Diagnostic accuracy of Raman spectroscopy for prostate cancer: a systematic review and meta-analysis
Introduction
Prostate cancer (PC), of which 70% occur in developed countries is the most common male cancer with 174,650 newly diagnosed cases and ranks second in cancer related death in 2019 in the United states (1,2). Thus, it causes a considerable public-health burden, but there is a strong potential to reduce PC specific mortality rates via screening (3).
In the past 20 years, the use of serum prostate-specific antigen (PSA) levels as a diagnostic tool for PC has increased detection at an early stage by increasing the number of men suggested for prostate biopsy (4-6). However, the level of PSA in serum is not an ideal cancer marker, because elevated PSA levels in many other conditions can cause overdiagnosis (4,5). Meanwhile, the gold standard for detection of PC is pathological examination using ultrasound-guided transrectal prostate biopsy (7). However, this procedure is invasive, accompanied by significant risk of complications, and costly (6). Researchers have been pursuing minimally invasive diagnostic methods that can provide diagnostic information at the molecular level and be reliable because of their high specificity and sensitivity (8).
In this respect, Raman spectroscopy (RS) has recently received attention as an attractive alternative for cancer detection (9). RS, which analyzes inelastic scattering of a photon having unique energy levels depending on every molecule type, has been used as an important diagnostic tool in many research disciplines (10,11). With development of spectroscopic instruments and technology, RS has advanced to evaluate cancer and precancerous lesions in multiple organs (12,13). RS has also been applied in the area of PC, and studies on it have revealed variations from adenocarcinoma to benign prostatic hyperplasia (BPH) at the molecular level (14,15). Crow et al. confirmed that RS can accurately discriminate BPH and three different grades of PC through a diagnostic algorithm using principal components analysis (PCA) method (16). Lopes et al. helped establish a concise spectral model to predict the concentration of spectral features to identify normal, BPH, and prostate carcinoma tissues in vitro (8).
The objective of our study was to work up a spectral model based on dispersive RS to differentiate the prostate biochemical differences between benign lesions and malignancy (14). Despite these efforts, it is difficult to define RS as a critical diagnostic method, because the number of samples for studies was small, and the sampling method, diagnostic algorithms, analysis tools, and RS settings in previous studies were not unified. Therefore, to verify the accuracy of RS as a diagnostic tool for PC by using current evidence, we did both a qualitative and quantitative analysis, as was essential.
We present the following article in accordance with the PRISMA reporting checklist (available at http://dx.doi.org/10.21037/tau-20-924).
Methods
Search strategy
We did comprehensive literature searches in the PubMed/Medline, Embase, and Cochrane library databases through March 2019. The terms for searching used included “prostate cancer” or “prostate tumor” or “prostate carcinoma” or “prostate malignancy” and “Raman” or “RS” or “Raman spectra” or “Raman spectrometry”. There were no restrictions on language or research type when doing the initial literature searches. Two authors (DK Kim and JH Kim) separately reviewed the titles and abstracts pursuant to inclusion and exclusion criteria and reviewed the identified articles. When opinions between each author are divided, they were solved by consensus through means of discussions with each other.
Trial inclusion and exclusion criteria
Included participant for this study were satisfied: blood/urine sample or cancer tissue of patients with PC; RS; blood/urine sample or prostate tissue of healthy volunteers; reported values [true positives (TP), true negatives (TN), false positives (FP) and false negatives (FN)] in sufficient detail to establish sensitivity and specificity at specified cutoff values for evaluating the diagnostic accuracy; and original articles. Whereas, studies involving non-human subject, studies with no control group, case reports, reviews, and duplicate studies were excluded.
Data extraction
Two authors (DK Kim and JJ Park) examined the full text of selected studies and extracted the trial level data from each study separately. When opinions between each author are divided, they were solved by consensus through means of discussions with each other. Extracted data contained study design, inclusion and exclusion criteria, country in which the study was conducted, number of patients or sample, sample type, main type of Raman algorithm, laser diode, and type of RS. The quantitative data, including TP, TN, FP, and FN, were also extracted directly or calculated from the sensitivity, specificity, positive predictive value (PPV), and negative predictive value (NPV) reported in included studies.
Study quality assessments and quality of evidence
The methodological quality was assessed using QUADAS-2. Risk of bias and applicability judgments in QUADAS-2 was assessed according to four domains, including patient selection, index test, reference standard, and flow and timing. Both the risk of bias and concerns about applicability were evaluated at three levels (low, high, and unclear). QUADAS-2 was done by Review Manager 5.3 (The Nordic Cochrane Center, The Cochrane Collaboration, Copenhagen, Denmark).
Statistical analysis
The accuracy of RS for diagnosis of PC was evaluated by means of pooled sensitivity, specificity, positive likelihood ratio, and negative likelihood ratio (NLR) values along with corresponding 95% confidence interval (CI). Moreover, a summary receiver operating characteristic (SROC) curve was also created by evaluating the effect of the threshold on the result according to the Moses-Shapiro-Littenberg method (17). For further assessing the statistical heterogeneity between trials, the I2 statistic and chi-square test were used for analysis. Either a P<0.05 for the Cochran Q statistic or an I2 statistic of >50% meant significant heterogeneity between trials (18). The publication bias wasn’t measured, because there is no established method for evaluating it in diagnostic meta-analysis. All of the above statistical analyses were done using Meta-Disc Version 1.4 (19).
Results
Systematic review process
The systematic review process using the PRISMA statement is summarized in Figure 1. Only published studies were included, in order to avoid publication bias. After a first literature search, we founded a total of 72 articles, which were reduced to 39 after duplicate removal. Then, 20 articles were removed by review of the titles and abstracts. Finally, 19 studies were included for qualitative analysis. Among them, five studies were selected in the final quantitative analysis by screening of the full text of the nineteen studies according to the inclusion and exclusion criteria. Table 1 shows the characteristics of the 5 studies included (8,14,16,20,21).
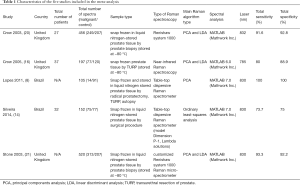
Full table
Among all included studies in the meta-analysis, three evaluated the diagnostic performance of RS in vitro, and only one study was conducted in vivo. Not all the eligible studies were published in English. Two studies were performed in Brazil. Others were conducted in the United Kingdom. The sample type was tissue or peripheral blood. Type of RS was not consistent in all studies.
Outcome
The extracted data of five studies were pooled and analyzed. We assessed overall diagnostic accuracy by calculating sensitivity, specificity, PLR, NLR, and diagnostic odds ratio (DOR). The overall sensitivity of RS was 89% (95% CI: 0.87–0.91) and specificity of RS was 91% (95% CI: 0.89–0.93), respectively (Figure 2). The heterogeneity of the among-study was statistically significant in the results of the sensitivity (Cochran Q statistic, P=0.000; I2 statistic, 87.1%) and specificity (Cochran Q statistic, P=0.000; I2 statistic, 88.8%). The overall PLR and NLR were 9.12 (95% CI: 4.15–20.08) and 0.14 (95% CI: 0.07–0.29), respectively. The DOR of RS demonstrated high accuracy (73.32; 95% CI: 18.43–291.73). The area under the curves (AUCs) of SROC curves was 0.93 (Figure 3).
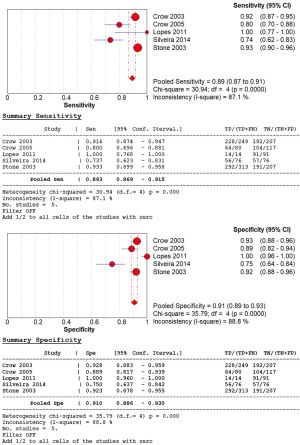
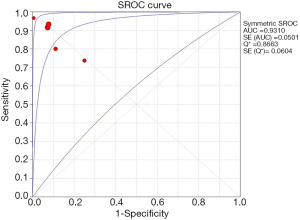
Quality assessment, qualitative risk of bias, and publication bias
Two authors separately assessed the quality of methodology according to the QUADAS-2. The assessments of the risk of bias and applicability concerns of the included studies are displayed in Figures 4,5. All domains of methodological quality were low except participant selection considering low sample size or in vitro level studies.
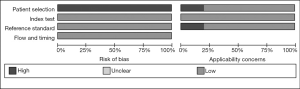
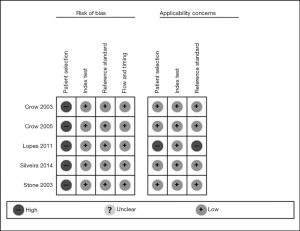
Discussion
By means of our study, it was confirmed that the use of RS for diagnosis of PC had a significant level of diagnostic accuracy. This study is, as far as we know, the first meta-analysis attempt to analyze the diagnostic accuracy of RS for PC, and we intend to contribute to an actual in vivo study by confirming the overall diagnostic accuracy by means of this study.
Several studies have been conducted on the application of RS to the diagnosis of various cancers. Lyng et al. attempted to distinguish malignant and benign lesions of the breast using RS in 2019 to identify significant spectral differences between benign and malignant tumors (22). In addition, Zhao et al. confirmed diagnostic applicability of RS for in vivo skin cancer diagnosis in 2017 (23). By means of these studies, the field in which RS is actually used in clinical practice is increasing, and our study is also aiming at this clinical application in the prostate-cancer field.
Recently, several studies have shown that RS using discriminant analysis (DA) has the potential to be used as a tool to distinguish prostate diseases from normal prostate tissues in vitro. Those studies investigated possibilities of RS for macroscopic identification of the main biochemicals presented in both normal and cancerous tissues for cancer diagnosis, and confirmation of the microscopic biochemical changes in cancerous cells for cancer staging. Also, RS has been able to identify endogenous biological markers related to benign and malignant prostate disease, such as proteins/amino acids, carbohydrates, lipids/phospholipids, and nucleic acids (14,16,24).
The discernment of tissues using the estimated Raman concentration and the Euclidean distance was supposed to be a competent method to discover the spectral features that are specific to a particular group, because the biochemical differences in the tissue related with the diseases can be easily founded and quantified by applying a proper spectral model (14,25). Aspects of transition at the molecular level of components that are associated with the pathophysiology cause fine but significant differences in the spectra (14,21). This study analyzed several works that attempted to identify differences in the spectra between normal prostate and PC in vitro. Structurally, the prostate gland is made of a glandular epithelium, smooth muscles, stroma, and a fibrous capsule, and these tissues are made of cells composed of various components. Malignant metamorphosis can occur both in the normal prostate and in benign prostate hyperplasia (8).
Dispersive RS has been used as an optical diagnostic method for both benign and malignant prostatic lesions. The leading distinction in the bands of PC compared to those of other tissues is observed in bands of structural proteins, cellular proteins, nucleic acids, and amino acids, such as tyrosine (21). Meanwhile, the spectra of normal prostates and benign hyperplasia biochemically similar to each other; so the BPH distinguishes the normal prostate by the presence of hyperplasia, without cancerous features (8).
The studies included in this meta-analysis used prostate tissue or urine samples. In one of the included studies, a urine sample was used; it showed a very high accuracy. However, despite the high diagnostic accuracy, the number of samples is very small, so further research is needed. For the application of RS, the pretreatment of prostate tissue was mainly done using liquid nitrogen, and the various methods of collecting tissue included biopsy, transurethral resection of the prostate (TURP), radical prostatectomy, and autopsy.
Some studies have suggested the use of multivariate analysis, such as PCA and DA (26). We can obtain the proper spectral information, which is determined by the composition of the analyzed substance, by distinguishing it from the random, uncorrelated variations by means of PCA. Crow et al. adopted RS to confirm and stage prostate adenocarcinoma in vitro using prostate tissues acquired by means of biopsy, using the PCA and linear discriminant analysis (LDA) (20). Stone et al. (21) examined and classified prostate tissues with BPH and PC by using Raman micro-spectrometry, using PCA and LDA. Crow et al. gathered Raman spectra from tissue fragments obtained by transurethral resection of prostates with the help of a fiber-optic cable combined with a Raman spectrometer, then developed an algorithm based on PCA and LDA for optical diagnosis and evaluated it in terms of sensitivity and specificity. Also, they researched cultures of human PC cell lines using micro-RS and established an algorithm to classify the samples by the method of PCA and LDA (16).
We included 6 in vitro studies. The other 15 studies included in the qualitative analysis were excluded from the studies finally selected for this meta-analysis, because those studies showed only pilot results or did not provide quantitative results for accuracy. The main limitations of our study lie in the small number of samples and in the heterogeneities caused by Raman settings, sample types, validation sets, and algorithms for analysis. These heterogeneities caused by discordance can lower the quality of the evidence in interpreting the results. Nevertheless, this is the first meta-analysis of the diagnostic accuracy of RS for PC. Also, we carried out GRADE quality assessment of the studies about diagnostic accuracy of RS (27). By means of this approach, we attempted to add evidence of the possibility of using RS as a diagnostic tool for PC. Well-designed, high-quality studies with large samples will be necessary to improve the level of evidence of these results.
Conclusions
RS is an optical diagnostic method with high potential for diagnosis and grading of PC and has advantages of real-time and convenient use. However, more research has to be done to overcome the limitations as evidence, because there were only a few samples in the current studies. Furthermore, in order to consider real-time use in an actual clinical setting, standardization and generalization of RS performance and analytical methods must be advanced.
Acknowledgments
Funding: This work was supported by the National Research Foundation of Korea (NRF) (No. 2018R1D1A1A02085980) and also supported by Soonchunhyang University Research Fund.
Footnote
Reporting Checklist: The authors have completed the PRISMA reporting checklist. Available at http://dx.doi.org/10.21037/tau-20-924
Peer Review File: Available at http://dx.doi.org/10.21037/tau-20-924
Conflicts of Interest: All authors have completed the ICMJE uniform disclosure form (available at http://dx.doi.org/10.21037/tau-20-924). The authors have no conflicts of interest to declare.
Ethical Statement: The authors are accountable for all aspects of the work in ensuring that questions related to the accuracy or integrity of any part of the work are appropriately investigated and resolved.
Open Access Statement: This is an Open Access article distributed in accordance with the Creative Commons Attribution-NonCommercial-NoDerivs 4.0 International License (CC BY-NC-ND 4.0), which permits the non-commercial replication and distribution of the article with the strict proviso that no changes or edits are made and the original work is properly cited (including links to both the formal publication through the relevant DOI and the license). See: https://creativecommons.org/licenses/by-nc-nd/4.0/.
References
- Taitt HE. Global trends and prostate cancer: a review of incidence, detection, and mortality as influenced by race, ethnicity, and geographic location. Am J Mens Health 2018;12:1807-23. [Crossref] [PubMed]
- Kohaar I, Petrovics G, Srivastava S. A rich array of prostate cancer molecular biomarkers: opportunities and challenges. Int J Mol Sci 2019;20:1813. [Crossref] [PubMed]
- Wong MC, Goggins WB, Wang HH, et al. Global incidence and mortality for prostate cancer: analysis of temporal patterns and trends in 36 countries. Eur Urol 2016;70:862-74. [Crossref] [PubMed]
- Ng CF, Chiu PK, Lam NY, et al. The Prostate Health Index in predicting initial prostate biopsy outcomes in Asian men with prostate-specific antigen levels of 4–10 ng/mL. Int Urol Nephrol 2014;46:711-7. [Crossref] [PubMed]
- Grönberg H, Adolfsson J, Aly M, et al. Prostate cancer screening in men aged 50-69 years (STHLM3): a prospective population-based diagnostic study. Lancet Oncol 2015;16:1667-76. [Crossref] [PubMed]
- Abdollah F, Ye Z, Miller DC, et al. Understanding the use of prostate biopsy among men with limited life expectancy in a statewide quality improvement collaborative. Eur Urol 2016;70:854-61. [Crossref] [PubMed]
- Glybochko PV, Alyaev YG, Amosov AV, et al. Multi-parametric MRI/US fusion guided biopsy for the diagnosis of prostate cancer. ur experience. Urologiia 2018.98-104. [Crossref] [PubMed]
- Lopes RM, Silveira L, Silva MARS, et al. Diagnostic model based on Raman spectra of normal, hyperplasia and prostate adenocarcinoma tissues in vitro. J Spectrosc (Hindawi) 2011;25:864710.
- Tu Q, Chang C. Diagnostic applications of Raman spectroscopy. Nanomedicine 2012;8:545-58. [Crossref] [PubMed]
- Huser T, Chan J. Raman spectroscopy for physiological investigations of tissues and cells. Adv Drug Deliv Rev 2015;89:57-70. [Crossref] [PubMed]
- Zhang Y, Hong H, Cai W. Imaging with Raman spectroscopy. Curr Pharm Biotechnol 2010;11:654-61. [Crossref] [PubMed]
- Kallaway C, Almond LM, Barr H, et al. Advances in the clinical application of Raman spectroscopy for cancer diagnostics. Photodiagnosis Photodyn Ther 2013;10:207-19. [Crossref] [PubMed]
- Lui H, Zhao J, McLean D, et al. Real-time Raman spectroscopy for in vivo skin cancer diagnosis. Cancer Res 2012;72:2491-500. [Crossref] [PubMed]
- Silveira L, Leite KRM, Silveira FL, et al. Discrimination of prostate carcinoma from benign prostate tissue fragments in vitro by estimating the gross biochemical alterations through Raman spectroscopy. Lasers Med Sci 2014;29:1469-77. [Crossref] [PubMed]
- Stone N, Kendall C, Shepherd N, et al. Near-infrared Raman spectroscopy for the classification of epithelial pre-cancers and cancers. J Raman Spectrosc 2002;33:564-73. [Crossref]
- Crow P, Molckovsky A, Stone N, et al. Assessment of fiberoptic near-infrared Raman spectroscopy for diagnosis of bladder and prostate cancer. Urology 2005;65:1126-30. [Crossref] [PubMed]
- Moses LE, Shapiro D, Littenberg B. Combining independent studies of a diagnostic test into a summary ROC curve: data‐analytic approaches and some additional considerations. Stat Med 1993;12:1293-316. [Crossref] [PubMed]
- Higgins JP, Thompson SG, Deeks JJ, et al. Measuring inconsistency in meta-analyses. BMJ 2003;327:557-60. [Crossref] [PubMed]
- Zamora J, Abraira V, Muriel A, et al. Meta-DiSc: a software for meta-analysis of test accuracy data. BMC Med Res Methodol 2006;6:31. [Crossref] [PubMed]
- Crow P, Stone N, Kendall C, et al. The use of Raman spectroscopy to identify and grade prostatic adenocarcinoma in vitro. Br J Cancer 2003;89:106-8. [Crossref] [PubMed]
- Stone N, Kendall C, Smith J, et al. Raman spectroscopy for identification of epithelial cancers. Faraday Discuss 2004;126:141-57; discussion 169-83. [Crossref] [PubMed]
- Lyng FM, Traynor D, Nguyen TNQ, et al. Discrimination of breast cancer from benign tumours using Raman spectroscopy. PLoS One 2019;14:e0212376. [Crossref] [PubMed]
- Zhao J, Zeng H, Kalia S, et al. Using Raman spectroscopy to detect and diagnose skin cancer in vivo. Dermatol Clin 2017;35:495-504. [Crossref] [PubMed]
- Stone N, Prieto MCH, Crow P, et al. The use of Raman spectroscopy to provide an estimation of the gross biochemistry associated with urological pathologies. Anal Bioanal Chem 2007;387:1657-68. [Crossref] [PubMed]
- Haka AS, Shafer-Peltier KE, Fitzmaurice M, et al. Diagnosing breast cancer by using Raman spectroscopy. Proc Natl Acad Sci U S A 2005;102:12371-6. [Crossref] [PubMed]
- Crow P, Barrass B, Kendall C, et al. The use of Raman spectroscopy to differentiate between different prostatic adenocarcinoma cell lines. Br J Cancer 2005;92:2166-70. [Crossref] [PubMed]
- Shapiro A, Gofrit ON, Pizov G, et al. Raman molecular imaging: a novel spectroscopic technique for diagnosis of bladder cancer in urine specimens. Eur Urol 2011;59:106-12. [Crossref] [PubMed]