Establishment and validation of a novel predictive model to quantify the risk of bone metastasis in patients with prostate cancer
Introduction
Prostate cancer (PCa) is the second most common malignancy among men and ranks as the fifth leading cause of cancer death worldwide (1). In the United States, PCa is the most commonly diagnosed cancer among men, with an estimated 174,650 new cases (20% of all cases) and 31,620 cancer-related deaths (10% of all cases) (2). However, despite the increasing survival rates of patients with PCa due to recent advancements in comprehensive treatment, metastatic disease is still related to a high mortality rate (3,4). Only 20–30% of metastatic PCa patients live to 5 years after diagnosis, and approximately 85% of cases suffer from bone metastasis (5,6). As the most common metastasis type in advanced PCa patients (7), bone metastasis seriously affects the quality of life due to skeletal complications (8). Early diagnosis and early treatment with antiresorptive drugs or radiopharmaceuticals can improve quality of life and even lead to better survival (9). However, according to the National Comprehensive Cancer Network (NCCN) guidelines, asymptomatic patients with PCa are not recommended for routine assessment or skeletal imaging (10). Furthermore, even if patients underwent imaging examinations, such as computed tomography (CT), magnetic resonance imaging (MRI), or bone scans, the recurrence of the disease may not be diagnosed in time because of the low sensitivity of these techniques (11). Thus, the prediction of bone metastasis is essential for choosing therapeutic strategies.
Although some biological parameters and clinical factors have been verified to be related to bone metastasis in patients with PCa, the relevant studies only analyzed scattered factors or had inadequate sample sizes (12-14). A previous study assessed several clinical characteristics in promoting bone metastasis based on large sample size (15). However, more comprehensive and in-depth analyses have not been performed. Therefore, this study aimed to investigate the clinicopathological factors related to bone metastasis, and the second aim was to establish an accurate predictive model for quantifying the risk of bone metastasis. An effective nomogram is a reliable graphical calculating tool that can predict outcomes with a combination of all risk variables, benefiting both doctors and patients (16). In this study, patients with PCa were screened and selected from the Surveillance, Epidemiology, and End Results (SEER) database to establish a novel nomogram for predicting bone metastasis. Compared with several similar models for predicting bone metastasis in PCa patients in recent studies (17,18), the model developed in this study displayed better predictive performance, and was based on larger population-based sample size. Moreover, we identified bone metastasis prediction points as a new independent factor for the overall survival of patients with PCa. Furthermore, decision curve analysis (DCA) and clinical impact curves (CIC) analyses were performed to investigate the model’s clinical value. With this tool, clinicians can evaluate bone metastasis’s risk in patients with PCa to provide them with appropriate examinations and individualized treatment.
We present the following article in accordance with the TRIPOD reporting checklist (available at http://dx.doi.org/10.21037/tau-20-1133).
Methods
Patients
The SEER database covers approximately 30% of the total American population and contains patients from different regions, including demographics, tumor morphology, staging system, and metastasis status (19). Information on patients diagnosed with PCa between 2010 and 2015 was retrieved from the SEER 18 Regs Research Data database using SEER-Stat 8.3.5 software, downloaded from https://seer.cancer.gov (20). The exclusion criteria were as follows: (I) patients diagnosed with more than one primary cancer; (II) aged <18 years; (III) diagnostic confirmation only based on clinical performance or without microscopic analysis; (IV) clinical background characteristics (age, race, and marital status), T stage, N stage, prostate-specific antigen (PSA) level, Gleason score, or metastasis status were unknown or untested. The study was conducted in accordance with the Declaration of Helsinki (as revised in 2013). As data from the SEER program is available for the public and does not need patient informed consent, and ethical approval was waived by the local Ethics Committee of the First Affiliated Hospital of Soochow University.
Study variables
Information on several clinicopathological characteristics was extracted for each patient. Age at diagnosis as a continuous variable was converted to a categorical variable based on the median value of 65. Patients were divided into four groups based on the eighth American Joint Committee on Cancer (AJCC) T stage, and two groups based on the eighth AJCC N stage (21). PSA level was classified as ≤20 and >20 ng/mL, which met the classification criteria of the NCCN guidelines (10). According to the consensus of the International Society of Urological Pathology (ISUP) in 2014, Gleason score was divided into five levels: grade 1 for score ≤6, grade 2 for score =3+4, grade 3 for score =4+3, grade 4 for score =8, grade 5 for score =9 or 10 (22), and patients were divided into these five groups accordingly. Marital status (married or unmarried), brain metastasis (absence or presence), liver metastasis (absence or presence), and lung metastasis (absence or presence) were considered as different categorical factors. Bone metastasis was the primary outcome variable in this study.
Nomogram construction and validation
All cases were randomly assigned to the training cohort and the validation cohort in a 1:1 ratio. After univariate unadjusted logistic regression analysis in the training cohort, significant variables (P<0.05) were further added into the multivariate logistic regression model to acquire the independent factors that predict bone metastasis in patients with PCa. The most significant variables were used to construct a visual prediction nomogram through the forward stepwise selection method in multivariable analysis (23). The concordance index (c-index) was used to evaluate the discrimination performance of the nomogram. A strong predictive power model usually has a high c-index, which fluctuates between 0.5 and 1.0 (24). The nomogram’s calibration power was validated by the calibration plots that showed the relationship between the predicted probabilities of bone metastasis and the actual ones. To reduce overfit bias, a bootstrapping method with 1,000 resamples was used in nomogram validation. Furthermore, the comparability between the predictive accuracy of the nomogram and that of other factors was measured based on receiver operating characteristic (ROC) curves. A larger value of the area under the ROC curve (AUC) indicated a stronger predictive power (25). All validation means mentioned above were used in both the training cohort and the validation cohort.
Clinical performance
The nomogram’s clinical usefulness was assessed by quantifying the net benefit (NB) at each risk in DCA (26,27). By calculating the number of true positive cases minus false-positive cases and weighing the correlative hazards brought by abandoning interventions against the negative effects of unnecessary treatment, the NB of each probability was eventually calculated (28). Also, according to the consequence of DCA, a CIC was generated to evaluate the clinical performance of the nomogram further. At each threshold probability, the number of patients classified as high risk in a nomogram and the outcome of bone metastasis was both displayed in the CIC (29).
Statistical analyses
A Student’s t-test or chi-square test was used to analyze continuous clinical characteristics and categorical variables. Kaplan-Meier and log-rank tests were performed to predict overall survival, and the Cox regression model was used to identify the independent factors for prognosis. All the statistical analyses, including univariate and multivariate logistic regression analysis, were performed using IBM SPSS Statistics software (version 24.0, SPSS Inc., Chicago, USA). Nomogram, c-index, calibration curves, ROC, DCA, and CIC, were calculated and visualized in R software (version 3.51, Foundation for Statistical Computing, Vienna, Austria) with relevant R packages, such as rms, Hmisc, calibrate ROCR, and rmda. All tests were two-sided, and P<0.05 was deemed statistically significant.
Results
Patient characteristics
The clinicopathological characteristics of patients with PCa in both groups are displayed in Table 1. A total of 168,414 cases, consisting of half in the training set and a half in the validation set, were eventually extracted from the SEER database after meeting the inclusion criteria. The mean age of the total cohort was 64.69 years, and the median age was 65 years. In this study, 2,607 cases in the training set and 2,747 cases in the validation set had bone metastasis at initial diagnosis, and the P value of the bone metastasis rate between the two sets was 0.052. All the P values of the chi-square tests and the Student’s t-tests in Table 1 were larger than 0.05, indicating no statistically significant differences between the two cohorts. Ten variables, including age, race, marital status, T stage, N stage, PSA level, Gleason grade, brain metastasis, liver metastasis, and lung metastasis, were significantly related to bone metastasis in both sets, all with P values <0.05 (Table 2).
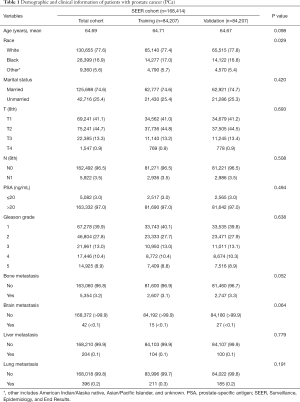
Full table
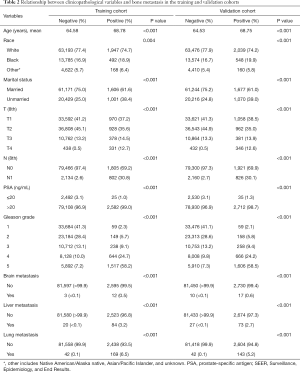
Full table
Independent predictors in the training set
Seven variables, including age, race, marital status, T stage, N stage, PSA level, and Gleason grade, which were significantly related to bone metastasis after univariate unadjusted logistic regression analysis (P<0.05), were put into the multivariate-adjusted logistic regression analyses using the stepwise selection technique. As shown in model 1 of Table 3, six variables were considered independent factors. In model 2 of Table 3, the other 3 variables describing distant metastasis status were added into the predictive system, and eventually, all of them were independently associated with bone metastasis after logistic regression analyses. As shown in predictive model 2, there were nine independent indicators for bone metastasis in PCa, including age, marital status, T stage, N stage, PSA level, Gleason grade, brain metastasis, liver metastasis and lung metastasis. Age >65 years, unmarried status, tumor with T4 stage, regional lymph node metastasis, PSA level >20 ng/mL, Gleason grade 5, and a diagnosis of other distant metastases were all high-risk factors for bone metastasis.
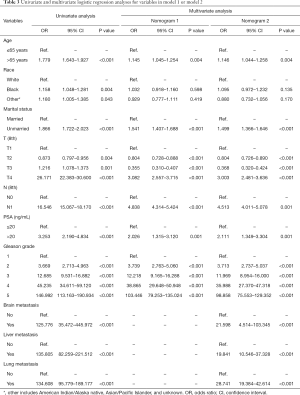
Full table
Construction and validation of nomograms
Six independent predictors in model 1 were incorporated into the construction of nomogram 1 to predict bone metastasis in patients with PCa (A, Figure 1). The nomogram displayed excellent predictive accuracy, with c-indexes of 0.911 (95% CI: 0.906–0.916, P<0.001) in the training set and 0.910 (95% CI: 0.905–0.915, P<0.001) in the validation set. Both the internal and external calibration curves reflected that the nomogram predicted metastasis well (Figure 2). A combination of the other three statistically pronounced metastatic sites and the clinicopathological predictors mentioned above were used to develop a new nomogram (nomogram 2, B, Figure 1). The c-indexes for the training and the validation sets were 0.917 (95% CI: 0.912–0.922, P<0.001) and 0.915 (95% CI: 0.910–0.920, P<0.001), respectively. The internal and external calibration plots of nomogram 2 were slightly closer to 45 degrees than those of nomogram 1 (Figure 2). These results suggest that the predictive model displayed slightly superior predictive performance when other distant metastatic sites were added into this predictive system. Also, the risk of bone metastasis in PCa patients could be calculated by adding up the detailed points given in Table 4 of each factor. High-risk factors, such as age >65 years, unmarried status, tumor with T4 stage, regional lymph node metastasis, PSA level >20 ng/mL, Gleason grade 5, and a diagnosis of other distant metastases, achieved the highest scores in each group.
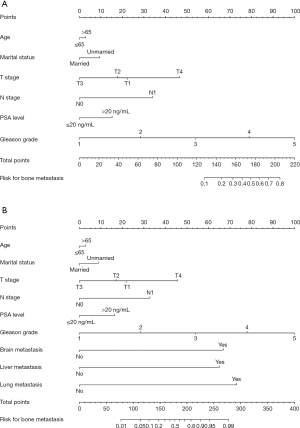
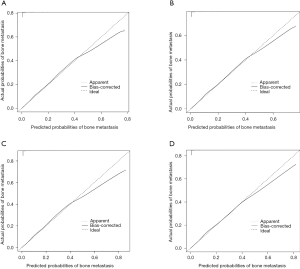
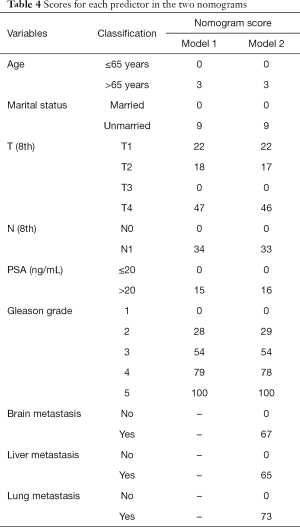
Full table
Comparison of nomograms and other factors
To compare the predictive performance of the two nomograms and other risk factors, we conducted ROC analysis in this study. For the training cohort, the AUC value of nomogram 2 was 0.911 (95% CI: 0.906–0.916, P<0.001), which was slightly higher than that of nomogram 1 (0.904, 95% CI: 0.989–0.909, P<0.001), and significantly higher than those of Gleason grade (0.883, 95% CI: 0.878–0.890, P<0.001), N stage (0.641, 95% CI: 0.628–0.653, P<0.001) and T stage (0.565, 95% CI: 0.552–0.577, P<0.001) (Figure 3A). For the validation cohort, the results of the ROC analyses were similar. The AUC value of nomogram 2 was 0.910 (95% CI: 0.905–0.915, P<0.001), which was slightly higher than that of nomogram 1 (0.904, 95% CI: 0.899–0.909, P<0.001), and significantly higher than those of Gleason grade (0.884, 95% CI: 0.879–0.890, P<0.001), N stage (0.637, 95% CI: 0.625–0.649, P<0.001) and T stage (0.557, 95% CI: 0.545–0.569, P<0.001) (Figure 3B). These outcomes suggest that compared to routine staging systems, the models we created show better accuracy in predicting bone metastasis in patients with PCa.
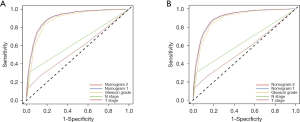
Clinical value of nomograms
DCA, a novel tool used to assess the clinical application value of predictive models, detailed different points of NB at each relevant probability. For the training set, the use of nomogram 2 to predict bone metastasis yielded greater NBs than the intervention-all or the intervention-none when the threshold probabilities ranged from 0.01 to 0.84 (Figure 4A). The threshold probability of 0.01 to 0.76 for bone metastasis was the most beneficial for prediction with nomogram 2 (Figure 4B) for the validation set. In comparison with nomogram 1, Gleason grade, N stage, and T stage, the higher NBs of the nomogram 2 indicated that nomogram 2 could predict bone metastasis in patients with PCa more accurately in both cohorts (Figure 4). To further evaluate the substantial clinical usefulness of nomogram 2, CIC analysis was adopted in both the training set and validation set. The results showed the number of predicted high-risk cases was larger than that of true positive cases when the risk threshold was in the range of 0–0.48 for the training set (Figure 4C) and 0–0.50 for the validation set (Figure 4D). Furthermore, it was also satisfactory for the cost-benefit ratios at each risk threshold mentioned above (Figure 4). To further determine the prognostic model’s daily application value, we divided all patients with PCa into two cohorts based on the median bone metastasis prediction points. Interestingly, patients with points higher than 62 had worse overall survival than those with points lower than 62 (P<0.001, Figure 5). Bone metastasis prediction points were investigated as a new factor and included in the multivariable Cox regression model and were finally demonstrated to be an independent variable for predicting patients’ overall survival with PCa (P<0.001, Table 5).
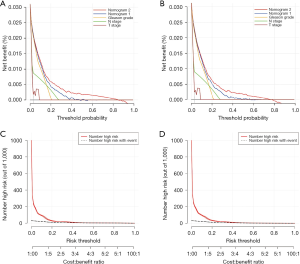
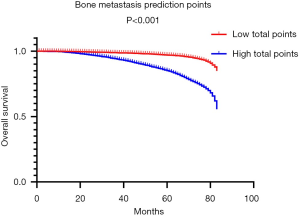
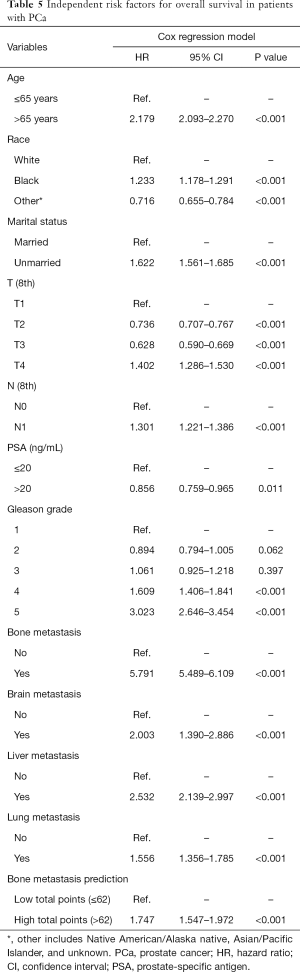
Full table
Discussion
Nowadays, nomograms have become significant modern medical decision tools, that can predict the risk of disease or survival outcomes (17,30). These models integrate a variety of individual risk factors and quantify each variable’s influence to visualize the results of different patients (31,32). Several nomograms for predicting the risk of bone metastasis in patients with PCa have been constructed in recent studies. Godtman et al. developed a risk model for helping some patients to avoid unnecessary bone scans based on thousands of cases from the National Prostate Cancer Register of Sweden (17). Zhang et al. used the clinicopathological information of 116 patients to establish a risk model consisting of multiparametric MRI-based markers and clinical variables (18). However, there was no nomogram in existence that was simultaneously based on abundant clinical data and had excellent predictive ability in previous studies. This study collected data from 168,414 patients diagnosed with PCa from the SEER database, and 5,354 cases suffered from bone metastasis. After univariate and multivariate logistic regression analyses, two novel nomograms for predicting bone metastasis’s risk were successfully constructed. Both internal and external validation of the two nomograms demonstrated the nomograms’ excellent predictive performance through the evaluation of c-indexes and calibration plots. Interestingly, nomogram 2, consisting of nomogram 1 and other distant organ metastasis variables, had a slightly better predictive ability than nomogram 1. Compared with other routine predictive systems, the two nomograms both showed better accuracy in predicting bone metastasis according to ROC analyses’ outcomes. Nevertheless, great discrimination performance and strong calibration power do not necessarily mean the established model could be useful in daily clinical application (31). Thus, DCA was performed to evaluate the nomograms’ clinical usefulness, and nomogram 2 showed better clinical utility in the proper range than other predictive systems. Additionally, CICs in both the training and validation sets based on DCA further demonstrated the good discriminatory ability of nomogram 2. Moreover, bone metastasis prediction points that measure bone metastasis’s probability was defined as a new prognostic factor in patients with PCa. Once patients are predicted to have high bone metastasis risk according to the nomogram, even if patients are not found to have bone metastasis at initial diagnosis, the doctors are responsible for suggesting close imaging examinations of the bone in the future.
This study provided novel insights into the correlation between clinicopathological characteristics and bone metastasis of patients with PCa. After logistic regression analysis, we found that older men with PCa were more likely to have bone metastasis than younger men, similar to several previous studies (33-35). Marital status has also been shown to be an independent factor for distant organ metastasis or overall survival in multiple malignant tumors (36-38). Jiang et al. demonstrated that married patients with metastatic PCa had better overall survival or cancer-specific survival than unmarried patients, which might be because married men tend to get more spiritual comfort and social support (39). The result that unmarried patients with PCa had a higher risk of progressive disease was consistent with a previous study (15). This may due to androgen changes after men get married, as it is well known that androgen plays an essential role in the development of PCa (40). Patients diagnosed at the T4 stage had the highest risk of bone metastasis among all cases. T1 patients had a greater risk of being diagnosed with bone metastasis than T2 or T3 patients. Although this is not an intuitive result, it is consistent with the findings from Guo et al. (15), though the specific reason behind this observation requires further exploration. Previous studies have illustrated that lymph node status, especially pelvic lymph nodes, is vital and therapeutically relevant predictor for patients with PCa (41,42). According to our research, patients experiencing regional lymph nodes usually have tumors with high histological grade (43), and are more likely to suffer from bone metastasis than N0 patients. PSA is a widely used tumor indicator for predicting treatment outcomes or even patient survival with PCa (39,44,45). Like previous studies’ classification methods (46-48), PSA level >20 ng/mL was defined as a high-risk group that increased the probability of bone metastasis. Gleason grade’s predictive ability, the most common classification standard for histological grading of patients with PCa (49), was further confirmed in this study.
Besides bone metastases, we also investigated liver metastasis, brain metastasis, and lung metastasis, even if patients diagnosed with these distant metastatic sites accounted for only a small portion of the entire cohort. In this study, approximately 80% of lung metastasis cases suffered from bone metastasis, and approximately 70% of cases with liver or brain metastases had simultaneous bone metastasis. This indicated that PCa cells had already metastasized to multiple organs when distant metastases were detected, consistent with the findings from several previous studies (33,50). This also indicated that patients with liver, brain, or lung metastases were more likely to have bone metastasis. Thus, these metastatic indicators were included in the predictive system to quantify their influence on bone metastasis. Eventually, nomogram 2 exhibited slightly stronger predictive power and better clinical performance, and therefore it might be a more informed choice to select nomogram 2 as the new model to predict the risk of bone metastasis in patients with PCa.
Our nomograms were established based on enough clinicopathological characteristics convenient to obtain in clinical practice, which made it possible to predict the risk of bone metastasis by collecting the prevalent information even if the patients with PCa had not been found with distant metastasis at initial diagnosis. Compared with routine diagnostic imaging examinations, the novel predictive models reduce radiation exposure and mitigate the economic burden for patients. Moreover, through comparing our model with others in previous studies, some advantages were found. Compared with the nomogram constructed by Godtman et al. (17), our model showed better predictive ability with a higher AUC value (0.800 vs. 0.911). The accuracy of Zhang et al.’s model was also excellent (18), but our model was based on larger sample size and more clinicopathological information.
However, several limitations of this study deserve consideration. First, this was a retrospective study with known inherent drawbacks. Although we tried our best to reduce selection bias, more well-designed prospective analyses are required in the future. Second, some genetic markers that might play an important role in predicting distant metastases were unable to be retrieved from the SEER database, and the construction of a complete predictive model containing both clinicopathological information and genetic markers will be the direction of our future efforts. Third, both the training and validation cohorts in this study were from the SEER dataset, and the overfit bias of the nomograms could have existed. Hence, validating the models using an independent external set with adequate sample size and abundant clinicopathological data will also be the subject of our future investigations.
Conclusions
In conclusion, we established the first practical model for predicting the risk of bone metastasis in patients with PCa based on clinicopathological characteristics extracted from a large population-based dataset. With accurate and clinically valuable predictions, patients can experience fewer unnecessary examinations and less medical costs, and physicians may improve individual treatment approaches and find more appropriate management strategies.
Acknowledgments
Funding: None.
Footnote
Reporting Checklist: The authors have completed the TRIPOD reporting checklist. Available at http://dx.doi.org/10.21037/tau-20-1133
Conflicts of Interest: Both authors have completed the ICMJE uniform disclosure form (available at http://dx.doi.org/10.21037/tau-20-1133). The authors have no conflicts of interest to declare.
Ethical Statement: The authors are accountable for all aspects of the work in ensuring that questions related to the accuracy or integrity of any part of the work are appropriately investigated and resolved. The study was conducted in accordance with the Declaration of Helsinki (as revised in 2013). As data from the SEER program is available for the public and does not need patient informed consent, and ethical approval was waived by the local Ethics Committee of the First Affiliated Hospital of Soochow University.
Open Access Statement: This is an Open Access article distributed in accordance with the Creative Commons Attribution-NonCommercial-NoDerivs 4.0 International License (CC BY-NC-ND 4.0), which permits the non-commercial replication and distribution of the article with the strict proviso that no changes or edits are made and the original work is properly cited (including links to both the formal publication through the relevant DOI and the license). See: https://creativecommons.org/licenses/by-nc-nd/4.0/.
References
- Bray F, Ferlay J, Soerjomataram I, et al. Global cancer statistics 2018: GLOBOCAN estimates of incidence and mortality worldwide for 36 cancers in 185 countries. CA Cancer J Clin 2018;68:394-424. [Crossref] [PubMed]
- Siegel RL, Miller KD, Jemal A. Cancer statistics, 2019. CA Cancer J Clin 2019;69:7-34. [Crossref] [PubMed]
- Berg KD, Thomsen FB, Mikkelsen MK, et al. Improved survival for patients with de novo metastatic prostate cancer in the last 20 years. Eur J Cancer 2017;72:20-7. [Crossref] [PubMed]
- Sartor O, de Bono JS. Metastatic Prostate Cancer. N Engl J Med 2018;378:645-57. [Crossref] [PubMed]
- Gillessen S, Attard G, Beer TM, et al. Management of Patients with Advanced Prostate Cancer: The Report of the Advanced Prostate Cancer Consensus Conference APCCC 2017. Eur Urol 2018;73:178-211. [Crossref] [PubMed]
- Steele CB, Li J, Huang B, et al. Prostate cancer survival in the United States by race and stage (2001-2009): Findings from the CONCORD-2 study. Cancer 2017;123 Suppl 24:5160-77. [Crossref] [PubMed]
- Shou J, Zhang Q, Wang S, et al. The prognosis of different distant metastases pattern in prostate cancer: A population based retrospective study. Prostate 2018;78:491-7. [Crossref] [PubMed]
- Kan C, Vargas G, Pape FL, et al. Cancer Cell Colonisation in the Bone Microenvironment. Int J Mol Sci 2016;17:1674. [Crossref] [PubMed]
- D'Oronzo S, Coleman R, Brown J, et al. Metastatic bone disease: Pathogenesis and therapeutic options: Up-date on bone metastasis management. J Bone Oncol 2019;15:004-4.
- Mohler JL, Antonarakis ES, Armstrong AJ, et al. Prostate Cancer, Version 2.2019, NCCN Clinical Practice Guidelines in Oncology. J Natl Compr Canc Netw 2019;17:479-505. [Crossref] [PubMed]
- Calais J, Cao M, Nickols NG. The Utility of PET/CT in the Planning of External Radiation Therapy for Prostate Cancer. J Nucl Med 2018;59:557-67. [Crossref] [PubMed]
- Liu X, Chen L, Fan Y, et al. IFITM3 promotes bone metastasis of prostate cancer cells by mediating activation of the TGF-beta signaling pathway. Cell Death Dis 2019;10:517. [Crossref] [PubMed]
- Macedo F, Ladeira K, Pinho F, et al. Bone Metastases: An Overview. Oncol Rev 2017;11:321. [PubMed]
- Owari T, Miyake M, Nakai Y, et al. Clinical Features and Risk Factors of Skeletal-Related Events in Genitourinary Cancer Patients with Bone Metastasis: A Retrospective Analysis of Prostate Cancer, Renal Cell Carcinoma, and Urothelial Carcinoma. Oncology 2018;95:170-8. [Crossref] [PubMed]
- Guo X, Zhang C, Guo Q, et al. The homogeneous and heterogeneous risk factors for the morbidity and prognosis of bone metastasis in patients with prostate cancer. Cancer Manag Res 2018;10:1639-46. [Crossref] [PubMed]
- Liu RZ, Zhao ZR, Ng CS. Statistical modelling for thoracic surgery using a nomogram based on logistic regression. J Thorac Dis 2016;8:E731-6. [Crossref] [PubMed]
- Godtman RA, Mansson M, Bratt O, et al. Development and validation of a prediction model for identifying men with intermediate- or high-risk prostate cancer for whom bone imaging is unnecessary: a nation-wide population-based study. Scand J Urol 2019;53:378-84. [Crossref] [PubMed]
- Zhang W, Mao N, Wang Y, et al. A Radiomics nomogram for predicting bone metastasis in newly diagnosed prostate cancer patients. Eur J Radiol 2020;128:109020. [Crossref] [PubMed]
- Cronin KA, Ries LA, Edwards BK. The Surveillance, Epidemiology, and End Results (SEER) Program of the National Cancer Institute. Cancer 2014;120 Suppl 23:3755-7. [Crossref] [PubMed]
- Huang YX, Lin YZ, Li JL, et al. Role of postoperative radiotherapy in pT3N0 rectal cancer: A risk-stratification system based on population analyses. Cancer Med 2019;8:1024-33. [Crossref] [PubMed]
- Paner GP, Stadler WM, Hansel DE, et al. Updates in the Eighth Edition of the Tumor-Node-Metastasis Staging Classification for Urologic Cancers. Eur Urol 2018;73:560-9.
- Epstein JI, Egevad L, Amin MB, et al. The 2014 International Society of Urological Pathology (ISUP) Consensus Conference on Gleason Grading of Prostatic Carcinoma: Definition of Grading Patterns and Proposal for a New Grading System. Am J Surg Pathol 2016;40:244-52. [PubMed]
- Sauerbrei W, Boulesteix AL, Binder H. Stability investigations of multivariable regression models derived from low- and high-dimensional data. J Biopharm Stat 2011;21:1206-31. [Crossref] [PubMed]
- Pencina MJ, D'Agostino RB. Overall C as a measure of discrimination in survival analysis: model specific population value and confidence interval estimation. Stat Med 2004;23:2109-23. [Crossref] [PubMed]
- Hanley JA, McNeil BJ. The meaning and use of the area under a receiver operating characteristic (ROC) curve. Radiology 1982;143:29-36. [Crossref] [PubMed]
- Vickers AJ, Cronin AM, Elkin EB, et al. Extensions to decision curve analysis, a novel method for evaluating diagnostic tests, prediction models and molecular markers. BMC Med Inform Decis Mak 2008;8:53. [Crossref] [PubMed]
- Vickers AJ, Elkin EB. Decision curve analysis: a novel method for evaluating prediction models. Med Decis Making 2006;26:565-74. [Crossref] [PubMed]
- Huang YQ, Liang CH, He L, et al. Development and Validation of a Radiomics Nomogram for Preoperative Prediction of Lymph Node Metastasis in Colorectal Cancer. J Clin Oncol 2016;34:2157-64. [Crossref] [PubMed]
- Kerr KF, Brown MD, Zhu K, et al. Assessing the Clinical Impact of Risk Prediction Models With Decision Curves: Guidance for Correct Interpretation and Appropriate Use. J Clin Oncol 2016;34:2534-40. [Crossref] [PubMed]
- He C, Mao Y, Wang J, et al. Nomograms predict long-term survival for patients with periampullary adenocarcinoma after pancreatoduodenectomy. BMC Cancer 2018;18:327. [Crossref] [PubMed]
- Balachandran VP, Gonen M, Smith JJ, et al. Nomograms in oncology: more than meets the eye. Lancet Oncol 2015;16:e173-80. [Crossref] [PubMed]
- Zhang YF, Ji H, Lu HW, et al. Postoperative survival analysis and prognostic nomogram model for patients with portal hypertension. World J Gastroenterol 2018;24:4499-509. [Crossref] [PubMed]
- Zhao F, Wang J, Chen M, et al. Sites of synchronous distant metastases and prognosis in prostate cancer patients with bone metastases at initial diagnosis: a population-based study of 16,643 patients. Clin Transl Med 2019;8:30. [Crossref] [PubMed]
- Zhuo L, Cheng Y, Pan Y, et al. Prostate cancer with bone metastasis in Beijing: an observational study of prevalence, hospital visits and treatment costs using data from an administrative claims database. BMJ Open 2019;9:e028214. [Crossref] [PubMed]
- Yamamoto T, Musunuru HB, Vesprini D, et al. Metastatic Prostate Cancer in Men Initially Treated with Active Surveillance. J Urol 2016;195:1409-14. [Crossref] [PubMed]
- Yan Y, Liu H, Mao K, et al. Novel nomograms to predict lymph node metastasis and liver metastasis in patients with early colon carcinoma. J Transl Med 2019;17:193. [Crossref] [PubMed]
- Bai DS, Chen P, Qian JJ, et al. Effect of marital status on the survival of patients with gallbladder cancer treated with surgical resection: a population-based study. Oncotarget 2017;8:26404-13. [Crossref] [PubMed]
- Zhou H, Zhang Y, Song Y, et al. Marital status is an independent prognostic factor for pancreatic neuroendocrine tumors patients: An analysis of the Surveillance, Epidemiology, and End Results (SEER) database. Clin Res Hepatol Gastroenterol 2017;41:476-86. [Crossref] [PubMed]
- Jiang WD, Yuan PC. Development and validation of prognostic nomograms for patients with metastatic prostate cancer. Int Urol Nephrol 2019;51:1743-53. [Crossref] [PubMed]
- Vaarala MH, Hirvikoski P, Kauppila S, et al. Identification of androgen-regulated genes in human prostate. Mol Med Rep 2012;6:466-72. [Crossref] [PubMed]
- Winter A, Engels S, Reinhardt L, et al. Magnetic Marking and Intraoperative Detection of Primary Draining Lymph Nodes in High-Risk Prostate Cancer Using Superparamagnetic Iron Oxide Nanoparticles: Additional Diagnostic Value. Molecules 2017;22:2192. [Crossref] [PubMed]
- Hoshi S, Hayashi N, Kurota Y, et al. Comparison of semi-extended and standard lymph node dissection in radical prostatectomy: A single-institute experience. Mol Clin Oncol 2015;3:1085-7. [Crossref] [PubMed]
- Wilczak W, Wittmer C, Clauditz T, et al. Marked Prognostic Impact of Minimal Lymphatic Tumor Spread in Prostate Cancer. Eur Urol 2018;74:376-86. [Crossref] [PubMed]
- Tikkinen KAO, Dahm P, Lytvyn L, et al. Prostate cancer screening with prostate-specific antigen (PSA) test: a clinical practice guideline. BMJ 2018;362:k3581. [Crossref] [PubMed]
- Kubo K, Wadasaki K, Kimura T, et al. Clinical features of prostate-specific antigen bounce after 125I brachytherapy for prostate cancer. J Radiat Res 2018;59:649-55. [Crossref] [PubMed]
- Zaman MU, Fatima N, Sajjad Z. Metastasis on bone scan with low prostate specific antigen (</=20 ng/ml) and Gleason's score (<8) in newly diagnosed Pakistani males with prostate cancer: should we follow Western guidelines? Asian Pac J Cancer Prev 2011;12:1529-32. [PubMed]
- Wu YH, Yang WC, Hu YW, et al. Definitive Radiotherapy for Older Patients with Prostate Cancer: Experience of a Medical Center in Taiwan. Sci Rep 2017;7:13880. [Crossref] [PubMed]
- Sekiguchi A, Ishiyama H, Satoh T, et al. 125Iodine monotherapy for Japanese men with low- and intermediate-risk prostate cancer: outcomes after 5 years of follow-up. J Radiat Res 2014;55:328-33. [Crossref] [PubMed]
- Dhom G. Classification and grading of prostatic carcinoma. Recent Results Cancer Res 1977.14-26. [Crossref] [PubMed]
- Deng Y, Bi R, Zhu Z, et al. A Surveillance, Epidemiology and End Results database analysis of the prognostic value of organ-specific metastases in patients with advanced prostatic adenocarcinoma. Oncol Lett 2019;18:1057-70. [Crossref] [PubMed]