Comprehensive analysis of glutathione peroxidase-1 (GPX1) expression and prognostic value in three different types of renal cell carcinoma
Introduction
Renal cell carcinoma (RCC) is a malignant tumor originating from the epithelial system of the renal parenchyma. Globally, the incidence of RCC varies greatly between countries and regions, with the highest rates in North America (11.7 per 100,000) and Western Europe (9.8 per 100,000) (1). RCC has a variety of pathological types, which differ in histology and genetics. Three of the most common types of RCC are kidney chromophobe cell carcinoma (KICH), kidney renal papillary cell carcinoma (KIRP) and kidney renal clear cell carcinoma (KIRC) (2,3). The diagnosis and management of RCC have improved significantly in the past decade and many small kidney tumors have been accidentally detected during screening. However, up to 17% of patients already have distant metastases at diagnosis (4). At present, surgery is still the main treatment for renal clear cell carcinoma (5). For advanced kidney cancer that cannot be surgically removed, molecularly targeted therapy can extend the patient’s life, but is only effective in 20–40% of cases (6). Therefore, there is an urgent need to explore the pathogenesis of RCC in order to identify potential biomarkers for the diagnosis, treatment and prognosis of the disease.
Glutathione peroxidase-1 (GPX1) is located on chromosome 3p21, and the expression product mainly exists in tissues with high oxygen tension such as the lungs, liver, and kidneys (7). It is one of the most important antioxidant enzymes in the human body and plays an important role in the degradation of reactive oxygen species (ROS), which can protect normal cells from oxidative stress (8,9). Studies have also found that tumor cells can produce excess ROS through multiple pathways, which could be associated with the origin, development and metastasis of tumors (10,11). Compared with normal tissues, GPX1 is upregulated in some specific cancer tissues. There are also significant differences in the expression of GPX1 between different tumors. For instance, the GPX1 expression is remarkably higher in cervical cancer, gastric cancer, and renal cancer than in lung cancer or lymphoma (12). Patients with hepatocellular carcinoma with a high expression of GPX1 have a higher risk of vascular invasion and a lower survival rate (13). Similarly, previous studies have found that the expression of GPX1promotes tumor proliferation and invasion and is increased in head and neck tumors (14).
In summary, the expression level of GPX1 has great potential in tumor diagnosis and prognosis evaluation. This study analyzed the practical information obtained by the correlation between GPX1 expression and the prognosis of KICH, KIRP and KIRC through different databases. We present the following article in accordance with the REMARK reporting checklist (available at http://dx.doi.org/10.21037/tau-20-1398).
Methods
Gene expression data
Data concerning mRNA expressions of GPX1 between tumors and adjacent normal tissues were obtained from the Oncomine database (https://www.oncomine.org) and the gene expression profiling interactive analysis database (GEPIA: http://gepia.cancer-pku.cn/). Oncomine is a cancer microarray database and web-based data mining platform containing 715 gene expression datasets from 86,733 clinical cancer specimens and normal control samples (15). This study used a P value of 0.01 with a fold change of 1.5 to screen and display the comparative results of GPX1 expression in cancer specimens and normal control samples.
GEPIA is an interactive web server that can be customized to analyze the RNA sequencing data of 9,736 tumors and 8,587 normal samples from The Cancer Genome Atlas (TCGA) and the Genotype-Tissue Expression (GTEx) projects (16). GEPIA was used to find the expression levels of GPX1 in 33 tumor types of TCGA with a final focus on three RCC subtypes for survival analysis.
Survival analysis
The University of Alabama Cancer database (UALCAN) (http://ualcan.path.uab.edu/) is an interactive web portal containing RNA sequences and clinical data from 33 types of cancers that analyzes the relative expression of genes in tumor and normal samples from the TCGA database and assesses the impact of gene expression levels and clinicopathological features on patient survival (17). UALCAN was used to analyze the survival and prognosis of RCC patients. Differences in GPX1 expression by gender, age, race, tumor stage, tumor grade, nodal metastasis, and TP53 mutation status were also analyzed. It should be noted that the subgroup analysis of each type of RCC was not identical due to sequencing-related issues or incomplete information on the available subgroups of tumors in the database.
DriverDBv3 (driverdb.tms.cmu.edu.tw/) is a database of cancer driver genes, which uses published bioinformatics algorithms to identify driver genes and mutations and interprets the complex information of cancer omics through concise data visualization (18). We used DriverDBv3 to corroborate the prognostic potential of GPX1 gene expression levels in different human cancers.
Function and pathway enrichment analysis of different types of RCC
The LinkedOmics (http://www.linkedomics.org/login.php) web tool was used to analyze the multi-omics data of the three RCCs in TCGA database. TCGA is a publicly available portal which provides multi-omics data for all 33 types of cancer as well as proteomics data based on mass spectrometry by the clinical proteomics tumor analysis association (CPTAC) (19). The biological processes (BP), cellular components (CC), molecular functions (MF), and the Kyoto Encyclopedia of Genes and Genome (KEGG) pathway of GPX1 were analyzed.
GeneMANIA is an online analytical tool for building protein-protein interaction (PPI) networks and predicting gene function based on existing genomic and proteomic data (20). Its uniqueness in a gene recommendation system is that it can infer the most informative interaction networks based on a list of queries (21). Using this tool we created a visual PPI network of GPX1.
RCC tissue specimen and control specimen
The renal specimens of three patients admitted to the First Affiliated Hospital of Guangxi Medical University in 2020 were collected. Two patients underwent nephrectomy for RCC, while the third patient had renal atrophy due to chronic stone obstruction and underwent nonfunctional nephrectomy. This third patient served as the control. For each patient’s specimen, a portion of the tissue was fixed with formalin and embedded in paraffin for immunohistochemical analysis to clarify the pathological type of the tumor, and the other remaining portion was fixed with RNA preservative and subjected to quantitative polymerase chain reaction (qPCR) testing. None of the patients had undergone anti-tumor therapy such as chemotherapy, radiation therapy, or molecular targeted therapy prior to surgery. We obtained signed informed consent from all patients. This research was approved by the Ethics Committee of the First Affiliated Hospital of Guangxi Medical University. The study was conducted in accordance with the Declaration of Helsinki (as revised in 2013).All procedures performed in this study involving human participants were in accordance with the Declaration of Helsinki (as revised in 2013).
Quantitative real-time polymerase chain reaction (qRT-PCR) analysis
The tissues of the two RCC tumors and the atrophic kidney were saved using RNAlater (Thermo Fisher Scientific, Waltham, MA, USA) after surgical resection. Total RNA was extracted using a Trizol reagent (Thermo Fisher Scientific, Waltham, MA, USA), and 2 µg of RNA was converted into cDNA using the RevertAid First Strand cDNA Synthesis Kit (Thermo Fisher Scientific, Waltham, MA, USA). Quantitative PCR was accomplished using the FastStart Universal SYBR Green Master (Rox) (Roche, Basal, Switzerland) in the ABI PRISM® 7300 real-time PCR system (Applied Biosystems, Foster City, CA, USA), and glyceraldehyde 3-phosphate dehydrogenase (GADPH) was used as an endogenous control. Finally, melting curves were used to monitor nonspecific amplifications, and the relative expression level was computed using the 2-ΔΔCt method. The primer sequences of GPX1 were as follows: 5'-TATCGAGAATGTGGCGTCCC-3' (forward) and 5'-TCTTGGCGTTCTCCTGAATGC-3' (reverse).
Immune infiltration level analysis of GPX1
In order to evaluate the correlation between the subpopulations of infiltrating immune cells and the GPX1 expression in the RCC samples, analysis was conducted with the tumor immune estimation resource (TIMER: https://cistrome.shinyapps.io/timer/), which is a web-based tool that uses RNA-Seq expression profiling data to detect the infiltration of immune cells in tumor tissues (22). The database provides the infiltration of 6 types of immune cells (B cells, CD4+ T cells, CD8+ T cells, neutrophils, macrophages, and dendritic cells) and evaluates the correlation between GPX1 expression and the immune cell abundance score with the Spearman correlation coefficient.
Statistical analysis
Statistical analysis was performed using SPSS 26.0 (IBM Corporation, Armonk, NY, USA) and GraphPad Prism 8 (GraphPad Software, La Jolla, CA, USA). Student's t test was used to analyze the expression levels of GPX1 mRNA in the three patient samples of kidney cancer tissue and atrophic kidney tissue. The Kaplan-Meier curve was used to analyze the relationship between GPX1 expression level and overall survival (OS) of RCC. A P value <0.05 was considered to be statistically significant.
Results
The expression level of GPX1 in different types of RCC
According to the Oncomine database analysis, the expression of GPX1 in RCC and adjacent normal tissues was different. The database included a total of 456 unique analyses including 38 high expressions and five low expressions on GPX1. The results indicated that the expression of GPX1 in kidney cancer was higher than that in normal tissues (Figure 1A).
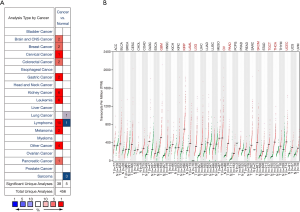
Further analysis of the expression of GPX1 in three types of RCC derived from TCGA and GTEx databases was conducted with the GEPIA program. The results suggested that the expression of GPX1 in KICH, KIRP, and KIRC were higher than that in adjacent normal tissue samples (Figure 1B).
The clinical prognostic value of GPX1 in different types of RCC
In order to explore the correlation between the expression level of GPX1 and prognosis in RCC, the GEPIA, UALCAN, and DriverDBv3 databases were used to perform the survival analysis based on the RNA sequencing data in TCGA. The results in the GEPIA database demonstrated that a high GPX1 expression was associated with a better overall survival (OS) prognosis in KIRP (P=0.023, HR =0.48) and a poorer OS in KICH (P=0.022, HR =7.9) (Figure 2). The DriverDBv3 database was used to further identify the potential value of GPX1 in assessing the prognosis of RCC (Figure 3). In KIRP, a high GPX1 expression was associated with a better OS (P=0.0229, HR =0.461), which was consistent with the results obtained in the GEPIA database. Conversely, the high expression of GPX1 in KIRC was associated with a poorer prognosis (P=0.0158, HR =1.7).
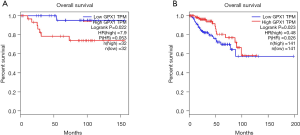
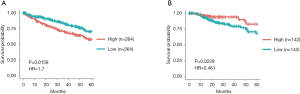
Lastly, the UALCAN database was also used for survival analysis (Figure 4). The results indicated that a high expression of GPX1 was associated with a poorer prognosis in KICH (P=0.012), which was consistent with the results in GEPIA. In KIRC patients, a high expression of GPX1 was associated with a poorer prognosis (P=0.036), which was also in agreement with the results obtained in the driverDBV3 database.
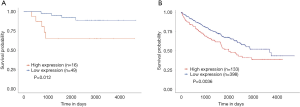
Taken together, these results suggest that GPX1 has potential value in predicting the prognosis of RCC.
Expression of GPX1 in different subgroups of RCC
The subgroup analysis of RCC using three different pathological types to explore the relationship between GPX1 expression and different clinical characteristics was performed with the UALCAN database. The expression of GPX1 was grouped according to gender, age, race, clinical stage, and lymph node metastasis. In addition, TP53 mutation groups and tumor grade groups were established for KICH and KIRC, respectively (Figure 5). Results indicated that the expression level of GPX1 varied according to the patients’ clinical characteristics, which also demonstrates the potential of GPX1 to act as a biomarker for RCC patients.
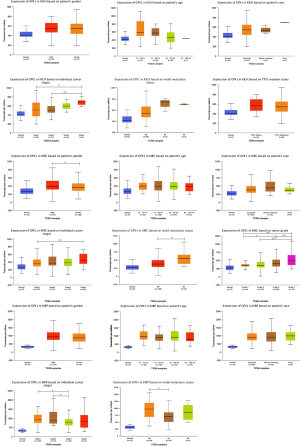
Analysis of the PPI network of GPX1
The results showed that the related genes interacting with the protein encoded by GPX1 were mainly involved in peroxidase activity, glutathione peroxidase activity, oxidoreductase activity, and antioxidant activity (Figure 6).
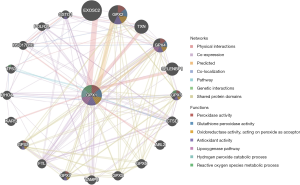
Functional enrichment of GPX1 in different types of RCC
The enrichment of functions in three different types of RCC were conducted by LinkedOmics, and showed similar results (Figure 7). In KICH, GPX1 was mainly enriched in the biological process of protein localization in the endoplasmic reticulum, and participated in the composition of the respiratory chain, the activity of oxidoreductase, and the glycosphingolipid metabolic pathway. In KIRC, GPX1 was mainly enriched in the translational elongation phase, the mitochondrial protein complex, the structural constituent of ribosome, and the hippo signaling pathway. In KIRP, the functional enrichment of GPX1 was similar to that in KIRC, and was mainly enriched in the mitochondrial respiratory chain complex assembly, the composition of the respiratory chain, and the ribosomal pathway.
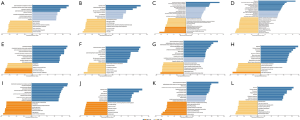
Analysis of the relationship between RCC and the abundance of immune infiltration based on the Timer database
TIMER was used to analyze the relationship between the expression of GPX1 and the abundance of various RCC immune infiltrations (Figure 8). The results showed that in KICH, the expression level of GPX1 was negatively correlated with the infiltration abundance of CD8+ T cells (r=−0.382, P=1.70e-03) and neutrophil (r=−0.27, P=2.97e-02). In KIRC, there was a positive correlation between the expression level of GPX1 and the infiltration abundance of B cells (r=0.099, P=3.45e-02), macrophages (r=0.175, P=1.94e-04), and dendritic cells (r=0.187, P=6.14e-05). In KIRP, there was a positive correlation between the expression level of GPX1 and the abundance of CD8+ T cells (r=0.163, P=8.66e−03), CD4+ T cells (r=0.368, P=1.13e−09), macrophages (r=0.325, P=1.50e−07), neutrophils (r=0.13, P=3.76e−02), and dendritic cells (r=0.286, P=3.28e−06).
Verification of the expression difference of GPX1 in RCC and normal tissues
A total of two patients with KICH and one patient with renal atrophy were included as the experimental group and the control group, respectively. The patient characteristics are shown in Table 1. The qPCR results showed that the expression of GPX1 in the tumor tissues of the two RCC patients was higher than that of the control group, and the difference was statistically significant (P<0.05) (Figure 9).

Full table
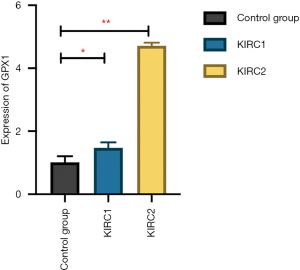
Discussion
RCC is the most common upper urinary tract malignant tumor in the urinary system, accounting for 2% of adult malignant tumors. In China, the incidence of RCC ranks second among urinary system tumors. Likewise, 73,820 RCCs were diagnosed in the United States in 2019, and approximately 15,000 deaths from RCC were recorded (23). With the continuing improvement in diagnosis and treatment, the 5-year survival rate of RCC has reached 74%, and only 12% of patients have advanced metastases (24). Early monitoring is the key to improving patient survival and prognosis. However, most types of RCC are not responsive to radiotherapy or chemotherapy. Apart from surgery, it is difficult to achieve good therapeutic outcomes with existing treatment modalities. Therefore, the search for sensitive biomarkers and specific therapeutic targets to provide personalized treatment options has important clinical significance for improving the RCC survival rate.
The protein encoded by GPX1 belongs to the glutathione peroxidase family and is generally distributed in the mitochondria and cytoplasm of cells (25). A number of researchers have shown that GPX1 has opposite effects in different tumors, and previous studies have reported that overexpression of GPX1 can inhibit the growth of pancreatic cancer cell lines (26). It has also been confirmed that GPX1 can reduce oxidative DNA mutations, and prevent the malignant change in tissues in the initial stage of the disease (27). Inversely, some studies have suggested that a high expression of GPX1 can lead to proliferation and invasion of cancer cells and induce cisplatin resistance in esophageal squamous cell carcinoma (28).
This study also found a similar conclusion through data mining. When GPX1 was highly expressed in KIRC and KICH, the OS of patients was worse than that in low expression cases, whereas in KIRP, the high expression of GPX1 heralded a better OS, and this conclusion was mutually confirmed across different databases. We also identified a relationship between the expression of GPX1 and the clinical characteristics of RCC patients. Unexpectedly, we found that the expression of GPX1 in stage 4 KICH was significantly higher than that in stages 1 and 2 (P<0.05). Similar results were also shown in KIRC. The expression of GPX1 in KIRC patients with stage N1 was also higher than that of patients with stage N0 (P<0.05), and the expression in patients with tumor grade 4 was higher than that of other grades. These results undoubtedly confirm that GPX1 can be used as a prognostic biomarker as well as a diagnostic biomarker for RCC. Interestingly, KIRP showed the opposite result. The expression of GPX1 in KIRP patients with stage N1 was lower than that of patients with stage N0 (P<0.05), and the expression of patients with tumor grade 3 was lower than that of patients with grades 1 and 2 (P<0.05). This was in accordance with the survival analysis finding that KIRP with a high GPX1 expression had a better prognosis. We speculated that GPX1 may play a role in inhibiting tumor progression in KIRP.
In recent years, the relationship between immune response and tumor prognosis has received increasing research attention (29,30). In this study, TIMER was used to analyze the correlation between GPX1 and 6 immune-infiltrating cells in RCC. We observed that the expression of GPX1 and CD8+T cells showed a moderately negative correlation in KICH, but a positive correlation in KIRP, which was consistent with the results found in other studies (31). High levels of activated CD8+T cells were associated with a better prognosis in various tumors (32,33). The relationship between the expression level of GPX1 and the infiltration of CD8+T cells may explain why the high expression of GPX1 causes the contrast in prognosis for KICH and KIRP patients. It is possible that cytotoxic CD8+T cells can target and kill tumor cells and may play a role in the inhibition of tumor growth. In addition, the increase in CD4+ T cells was associated with better outcomes in certain cancers (34). Infiltrating CD4 + T cells can participate in the TGFβ1/YBX1/HIF2α signaling pathway to inhibit RCC cell proliferation (35). In this study, GPX1 showed a moderate positive correlation with CD4+ T cells in KIRP, and we tentatively proposed that GPX1 may be correlated with the TGFβ1/YBX1/HIF2α signaling pathway. Nevertheless, further studies are required to confirm this conclusion.
GPX4 is a member of the GPX family. The cystine/glutamate antiporter system consisting of SLC3A2 and SLC7A11 translocates cystine into the cell and then synthesizes GPX4 by the glutamate cysteine ligase action. Previous studies have found that SLC7A11 is highly expressed in a variety of tumors, and increases cystine transport and intracellular synthesis of GPX4, which thus avoids ferroptosis due to excessive cellular ROS accumulation causing the tumor development (36). Our research found an interaction between GPX1 and GPX4 in the PPI network, including physical connection and co-expression. Therefore, GPX1 may also be involved in this process.
The functional enrichment analysis showed that, in the three RCC subtypes, GPX1 was enriched in the hippo signaling pathway, the inactivation of its upstream regulatory molecules can lead to tumor development. The relationship between RCC and the hippo signaling pathway has also been reported in recent studies and suggests new approaches for the clinical treatment of RCC (37). We therefore speculate that during tumor progression, these upstream regulatory molecules affecting the Hippo signaling pathway may also be involved in influencing GPX1 expression, thus promoting tumor development. This conjecture still needs to be confirmed by further experiments.
Conclusions
In this study, we performed a multidimensional bioinformatics analysis of the expression and prognostic value of GPX1 in three types of RCC. In addition, clinical samples were collected for testing to further validate the results obtained in the data analysis. Our study demonstrated the relationship between GPX1 and RCC prognosis, and further explored the role of GPX1 in RCC through its functional enrichment, protein interaction network, and immune infiltration. More importantly, these findings provide a theoretical basis for GPX1 to be considered as a potential diagnostic and prognostic biomarker for RCC.
Acknowledgments
Funding: None.
Footnote
Reporting Checklist: The authors have completed the REMARK reporting checklist. Available at http://dx.doi.org/10.21037/tau-20-1398
Data Sharing Statement: Available at http://dx.doi.org/10.21037/tau-20-1398
Conflicts of Interest: All authors have completed the ICMJE uniform disclosure form (available at http://dx.doi.org/10.21037/tau-20-1398). The authors have no conflicts of interest to declare.
Ethical Statement: The authors are accountable for all aspects of the work in ensuring that questions related to the accuracy or integrity of any part of the work are appropriately investigated and resolved. We obtained signed informed consent from all patients. This research was approved by the Ethics Committee of the First Affiliated Hospital of Guangxi Medical University. The study was conducted in accordance with the Declaration of Helsinki (as revised in 2013).
Open Access Statement: This is an Open Access article distributed in accordance with the Creative Commons Attribution-NonCommercial-NoDerivs 4.0 International License (CC BY-NC-ND 4.0), which permits the non-commercial replication and distribution of the article with the strict proviso that no changes or edits are made and the original work is properly cited (including links to both the formal publication through the relevant DOI and the license). See: https://creativecommons.org/licenses/by-nc-nd/4.0/.
References
- Capitanio U, Bensalah K, Bex A, et al. Epidemiology of Renal Cell Carcinoma. Eur Urol 2019;75:74-84. [Crossref] [PubMed]
- Srigley JR, Delahunt B, Eble JN, et al. The International Society of Urological Pathology (ISUP) Vancouver Classification of Renal Neoplasia. Am J Surg Pathol 2013;37:1469-89. [Crossref] [PubMed]
- Shuch B, Amin A, Armstrong AJ, et al. Understanding pathologic variants of renal cell carcinoma: distilling therapeutic opportunities from biologic complexity. Eur Urol 2015;67:85-97. [Crossref] [PubMed]
- Mollica V, Di Nunno V, Massari F. Pembrolizumab plus axitinib: a new treatment option for patients with metastatic renal cell carcinoma. Chin Clin Oncol 2019;8:S21. [Crossref] [PubMed]
- Patel HD, Gorin MA, Gupta N, et al. Mortality trends and the impact of lymphadenectomy on survival for renal cell carcinoma patients with distant metastasis. Can Urol Assoc J 2016;10:389-95. [Crossref] [PubMed]
- Wang L, Cai W, Kong W, et al. Plasma fibrinogen as prognostic predictor in patients with metastatic renal cell carcinoma receiving target therapy. Transl Cancer Res 2018;7:1384-92. [Crossref]
- Arsova-Sarafinovska Z, Matevska N, Eken A, et al. Glutathione peroxidase 1 (GPX1) genetic polymorphism, erythrocyte GPX activity, and prostate cancer risk. Int Urol Nephrol 2009;41:63-70. [Crossref] [PubMed]
- Ahwach SM, Thomas M, Onstead-Haas L, et al. The glutathione mimic ebselen inhibits oxidative stress but not endoplasmic reticulum stress in endothelial cells. Life Sci 2015;134:9-15. [Crossref] [PubMed]
- Brigelius-Flohé R, Maiorino M. Glutathione peroxidases. Biochim Biophys Acta 2013;1830:3289-303. [Crossref] [PubMed]
- Kang SW, Lee S, Lee EK. ROS and energy metabolism in cancer cells: alliance for fast growth. Arch Pharm Res 2015;38:338-45. [Crossref] [PubMed]
- Li WJ, Nie SP, Yao YF, et al. Ganoderma atrum Polysaccharide Ameliorates Hyperglycemia-Induced Endothelial Cell Death via a Mitochondria-ROS Pathway. J Agric Food Chem 2015;63:8182-91. [Crossref] [PubMed]
- Wei R, Qiu H, Xu J, et al. Expression and prognostic potential of GPX1 in human cancers based on data mining. Ann Transl Med 2020;8:124. [Crossref] [PubMed]
- Zhao H, Tang J, Xu J, et al. Selenoprotein Genes Exhibit Differential Expression Patterns Between Hepatoma HepG2 and Normal Hepatocytes LO2 Cell Lines. Biol Trace Elem Res 2015;167:236-41. [Crossref] [PubMed]
- Dequanter D, Dok R, Koolen L, et al. Prognostic Significance of Glutathione Peroxidase Levels (GPx1) in Head and Neck Cancers. Front Oncol 2017;7:84. [Crossref] [PubMed]
- Rhodes DR, Kalyana-Sundaram S, Mahavisno V, et al. Oncomine 3.0: genes, pathways, and networks in a collection of 18,000 cancer gene expression profiles. Neoplasia 2007;9:166-80. [Crossref] [PubMed]
- Tang Z, Li C, Kang B, et al. GEPIA: a web server for cancer and normal gene expression profiling and interactive analyses. Nucleic Acids Res 2017;45:W98-102. [Crossref] [PubMed]
- Chandrashekar DS, Bashel B, Balasubramanya SAH, et al. UALCAN: A Portal for Facilitating Tumor Subgroup Gene Expression and Survival Analyses. Neoplasia 2017;19:649-58. [Crossref] [PubMed]
- Liu SH, Shen PC, Chen CY, et al. DriverDBv3: a multi-omics database for cancer driver gene research. Nucleic Acids Res 2020;48:D863-70. [PubMed]
- Vasaikar SV, Straub P, Wang J, et al. LinkedOmics: analyzing multi-omics data within and across 32 cancer types. Nucleic Acids Res 2018;46:D956-63. [Crossref] [PubMed]
- Warde-Farley D, Donaldson SL, Comes O, et al. The GeneMANIA prediction server: biological network integration for gene prioritization and predicting gene function. Nucleic Acids Res 2010;38:W214-20. [Crossref] [PubMed]
- Mostafavi S, Morris Q. Combining many interaction networks to predict gene function and analyze gene lists. Proteomics 2012;12:1687-96. [Crossref] [PubMed]
- Li T, Fan J, Wang B, et al. TIMER: A Web Server for Comprehensive Analysis of Tumor-Infiltrating Immune Cells. Cancer Res 2017;77:e108-10. [Crossref] [PubMed]
- Siegel RL, Miller KD, Jemal A. Cancer statistics, 2019. CA Cancer J Clin 2019;69:7-34. [Crossref] [PubMed]
- Siegel RL, Miller KD, Jemal A. Cancer Statistics, 2017. CA Cancer J Clin 2017;67:7-30. [Crossref] [PubMed]
- Cheng Y, Xu T, Li S, et al. GPX1, a biomarker for the diagnosis and prognosis of kidney cancer, promotes the progression of kidney cancer. Aging (Albany NY) 2019;11:12165-76. [Crossref] [PubMed]
- Liu J, Hinkhouse MM, Sun W, et al. Redox regulation of pancreatic cancer cell growth: role of glutathione peroxidase in the suppression of the malignant phenotype. Hum Gene Ther 2004;15:239-50. [Crossref] [PubMed]
- Baliga MS, Wang H, Zhuo P, et al. Selenium and GPx-1 overexpression protect mammalian cells against UV-induced DNA damage. Biol Trace Elem Res 2007;115:227-42. [Crossref] [PubMed]
- Gan X, Chen B, Shen Z, et al. High GPX1 expression promotes esophageal squamous cell carcinoma invasion, migration, proliferation and cisplatin-resistance but can be reduced by vitamin D. Int J Clin Exp Med 2014;7:2530-40. [PubMed]
- Pan JH, Zhou H, Cooper L, et al. LAYN Is a Prognostic Biomarker and Correlated With Immune Infiltrates in Gastric and Colon Cancers. Front Immunol 2019;10:6. [Crossref] [PubMed]
- Chen B, Lai J, Dai D, et al. JAK1 as a prognostic marker and its correlation with immune infiltrates in breast cancer. Aging (Albany NY) 2019;11:11124-35. [Crossref] [PubMed]
- Zhang S, Zhang E, Long J, et al. Immune infiltration in renal cell carcinoma. Cancer Sci 2019;110:1564-72. [Crossref] [PubMed]
- Youngblood B, Hale JS, Kissick HT, et al. Effector CD8 T cells dedifferentiate into long-lived memory cells. Nature 2017;552:404-9. [Crossref] [PubMed]
- Yao J, Xi W, Zhu Y, et al. Checkpoint molecule PD-1-assisted CD8(+) T lymphocyte count in tumor microenvironment predicts overall survival of patients with metastatic renal cell carcinoma treated with tyrosine kinase inhibitors. Cancer Manag Res 2018;10:3419-31. [Crossref] [PubMed]
- Stanton SE, Disis ML. Clinical significance of tumor-infiltrating lymphocytes in breast cancer. J Immunother Cancer 2016;4:59. [Crossref] [PubMed]
- Wang Y, Wang Y, Xu L, et al. CD4 + T cells promote renal cell carcinoma proliferation via modulating YBX1. Exp Cell Res 2018;363:95-101. [Crossref] [PubMed]
- Kajarabille N, Latunde-Dada GO. Programmed Cell-Death by Ferroptosis: Antioxidants as Mitigators. Int J Mol Sci 2019;20:4968. [Crossref] [PubMed]
- Chen F, Zhang Y, Şenbabaoğlu Y, et al. Multilevel Genomics-Based Taxonomy of Renal Cell Carcinoma. Cell Rep 2016;14:2476-89. [Crossref] [PubMed]
(English Language Editor: D. Fitzgerald)