Machine learning-assisted decision-support models to better predict patients with calculous pyonephrosis
Introduction
Pyonephrosis is an acute infection involving the containment of pus within an obstructed collecting system, which could be secondary to hydronephrosis caused by the obstruction of the upper urinary tract, or pyelonephritis (1). It is also defined as infective hydronephrosis, is typically associated with renal pelvis abscess formation, and is most commonly a complication of a ureteral obstruction (2,3). Calculous pyonephrosis is often caused by obstructive urolithiasis and tends to develop into urosepsis rapidly (4). Sepsis and severe sepsis are life-threatening situations requiring urgent medical intervention, placing a heavy burden on patients and society (4-6). Urosepsis refers to sepsis due to urinary tract or male reproductive system’s infection, accounting for approximately 9% of severe sepsis cases (4,7). Urosepsis has a very high mortality rate, rapid detection, and appropriate treatment initiation are crucial (8). For the management of urosepsis, early empiric antimicrobial therapy and source control is of utmost importance. Drainage of obstruction and abscesses and removal of foreign bodies is the most important strategy for source control and must be performed immediately (9). Therefore, it is extremely important to identify calculous pyonephrosis before making treatment decisions for patients with obstructive hydronephrosis. However, a fair proportion of patients with pyonephrosis are asymptomatic, and some patients have symptoms similar to those of acute pyelonephritis or hydronephrosis, which makes the early accurate identification of pyonephrosis challenging (10-12). Delayed diagnosis may sometimes result in catastrophic outcomes.
Several researchers have tried ultrasound, computerized tomography (CT), and magnetic resonance imaging (MRI) for preoperative prediction of pyonephrosis (13-15). However, these methods were found to have certain limitations and could not achieve satisfactory prediction efficacy. Moreover, pyonephrosis imaging findings were not entirely consistent due to various degrees of hydronephrosis and infection. This study aimed to develop predictive models for calculous pyonephrosis using clinical parameters, laboratory test results, and imaging findings.
Machine learning (ML) is the semi-automated extraction of knowledge and insight from data (16). Developed within the fields of statistics, computer science and artificial intelligence, it allows the training of algorithms that can discover and identify complex patterns and relationships faster than conventional statistical models that focus on only a handful of patient variables (16). The superior ability of ML algorithms to improve the accuracy of predicting diseases and subsequent outcomes compared to traditional statistical models has led to the extensive application of ML algorithms in the field of clinical research (17,18). Considering this, we applied ML algorithms to the dataset in the present study, in order to identify patients at high risk of harboring pyonephrosis before making treatment decisions. We present the following article in accordance with the TRIPOD reporting checklist (available at: http://dx.doi.org/10.21037/tau-20-1208).
Methods
This study has conformed to the provisions of the Declaration of Helsinki (as revised in 2013). The study was approved by the Institutional Review Board of Tongji Hospital, Tongji Medical College, Huazhong University of Science and Technology (2019#S1159), with a waiver of informed consent due to its retrospective nature.
Patient selection and study parameters
In this single-center retrospective study, we searched the medical records for all patients with calculous pyonephrosis or hydronephrosis at the Tongji Hospital of Tongji Medical College, Huazhong University of Science and Technology between March 2013 and March 2018. We used multiple imputation to fill in missing data. The inclusion criteria were as follows: (I) adult patients aged ≥18 years; (II) patients with upper urinary tract stones; (III) surgical procedures [retrograde ureteral stent insertion, percutaneous nephrostomy (PCN), or percutaneous nephrolithotomy (PCNL)] performed for all patients; and (IV) complete clinical data including signs or symptoms, imaging examinations (ultrasonography, abdominal X-ray, and non-enhanced CT), and laboratory test results. Exclusion criteria were as follows: (I) no hydronephrosis in the affected kidney; (II) had undergone nephrostomy or retrograde ureteral stent insertion before admission; (III) had received endoscopic surgery before admission and were admitted to our center to treat residual stones; (IV) had received ultrasonography and CT scans at other hospitals; and (V) had incomplete clinical data in medical records.
Preoperative clinical data of all enrolled patients included basic demographic data, clinical signs or symptoms (fever and renal colic), history of urinary tract infection (UTI) within the past 3 months, chronic comorbidities (hypertension and diabetes), characteristics of renal or ureteral stones, characteristics of the affected and contralateral kidneys, and laboratory analyses performed on blood and urine samples. The degree of hydronephrosis was classified as mild, moderate, and severe by ultrasound, according to Noble’s grading system (19). The relevant laboratory parameters included preoperative peripheral white blood cell (WBC) counts, preoperative peripheral neutrophil counts, serum C-reactive protein (CRP), urine leukocyte counts, urine nitrite, and urine culture results. Urine culture with a single microorganism growth of 105 colony forming units (CFU)/mL for a sterile midstream urine sample and 104 CFU/mL for a catheterized sample were considered positive results (20). The CT attenuation value [Hounsfield units (HU)] of renal pelvis urine was obtained and calculated automatically from picture archiving and communication systems (PACS) (13).
Confirmation of calculous pyonephrosis
The presence of upper urinary tract calculi was confirmed by experienced radiologists using non-enhanced CT scans. Pyonephrosis was defined as the presence of pus or purulence aggregated in the renal collecting duct system. Diagnosis of pyonephrosis was based on the pus observed by clinicians during endoscopic surgery or surgical drainage (PCN or retrograde ureteral stent insertion), which was known as the “gold standard”, and experienced urologists performed this at our center.
Development, validation, and performance of ML-based models
The primary dataset was randomly split into two datasets: 70% for model training and 30% for model testing. For model training, data from the training set were used to approximate model parameters. A total of 5 ML algorithms were performed to build predictive models: logistic regression (LR), LR optimized by the least absolute shrinkage and selection operator (Lasso) regularization (Lasso-LR), support vector machine (SVM) integrated with recursive feature elimination (RFE), random forest (RF) classifier, and extreme gradient boosting (XGBoost).
LR is one of the most common ML algorithms for the classification of binary outcomes. We performed univariate and multivariable LR analysis to investigate the association between clinical variables and pyonephrosis. Also, according to multivariable analysis results, we selected significant predictors (P<0.05) and their corresponding coefficients to construct the predictive model. The LR model was derived from the following formula:
[1]
where Y is the output, βi is the nonzero coefficient, and xi is the selected clinical feature based on the results of the multivariable LR analysis (21).
The Lasso is a popular ML algorithm with outstanding feature selection capability, and it preferentially shrinks some predictor coefficients to zero by penalizing the absolute values of the regression coefficients (22,23). In this study, the optimized LR coefficients were estimated given a boundary (“L1 Norm”) to the sum of absolute standardized regression coefficients (22,23). The Lasso-LR model was also derived from the formula (I).
To acquire the probability of pyonephrosis in the LR and Lasso-LR, we then converted output values of models to the probabilities (Pi) by employing a sigmoid function:
[2]
where Y is the output value of predictive models, and Pi indicates the probabilities of harboring pyonephrosis (21).
The SVM is a supervised learning model with an associated learning algorithm that analyzes data used for classification and regression (24). The objective of applying SVM is to find the best line in 2 dimensions or the best hyperplane in >2 dimensions to help separate the space into classes (24). In the present study, RFE was integrated with the SVM classifier training, and the SVM model training was based on the use of a radial basis function kernel. The RFE was initially proposed to enable SVM to perform feature selection by iteratively training a model, ranking features, and then removing the lowest ranking features (25). The iteration was repeated until the desired number of features was reached. By adding the ranked features returned by the SVM one by one from most to least important, we eventually selected parameters that produced the greatest accuracy and the lowest average error.
The RF is an ensemble learning method that performs classification or regression by combining the voting results of multiple decision trees; it has been employed extensively in the fields of clinical research and bioinformatics (26). Bootstrap aggregation, also called bagging, is the core of RF algorithms. Each decision tree is trained on randomly sampled subsets in the training data, while sampling is undertaken with the replacement. The final RF model is constructed based on the majority vote results from individually developed decision trees in the forest. In this study, we used metrics of the mean decrease in accuracy (MDA) and the mean decrease in Gini (MDG) to assess the importance of various features in constructing the RF model. The MDA of a variable is determined during the out of bag (OOB) error calculation phase. The more the RF accuracy decreases due to the exclusion of a single variable, the more important that variable is deemed. Therefore, variables with a large MDA are more important for the data classification (27). The MDG is the average of a variable’s total decrease in node impurity, weighted by the proportion of samples reaching that node in each decision tree in the RF (27). A higher MDG indicates higher variable importance.
Like the RF, gradient boosting is an ML algorithm for regression and classification problems, which produces a prediction model in the form of an ensemble of weak prediction models, typically decision trees. The XGBoost is one of the implementations of the gradient boosting concept. As an ensemble tree model, XGBoost uses multiple iterative gradient boosters to construct a strong classification system (28). It uses a more regularized model formalization to control over-fitting, which gives it better performance.
Model evaluation was carried out by examining discrimination. The receiver operating characteristic (ROC) curve analysis was used to evaluate the discrimination ability of predictive models in both the training and testing datasets; the area quantified each model’s discrimination ability under the ROC curve (AUC). Moreover, discrimination metrics including accuracy, sensitivity, specificity, Youden index (YI), positive predictive value (PPV), and negative predictive value (NPV) were also applied to assess the discriminative power of predictive models. Comparisons between ROC curves were performed using the method described by DeLong et al. (29). As LR analysis was one of the most widely used statistical methods, we used the LR model as the reference in the pairwise comparison of AUC value. Decision curve analysis (DCA) was conducted to determine the clinical net benefit associated with using the predictive models at different threshold probabilities in the patient cohort.
Statistical analysis
Data were analyzed using the statistical software SPSS version 22.0 (IBM Corp., NY, USA) and R software (Version 3.6.0; https://www.R-project.org). In both the training and testing datasets, patients were assigned to the pyonephrosis group and non-pyonephrosis group. The Mann-Whitney U test and chi-square test or Fisher’s exact test were applied to compare the demographic data and laboratory parameters of the pyonephrosis and non-pyonephrosis groups. The following R packages were used in data analysis: “rms”, “glmnet”, “caret”, “rpart”, “randomForest”, “gplots”, “e1071”, “kernlab”, “pROC”, “nricens”, “xgboost”, “DiagrammeR”, “rsvg”, and “MachineShop”. Statistical significance was set as P<0.05.
Results
Baseline clinical characteristics and laboratory test results
Strictly conforming to the inclusion and exclusion criteria, 322 patients were considered eligible for enrollment in the present study. Table 1 lists the preoperative clinical characteristics of the total population (n=322). All 322 obstructive hydronephrosis patients with upper urinary tract stones were divided into the pyonephrosis (n=76) and non-pyonephrosis (n=246) groups. The pyonephrosis group was more likely to be associated with younger female patients. The distribution of the presence of renal colic, hypertension, diabetes, hyperuricemia, staghorn calculi, and congenital renal malformation was similar between the two groups. The two groups were also similar for stone size and serum creatinine levels. Patients with pyonephrosis had higher stone density (1,395 vs. 1,214 HU, P=0.001) and a higher attenuation value of the renal pelvis (14.45 vs. 6.40 HU, P<0.001) than those with non-pyonephrosis. More patients in the pyonephrosis group were associated with UTI, fever, severe hydronephrosis, and atrophy of the contralateral kidney (SHACK). The comparison of laboratory test results between the two groups is shown in Table 2. The pyonephrosis group had higher WBC counts, neutrophil counts, serum CRP level, urine leukocyte counts, and the possibility of harboring a positive urine culture than the non-pyonephrosis group. Sites were similar in the distribution of the presence of urinary nitrite. Additionally, baseline characteristics and laboratory test results were comparable in both the training (Tables S1,S2) and testing cohorts (Tables S3,S4), which were consistent with the overall population.
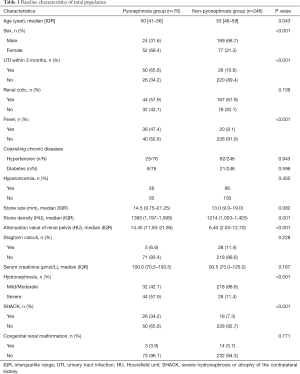
Full table
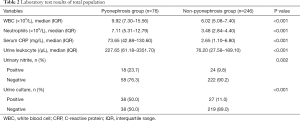
Full table
ML-assisted models
Using univariable and multivariable LR analyses, we looked at outcome predictive features. Table 3 details the results of these analyses in the training dataset. For the diagnosis of pyonephrosis, the attenuation value of the renal pelvis [odds ratio (OR) =1.38; 95% confidence interval (CI): 1.14–1.66; P=0.001], hydronephrosis (OR =22.35; 95% CI: 2.85–175.54; P=0.003), urine leukocyte (OR =1.001; 95% CI: 1.000–1.001; P=0.005) and urine culture (OR =14.29; 95% CI: 1.25–164.16; P=0.033) were the statistically significant elements in the multivariable analysis. According to their respective coefficients, the LR model was constructed using the following formula: Y = – 11.03 + 0.32 × (attenuation value of renal pelvis) + 3.12 × (hydronephrosis) + 0.001 × (urine leukocyte) + 2.66 × (urine culture). In this formula, binary predictor variables were valued as 0 or 1.
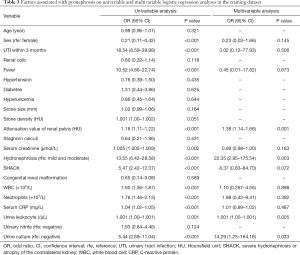
Full table
Considering that the absolute value of the coefficients from the Lasso regression analysis represents each feature’s contribution, the clinical features with an absolute value of the coefficients >0.1 were selected as the parameters included in the construction of the Lasso-LR model. Finally, sex, staghorn calculi, hypertension, renal colic, attenuation value of renal pelvis, neutrophils, UTI within 3 months, urine culture, SHACK, and hydronephrosis were the selected features (Figure 1). The Lasso-LR model was conducted by using the following formula: Y = –5.11 − 0.68 × (sex) – 0.47 × (staghorn calculi) − 0.28 × (hypertension) – 0.27 × (renal colic) + 0.13 × (attenuation value of renal pelvis) + 0.19 × (neutrophils) + 1.09 × (UTI within 3 months) + 1.17 × (urine culture) + 1.26 × SHACK + 1.81 × (hydronephrosis). Binary predictor variables were also valued as 0 or 1 in this formula.

Distribution for features with RFE-SVM analysis is depicted in Figure 2A. In the RFE-SVM analysis, 15 clinical parameters were selected as the final candidates for constructing the predictive model without impacting the prediction accuracy of the model, including serum CRP, neutrophils, WBC, UTI within 3 months, hydronephrosis, attenuation value of renal pelvis, fever, urine culture, sex, SHACK, serum creatinine, stone density, urine leukocyte, age, and stone size (Figure 2B). As depicted in Figure 2B, with the ranking of the features ahead being added to the SVM model one by one, the AUC value of the model also increased incrementally, and the addition of stone size yielded the highest AUC.
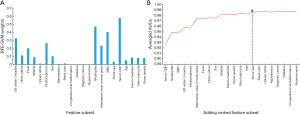
The RF model’s feature selection process and the distribution of feature importance are illustrated in Figure 3. Based on different combinations of clinical parameters, each tree in the forest votes for the major classification, and the final classification of the RF model is derived from the majority of these votes (Figure 3B). The best number of trees and the best number of variables tried at each split were 76 and 5, respectively. The OOB estimate of error rate was 5.33%, suggesting that the generalization error was satisfactory. The top 5 most important features for the MDA were serum CRP, neutrophils, attenuation value of renal pelvis, WBC, and hydronephrosis (Figure 3A). For the MDG, the top 5 most relevant predictors were serum CRP, neutrophils, WBC, attenuation value of renal pelvis, and UTI within 3 months (Figure 3A). Overall, the results of feature importance ranking were similar between MDA and MDG.
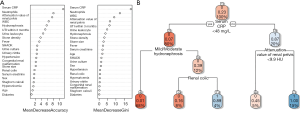
The XGBoost model is developed based on the gradient boosting trees. The typical ensemble of two trees in the model is shown in Figure 4A. The gain on each node was the contribution of the selected feature, and we eventually acquired the ranking results of feature importance after summing up all the contributions for each feature. Also, we performed clustering on features according to their importance ranking order (Figure 4B). In the XGBoost model, serum CRP was the most important clinical feature, followed by renal pelvis’s attenuation value, neutrophils, and hydronephrosis.
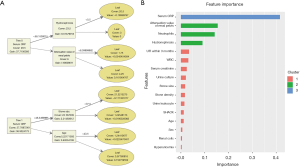
Comparison between ML-based models
Among these models, SVM yielded the highest AUC (0.985, 95% CI: 0.970–1.000), followed by XGBoost (AUC =0.981, 95% CI: 0.954–1.000), Lasso-LR (AUC =0.977, 95% CI: 0.958–0.996), LR (AUC =0.936, 95% CI: 0.905–0.968), and RF (AUC =0.920, 95% CI: 0.870–0.970) (Figure 5A). Similarly, in the testing dataset, SVM yielded the highest AUC (0.977, 95% CI: 0.952–1.000), followed by Lasso-LR (AUC =0.959, 95% CI: 0.921–0.997), XGBoost (AUC =0.958, 95% CI: 0.902–1.000), LR (AUC =0.932, 95% CI: 0.878–0.987), and RF (AUC =0.868, 95% CI: 0.779–0.958) (Figure 5B). The XGBoost model had the highest YI (0.962) than the other models (Table 4). Because the YI was calculated as a summation of the sensitivity and specificity minus 1, the highest YI indicated that both the sensitivity and specificity of the XGBoost model are reasonably well relative to other predictive models. Using the DeLong method with Bonferroni correction, a pairwise comparison of ROC curves was performed. The AUCs of Lasso-LR, SVM, and XGBoost were significantly greater than that of LR, while there were no significant differences between the AUC of LR and that of RF (Table S5). The DCA showed that the SVM and XGBoost had a higher net benefit for threshold probabilities >20% (Figure 6). Compared with the LR model, other ML-based models significantly improved risk prediction at calculous pyonephrosis threshold probabilities >10%.
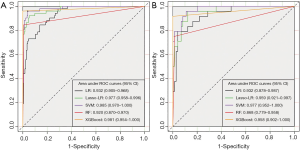
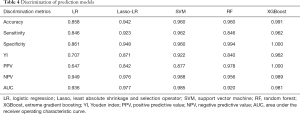
Full table
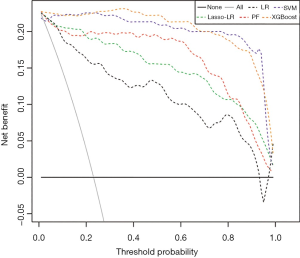
Discussion
Hydronephrosis is the dilation of the renal pelvis or calyces due to obstruction to urine flow downstream. On the other hand, pyonephrosis refers to an infected hydronephrosis status associated with suppurative destruction of the renal parenchyma (1-3). Patients with calculous pyonephrosis may present with a variety of clinical symptoms ranging from asymptomatic bacteriuria to urosepsis. Nonspecific complaints and symptoms may be the only manifestations noted in some patients with calculous pyonephrosis; therefore, it can sometimes be difficult to differentiate between infected hydronephrosis and true pyonephrosis (12). Due to the high risk of progressing into urosepsis, sepsis-related morbidity and mortality, and the renal functional loss, rapid diagnosis and treatment are essential to avoid extravasation, sepsis, and parenchymal loss (12). Therefore, early accurate identification of calculous pyonephrosis is of paramount importance. Unfortunately, currently, there are no widely accepted predictive models to predict calculous pyonephrosis accurately, and the discrimination ability of various models remains modest (13,15). To date, the preoperative diagnosis of calculous pyonephrosis is still highly dependent on the good reasoning and judgment of clinicians. Predictors of developing pyonephrosis include a long duration of symptoms, abnormal anatomy, and the presence of renal calculi (4,30). Laboratory tests, including blood counts, serum chemistry and creatinine, and urinalysis with culture, also have important implications in diagnosing pyonephrosis.
ML algorithms have been successfully used for predicting outcomes in other fields of medicine, including the identification of lung cancer based on routine blood indices and the in-hospital rupture of type A aortic dissection (31,32). Given the excellent performance of ML algorithms in classification, we employed 5 ML algorithms in our study to determine relevant risk factors. We then developed and validated 5 novel prediction models to identify patients at high risk of harboring calculous pyonephrosis before making treatment decisions.
It is thought that UTIs are more common in women (33). We found more female patients in the pyonephrosis group of both the training and testing datasets. Sex was a significant predictor in the construction of both the Lasso-LR and SVM models. However, the result of multivariable LR analysis showed that gender was not a significant risk factor for pyonephrosis. This was consistent with the finding of a previous publication (30). In comparing laboratory test results, all variables except urinary nitrite showed significant differences between the pyonephrosis group and non-pyonephrosis group.
For the evaluation of hydronephrosis, non-contrast CT was previously often focused on nonspecific findings, such as the thickening of the renal pelvis and stranding of the perirenal fat (34). However, recent studies have successfully demonstrated that the CT attenuation value could be used to differentiate hydronephrosis from pyonephrosis (13,15). Not surprisingly, renal pelvis’ attenuation value on non-contrast CT was one of the most important indicators in all 5 predictive models. Moreover, the severity of hydronephrosis was irrelevant to pyonephrosis diagnosis in a study performed by Yuruk et al. (15). In contrast, in our patient cohort, patients with severe hydronephrosis were more likely to have pyonephrosis than those with mild or moderate hydronephrosis.
It is well known that CRP is one of the most commonly used biomarkers of inflammation and could be used for upper and lower UTI differentiation (35). Somewhat intriguingly, in our study, serum CRP was the strongest predictor identified by SVM, RF, and XGBoost. Meanwhile, serum CRP was not significantly related to pyonephrosis in LR and Lasso-LR.
Although both WBC and neutrophil counts are the most important, nonspecific biomarkers of infectious disease, neutrophils outperformed WBCs in the prediction of pyonephrosis. Concerning symptoms, renal colic, and fever did not show a major contribution in the 5 models. This may be in part due to the variability in clinical symptoms of pyonephrosis. Also, the predictive value of characteristics of upper urinary stones (stone size, stone density, and staghorn calculi) was also unsatisfactory. Nonetheless, contrary to our findings, Patodia et al. (30) reported that the presence of staghorn calculi was independently associated with pyonephrosis in a multivariable LR analysis of their patient cohort.
Urinalysis and urine culture play a key role in the diagnosis of UTIs (36). Data obtained in our study showed that urine leukocytes and urine culture were important predictors across all 5 models. Regrettably, we did not include the results of the urinalysis and urine culture of samples from the obstructed collecting system in this study.
For the performance of ML-based models, the Lasso-LR model showed the best discriminative power with an AUC of 0.985 (95% CI: 0.970–1.000), followed by XGBoost (AUC =0.981, 95% CI: 0.954–1.000), Lasso-LR (AUC =0.977, 95% CI: 0.958–0.996), LR (AUC =0.936, 95% CI: 0.905–0.968), and RF (AUC =0.920, 95% CI: 0.870–0.970). Additionally, all models had satisfactory sensitivity, specificity, PPV, and NPV. In a similar study regarding the evaluation of the single-use of the attenuation value of the renal pelvis in predicting pyonephrosis, Yuruk et al. (15) demonstrated that a cutoff value of HU >9.21 could be used to diagnose the presence of pyonephrosis with 65.96% sensitivity and 87.93% specificity. This implied that the inclusion of multiple clinical predictor variables into a statistical classification model might significantly improve predictive ability (discrimination and clinical net benefit) compared to the model based on a single important predictor. Many studies have demonstrated that ML-assisted models were markedly better than conventional statistical modeling in predicting clinical outcomes (37,38). In the present study, all models except RF outperformed LR. This may be due in part to the fact that other ML algorithms perform better in dealing with complex, high-dimensional data compared with a conventional regression algorithm. It is noteworthy that XGBoost seemed to be the model with the highest discrimination power given all discrimination metrics. Accordingly, we strongly recommend the use of the XGBoost model in the early diagnosis of calculous pyonephrosis. Our models performed similarly on the training and testing datasets, indicating that overfitting was not a frustrating issue of ML algorithms within our data.
Despite several strengths, our study had certain limitations. First, the data on patients with obstructed hydronephrosis in our study cohort were retrospectively collected at a single institution, which may have resulted in selection bias. Second, we did not introduce the results of the urinalysis and urine culture of samples from the obstructed renal pelvis, which may have offered better predictive value. Also, it should be noted that our present models’ excellent discriminatory efficiency might be related to the small sample size of this study. Thus, before a broader clinical application, a prospective external validation on a larger scale is warranted.
Conclusions
In summary, we developed 5 ML-based models to assist clinicians in the early identification of the individualized risk of pyonephrosis for patients with obstructed hydronephrosis. Altogether, the XGBoost model seemed to have the best discriminative power. Our results illustrated the benefits associated with the use of ML-assisted models. We believe that the use of these models will protect patients and clinicians in the future and allow clinicians to avoid potentially severe septic complications associated with an infected obstructed system through the early and accurate identification of patients with calculous pyonephrosis. Of course, further validation across multiple institutions involving a large sample size is needed.
Acknowledgments
Funding: None.
Footnote
Reporting Checklist: The authors have completed the TRIPOD reporting checklist. Available at http://dx.doi.org/10.21037/tau-20-1208
Data Sharing Statement: Available at http://dx.doi.org/10.21037/tau-20-1208
Conflicts of Interest: All authors have completed the ICMJE uniform disclosure form (available at http://dx.doi.org/10.21037/tau-20-1208). The authors have no conflicts of interest to declare.
Ethical Statement: The authors are accountable for all aspects of the work in ensuring that questions related to the accuracy or integrity of any part of the work are appropriately investigated and resolved. This study has conformed to the provisions of the Declaration of Helsinki (as revised in 2013). The study was approved by the Institutional Review Board of Tongji Hospital, Tongji Medical College, Huazhong University of Science and Technology (2019#S1159), with a waiver of informed consent due to its retrospective nature.
Open Access Statement: This is an Open Access article distributed in accordance with the Creative Commons Attribution-NonCommercial-NoDerivs 4.0 International License (CC BY-NC-ND 4.0), which permits the non-commercial replication and distribution of the article with the strict proviso that no changes or edits are made and the original work is properly cited (including links to both the formal publication through the relevant DOI and the license). See: https://creativecommons.org/licenses/by-nc-nd/4.0/.
References
- Choi J, Jang J, Choi H, et al. Ultrasonographic features of pyonephrosis in dogs. Vet Radiol Ultrasound 2010;51:548-53. [Crossref] [PubMed]
- Pearle MS, Pierce HL, Miller GL, et al. Optimal method of urgent decompression of the collecting system for obstruction and infection due to ureteral calculi. J Urol 1998;160:1260-4. [Crossref] [PubMed]
- Kuntz JA, Berent AC, Weisse CW, et al. Double pigtail ureteral stenting and renal pelvic lavage for renal-sparing treatment of obstructive pyonephrosis in dogs: 13 cases (2008-2012). J Am Vet Med Assoc 2015;246:216-25. [Crossref] [PubMed]
- Liang X, Huang J, Xing M, et al. Risk factors and outcomes of urosepsis in patients with calculous pyonephrosis receiving surgical intervention: a single-center retrospective study. BMC Anesthesiol 2019;19:61. [Crossref] [PubMed]
- Stoller J, Halpin L, Weis M, et al. Epidemiology of severe sepsis: 2008-2012. J Crit Care 2016;31:58-62. [Crossref] [PubMed]
- Khwannimit B, Bhurayanontachai R. The direct costs of intensive care management and risk factors for financial burden of patients with severe sepsis and septic shock. J Crit Care 2015;30:929-34. [Crossref] [PubMed]
- Levy MM, Artigas A, Phillips GS, et al. Outcomes of the Surviving Sepsis Campaign in intensive care units in the USA and Europe: a prospective cohort study. Lancet Infect Dis 2012;12:919-24. [Crossref] [PubMed]
- Bonkat G, Cai T, Veeratterapillay R, et al. Management of Urosepsis in 2018. Eur Urol Focus 2019;5:5-9. [Crossref] [PubMed]
- Bonkat G, Pickard R, Bartoletti R, et al. Guidelines on urological infections. Arnhem, The Netherlands: European Association of Urology, 2018.
- Li H, Xie F, Zhao C, et al. Primary mucinous adenocarcinoma of the renal pelvis misdiagnosed as calculous pyonephrosis: a case report and literature review. Transl Androl Urol 2020;9:781-8. [Crossref] [PubMed]
- Browne RF, Zwirewich C, Torreggiani WC. Imaging of urinary tract infection in the adult. Eur Radiol 2004;14 Suppl 3:E168-83. [PubMed]
- Li AC, Regalado SP. Emergent percutaneous nephrostomy for the diagnosis and management of pyonephrosis. Semin Intervent Radiol 2012;29:218-25. [Crossref] [PubMed]
- Basmaci I, Sefik E. A novel use of attenuation value (Hounsfield unit) in non-contrast CT: diagnosis of pyonephrosis in obstructed systems. Int Urol Nephrol 2020;52:9-14. [Crossref] [PubMed]
- Chan JHM, Tsui EYK, Luk SH, et al. MR diffusion-weighted imaging of kidney: Differentiation between hydronephrosis and pyonephrosis. Clin Imaging 2001;25:110-3. [Crossref] [PubMed]
- Yuruk E, Tuken M, Sulejman S, et al. Computerized tomography attenuation values can be used to differentiate hydronephrosis from pyonephrosis. World J Urol 2017;35:437-42. [Crossref] [PubMed]
- Lynch CJ, Liston C. New machine-learning technologies for computer-aided diagnosis. Nat Med 2018;24:1304-5. [Crossref] [PubMed]
- Chen JH, Asch SM. Machine Learning and Prediction in Medicine - Beyond the Peak of Inflated Expectations. N Engl J Med 2017;376:2507-9. [Crossref] [PubMed]
- Rajkomar A, Dean J, Kohane I. Machine Learning in Medicine. N Engl J Med 2019;380:1347-58. [Crossref] [PubMed]
- Noble VE, Brown DF. Renal ultrasound. Emerg Med Clin North Am 2004;22:641-59. [Crossref] [PubMed]
- Foong KS, Munigala S, Jackups R Jr, et al. Incidence and Diagnostic Yield of Repeat Urine Culture in Hospitalized Patients: an Opportunity for Diagnostic Stewardship. J Clin Microbiol 2019;57:e00910-19. [Crossref] [PubMed]
- Hou Y, Bao ML, Wu CJ, et al. A machine learning-assisted decision-support model to better identify patients with prostate cancer requiring an extended pelvic lymph node dissection. BJU Int 2019;124:972-83. [Crossref] [PubMed]
- Archer KJ, Williams AA. L1 penalized continuation ratio models for ordinal response prediction using high-dimensional datasets. Stat Med 2012;31:1464-74. [Crossref] [PubMed]
- Van Calster B, van Smeden M, De Cock B, et al. Regression shrinkage methods for clinical prediction models do not guarantee improved performance: Simulation study. Stat Methods Med Res 2020;29:3166-78. [Crossref] [PubMed]
- Van Belle V, Van Calster B, Van Huffel S, et al. Explaining Support Vector Machines: A Color Based Nomogram. PLoS One 2016;11:e0164568. [Crossref] [PubMed]
- Guyon I, Weston J, Barnhill S, et al. Gene Selection for Cancer Classification using Support Vector Machines. Mach Learn 2002;46:389-422. [Crossref]
- Petralia F, Wang P, Yang J, et al. Integrative random forest for gene regulatory network inference. Bioinformatics 2015;31:i197-205. [Crossref] [PubMed]
- Han H, Guo X, Yu H. Variable selection using Mean Decrease Accuracy and Mean Decrease Gini based on Random Forest. 2016 7th IEEE International Conference on Software Engineering and Service Science (ICSESS); 26-28 Aug. 2016; Beijing, China. IEEE, 2016.
- Lopez V, Fernandez A, Garcia S, et al. An insight into classification with imbalanced data: Empirical results and current trends on using data intrinsic characteristics. Information Sciences 2013;250:113-41. [Crossref]
- DeLong ER, DeLong DM, Clarke-Pearson DL. Comparing the areas under two or more correlated receiver operating characteristic curves: a nonparametric approach. Biometrics 1988;44:837-45. [Crossref] [PubMed]
- Patodia M, Goel A, Singh V, et al. Are there any predictors of pyonephrosis in patients with renal calculus disease? Urolithiasis 2017;45:415-20. [Crossref] [PubMed]
- Wu J, Zan X, Gao L, et al. A Machine Learning Method for Identifying Lung Cancer Based on Routine Blood Indices: Qualitative Feasibility Study. JMIR Med Inform 2019;7:e13476. [Crossref] [PubMed]
- Wu J, Qiu J, Xie E, et al. Predicting in-hospital rupture of type A aortic dissection using Random Forest. J Thorac Dis 2019;11:4634-46. [Crossref] [PubMed]
- Flores-Mireles AL, Walker JN, Caparon M, et al. Urinary tract infections: epidemiology, mechanisms of infection and treatment options. Nat Rev Microbiol 2015;13:269-84. [Crossref] [PubMed]
- Fultz PJ, Hampton WR, Totterman SM. Computed tomography of pyonephrosis. Abdom Imaging 1993;18:82-7. [Crossref] [PubMed]
- Xu RY, Liu HW, Liu JL, et al. Procalcitonin and C-reactive protein in urinary tract infection diagnosis. BMC Urol 2014;14:45. [Crossref] [PubMed]
- Gupta K, Grigoryan L, Trautner B. Urinary Tract Infection. Ann Intern Med 2017;167:ITC49-ITC64. [Crossref] [PubMed]
- Ross EG, Shah NH, Dalman RL, et al. The use of machine learning for the identification of peripheral artery disease and future mortality risk. J Vasc Surg 2016;64:1515-1522.e3. [Crossref] [PubMed]
- Wong NC, Lam C, Patterson L, et al. Use of machine learning to predict early biochemical recurrence after robot-assisted prostatectomy. BJU Int 2019;123:51-7. [Crossref] [PubMed]