAO) (25). Through the analysis of epigenomic elements of the promoter, it is found that ESRRG is a new obesity-susceptibility gene (26). It is well known that obesity and hypertension are highly correlated. Based on this, we speculate that ESRRG is associated with hypertension. ESRRG is highly expressed in the kidney and plays a major role in normal embryonic kidney development (27). The lack of ESRRG in renal epithelial cells (RECs) causes serious renal energy and absorption dysfunction and renal failure. The expression of ESRRG is positively correlated with renal function and decreases in patients with chronic kidney disease (CKD) (28). CKD is one of the causes of RCC (29). ESRRA and ESRRG drive changes in HIF1A and HIF2A, which are important in developing ccRCC molecular phenotypes (30). In addition, ESRRG also plays a role in other tumors, including breast cancer, endometrial cancer, gastric cancer, liver cancer, and prostate cancer. Further exploration of the role of ESRRG in the occurrence and development of hypertension-related RCC will help us find new therapeutic targets for RCC, thereby alleviating the situation of drug resistance in the treatment of renal cancer. FN1 is a gene that has been widely explored. The expression of FN1 is increased in patients with hypertension (31). Several studies show a strong correlation between FN1 and RCC (32,33). FN1 expression in RCC is related to a higher disease-related mortality rate, indicating a probable role in RCC progression (34). Apoptosis genes such as PYCARD have shown potential to improve the prognosis of other cancers and may be demonstrated by further research to have the same potential in RCC (35). Transcription factor SAP30 is important to activation of expression of NETO2 gene in ccRCC. Meanwhile, mRNA level of SAP30 increased significantly and was positively correlated with NETO2 gene expression (36).
In our data, miR-429, miR-200b-3p, miR-30e-5p, miR-30b-5p, miR-221-5p, miR-205-5p, miR-152-3p and miR-137 may sponge with corresponding genes and act on hypertension-related RCC. Has-miR-429 is one of the most studied miRNAs in RCC. It is reported that miR-429 suppresses tumor cell proliferation, metastasis and epithelial-mesenchymal transition by direct targeting of BMI1, E2F3 and VEGF in RCC (37,38). MiR-137 inhibits the growth and invasion of ccRCC cells, and induces apoptosis, acting as a tumor suppressor gene. MiR-137 works by targeting 3'-UTR of RLIP76 which is an oncogene identified in ccRCC (39).
Conclusions
According to existing literature reports, several genes such as CRIP1, ESRRG, FN1, PYCARD, and SAP30 are related to hypertension or RCC. Through the verification of CTD, GEPIA and Starbase databases, we found that the two genes CRIP1, ESRRG and their corresponding predicted miRNAs are most likely instructive for further exploration of hypertension-related RCC and contribute to finding new therapeutic targets.
However, some limitations also exist. primarily, this is a microarray analysis study and our data were acquired from a publicly available database. Given that gene expression is not necessarily equivalent to protein expression, we need further in vivo and in vitro experiments to clarify molecular mechanisms of key genes for clinical applications. Secondly, prospective clinical studies may validate our point of view better.
Acknowledgments
Funding: This work was supported by research grants from the Natural Science Foundation of China (no. 81972393, 81772705, 31570775).
Footnote
Reporting Checklist: The authors have completed the MDAR reporting checklist. Available at http://dx.doi.org/10.21037/tau-20-817
Conflicts of Interest: All authors have completed the ICMJE uniform disclosure form (available at http://dx.doi.org/10.21037/tau-20-817). The authors have no conflicts of interest to declare.
Ethical Statement: The authors are accountable for all aspects of the work in ensuring that questions related to the accuracy or integrity of any part of the work are appropriately investigated and resolved. All procedures performed in this study were in accordance with the ethical standards of the institutional and/or national research committee and with the Helsinki Declaration (as revised in 2013).
Open Access Statement: This is an Open Access article distributed in accordance with the Creative Commons Attribution-NonCommercial-NoDerivs 4.0 International License (CC BY-NC-ND 4.0), which permits the non-commercial replication and distribution of the article with the strict proviso that no changes or edits are made and the original work is properly cited (including links to both the formal publication through the relevant DOI and the license). See: https://creativecommons.org/licenses/by-nc-nd/4.0/.
References
- Saad AM, Gad MM, Al-Husseini MJ, et al. Trends in Renal-Cell Carcinoma Incidence and Mortality in the United States in the Last 2 Decades: A SEER-Based Study. Clin Genitourin Cancer 2019;17:46-57.e5. [Crossref] [PubMed]
- Znaor A, Lortet-Tieulent J, Laversanne M, et al. International variations and trends in renal cell carcinoma incidence and mortality. Eur Urol 2015;67:519-30. [Crossref] [PubMed]
- Siegel RL, Miller KD, Jemal A. Cancer statistics, 2020. CA: a cancer journal for clinicians 2020;70:7-30. [Crossref] [PubMed]
- Chen W, Sun K, Zheng R, et al. Cancer incidence and mortality in China, 2014. Chin J Cancer Res 2018;30:1-12. [Crossref] [PubMed]
- Ljungberg B, Bensalah K, Canfield S, et al. EAU guidelines on renal cell carcinoma: 2014 update. Eur Urol 2015;67:913-24. [Crossref] [PubMed]
- Zhang X, Ren J, Yan L, et al. Cytoplasmic expression of pontin in renal cell carcinoma correlates with tumor invasion, metastasis and patients' survival. PLoS One 2015;10:e0118659. [Crossref] [PubMed]
- Di Pietro G, Luu HN, Spiess PE, et al. Biomarkers and new therapeutic targets in renal cell carcinoma. Eur Rev Med Pharmacol Sci 2018;22:5874-91. [PubMed]
- Chen W, Hill H, Christie A, et al. Targeting renal cell carcinoma with a HIF-2 antagonist. Nature 2016;539:112-7. [Crossref] [PubMed]
- Morais C, Gobe G, Johnson DW, et al. The emerging role of nuclear factor kappa B in renal cell carcinoma. Int J Biochem Cell Biol 2011;43:1537-49. [Crossref] [PubMed]
- Kearney PM, Whelton M, Reynolds K, et al. Global burden of hypertension: analysis of worldwide data. Lancet 2005;365:217-23. [Crossref] [PubMed]
- Radišauskas R, Kuzmickiene I, Milinaviciene E, et al. Hypertension, serum lipids and cancer risk: A review of epidemiological evidence. Medicina (Kaunas) 2016;52:89-98. [Crossref] [PubMed]
- Shen T, Shu XO, Xiang YB, et al. Association of hypertension and obesity with renal cell carcinoma risk: a report from the Shanghai Men's and Women's Health Studies. Cancer Causes Control 2015;26:1173-80. [Crossref] [PubMed]
- Hofmann JN, Corley DA, Zhao WK, et al. Chronic kidney disease and risk of renal cell carcinoma: differences by race. Epidemiology 2015;26:59-67. [Crossref] [PubMed]
- Mariusdottir E, Ingimarsson JP, Jonsson E, et al. Occupation as a risk factor for renal cell cancer: a nationwide, prospective epidemiological study. Scand J Urol 2016;50:181-5. [Crossref] [PubMed]
- Macleod LC, Hotaling JM, Wright JL, et al. Risk factors for renal cell carcinoma in the VITAL study. J Urol 2013;190:1657-61. [Crossref] [PubMed]
- Hidayat K, Du X, Zou SY, et al. Blood pressure and kidney cancer risk: meta-analysis of prospective studies. J Hypertens 2017;35:1333-44. [Crossref] [PubMed]
- Chow WH, Gridley G, Fraumeni JF Jr, et al. Obesity, hypertension, and the risk of kidney cancer in men. N Engl J Med 2000;343:1305-11. [Crossref] [PubMed]
- Choi MY, Jee SH, Sull JW, et al. The effect of hypertension on the risk for kidney cancer in Korean men. Kidney Int 2005;67:647-52. [Crossref] [PubMed]
- Sharifi N, Farrar WL. Perturbations in hypoxia detection: a shared link between hereditary and sporadic tumor formation? Med Hypotheses 2006;66:732-5. [Crossref] [PubMed]
- Gago-Dominguez M, Castelao JE, Yuan JM, et al. Lipid peroxidation: a novel and unifying concept of the etiology of renal cell carcinoma (United States). Cancer Causes Control 2002;13:287-93. [Crossref] [PubMed]
- Kaelin WG Jr. The von Hippel-Lindau gene, kidney cancer, and oxygen sensing. J Am Soc Nephrol 2003;14:2703-11. [Crossref] [PubMed]
- Zeller T, Schurmann C, Schramm K, et al. Transcriptome-Wide Analysis Identifies Novel Associations With Blood Pressure. Hypertension 2017;70:743-50. [Crossref] [PubMed]
- Gueller S, Goodridge HS, Niebuhr B, et al. Adaptor protein Lnk inhibits c-Fms-mediated macrophage function. J Leukoc Biol 2010;88:699-706. [Crossref] [PubMed]
- Blass G, Mattson DL, Staruschenko A. The function of SH2B3 (LNK) in the kidney. Am J Physiol Renal Physiol 2016;311:F682-5. [Crossref] [PubMed]
- Eichner LJ, Giguere V. Estrogen related receptors (ERRs): a new dawn in transcriptional control of mitochondrial gene networks. Mitochondrion 2011;11:544-52. [Crossref] [PubMed]
- Dong SS, Guo Y, Zhu DL, et al. Epigenomic elements analyses for promoters identify ESRRG as a new susceptibility gene for obesity-related traits. Int J Obes (Lond) 2016;40:1170-6. [Crossref] [PubMed]
- Berry R, Harewood L, Pei L, et al. Esrrg functions in early branch generation of the ureteric bud and is essential for normal development of the renal papilla. Hum Mol Genet 2011;20:917-26. [Crossref] [PubMed]
- Zhao J, Lupino K, Wilkins BJ, et al. Genomic integration of ERRgamma-HNF1beta regulates renal bioenergetics and prevents chronic kidney disease. Proc Natl Acad Sci U S A 2018;115:E4910-9. [Crossref] [PubMed]
- Hofmann JN, Purdue MP. CKD and risk of renal cell carcinoma: a causal association? J Am Soc Nephrol 2014;25:2147-8. [Crossref] [PubMed]
- Neely BA, Wilkins CE, Marlow LA, et al. Proteotranscriptomic Analysis Reveals Stage Specific Changes in the Molecular Landscape of Clear-Cell Renal Cell Carcinoma. PLoS One 2016;11:e0154074. [Crossref] [PubMed]
- Stoynev N, Dimova I, Rukova B, et al. Gene expression in peripheral blood of patients with hypertension and patients with type 2 diabetes. J Cardiovasc Med (Hagerstown) 2014;15:702-9. [Crossref] [PubMed]
- Waalkes S, Atschekzei F, Kramer MW, et al. Fibronectin 1 mRNA expression correlates with advanced disease in renal cancer. BMC Cancer 2010;10:503. [Crossref] [PubMed]
- Song E, Song W, Ren M, et al. Identification of potential crucial genes associated with carcinogenesis of clear cell renal cell carcinoma. J Cell Biochem 2018;119:5163-74. [Crossref] [PubMed]
- Steffens S, Schrader AJ, Vetter G, et al. Fibronectin 1 protein expression in clear cell renal cell carcinoma. Oncol Lett 2012;3:787-90. [PubMed]
- Rajandram R, Pat BK, Li J, et al. Expression of apoptotic tumour necrosis factor receptor-associated factor, caspase recruitment domain and cell death-inducing DFF-45 effector genes in therapy-treated renal cell carcinoma. Nephrology (Carlton) 2009;14:205-12. [Crossref] [PubMed]
- Snezhkina AV, Nyushko KM, Zaretsky AR, et al. Transcription Factor SAP30 Is Involved in the Activation of NETO2 Gene Expression in Clear Cell Renal Cell Carcinoma. Mol Biol (Mosk) 2018;52:451-9. [PubMed]
- Qiu M, Liang Z, Chen L, et al. MicroRNA-429 suppresses cell proliferation, epithelial-mesenchymal transition, and metastasis by direct targeting of BMI1 and E2F3 in renal cell carcinoma. Urol Oncol 2015;33:332.e9-e18. [Crossref] [PubMed]
- Chen D, Li Y, Li Y, et al. Tumor suppressive microRNA429 regulates cellular function by targeting VEGF in clear cell renal cell carcinoma. Mol Med Rep 2016;13:1361-6. [Crossref] [PubMed]
- Wang M, Gao H, Qu H, et al. MiR-137 suppresses tumor growth and metastasis in clear cell renal cell carcinoma. Pharmacol Rep 2018;70:963-71. [Crossref]