A new risk stratification system of prostate cancer to identify high-risk biochemical recurrence patients
Introduction
Prostate cancer (PCa) is the second most commonly diagnosed cancer and the fifth leading cause of cancer related deaths globally (1). There have been four previous studies which have reported that approximately 20–40% of patients experience a biochemical recurrence (BCR) after radical prostatectomy (RP) (2-4). According to the guidelines of the European Association of Urology and the American Urological Association, BCR is defined as two consecutive prostate-specific antigen (PSA) values of ≥0.2 ng/mL following RP (5,6). Although the BCR is not equivalent to clinical recurrence after RP (5), it is a decisive risk factor for PCa-specific mortality and overall mortality (7). In the absence of secondary therapy following BCR, around 30% of patients have a median period of 5–8 years before clinical progression and 32–45% among these patients would suffer PCa-specific mortality within 15 years (8). Therefore, it is crucial to identify these high-risk patients early and accurately in order to evaluate their prognosis and tailor patient-specific follow-up and management.
Clinicopathological parameters such as the Gleason score (GS), pathological tumor staging (pT), and PSA are associated with the survival of PCa patients and can predict the incidence of BCR. In 1998, D’Amico et al. (9) first proposed a risk classification method based on pT staging, GS, and PSA to predict the risk of BCR. Subsequently, the CAPRA nomogram by Cooperberg et al. (10), the nomogram by Stephenson et al. (11), a risk classification system by the National Comprehensive Cancer Network (12), and the Genomic Prostate Score (GPS) (13) are also based on clinical pathology variables. However, current risk stratification methods explain only a proportion of the observed variation in clinical outcomes and is not accurate enough to identify patients at high-risk of BCR (14-16). Molecular properties or biomarkers, such as alterations at both the genetic and protein levels, have been identified which allows the identification of risk-predictive signatures that can improve the clinical outcome (17-20). However, there is a critical requirement to improve the evaluation of BCR (21).
In this study, we aimed to: (I) identify mRNAs associated with BCR by Cox regression analysis of mRNA sequencing data obtained from the gene expression omnibus (GEO) and the Cancer Genome Atlas (TCGA) database; (II) construct and validate the mRNA signature and a nomogram combined signature with clinicopathological parameters to identify patients with high-risk BCR; and (III) analyse biological signaling pathway of the mRNA signature by Gene Set Enrichment Analysis (GSEA). We present the following article in accordance with the TRIPOD reporting checklist (available at http://dx.doi.org/10.21037/tau-20-1019).
Methods
Data processing
In this study, three independent datasets with their gene expression profile matrix files and corresponding clinical data were downloaded from the GEO dataset (https://www.ncbi.nlm.nih.gov/geo/). These included the GSE21034, GSE70770, and GSE116918 datasets. The average expression value for mRNA was used if multiple probes matched a single mRNA. The mRNAs with average expression values <1 were removed. The ‘sva’ package (Version: 3.36.0; http://bioconductor.org/packages/release/bioc/html/sva.html) was used to remove batch effects.
RNA-sequencing data and the corresponding clinical data of patients with PCa were downloaded from the TCGA (https://portal.gdc.cancer.gov). The count data (RNAseq) was transformed by variance stabilizing transformation using “DESeq2” package. The mRNAs with an average expression value <1 were removed. A total of 977 patients with complete clinical information, including PSA, GS and pT staging were enrolled. This consisted of 405 patients from the TCGA dataset, 131 from the GSE21034 dataset, 193 from the GSE70770 dataset, and 248 from the GSE116918 dataset.
The TCGA and GEO datasets were extracted from a public database and required no ethical approval. The study was conducted in accordance with the Declaration of Helsinki (as revised in 2013).
Construction and validation of the mRNA-based prognostic signature
In order to obtain a consistent formula and a uniform cutoff value to divide PCa patients into low- and high-risk groups, we normalized for the expression values of mRNAs in TCGA, GSE21034, GSE70770, and GSE116918 datasets with a standard deviation (SD) =1 and mean value =0. Univariate Cox regression analysis was used to analyze the association between gene expression and BCR in each cohort. P values of <0.05 were considered statistically significant. Common prognostic genes between the four datasets were selected and LASSO analysis was performed to pinpoint which mRNAs could be used for the prediction of BCR using the “glmnet” package. Finally, based on the results of the LASSO analysis, we performed multivariate Cox regression analyses to establish the mRNA signature from the TCGA dataset. The risk score for each patient was calculated by combining the expression values of prognostic genes and their regression coefficients. All patients were classified into either high-risk or low-risk groups based on their median risk score. The risk score of each patient in the GSE21034, GSE70770, and GSE116918 datasets were calculated using the same formula. Each dataset was divided into high and low-risk groups according to the same cutoff values. Kaplan-Meier analysis with log-rank test was used to assess the difference in BCR-free survival between the high-risk and low-risk groups. The time-dependent receiver operating characteristic (ROC) analysis with area under the curve (AUC) was used to evaluate the diagnostic performance of the signature. A concordance index (C-index) using 1000 bootstrap resamples was also used to assess the prognostic accuracy of the signature with clinical data. We used the “survcomp” package to compare the C-index between the 9-mRNA signature and D’Amico model (9) which was developed based on the clinical T stage, preoperative PSA, and GS.
Independence of the mRNA signature from clinical characteristics
Univariate and multivariable Cox regression analyses were performed to assess whether the risk score was independent of GS, pT staging, or PSA. To further identify whether the mRNA signature was a statistically significant prognostic factor in its own regard, the patients were stratified by clinical characteristics and a Kaplan-Meier survival analysis was used to assess the difference in BCR-free survival between high-risk and low-risk subgroups.
Construction of the nomogram
The nomogram was constructed by combining the mRNA-based prognostic signature and clinical variables from the TCGA dataset. Calibration plots and C-index were performed to assess the prognostic performance of the nomogram. The C-index was calculated using a bootstrap method with 1,000 resamples and displayed a degree of consistency between the actual observed outcome probabilities and predicted probabilities. The x and y-axis of the calibration plot showed nomogram-predicted recurrence and observed outcome, respectively. The 45° line represented the best prediction.
Functional enrichment analysis and gene set enrichment analysis
GO and KEGG pathway enrichment analyses were conducted to identify the biological processes, cellular components, and molecular functions of the mRNAs related to BCR identified in the four datasets. Functions displaying significance were explored using the “clusterProfile” package. Gene set enrichment analysis (GSEA) was performed to identify significantly altered molecular mechanisms associated with the prognostic gene signature using the JavaGSEA software. A total of 405 PCa samples from the TCGA dataset were divided into high and low-risk groups according to the median risk score and annotated gene set c2.cp.kegg.v7.0.symbols.gmt was explored to identify enriched KEGG pathways associated with the poor prognosis of the high-risk group. FDR <0.05 and |NES| >1 were considered as the cut-off criteria.
Statistical analysis
All statistical analysis was conducted in R v. 3.6.1. Cox regression analysis was performed using the “survival” package to evaluate survival probability. The hazard ratio (HR) and 95% confidence interval (CI) were used to assess the association between signature and survival. Chi-square test or Fisher’s exact tests were used to examine the association between the patients with and without BCR and the clinical information. All P values <0.05 were considered statistically significant.
Results
Identification of commonly BCR-related mRNAs of four datasets
Candidate genes that were associated with BCR from each dataset were identified and the most credible prognostic genes of the four overlapping datasets were selected. The prognostic signature was then developed using the Cox proportional hazard regression model and the TCGA dataset, followed by validation using the GSE21034, GSE70770, and GSE116918 datasets. Finally, we developed a nomogram based on the prognostic signature and clinicopathological parameters. These were again validated using the GSE21034, GSE70770, and GSE116918 datasets (Figure 1).
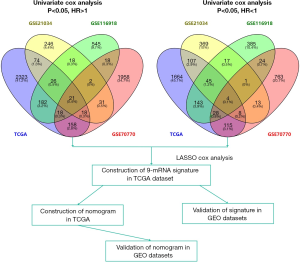
Clinical characteristics of the patients (PSA, GS, pT staging, and follow-up time) from the TCGA and GEO datasets are shown in Table 1 and Table S1. The median follow-up time for the TCGA, GSE21034, GSE70770, and GSE116918 cohorts were 31.5, 47.5, 54.1, and 78.3 months, respectively. The platforms for the GSE21034, GSE70770, and GSE116918 datasets were the Affymetrix Human Exon 1.0 ST Array, Illumina HumanHT-12 V4.0 expression beadchip, and Almac Diagnostics Prostate Disease Specific Array (Table S2).
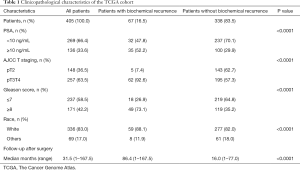
Full table
In this study, a univariate Cox regression analysis was performed for each dataset and candidate prognostic mRNAs that were significantly associated with BCR were identified using the cutoff values of P<0.05 and |HR| >1.0. A total of 2,822 mRNAs in the TCGA dataset, 436 mRNAs in the GSE21034 dataset, 2,243 mRNAs in the GSE70770 dataset, and 830 mRNAs in the GSE116918 dataset were identified as candidate risk factors. A total of 2,115 mRNAs in the TCGA dataset, 564 mRNAs in the GSE21034 dataset, 956 mRNAs in the GSE70770 dataset, and 647 mRNAs in the GSE116918 dataset were identified as candidate protective factors. Candidate prognostic mRNAs from the four datasets were intersected, and a total of 21 mRNAs associated with increased risk and 4 mRNAs associated with protection were identified.
Construction of the mRNA-based prognostic signature
To further analyse the mRNAs common to all the datasets which were associated with BCR, LASSO analysis was used to shrink all the regression coefficients towards zero and select variables simultaneously. The minimum criteria as the optimal lambda values were determined through 10-fold cross-validations (Figure 2). Finally, using multivariate Cox analysis, we identified a 9-mRNA signature that was significantly associated with BCR risk in PCa patients. The univariate Cox regression analysis result for the 9-mRNAs is shown in Table S3. The mRNAs C9orf152 and EPHX2 with HR <1 were considered protective mRNAs, whereas the mRNAs ASPM, MMP11, CENPF, KIF4A, COL1A1, ASPN, and FANCI with HR >1 were regarded as risk-associated mRNAs. The risk score was calculated as follows: [0.68 × Expression value of ASPM] + [0.79 × Expression value of MMP11] + [0.51 × Expression value of CENPF] + [0.14 × Expression value of KIF4A] + [1.17 × Expression value of COL1A1] + [0.39 × Expression value of ASPN] + [1.11 × Expression value of FANCI] + [(-1.59) × Expression value of C9orf152] + [(−1.29) × Expression value of EPHX2].
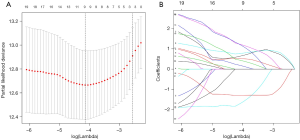
The TCGA cohort was stratified into either a high-risk group or low-risk group based on the median risk score. The risk score distribution, mRNA expression, and BCR status of patients from the TCGA dataset are shown and ranked based on the risk score values of the 9-mRNA signature (Figure 3A).
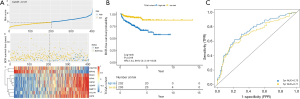
The Kaplan-Meier survival curves showed that the patients with the higher risk scores had significantly higher risk of BCR compared to those in the low risk group (HR =3.72, 95% CI: 2.30–6.00, P<0.0001; Figure 3B). The AUCs for 3- and 5-year BCR-free survival predictions for the 9-mRNA signature were 0.75 and 0.71, respectively (Figure 3C). This indicated that the 9-mRNA signature had high sensitivity and specificity as a diagnostic marker. The C-index of the 9-mRNA signature was 0.72 (95% CI: 0.66–0.78, P<0.0001). The C-index for BCR-free survival predictions for the 9-mRNA signature was higher than D’Amico model (9) for the TCGA dataset, but it was not statistically significant (Table S4).
Additionally, multivariate Cox regression analysis showed that the 9-mRNA signature was an independent risk factor after adjusting for the clinicopathological variables (Table 2). The Kaplan-Meier survival curves showed that the 9-mRNA signature was still a statistically significant prognostic factor regardless of clinical data (Figure S1).
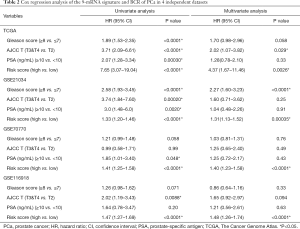
Full table
Validation of the 9-mRNA signature
To further evaluate the diagnostic potential of the 9-mRNA signature, we performed the same analysis as mentioned above for three external validation datasets, including GSE21034, GSE70770, and GSE116918. The risk scores for all the patients in the validation cohorts were calculated using the same formula and were divided into high and a low-risk groups based on the same cutoff. The risk score distribution, mRNA expression, and BCR status of patients for these external validation datasets can be found in Figure 4A,B,C. Significantly higher BCR-free survival rates were observed in the low-risk groups, compared with the high-risk groups for each validation dataset (HR >1, P<0.05; Figure 4D,E,F).
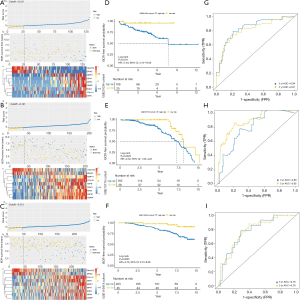
ROC curve analysis also displayed good prognostic performance for patients in all the datasets (Figure 4G,H,I). The C-index of the GSE21034, GSE70770, and GSE116918 datasets were 0.79 (95% CI: 0.71–0.87, P<0.0001), 0.74 (95% CI: 0.67–0.80, P<0.0001), 0.71 (95% CI: 0.64–0.78, P<0.0001), respectively. Furthermore, we compared the C-index between the 9-mRNA signature and D’Amico model (Table S4). Although not statistically significant, the C-index for the BCR-free survival predictions for the 9-mRNA signature was higher than the D’Amico model (9) for the GSE70770 and GSE116918 datasets. The univariate and multivariate Cox regression analysis also indicated that the 9-mRNA signature was an independent risk factor (Table 2).
Construction of the nomogram
To quantitively predict the probability of BCR during PCa, we created a nomogram by combining the mRNA-based prognostic signature and clinical variables and applied it to the TCGA dataset to predict 3- and 5-year BCR probability (Figure 5). The mRNA signature-based nomogram performed well and could predict the BCR for the TCGA dataset (C-index =0.74, 95% CI: 0.68–0.80, P<0.0001), GSE21034 dataset (C-index =0.85, 95% CI: 0.78–0.91, P<0.0001), GSE70770 dataset (C-index =0.76, 95% CI: 0.70–0.83, P<0.0001), and GSE116918 dataset (C-index =0.71, 95% CI: 0.64–0.79, P<0.0001). The C-index for BCR-free survival predictions for the nomogram was higher than D’Amico model for the TCGA, GSE21034, GSE70770 and GSE116918 datasets (Table S5). The calibration plots also demonstrated that the nomogram performed highly and was an ideal model both for the TCGA cohort and validation cohorts (Figure 6).
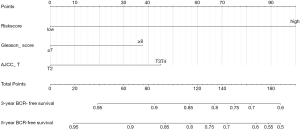
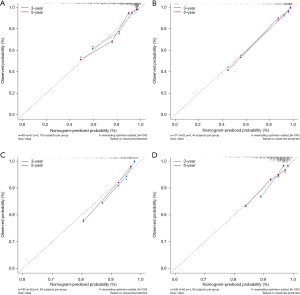
Validation of the expression of the 9 mRNAs
The expression values of the 9 mRNAs were validated using the UALCAN website (http://ualcan.path.uab.edu/). The mRNA expression values for ASPM, ASPN, C9orf152, CENPF, COL1A1, FANCI, KIF4A, and MMP11 were significantly increased in PCa tissue compared with normal tissues. In contrast, EPHX2 expression was significantly decreased (Figure 7). We also used the Human protein atlas website (https://www.proteinatlas.org/) to explore protein expression of the 8 mRNAs. Typical immunohistochemistry of the 8 mRNAs (except ASPM, not recorded in the website) in tumor and normal prostate tissues are shown in Figure 8.
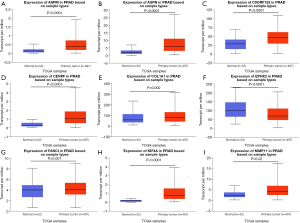
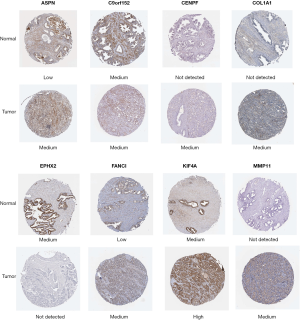
Functional enrichment analysis and gene set enrichment analysis
The mRNAs from the four datasets were significantly involved in nuclear division, organelle fission, and mitotic nuclear division (Figure 9A). We also performed GSEA analysis on the high- and low-risk groups from the TCGA dataset to identify biological pathways significantly associated with the 9-mRNA signature. Based on the cut-off criteria of FDR <0.05, three KEGG pathways were significantly enriched in the high-risk group. These included the cell cycle pathway, DNA replication pathway, and mismatch repair pathway (Figure 9B). These significantly altered pathways in the high-risk group were closely related to the malignant properties of PCa, and especially related to the BCR of PCa.
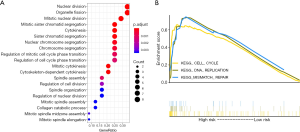
Discussion
Approximately 30% of PCa patients after RP would experience BCR. These patients only have a median period of between 5-8 years prior to clinical progression and require immediate intervention. Early prediction of BCR is therefore important to reduce the mortality of PCa, and a new predictive method to stratify PCa patients into those at high or low risk of BCR has significant clinical applications.
Our enhanced understanding of genetic changes during PCa has facilitated the identification of predictive and prognostic signatures; allowing personalized diagnoses and treatment management. Numerous studies have reported various genetic signatures; including mRNA, miRNA, and lncRNA signatures that could predict BCR (22-24). However, these signatures have different predictive potential, have not been well verified, and their clinical applications are poorly understood. At present, much of the sequencing data for PCa has been uploaded to the publicly available TCGA and GEO databases, which has allowed a comprehensive bioinformatic analyse to discover and validate novel prognostic signatures for PCa.
In this retrospective study, a 9-mRNA prognostic signature was identified which could predict the BCR of PCa in the TCGA dataset. Patients at high risk of BCR had a significantly poorer prognosis than those at low risk. Moreover, C-index and ROC analysis identified that the 9-mRNA signature was excellent for predicting the 3-, 5- and 10-year BCR events. Furthermore, the 9-mRNA signature was shown to be a strong prognostic and independent risk factor after adjusting for clinicopathological variables, including the GS, T staging, and PSA. We further validated the 9-mRNA signature in three external datasets (GSE21034, GSE70770, GSE116918) and found similar results. These results demonstrated that the 9-mRNA signature could effectively stratify patients into high- and low-risk groups, predict BCR-free survival probability, and was an effective prognostic factor for PCa. Subsequently, a nomogram combining the 9-mRNA signature and clinicopathologic features was created and validated in our study with the aim of aiding clinical decision-making for physicians. Notably, calibration plots demonstrated that the nomogram was effective for predicting the BCR in the discovery and validation sets.
Our 9-mRNA signature displayed better diagnostic potential with a 5-year AUC of 0.71 in the TCGA cohort, 0.81 in the GSE21034 cohort, 0.92 in the GSE70770 cohort, and 0.78 in the GSE116918 cohort. The mRNA-based nomogram could be used to stimulate new methods and ideas based on adjuvant therapies for future clinical research and stratify high- and low-risk BCR patients. Moreover, the C-index of the mRNA-based nomogram was higher than D’Amico model (9) in the TCGA and validation datasets. However, the 9-mRNA signature needs further clinical validation and we will conduct a long-term follow-up of PCa patients to verify our nomogram in the future.
In the 9-mRNA signature, EPHX2 mRNA has been associated with AR in primary PCa. Here, its inhibition could decrease AR signaling in vitro and enhance the effects of antiandrogenic flutamide in PCa cells (25). The presence of EPHX2 in the signature made us confident that the mRNA signature was reliable. Pai et al. (26) found that ASPM mRNA (a potential regulator of Wnt signaling) was significantly up-regulated in primary and metastatic PCa and was positively correlated with low BCR-free survival probability. MMP11 mRNA is associated with poor prognosis for PCa (27) and MMP11 expression in advanced PCa is significantly higher than in localized PCa (28). Aytes et al. discovered that co-expression of CENPF and FOXM1 is a signature for poor prognosis in PCa. Moreover, CENPF and FOXM1 act as synergistic master regulators to promote tumor growth and PCa malignancy (29). ASPN as a critical mesenchymal stromal cell factor is extensively affected by the PCa microenvironment and is correlated with PCa, BCR, and metastasis (30,31). The studies above highlighted that the inclusion of EPHX2, ASPM, MMP11, CENPF, and ASPN in the 9-mRNA signature was warranted. However, the roles of the remaining four mRNAs (C9orf152, KIF4A, COL1A1 and FANCI) in PCa are unknown. Additionally, these four mRNAs may have potential for molecular targeted therapy, as this study showed their association with biological pathways involved in PCa. Future studies will therefore focus on these four mRNAs and investigate their roles in PCa.
Conclusions
Here, we identified and validated a 9-mRNA signature which could predict the BCR of PCa, precisely stratify patients into those at high or low risk of BCR, and augment the ability of clinicians to decide on personalized therapies. However, further controlled trials are needed to validate our results and to test their clinical applications for individualized management of PCa.
Acknowledgments
Funding: This study was supported by grants from National Natural Science Foundation of China (No. 81600542 and 81670643); the Natural Science Foundation of Guangdong Province (2020A1515010464), the Guangdong Basic and Applied Basic Research Foundation (Grant NO. 2019A1515110033); Distinguished Young Talents in Higher Education Foundation of Guangdong Province (Grant NO. 2019KQNCX115 and 2020KZDZX1168); China Postdoctoral Science Foundation (Grant NO. 2019M662865); Achievement cultivation and clinical transformation application cultivation projects of the First Affiliated Hospital of Guangzhou Medical University (Grant NO. ZH201908).
Footnote
Reporting Checklist: The authors have completed the TRIPOD reporting checklist. Available at http://dx.doi.org/10.21037/tau-20-1019
Peer Review File: Available at http://dx.doi.org/10.21037/tau-20-1019
Conflicts of Interest: All authors have completed the ICMJE uniform disclosure form (available at http://dx.doi.org/10.21037/tau-20-1019). The authors have no conflicts of interest to declare.
Ethical Statement: The authors are accountable for all aspects of the work in ensuring that questions related to the accuracy or integrity of any part of the work are appropriately investigated and resolved. The study was conducted in accordance with the Declaration of Helsinki (as revised in 2013). The TCGA and GEO datasets were extracted from a public database and required no ethical approval.
Open Access Statement: This is an Open Access article distributed in accordance with the Creative Commons Attribution-NonCommercial-NoDerivs 4.0 International License (CC BY-NC-ND 4.0), which permits the non-commercial replication and distribution of the article with the strict proviso that no changes or edits are made and the original work is properly cited (including links to both the formal publication through the relevant DOI and the license). See: https://creativecommons.org/licenses/by-nc-nd/4.0/.
References
- Siegel RL, Miller KD, Jemal A. Cancer statistics, 2020. CA Cancer J Clin 2020;70:7-30. [Crossref] [PubMed]
- Liesenfeld L, Kron M, Gschwend JE, et al. Prognostic Factors for Biochemical Recurrence More than 10 Years after Radical Prostatectomy. J Urol 2017;197:143-8. [Crossref] [PubMed]
- Ludwig WW, Feng Z, Trock BJ, et al. Prostate Specific Antigen Testing after Radical Prostatectomy-Can We Stop at 20 Years? J Urol 2018;199:114-9. [Crossref] [PubMed]
- Pak S, You D, Jeong IG, et al. Time to biochemical relapse after radical prostatectomy and efficacy of salvage radiotherapy in patients with prostate cancer. Int J Clin Oncol 2019;24:1238-46. [Crossref] [PubMed]
- Cookson MS, Aus G, Burnett AL, et al. Variation in the definition of biochemical recurrence in patients treated for localized prostate cancer: the American Urological Association Prostate Guidelines for Localized Prostate Cancer Update Panel report and recommendations for a standard in the reporting of surgical outcomes. J Urol 2007;177:540-5. [Crossref] [PubMed]
- Cornford P, Bellmunt J, Bolla M, et al. EAU-ESTRO-SIOG Guidelines on Prostate Cancer. Part II: Treatment of Relapsing, Metastatic, and Castration-Resistant Prostate Cancer. Eur Urol 2017;71:630-42. [Crossref] [PubMed]
- Van den Broeck T, van den Bergh RCN, Arfi N, et al. Prognostic Value of Biochemical Recurrence Following Treatment with Curative Intent for Prostate Cancer: A Systematic Review. Eur Urol 2019;75:967-87. [Crossref] [PubMed]
- Brockman JA, Alanee S, Vickers AJ, et al. Nomogram Predicting Prostate Cancer-specific Mortality for Men with Biochemical Recurrence After Radical Prostatectomy. Eur Urol 2015;67:1160-7. [Crossref] [PubMed]
- D'Amico AV, Whittington R, Malkowicz SB, et al. Biochemical outcome after radical prostatectomy, external beam radiation therapy, or interstitial radiation therapy for clinically localized prostate cancer. JAMA 1998;280:969-74. [Crossref] [PubMed]
- Cooperberg MR, Pasta DJ, Elkin EP, et al. The University of California, San Francisco Cancer of the Prostate Risk Assessment score: a straightforward and reliable preoperative predictor of disease recurrence after radical prostatectomy. J Urol 2005;173:1938-42. [Crossref] [PubMed]
- Stephenson AJ, Scardino PT, Eastham JA, et al. Postoperative nomogram predicting the 10-year probability of prostate cancer recurrence after radical prostatectomy. J Clin Oncol 2005;23:7005-12. [Crossref] [PubMed]
- Mohler JL. The 2010 NCCN clinical practice guidelines in oncology on prostate cancer. J Natl Compr Canc Netw 2010;8:145. [Crossref] [PubMed]
- Knezevic D, Goddard AD, Natraj N, et al. Analytical validation of the Oncotype DX prostate cancer assay - a clinical RT-PCR assay optimized for prostate needle biopsies. BMC Genomics 2013;14:690. [Crossref] [PubMed]
- Shariat SF, Karakiewicz PI, Suardi N, et al. Comparison of Nomograms With Other Methods for Predicting Outcomes in Prostate Cancer: A Critical Analysis of the Literature. Clin Cancer Res 2008;14:4400-7. [Crossref] [PubMed]
- Lorent M, Maalmi H, Tessier P, et al. Meta-analysis of predictive models to assess the clinical validity and utility for patient-centered medical decision making: application to the CAncer of the Prostate Risk Assessment (CAPRA). BMC Med Inform Decis Mak 2019;19:2. [Crossref] [PubMed]
- Remmers S, Verbeek JFM, Nieboer D, et al. Predicting biochemical recurrence and prostate cancer-specific mortality after radical prostatectomy: comparison of six prediction models in a cohort of patients with screening- and clinically detected prostate cancer. BJU Int 2019;124:635-42. [Crossref] [PubMed]
- Amaro A, Esposito AI, Gallina A, et al. Validation of proposed prostate cancer biomarkers with gene expression data: a long road to travel. Cancer Metastasis Rev 2014;33:657-71. [Crossref] [PubMed]
- Hiser WM, Sangiorgio V, Bollito E, et al. Tissue-based multigene expression tests for pretreatment prostate cancer risk assessment: current status and future perspectives. Future Oncol 2018;14:3073-83. [Crossref] [PubMed]
- Spratt DE, Zhang J, Santiago-Jiménez M, et al. Development and Validation of a Novel Integrated Clinical-Genomic Risk Group Classification for Localized Prostate Cancer. J Clin Oncol 2018;36:581-90. [Crossref] [PubMed]
- Fine ND, LaPolla F, Epstein M, et al. Genomic Classifiers for Treatment Selection in Newly Diagnosed Prostate Cancer. BJU Int 2019. [Epub ahead of print]. [Crossref] [PubMed]
- Lin X, Kapoor A, Gu Y, et al. Assessment of biochemical recurrence of prostate cancer Int J Oncol 2019;55:1194-212. (Review). [Crossref] [PubMed]
- Penney KL, Sinnott JA, Fall K, et al. mRNA expression signature of Gleason grade predicts lethal prostate cancer. J Clin Oncol 2011;29:2391-6. [Crossref] [PubMed]
- Shao N, Tang H, Qu Y, et al. Development and validation of lncRNAs-based nomogram for prediction of biochemical recurrence in prostate cancer by bioinformatics analysis. J Cancer 2019;10:2927-34. [Crossref] [PubMed]
- Zhao Z, Weickmann S, Jung M, et al. A Novel Predictor Tool of Biochemical Recurrence after Radical Prostatectomy Based on a Five-MicroRNA Tissue Signature. Cancers (Basel) 2019;11:1603. [Crossref] [PubMed]
- Vainio P, Gupta S, Ketola K, et al. Arachidonic acid pathway members PLA2G7, HPGD, EPHX2, and CYP4F8 identified as putative novel therapeutic targets in prostate cancer. Am J Pathol 2011;178:525-36. [Crossref] [PubMed]
- Pai VC, Hsu CC, Chan TS, et al. ASPM promotes prostate cancer stemness and progression by augmenting Wnt-Dvl-3-β-catenin signaling. Oncogene 2019;38:1340-53. [Crossref] [PubMed]
- Roscilli G, Cappelletti M, De Vitis C, et al. Circulating MMP11 and specific antibody immune response in breast and prostate cancer patients. J Transl Med 2014;12:54. [Crossref] [PubMed]
- Fernandez-Gomez JM, Eiro N, García-Rodríguez JJ, et al. Metalloproteinase 11, potential marker and molecular target in advanced and castration-resistant prostate cancer. Culture study of peritumoral fibroblasts. Actas Urol Esp 41:376-82. [Crossref] [PubMed]
- Aytes A, Mitrofanova A, Lefebvre C, et al. Cross-species regulatory network analysis identifies a synergistic interaction between FOXM1 and CENPF that drives prostate cancer malignancy. Cancer Cell 2014;25:638-51. [Crossref] [PubMed]
- Rochette A, Boufaied N, Scarlata E, et al. Asporin is a stromally expressed marker associated with prostate cancer progression. Br J Cancer 2017;116:775-84. [Crossref] [PubMed]
- Hughes RM, Simons BW, Khan H, et al. Asporin Restricts Mesenchymal Stromal Cell Differentiation, Alters the Tumor Microenvironment, and Drives Metastatic Progression. Cancer Res 2019;79:3636-50. [Crossref] [PubMed]