Predictive model containing PI-RADS v2 score for postoperative seminal vesicle invasion among prostate cancer patients
Introduction
The incidence of seminal vesicle invasion (SVI) has decreased over time because of the application of serum prostate specific antigen (PSA) for prostate cancer screening, and the prevalence of SVI in a contemporary surgical series was 3–17.6% (1-5). SVI is a well-established indicator of adverse prognosis. Compared to organ-confined disease or extra prostatic extension (EPE), SVI is associated with worse outcome, a higher rate of recurrence and mortality (6). In patients with suspected SVI, multimodal therapy should be considered, such as radical prostatectomy (RP) combined with radiotherapy. Due to poor prognosis, treatment and operation should think carefully for patients with SVI. Therefore, accurate presurgical diagnosis of SVI is critical for urologists to make a treatment decision.
Several models that rely on clinical information and biopsy data have been developed for the prediction of prostate cancer staging, such as the Partin tables (7) and the nomogram developed by Gallina et al. (8). These models show high sensitivity (90%) but low specificity (30–60%) for the detection of SVI (9). On the contrary, the prediction accuracy of multiparametric magnetic resonance imaging (mpMRI) for SVI reveals high specificity (>90%) but poor and heterogeneous sensitivity (30–70%) overall (10). The recent models that added mpMRI information to predictive models yield better accuracy, such as the combination of mpMRI and the Partin tables (11) and the novel nomogram developed by Martini et al. (12). However, these models rely on the SVI findings of mpMRI, and none of the models currently integrate the Prostate Imaging Reporting and Data System version 2 (PI-RADS v2) score to predict post-operative SVI in patients without SVI on preoperative mpMRI.
Therefore, we aimed to construct a new model based on the PI-RADS v2 for the prediction of SVI in patients without SVI on preoperative mpMRI. This predictive model may also serve as a tool for the urologists to make surgical plans. We present the following article in accordance with the TRIPOD reporting checklist (available at http://dx.doi.org/10.21037/tau-20-989).
Methods
Patient population
We retrospectively reviewed a total of 787 patients who underwent RP for prostate cancer at our institution between January 2012 and July 2019. The inclusion criteria were as follows: patients with a preoperative serum PSA level <10 ng/mL who underwent preoperative mpMRI before prostate biopsies. Patients who underwent prior hormonal therapy or radiotherapy as well as patients with incomplete data were excluded from the cohort. Seven patients were excluded because of suspicious SVI on preoperative mpMRI. Finally, a total of 262 patients were enrolled in the study.
Clinicopathological characteristics
All patient data, including age, body mass index (BMI), preoperative PSA level, free/total PSA ratio (f/t PSA), prostate volume (PV) measured by trans rectal ultrasound (TRUS), the percentage of positive systematic biopsies, maximum cancer percentage per core, clinical stage, biopsy Gleason score (GS), and pathological characteristics of specimens following RP, were collected. PSA density (PSAD) was calculated by dividing total PSA by the PV. Cancer of the prostate risk assessment (CAPRA) score was calculated according to the UCSF-CAPRA scoring system.
Biopsy procedure and histopathology
All patients underwent TRUS-guided systematic biopsies. All biopsy specimens were evaluated by two dedicated genitourinary pathologists to determine the cancer diagnosis and the GS in positive cases. The patients were classified into the following five groups using the new GS grading system: grade group 1, GS 6; grade group 2, GS 3+4=7; grade group 3, GS 4+3=7; grade group 4, GS 8; and grade group 5, GS 9 and 10.
MpMRI
MRI was performed using a 3.0T Discovery MR750 HDx (GE Healthcare, Waukesha, WI, USA) without the use of an endorectal coil. The imaging protocol included axial T1-weighted images of the pelvis, axial T2-weighted fast spin-echo images centered on the prostate, and dynamic contrast-enhanced images. In addition, axial diffusion-weighted imaging was performed with b-values of 0, 800, and 1,400 s/mm2.
MpMRI interpretation.
MRI images were retrospectively interpreted by one of the two experienced radiologists with >5 years’ experience in reading prostate MRIs. Any disagreement in the process of interpretation was resolved by the senior adjudicating radiologist. According to PI-RADS v2 assessment categories, clinically significant cancer is highly unlikely or unlikely to be present in lesions of PI-RADS 1 or 2 (13,14). What’s more, lesions with PI-RADS >2 were defined as MRI-visible lesion, which could be considered for targeted biopsy. For patients with PI-RADS 3, it may be beneficial to perform follow-up rather than immediate biopsy, as most lesions can be reclassified after a manageable period of time (15). PI-RAD 4 or 5 means highly or very highly likely existence of clinically significant cancer, which biopsy should be considered (14). Targeted MR biopsy should be considered for PI-RADS assessment category 4 or 5 lesions but not for PI-RADS 1 or 2 (16). The 3 point “Likert” scale was associated directly with clinical decisions. So, the probability of cancer was evaluated and scored on a three-point scale based on the PI-RADS v2 score, where group “negative” (PI-RADS 1–2) = low probability, group “suspicious” (PI-RADS 3) = equivocal, and group “positive” (PI-RADS 4–5) = high or very high probability.
Statistical analysis
The endpoint of the study was the identification of the presence of SVI on the RP specimens. Univariate analysis was performed to investigate the associations between clinical and pathological risk factors and the presence of SVI in patients with negative SVI on mpMRI. The factors evaluated for the prediction of SVI were age, BMI, preoperative PSA level, f/t PSA, PV, PSAD, the percentage of positive systematic biopsies, maximum cancer percentage per core, CAPRA score, Gleason grade group (GGG), and PI-RADS v2 score. Continuous variables were compared using Student’s t-test and the Mann–Whitney U test, and categorical variables were compared using Chi-square test or Fisher’s exact text, as appropriate. Univariate and multivariate binary logistic regression analyses were conducted to identify independent predictors of SVI.
We constructed the logistic regression model for prediction of the diagnosis of SVI, by utilizing selected variables based on the results of multivariate logistic regression analysis. Discrimination was measured using the area under the curve (AUC) derived from the receiver operating characteristic (ROC) curves. With the optimal cutoff value according to Youden’s index, the performance of the model was assessed through analysis of sensitivity, specificity, positive predictive value, and negative predictive value.
Statistical analyses were performed using SPSS (version 24.0; SPSS Inc., Chicago, IL, USA). All analyses were two-sided, with statistical significance set at P<0.05. All procedures performed in this study were in accordance with the Declaration of Helsinki (as revised in 2013). Due to no influence on therapeutic strategy or need for patients’ follow-up, the Institutional Review Board replied that there was no need for ethical approval. And because of the retrospective nature of the research, the requirement for informed consent was waived.
Results
Baseline clinicopathological characteristics of the study cohort
The baseline clinical and pathological characteristics of the total 262 patients are summarized in Table 1. The median age was 66 years, the interquartile range (IQR) was 62–71 years, the median preoperative PSA level was 7.51 ng/mL (IQR, 6.07–8.63 ng/mL), and 30 patients (11.5%) presented with SVI on RP specimens.

Full table
Univariate and multivariate analyses
Univariate and multivariate logistic analyses in patients with negative SVI on mpMRI are described in Table 2. In the univariate analysis, SVI (+) patients had a statistically significantly higher percentage of positive systematic biopsies [odds ratio (OR), 1.019; 95% confidence interval (CI), 1.002–1.036; P=0.028], a higher maximum cancer percentage per core (OR, 1.019; 95% CI, 1.003–1.035; P=0.018), higher GGGs (OR, 1.747; 95% CI, 1.284–2.378; P<0.001), higher CAPRA score (OR 1.461; 95% CI, 1.119–1.908; P=0.005), and a higher PI-RADS score (OR, 4.095; 95% CI, 1.688–9.938; P=0.002) compared with SVI (-) patients. No difference was observed in age, BMI, PSA, f/t PSA, PV, and PSAD between SVI (+) and SVI (-) patients.
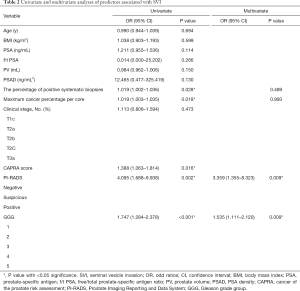
Full table
CAPRA score, calculated from five variables including the GS and the maximum cancer percentage per core, was insignificantly associated with SVI (P=0.179) in the multivariate analysis which containing three variables: CAPRA score, the percentage of positive systematic biopsies and PIRADS (Table S1).
In the multivariate analysis, which excluding CAPRA score, GGG and PI-RADS remained significantly associated with SVI, suggesting that these variables were independent risk predictors for the diagnosis of SVI.
With the application of the coefficients of the logistic function, a predictive model for postoperative SVI was constructed using selected risk factors, including GGG and PI-RADS scores, as follows:
logit (P) = ln [(P/(1-P)] = -4.661+0.428×GGG+1.212×PI-RADS.
ROC analysis was performed to assess the accuracy of this model, as shown in Figure 1. The predictive model applied for SVI yielded an AUC of 0.746, with a 95% CI of 0.662–0.831 (P<0.001). At the optimal cutoff predictive value of 0.100, it showed a sensitivity of 83.3% (95% CI, 64.5–93.7%), a specificity of 58.6% (95% CI, 52.0–65.0%), positive predictive value of 20.7% (95% CI, 14.1–29.2%), and a negative predictive value of 96.5% (95% CI, 91.5–98.7%).
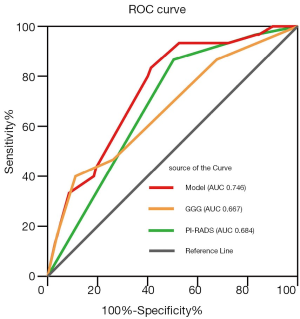
Discussion
In our cohort, SVI was reported on the RP specimens in 30 patients (11.5%). Using the selected risk factors, containing biopsy GGG and the PI-RADS v2 score, a predictive model for postoperative SVI was constructed, which revealed a high negative predictive value of 96.5% (95% CI, 91.5–98.7%) at the optimal cutoff predictive value.
SVI is defined as pathologically invasion of the muscular wall of the extraprostatic seminal vesicle. The incidence of SVI seemed to be heterogeneous, ranging from 3% to 17.6% in recent studies (1-5). In our cohort, the rate of SVI was 11.5%, which is consistent with the rate reported in recent literature which the median PSA level of the patients (5.9–7.8 ng/mL) is similar with ours (7.51 ng/mL) (3-5).
SVI is considered to be one of the most adverse prognostic findings in prostate cancer, which affects the biochemical progression-free survival and disease-specific survival. The 5- and 10-year biochemical failure rate for SVI was reported as 60% and 72%, which was significantly higher than that for pT2 patients (17). In a study containing 31,415 patients, Kristiansen et al. showed that compared to EPE alone, patients with SVI had a higher risk of clinical progression and death after RP (6).
According to National Comprehensive Cancer Network (NCCN) guideline version 2. 2020, patients with SVI are defined as very high-risk group, which treatment should think carefully for them. Asymptomatic patients with <5 years life expectancy is only considered for androgen deprivation therapy (ADT), external beam radiotherapy or observation. Only selected patients are recommended to undergo surgery and more effective treatments. Besides, patients with SVI influenced the choice of nerve-sparing RP, which could improve urinary continence and erectile function and is recommend in men with localized prostate cancer. According to EAU-ESTRO-SIOG Guidelines on Prostate Cancer, high risk of extracapsular disease is contraindication for nerve-sparing RP (18). However, EPE and SVI did not always show up together (19,20). In our cohort, EPE and SVI coexisted in 46.7% (14/30) of patients, and the other 16 patients without EPE who are unsuitable for nerve-sparing RP. It’s of great importance to exclude these unsuitable patients before operating nerve-sparing RP. On the contrary, in the case of high likelihood of SVI, additional therapies should be discussed, for example, neoadjuvant chemotherapy (21). In terms of prognosis and therapy strategy, preoperative prediction of SVI is of great significance.
MpMRI examination plays an important role in clinical staging, which is recommended to all patients who are suspected of PCs in our institution. According to a contemporary critical meta-analysis that included a total 5,677 patients from 34 studies, MRI shows high specificity (0.96, 95% CI, 0.95–0.97) but poor and heterogeneous sensitivity (0.58, 95% CI, 0.47–0.68) (10), which means that more than half of the patients with SVI were underestimated in the worst cases. This is possible because radiologists have focused on high-specificity reading to minimize unnecessary exclusion of men from curative treatment.
Several models have been constructed in an effort to predict SVI in prostate cancer. Lughezzani et al. compared three different models that did not consider mpMRI results, including Partin tables, the European Society of Urological Oncology (ESUO) criteria, and the Gallina nomogram, for the prediction of SVI (9). This study showed that all these three tools had high sensitivity (92.7%, 89%, and 90.8%, respectively) but poor specificity (33.1%, 56.3%, and 47.6%, respectively), which confirms that these models overestimated the probability of SVI during application. MpMRI has been considered as an important part of these models for providing detailed anatomical information. Grivas et al. discovered that the AUC values of Partin with MRI predictive models were higher than those of Partin and MRI alone (0.929, 0.837, and 0.884), which showed that adding mpMRI findings to Partin tables could improve its predictive accuracy (11). Martini et al. developed a mpMRI and clinical data-based nomogram for the prediction of SVI, and this nomogram showed a relatively high AUC (0.847) (12). However, these results are limited by the relatively small number of SVI cases with respect to the variables included in the model. Besides, these models containing mpMRI findings highly rely on the negative or positive SVI results of mpMRI. To our knowledge, none of the models currently integrate the PI-RADS v2 score to predict postoperative SVI in SVI (-) patients on preoperative mpMRI.
The PI-RADS scoring system has shown a great value in predicting biopsy outcome (22), biochemical recurrence (23), infiltration of the neurovascular bundles (24), and EPE (25). Our previous studies have shown similar results, confirming the value of PI-RADS in predicting prostate cancer and clinically significant prostate cancer in men undergoing repeat prostate biopsy and in predicting pelvic lymph node metastasis at RP (26,27). After adjusting for the other confounding factors, the GS and PI-RADS score were found to be independent risk factors for the prediction of SVI. On that basis, a model including PI-RADS to predict postoperative SVI in patients without SVI on mpMRI was constructed in this study. The AUC value of this model was 0.746 (P<0.001), which is better than that of mpMRI alone. This model also showed high sensitivity and negative predictive value, which indicates that it can precisely distinguish the real SVI (-) patients and help the urologists for the formulation of treatment plans.
Koh et al. found that among 275 patients with PSA ≤10 ng/mL and no cancer at the base in systematic biopsy results, none had SVI (28). Through creating probability plot graphs, Zlotta et al. pointed out that patients with PSA <10 ng/mL have a risk of SVI <5% when GS on biopsy is <7 or when the percentage of biopsies affected by cancer is <50% (29). In our series, we also observed that among the 111 patients with PI-RADS v2 score <4 and Gleason grade <8, extremely few patients (n=2) had SVI, which yielded only a 1.8% incidence of SVI with a high negative predictive value of 98.2% (95% CI, 93.0–99.6%).
PSA, a widely used serum marker for prostate cancer screening, plays an important role in other predictive models for SVI. In our study, the PSA level in SVI (+) patients was higher than that in SVI (-) patients, but without any significant difference (Median: 7.75 vs. 7.44 ng/mL, P=0.114). This is most probably because we selected patients with PSA <10 ng/mL, which narrowed the difference between the two groups. PSAD and f/t PSA did not show a significant difference as well as.
CAPRA score, which is calculated from the PSA level, the GS, the clinical T stage, the percentage of positive prostate biopsies and the patient age at diagnosis, has a great capacity in predicting prostate cancer outcomes, such as biochemical recurrence (30), metastatic potential (31), and prostate cancer-specific death (32). In our study, CAPRA wasn’t an independent risk predictor for the diagnosis of SVI (P=0.070) after adjusting other confounding factors. And further research is required for the value of CAPRA score for predicting SVI.
Emerging technologies and prostate cancer biomarkers are playing a vital role in prostate cancer diagnosis and treatment (33,34). Micro-ultrasound is a novel high-resolution imaging technology for diagnosing prostate cancer which is complementary for mpMRI (35-38). Compare to mpMRI, micro-ultrasound, which promises real-time visualization of suspicious lesions and targeting of biopsies, has shown same or superior sensitivity (37). For detecting clinically significant prostate cancer, micro-ultrasound biopsy has shown a higher rate with fewer biopsied cores (39), and could found prostate cancer missed by all other techniques (34). However, additional studies are needed to explore the application of micro-ultrasound for prostate cancer staging and predicting SVI.
Several limitations should be considered. First, these results were obtained from a retrospective cohort, thus bringing a certain risk of selection bias. Second, the predictive model was constructed on the basis on a small sample size from a single institution, and the accuracy of this model requires internal and external validation in a multicenter study to assess its wider applicability. Third, due to improvement in data quality over time and different MRI protocols performed in patients, there might be a certain difference in the outcome of MRI. Finally, the MRI performed previously at our institution could not meet the PI-RADS v2.1 technical stander (40), which would aggravate transferability for clinical usage, and the role of the PI-RADS v2.1 score for predicting SVI need further investigation. However, PI-RADS v2 has showed satisfactory inter-reader variability in previous study (41).
Conclusions
In the present study, PI-RADS assessment has been proved to be one of the valuable predictors of SVI in SVI (-) patients on mpMRI. The PI-RADS v2 score <4 in prostate cancer patients with PSA <10 ng/mL is associated with a very low risk of SVI. A model based on biopsy Gleason grade and PI-RADS v2 score may help to predict SVI and serve as a tool for the urologists to make surgical plans.
Acknowledgments
The authors thank the entire staff of the Department of Urology, Peking University First Hospital.
Funding: This work was supported by Peking University Medicine Seed Fund for Interdisciplinary Research (BMU2020MI003).
Footnote
Reporting Checklist: The authors have completed the TRIPOD reporting checklist. Available at http://dx.doi.org/10.21037/tau-20-989
Peer Review File: Available at http://dx.doi.org/10.21037/tau-20-989
Data Sharing Statement: Available at http://dx.doi.org/10.21037/tau-20-989
Conflicts of Interest: All authors have completed the ICMJE uniform disclosure form (available at http://dx.doi.org/10.21037/tau-20-989). GS serves as an unpaid editorial board member of Translational Andrology and Urology from Jun 2019 to May 2021. The other authors have no conflicts of interest to declare.
Ethical Statement: The authors are accountable for all aspects of the work in ensuring that questions related to the accuracy or integrity of any part of the work are appropriately investigated and resolved. All procedures performed in this study were in accordance with the Declaration of Helsinki (as revised in 2013). Due to no influence on therapeutic strategy or need for patients’ follow-up, the Institutional Review Board replied that there was no need for ethical approval. And because of the retrospective nature of the research, the requirement for informed consent was waived.
Open Access Statement: This is an Open Access article distributed in accordance with the Creative Commons Attribution-NonCommercial-NoDerivs 4.0 International License (CC BY-NC-ND 4.0), which permits the non-commercial replication and distribution of the article with the strict proviso that no changes or edits are made and the original work is properly cited (including links to both the formal publication through the relevant DOI and the license). See: https://creativecommons.org/licenses/by-nc-nd/4.0/.
References
- Eggener SE, Roehl KA, Smith ND, et al. Contemporary survival results and the role of radiation therapy in patients with node negtive seminal vesicle invasion following radical prostatectomy. J Urol 2005;173:1150-5. [Crossref] [PubMed]
- Pierorazio PM, Ross AE, Schaeffer EM, et al. A contemporary analysis of outcomes of adenocarcinoma of the prostate with seminal vesicle invasion (pT3b) after radical prostatectomy. J Urol 2011;185:1691-7. [Crossref] [PubMed]
- Gandaglia G, Ploussard G, Valerio M, et al. The Key Combined Value of Multiparametric Magnetic Resonance Imaging, and Magnetic Resonance Imaging–targeted and Concomitant Systematic Biopsies for the Prediction of Adverse Pathological Features in Prostate Cancer Patients Undergoing Radical Prostatectomy. Eur Urol 2020;77:733-41. [Crossref] [PubMed]
- Jambor I, Falagario U, Ratnani P, et al. Prediction of biochemical recurrence in prostate cancer patients who underwent prostatectomy using routine clinical prostate multiparametric MRI and decipher genomic score. J Magn Reson Imaging 2020;51:1075-85. [Crossref] [PubMed]
- Waingankar N, Martini A, Griffiths L, et al. Weighted Gleason Grade Group (WGGG): A new prostate cancer biopsy reporting system with prognostic potential. Urol Oncol 2020;38:78.e15-78.e21. [Crossref] [PubMed]
- Kristiansen A, Drevin L, Delahunt B, et al. Prognostic significance and biopsy characteristics of prostate cancer with seminal vesicle invasion on radical prostatectomy: a nationwide population-based study. Pathology 2017;49:715-20. [Crossref] [PubMed]
- Eifler JB, Feng Z, Lin BM, et al. An updated prostate cancer staging nomogram (Partin tables) based on cases from 2006 to 2011. BJU Int 2013;111:22-9. [Crossref] [PubMed]
- Gallina A, Chun FKH, Briganti A, et al. Development and Split-Sample Validation of a Nomogram Predicting the Probability of Seminal Vesicle Invasion at Radical Prostatectomy. Eur Urol 2007;52:98-105. [Crossref] [PubMed]
- Lughezzani G, Zorn KC, Budäus L, et al. Comparison of Three Different Tools for Prediction of Seminal Vesicle Invasion at Radical Prostatectomy. Eur Urol 2012;62:590-6. [Crossref] [PubMed]
- de Rooij M, Hamoen EHJ, Witjes JA, et al. Accuracy of Magnetic Resonance Imaging for Local Staging of Prostate Cancer: A Diagnostic Meta-analysis. Eur Urol 2016;70:233-45. [Crossref] [PubMed]
- Grivas N, Hinnen K, de Jong J, et al. Seminal vesicle invasion on multi-parametric magnetic resonance imaging: Correlation with histopathology. Eur J Radiol 2018;98:107-12. [Crossref] [PubMed]
- Martini A, Gupta A, Cumarasamy S, et al. Novel nomogram for the prediction of seminal vesicle invasion including multiparametric magnetic resonance imaging. Int J Urol 2019;26:458-64. [Crossref] [PubMed]
- Mathur S, O'Malley ME, Ghai S, et al. Correlation of 3T multiparametric prostate MRI using prostate imaging reporting and data system (PIRADS) version 2 with biopsy as reference standard. Abdom Radiol (NY) 2019;44:252-8. [Crossref] [PubMed]
- Weinreb JC, Barentsz JO, Choyke PL, et al. PI-RADS Prostate Imaging - Reporting and Data System: 2015, Version 2. Eur Urol 2016;69:16-40. [Crossref] [PubMed]
- Steinkohl F, Gruber L, Bektic J, et al. Retrospective analysis of the development of PIRADS 3 lesions over time: when is a follow-up MRI reasonable? World J Urol 2018;36:367-73. [Crossref] [PubMed]
- Barentsz JO, Weinreb JC, Verma S, et al. Synopsis of the PI-RADS v2 Guidelines for Multiparametric Prostate Magnetic Resonance Imaging and Recommendations for Use. Eur Urol 2016;69:41-9. [Crossref] [PubMed]
- Algarra R, Barba J, Merino I, et al. Prognostic value of seminal vesicle involvement due to prostate cancer in radical prostatectomy specimens. Actas Urol Esp 2015;39:144-53. [Crossref] [PubMed]
- Mottet N, Bellmunt J, Bolla M, et al. EAU-ESTRO-SIOG Guidelines on Prostate Cancer. Part 1: Screening, Diagnosis, and Local Treatment with Curative Intent. Eur Urol 2017;71:618-29. [Crossref] [PubMed]
- Billis A, Teixeira DA, Stelini RF, et al. Seminal vesicle invasion in radical prostatectomies: which is the most common route of invasion? Int Urol Nephrol 2007;39:1097-102. [Crossref] [PubMed]
- Epstein JI, Partin AW, Potter SR, et al. Adenocarcinoma of the prostate invading the seminal vesicle: prognostic stratification based on pathologic parameters. Urology 2000;56:283-8. [Crossref] [PubMed]
- Pignot G, Maillet D, Gross E, et al. Systemic treatments for high-risk localized prostate cancer. Nat Rev Urol 2018;15:498-510. [Crossref] [PubMed]
- Washino S, Okochi T, Saito K, et al. Combination of prostate imaging reporting and data system (PI-RADS) score and prostate-specific antigen (PSA) density predicts biopsy outcome in prostate biopsy naive patients. BJU Int 2017;119:225-33. [Crossref] [PubMed]
- Park SY, Oh YT, Jung DC, et al. Prediction of biochemical recurrence after radical prostatectomy with PI-RADS version 2 in prostate cancers: initial results. Eur Radiol 2016;26:2502-9. [Crossref] [PubMed]
- Sauer M, Weinrich JM, Fraune C, et al. Accuracy of multiparametric MR imaging with PI-RADS V2 assessment in detecting infiltration of the neurovascular bundles prior to prostatectomy. Eur J Radiol 2018;98:187-92. [Crossref] [PubMed]
- Matsuoka Y, Ishioka J, Tanaka H, et al. Impact of the Prostate Imaging Reporting and Data System, Version 2, on MRI Diagnosis for Extracapsular Extension of Prostate Cancer. AJR Am J Roentgenol 2017;209:W76-W84. [Crossref] [PubMed]
- Huang C, Song G, Wang H, et al. MultiParametric Magnetic Resonance Imaging-Based Nomogram for Predicting Prostate Cancer and Clinically Significant Prostate Cancer in Men Undergoing Repeat Prostate Biopsy. Biomed Res Int 2018;2018:6368309. [Crossref] [PubMed]
- Huang C, Song G, Wang H, et al. Preoperative PI-RADS Version 2 scores helps improve accuracy of clinical nomograms for predicting pelvic lymph node metastasis at radical prostatectomy. Prostate Cancer Prostatic Dis 2020;23:116-26. [Crossref] [PubMed]
- Koh H, Kattan MW, Scardino PT, et al. A Nomogram to Predict Seminal Vesicle Invasion by the Extent and Location of Cancer in Systematic Biopsy Results. J Urol 2003;170:1203-8. [Crossref] [PubMed]
- Zlotta AR, Roumeguère T, Ravery V, et al. Is Seminal Vesicle Ablation Mandatory for All Patients Undergoing Radical Prostatectomy?: A Multivariate Analysis on 1283 Patients. Eur Urol 2004;46:42-9. [Crossref] [PubMed]
- Cooperberg MR, Pasta DJ, Elkin EP, et al. The University of California, San Francisco Cancer of the Prostate Risk Assessment score: a straightforward and reliable preoperative predictor of disease recurrence after radical prostatectomy. J Urol 2005;173:1938-42. [Crossref] [PubMed]
- Huang QX, Xiao CT, Chen Z, et al. Combined analysis of CRMP4 methylation levels and CAPRA-S score predicts metastasis and outcomes in prostate cancer patients. Asian J Androl 2018;20:56-61. [Crossref] [PubMed]
- Zelic R, Garmo H, Zugna D, et al. Predicting Prostate Cancer Death with Different Pretreatment Risk Stratification Tools: A Head-to-head Comparison in a Nationwide Cohort Study. Eur Urol 2020;77:180-8. [Crossref] [PubMed]
- Kohaar I, Petrovics G, Srivastava S. A Rich Array of Prostate Cancer Molecular Biomarkers: Opportunities and Challenges. Int J Mol Sci 2019;20:1813. [Crossref] [PubMed]
- Rodríguez Socarrás ME, Gomez Rivas J, Cuadros Rivera V, et al. Prostate Mapping for Cancer Diagnosis: The Madrid Protocol. Transperineal Prostate Biopsies Using Multiparametric Magnetic Resonance Imaging Fusion and Micro-Ultrasound Guided Biopsies. J Urol 2020;204:726-33. [Crossref] [PubMed]
- Ghai S, Eure G, Fradet V, et al. Assessing Cancer Risk on Novel 29 MHz Micro-Ultrasound Images of the Prostate: Creation of the Micro-Ultrasound Protocol for Prostate Risk Identification. J Urol 2016;196:562-9. [Crossref] [PubMed]
- Zhang M, Wang R, Wu Y, et al. Micro-Ultrasound Imaging for Accuracy of Diagnosis in Clinically Significant Prostate Cancer: A Meta-Analysis. Front Oncol 2019;9:1368. [Crossref] [PubMed]
- Laurence Klotz CM. Can high resolution micro-ultrasound replace MRI in the diagnosis of prostate cancer? Eur Urol Focus 2020;6:419-23. [Crossref] [PubMed]
- Wiemer L, Hollenbach M, Heckmann R, et al. Evolution of Targeted Prostate Biopsy by Adding Micro-Ultrasound to the Magnetic Resonance Imaging Pathway. Eur Urol Focus 2020. [Crossref] [PubMed]
- Claros OR, Tourinho-Barbosa RR, Fregeville A, et al. Comparison of Initial Experience with Transrectal Magnetic Resonance Imaging Cognitive Guided Micro-Ultrasound Biopsies versus Established Transperineal Robotic Ultrasound Magnetic Resonance Imaging Fusion Biopsies for Prostate Cancer. J Urol 2020;203:918-25. [Crossref] [PubMed]
- Turkbey B, Rosenkrantz AB, Haider MA, et al. Prostate Imaging Reporting and Data System Version 2.1: 2019 Update of Prostate Imaging Reporting and Data System Version 2. Eur Urol 2019;76:340-51. [Crossref] [PubMed]
- Rosenkrantz AB, Ginocchio LA, Cornfeld D, et al. Interobserver Reproducibility of the PI-RADS Version 2 Lexicon: A Multicenter Study of Six Experienced Prostate Radiologists. Radiology 2016;280:793-804. [Crossref] [PubMed]