Myeloid-derived suppressor cell (MDSC) key genes analysis in rat anti-CD28-induced immune tolerance kidney transplantation
Introduction
Immunological tolerance is the state in which the immune system shows unresponsiveness towards specific antigens like allografts while normally responding to other harmful foreign antigens (1,2). In kidney transplantation, the high rejection rate as well as many morbidities like nephrotoxicity are critical (3,4). Because of the rare appearance of spontaneous tolerance in normal cases (5), the ability to induce immune tolerance in transplant recipients would be of great significance. Current methods for inducing kidney transplantation immune tolerance are bone marrow transplantation (6), using regulatory immune cells including myeloid-derived suppressor cells (MDSCs) (7), regulatory T cells (Tregs) (8), regulatory B cells (9) and mesenchymal stem cell (MSC) infusion (10).
MDSCs are heterogeneous progenitor and immature myeloid cells (11). They play a significant role in immune tolerance by virtue of multiple strong cell-cell interactions with T cells. MDSCs can secrete reactive oxygen species and nitric oxide to suppress T cell responses (12) and disrupt cell binding by nitration of tyrosine (13). Recent reports from Vanhove showed that MDSCs in rat kidney transplant recipients treated with anti-CD28 could induce immune tolerance (14,15).
In transplant tolerance, Tregs are thought to play a leading role (8) and MDSCs can regulate and interact with Tregs in direct and indirect ways by building a graft-to-periphery gradient to recruit Tregs and maintain tolerance (15). MDSCs also have the remarkable ability to regulate the differentiation of Tregs through cell-cell contacts and the production of cytokines (11,14). Finally, MDSCs cooperate with Tregs to prolong allograft survival (16). These studies suggest that cell therapies based on MDSCs may become an important research direction for the induction of transplant tolerance.
Microarrays are a widely used high-throughput tool which can generate gene expression profiles, and further analysis of microarray data may reveal the etiology of phenotypic differences. A previous study published a gene expression profile comparing blood MDSCs in syngenic and tolerant rat kidney transplant recipients (15). In this study, we performed further analysis of this microarray data to better understand the factors affecting transplant tolerance. By using multiple bioinformatics methods to analyze the public gene expression profiles, differentially expressed genes (DEGs) in the tolerant and syngenic samples were identified based on use of the Linear Models for Microarray Data (LIMMA) package in the R environment. Gene ontology (GO) and Kyoto Encyclopedia of Genes and Genomes (KEGG) pathways were analyzed in the Database for Annotation, Visualization and Integrated Discovery (DAVID). A protein-protein interaction (PPI) network was built in the STRING database and visualized in Cytoscape. Utilizing these bioinformatic tools, the gene expression profiles of tolerant and syngenic samples after kidney transplantation could be better visualized.
We present the following article in accordance with the MDAR reporting checklist (available at http://dx.doi.org/10.21037/tau-20-943).
Methods
Microarray data
From the Gene Expression Omnibus (GEO) database (https://www.ncbi.nlm.nih.gov/geo/), we extracted microarray data from accession number GSE28545 (species: Rattus norvegicus; platform: GPL2996; samples: GSM706861–GSM706865) (15), which compared the levels of blood MDSCs from three tolerant allogeneic kidney transplant recipients with two syngenic kidney transplant recipients at 100 days post-transplantation, which is the only dataset in GEO database focusing on the following issue with great data integrity. To verify the feasibility for cross-comparison, we used the value distribution of the GEO2R tool (17) (https://www.ncbi.nlm.nih.gov/geo/geo2r/) in the GEO database. All procedures performed in this study were in accordance with the Declaration of Helsinki (as revised in 2013).
Data processing and identification of DEGs
To determine DEGs in tolerant and syngenic kidney transplant recipients, the affy and LIMMA packages in Bioconductor were used in the R language environment (2), which also helped us in statistical analysis. Comparisons between expression values were carried out in R language by Bayes tests. T-tests were applied to the original expression data, and DEGs were selected based on a threshold corresponding adjusted P value <0.05 and a log fold change >1.5. The gplots package in Bioconductor was used to display the heatmap of the DEGs using different colors to visualize the regulated DEGs.
Enrichment analysis of DEGs
Enrichment analysis of the DEGs was conducted in the DAVID database (https://david.ncifcrf.gov/) (18) using GO and KEGG pathway analysis (19). In GO, biological process (BP), cellular components (CC) and molecular function (MF) of the DEGs were analyzed using a threshold P value <0.05 and a gene count >10, while the threshold for KEGG pathways was P<0.05 and gene count >5.
PPI network construction
By inputting the gene symbols of all DEGs into the STRING database (https://string-db.org/) (20), the interactions between proteins were predicted, with Rattus norvegicus as the chosen species and the medium confidence score set at 0.4. After exporting the string interactions, the network was visualized using Cytoscape software (http://www.cytoscape.org/) (21).
Hub gene analysis
CytoHubba (http://apps.cytoscape.org/apps/cytohubba) was used to determine the hub genes in the entire PPI network (22). CytoHubba provides 12 algorithms to determine the significance of the nodes. After calculating the node scores in different algorithms, the hubba nodes indicating hub genes were selected.
Statistical analysis
According to the results calculated as above, DEGs whose P value <0.05 and log fold change >1.5 were selected. Statistical analyses were performed with R language. A t-test was used to compare tolerant and syngeneic samples. Two-tailed statistical tests with P≤0.05 were considered as statistically significant.
Results
Identification of DEGs
Given that the distribution of value data was at the same level, the feasibility for cross-comparison could be ensured (Figure 1). Based on the expression values in dataset GSE28545, 338 DEGs were obtained. Among the DEGs, 27 genes were upregulated and 311 genes were downregulated in samples from tolerant allogeneic kidney transplant recipients compared to syngenic samples. The heatmap of the DEGs is shown in Figure 2.
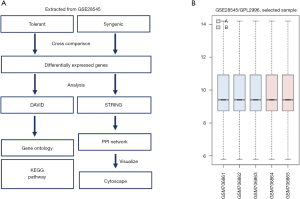
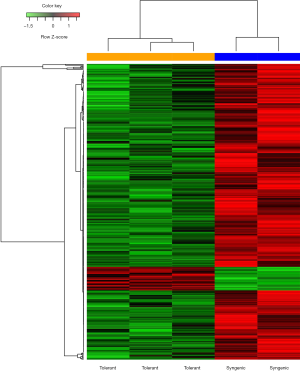
Enrichment analysis for the DEGs
From the GO analysis in the DAVID database, the top six GO terms in BP, CC and MF are displayed in Figure 3. In the BP ontology, we found that response-related items were the most significant GO categories, including response to organic cyclic compound (17 genes) and response to drug (22 genes). In addition, positive regulation of transcription was also among the top categories, including DNA-templated positive regulation of transcription (19 genes) and positive regulation of transcription from the RNA polymerase II promoter (26 genes). Inflammatory response (12 genes) and immune response (11 genes) were also enriched.
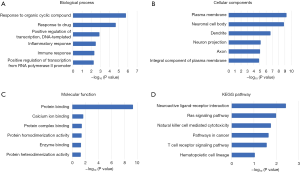
In the CC ontology, most genes were associated with the plasma membrane (98 genes). Neurons were also closely related to the DEGs, with the most prominent enriched categories including neuronal cell body (29 genes), dendrite (24 genes), neuron projection (19 genes) and axon (18 genes).
In the MF ontology, binding-related items made up the majority of the categories, with protein binding (56 genes) as most enriched, followed by calcium ion binding (18 genes), protein complex binding (11 genes) and enzyme binding (11 genes). In addition, other enriched categories in MF were related to protein activity: for instance, protein homodimerization activity (19 genes) and protein heterodimerization activity (14 genes).
Furthermore, through KEGG pathway analysis, we found that neuroactive ligand-receptor interaction category (15 genes) was most closely related to the DEGs, verifying the results of the CC ontology. Ras signaling pathway (12 genes) was the primary term, followed by immune-related pathways including natural killer cell mediated cytotoxicity (7 genes) and T cell receptor signaling pathway (7 genes). The genes included in the KEGG pathways are shown in Table 1.
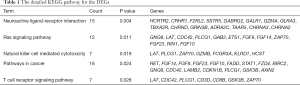
Full table
PPI network construction
The string interactions among the DEGs were exported from STRING and imported into Cytoscape. The entire PPI network consisted of 192 nodes and 469 edges and is shown in Figure 4, in which upregulated genes are colored red. Meanwhile, the sizes of the nodes were continuously mapped, with nodes of smaller P values having a rather large size.
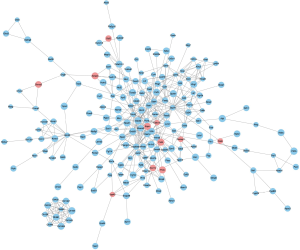
Hub gene analysis
In cytoHubba, we selected the top 15 hub genes ranked by degree of connectivity. The degrees of connectivity for the hub genes are listed in Table 2. Frequently studied genes like Zap70, Cdc42, Stat1, Stat4, Ccl5 and Cxcr3 are in this list and are also among the most frequent genes determined by other algorithms. Precisely calculated by cytoscape, these genes have the strongest interactions with each other (Figure 5) and the other DEGs, and formed the key cluster of the whole PPI interaction, so we predict they will play key roles in immune tolerance in the case.
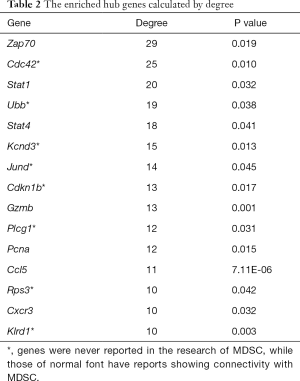
Full table
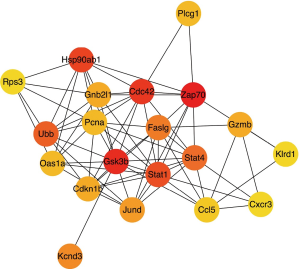
Discussion
Anti-CD28 therapy has been presented as an effective immune regulatory strategy by preventing T cell co-stimulation while favoring co-inhibition, since inhibitory signals transmitted through CTLA-4, PD-L1 and B7 would not be affected (23). Preventing CD28 access to its ligands with the CD80/86 antagonists abatacept (Orencia1; Bristol-Myers Squibb, New York, NY, USA) and belatacept (Nulogix1; Bristol-Myers Squibb) has demonstrated immunosuppressive efficacy in the clinic (24). The most ideal clinical application for MDSC therapy is tolerance induction. MDSCs play an important role in anti-graft rejection and immune tolerance induction. The therapeutic value of MDSCs has been recognized in patients with cancer, inflammation and autoimmune disease (7).
In this study, we identified DEGs in MDSCs comparing tolerant and non-tolerant recipients of rat kidney transplants. We then analyzed their functions by GO annotation and KEGG pathway enrichment. Finally, the interrelationships among them were investigated using PPI network construction. As a result, hub genes were identified which may play important roles in MDSC function and could be potential targets for therapy involving MDSC-induced immune tolerance.
Through GO annotation in the DAVID database, we analyzed the biological function of the DEGs. First, in the CC ontology, we found that the majority of the DEGs were enriched in cell membrane-related items, while other DEGs were enriched in non-membrane-related items such as extracellular matrix and actin cytoskeleton. The results reflect that tolerance likely occurs through complex cellular molecular mechanisms involving both membrane and non-membrane structure. Thus, in the BP GO category, our data showed that the most significant items were stimulus-related terms such as extra-cellular response. Other enriched categories in BP included items associated with response to organ cyclic compound, response to drug, positive regulation of transcription and immune responses. In the CC ontology, DEGs showed associations with plasma membrane, neuronal cell body, dendrite, neuron projection and axon. In the MF ontology, protein binding showed a significant enrichment in the DEGs, followed by calcium ion binding, protein complex binding and enzyme binding.
KEGG pathway analysis can reveal more precise biological functions of genes than GO analysis. In the present study, three pathways were enriched, including cytokine-cytokine receptor interaction and pathways in immune tolerance which may participate in neuroactive ligand-receptor interaction, Ras signaling pathway, natural killer cell mediated cytotoxicity and pathways in cancer. Some of these pathways have already been documented in recent studies; for example, Ras signaling plays an important role during myeloid development. Studies have also shown recruitment of macrophages and MDSCs into the tumor stroma resulting in suppression of the anti-tumor response (25).
With 192 nodes and 469 edges constituting the entire PPI network, a series of hub proteins was observed to form a local network including Espn, Cdkn1b, F2d4, Gorasp1, Pnck and Acvr1c. For hub gene analysis, the affy and LIMMA packages in Bioconductor were employed in the R language environment. Using these tools, we obtained 338 DEGs in MDSCs from rat transplant recipients treated with and without anti-CD28, including 27 upregulated and 311 downregulated DEGs. Among the DEGs, a number of them showed a remarkable fold change (greater than 5) in anti-CD28-treated rats compared with non-treated rats, including Zap70, Stat1, Stat4, Gzmb, Pcna, Ccl5 and Cxcr3, with 29, 20, 18, 13, 12, 11 and 10 degrees of connectivity, respectively. Therefore, we hypothesize that the DEGs are potential biomarkers for distinguishing or predicting anti-CD28-induced immune tolerance. However, the mechanism remains unclear and further verification experiments are needed. In addition, evidence indicates that some DEGs may contribute to the induction of immune tolerance by anti-CD28. By binding of tSH2 domain and the doubly-phosphorylated ITAM motifs of CD3 chains, ZAP70 is recruited in the T-cell receptor (TCR) complex (26), and contributed to T cell-mediated immunological diseases (27). The frequency of ZAP70 cells was significantly correlated with monocytic MDSCs (M-MDSCs) level (28). GzmB expression was found in both mice and human MDSC, B16F10 melanoma cells decreased in invasive potential co-cultured with perforin/GzmB−/− MDSCs (29). STAT4 are major hubs regulating MDSC-derived macrophages in anti-tumor process (30). Another study focusing on in head and neck squamous cell carcinoma showed STAT1 inhibits MDSC accumulation in T cell-mediated antitumor immune responses (31). For example, CCL5 was shown to contribute to MDSC immunosuppression by establishing a graft-to-periphery CCL5 gradient in tolerant kidney allograft recipients which controlled recruitment of Tregs to the graft, where they likely participated in maintaining tolerance (15). Another study suggested that administration of resveratrol into IL-10−/− mice induced immunosuppressive CD11b+/Gr-1+ MDSCs in the colon, which correlated with reversal of established chronic colitis and downregulation of mucosal and systemic CXCR3+-expressing effector T cells as well as inflammatory cytokines in the colon (32). Jiang et al. demonstrated that suppression of IL-6-dependent suppressor of cytokine signaling 3 in MDSCs induced phosphorylation of JAK1, JAK2, TYK2, STAT1 and STAT3 proteins, which correlated with T cell suppression in MDSCs in vitro (33). Taken together, these studies suggest that the DEGs identified in our study may contribute to anti-CD28-induced immune tolerance through complex mechanisms.
Conclusions
In summary, our study provided preliminary research into the mechanisms of MDSCs in anti-CD28-induced immune tolerance in rat kidney transplantation. DEGs in tolerant and immunoreactive groups were screened by computational bioinformatics methods. In addition, hub genes and signaling pathways were identified as potential targets in MDSCs from immune tolerant rats. Greater insights may thus be obtained into the etiology and mechanisms of transplant tolerance with potential clinical applications for kidney transplant patients.
Acknowledgments
Funding: This study was supported by the National Key R&D Program of China (2018YFA0107501 to Ruiming Rong, 2018YFA0107502 to Cheng Yang), the National Natural Science Foundation of China (81770747 to Ruiming Rong, 81770746 to Cheng Yang), Shanghai Rising-Star Program (19QA1406300 to Cheng Yang) and the Medical, Health Talents Training Plan for the Excellent Youth of Shanghai Municipal (2018YQ50 to Cheng Yang) and 2019 Shanghai Youth Talent Development Program.
Footnote
Reporting Checklist: The authors have completed the MDAR reporting checklist. Available at http://dx.doi.org/10.21037/tau-20-943
Peer Review File: Available at http://dx.doi.org/10.21037/tau-20-943
Conflicts of Interest: All authors have completed the ICMJE uniform disclosure form (available at http://dx.doi.org/10.21037/tau-20-943). The authors have no conflicts of interest to declare.
Ethical Statement: The authors are accountable for all aspects of the work in ensuring that questions related to the accuracy or integrity of any part of the work are appropriately investigated and resolved. All procedures performed in this study were in accordance with the Declaration of Helsinki (as revised in 2013).
Open Access Statement: This is an Open Access article distributed in accordance with the Creative Commons Attribution-NonCommercial-NoDerivs 4.0 International License (CC BY-NC-ND 4.0), which permits the non-commercial replication and distribution of the article with the strict proviso that no changes or edits are made and the original work is properly cited (including links to both the formal publication through the relevant DOI and the license). See: https://creativecommons.org/licenses/by-nc-nd/4.0/.
References
- Sykes M. Immune tolerance in recipients of combined haploidentical bone marrow and kidney transplantation. Bone Marrow Transplant 2015;50 Suppl 2:S82-6. [Crossref] [PubMed]
- Ritchie ME, Phipson B, Wu D, et al. limma powers differential expression analyses for RNA-sequencing and microarray studies. Nucleic Acids Res 2015;43:e47. [Crossref] [PubMed]
- Lamb KE, Lodhi S, Meier-Kriesche HU. Long-term renal allograft survival in the United States: a critical reappraisal. Am J Transplant 2011;11:450-62. [Crossref] [PubMed]
- Morris H, DeWolf S, Robins H, et al. Tracking donor-reactive T cells: Evidence for clonal deletion in tolerant kidney transplant patients. Sci Transl Med 2015;7:272ra10. [Crossref] [PubMed]
- Cippà PE, Fehr T. Spontaneous tolerance in kidney transplantation--an instructive, but very rare paradigm. Transpl Int 2011;24:534-5. [Crossref] [PubMed]
- Kawai T, Leventhal J, Wood K, et al. Summary of the Third International Workshop on Clinical Tolerance. Am J Transplant 2019;19:324-30. [Crossref] [PubMed]
- Zhang W, Li J, Qi G, et al. Myeloid-derived suppressor cells in transplantation: the dawn of cell therapy. J Transl Med 2018;16:19. [Crossref] [PubMed]
- Martin-Moreno PL, Tripathi S, Chandraker A, Regulatory T. Cells and Kidney Transplantation. Clin J Am Soc Nephrol 2018;13:1760-4. [Crossref] [PubMed]
- Peng B, Ming Y, Yang C. Regulatory B cells: the cutting edge of immune tolerance in kidney transplantation. Cell Death Dis 2018;9:109. [Crossref] [PubMed]
- Casiraghi F, Perico N, Gotti E, et al. Kidney transplant tolerance associated with remote autologous mesenchymal stromal cell administration. Stem Cells Transl Med 2020;9:427-32. [Crossref] [PubMed]
- Gabrilovich DI, Nagaraj S. Myeloid-derived suppressor cells as regulators of the immune system. Nat Rev Immunol 2009;9:162-74. [Crossref] [PubMed]
- Kusmartsev S, Su Z, Heiser A, et al. Reversal of myeloid cell-mediated immunosuppression in patients with metastatic renal cell carcinoma. Clin Cancer Res 2008;14:8270-8. [Crossref] [PubMed]
- Nagaraj S, Gupta K, Pisarev V, et al. Altered recognition of antigen is a mechanism of CD8+ T cell tolerance in cancer. Nat Med 2007;13:828-35. [Crossref] [PubMed]
- Dugast AS, Haudebourg T, Coulon F, et al. Myeloid-derived suppressor cells accumulate in kidney allograft tolerance and specifically suppress effector T cell expansion. J Immunol 2008;180:7898-906. [Crossref] [PubMed]
- Dilek N, Poirier N, Usal C, et al. Control of transplant tolerance and intragraft regulatory T cell localization by myeloid-derived suppressor cells and CCL5. J Immunol 2012;188:4209-16. [Crossref] [PubMed]
- Adeegbe D, Serafini P, Bronte V, et al. In vivo induction of myeloid suppressor cells and CD4(+)Foxp3(+) T regulatory cells prolongs skin allograft survival in mice. Cell Transplant 2011;20:941-54. [Crossref] [PubMed]
- Barrett T, Wilhite SE, Ledoux P, et al. NCBI GEO: archive for functional genomics data sets--update. Nucleic Acids Res 2013;41:D991-5. [Crossref] [PubMed]
- Huang DW, Sherman BT, Tan Q, et al. DAVID Bioinformatics Resources: expanded annotation database and novel algorithms to better extract biology from large gene lists. Nucleic Acids Res 2007;35:W169-75. [Crossref] [PubMed]
- Kanehisa M, Goto S, Sato Y, et al. Data, information, knowledge and principle: back to metabolism in KEGG. Nucleic Acids Res 2014;42:D199-205. [Crossref] [PubMed]
- Szklarczyk D, Franceschini A, Wyder S, et al. STRING v10: protein-protein interaction networks, integrated over the tree of life. Nucleic Acids Res 2015;43:D447-52. [Crossref] [PubMed]
- Saito R, Smoot ME, Ono K, et al. A travel guide to Cytoscape plugins. Nat Methods 2012;9:1069-76. [Crossref] [PubMed]
- Chin CH, Chen SH, Wu HH, et al. cytoHubba: identifying hub objects and sub-networks from complex interactome. BMC Syst Biol 2014;8 Suppl 4:S11. [Crossref] [PubMed]
- Poirier N, Mary C, Dilek N, et al. Preclinical efficacy and immunological safety of FR104, an antagonist anti-CD28 monovalent Fab' antibody. Am J Transplant 2012;12:2630-40. [Crossref] [PubMed]
- Poirier N, Dilek N, Mary C, et al. FR104, an antagonist anti-CD28 monovalent fab' antibody, prevents alloimmunization and allows calcineurin inhibitor minimization in nonhuman primate renal allograft. Am J Transplant 2015;15:88-100. [Crossref] [PubMed]
- Clark CE, Hingorani SR, Mick R, et al. Dynamics of the immune reaction to pancreatic cancer from inception to invasion. Cancer Res 2007;67:9518-27. [Crossref] [PubMed]
- Gangopadhyay K, Manna B, Roy S, et al. An allosteric hot spot in the tandem-SH2 domain of ZAP-70 regulates T-cell signaling. Biochem J 2020;477:1287-308. [Crossref] [PubMed]
- Takeuchi Y, Hirota K, Sakaguchi S. Impaired T cell receptor signaling and development of T cell-mediated autoimmune arthritis. Immunol Rev 2020;294:164-76. [Crossref] [PubMed]
- Zahran AM, Moeen SM, Thabet AF, et al. Monocytic myeloid-derived suppressor cells in chronic lymphocytic leukemia patients: a single center experience. Leuk Lymphoma 2020;61:1645-52. [Crossref] [PubMed]
- Dufait I, Pardo J, Escors D, et al. Perforin and Granzyme B Expressed by Murine Myeloid-Derived Suppressor Cells: A Study on Their Role in Outgrowth of Cancer Cells. Cancers (Basel) 2019;11:808. [Crossref] [PubMed]
- Bayik D, Tross D, Klinman DM. Factors Influencing the Differentiation of Human Monocytic Myeloid-Derived Suppressor Cells Into Inflammatory Macrophages. Front Immunol 2018;9:608. [Crossref] [PubMed]
- Ryan N, Anderson K, Volpedo G, et al. STAT1 inhibits T-cell exhaustion and myeloid derived suppressor cell accumulation to promote antitumor immune responses in head and neck squamous cell carcinoma. Int J Cancer 2020;146:1717-29. [Crossref] [PubMed]
- Singh UP, Singh S, Iqbal N, et al. IFN-gamma-inducible chemokines enhance adaptive immunity and colitis. J Interferon Cytokine Res 2003;23:591-600. [Crossref] [PubMed]
- Jiang M, Chen J, Zhang W, et al. Interleukin-6 Trans-Signaling Pathway Promotes Immunosuppressive Myeloid-Derived Suppressor Cells via Suppression of Suppressor of Cytokine Signaling 3 in Breast Cancer. Front Immunol 2017;8:1840. [Crossref] [PubMed]