Identification of a novel immune-related microRNA prognostic model in clear cell renal cell carcinoma
Introduction
Kidney cancer is a highly complex disease that may involve different types of kidney tumors, with renal cell carcinoma (RCC) being the most abundant amongst these. There are three types of kidney cancer: clear cell RCC (ccRCC), kidney renal papillary cell carcinoma (KIRP) or papillary RCC (pRCC), and kidney chromophobe (KICH) or chromophobe RCC (chRCC) (1). ccRCC is the most common type of kidney cancer, and is associated with the worst overall survival (OS) rate and high morbidity (2). The relationship between genetic factor alterations and the occurrence and development of ccRCC is not very clear, and thus the treatment options for ccRCC are relatively limited (3,4). Therefore, it is important to understand how certain prognostic factors affect the diagnosis and treatment of ccRCC patients.
MicroRNA (miRNA) is a single-stranded molecule of approximately 22 nucleotides, which are an important part of non-coding RNAs. miRNAs perform a vital biological process in inhibiting messenger RNA (mRNA) translation and expression by binding 3' untranslated regions (UTR) or 5' UTR (5). A great many miRNAs have been discovered by the latest human genome-sequencing technology, and an abundance of evidence suggests that miRNA controls the processes involved in the initiation and development of tumors, including cell proliferation, differentiation, growth, apoptosis, and aging, by regulating oncogenes or tumor suppressor genes (6). A number of studies have reported several miRNAs to be abnormally expressed in KIRC patients and capable of performing many important biological functions. Chen et al. distinguished between normal kidney tissues and ccRCC tissues by constructing a prognostic model of 11 deregulated miRNAs (7). Heinzelmann et al. suggested that specific miRNAs can distinguish between metastatic and non-metastatic ccRCC (8), while Wu et al. showed that a 4-miRNA-expression signature could determine the metastasis status of ccRCC patients (9). Some non-coding RNAs such as lncRNA and circle RNA play an important role in the development of ccRCC. For example, LINC02747 promotes the proliferation of ccRCC by inhibiting miR-608 and activating TFE3 (10). Wang et al. found that hsa_circ_0001451 was significantly down-regulated in RCC tissues and closely related to clinicopathological features and OS (11).
The immune system plays a vital role in the pathogenesis of tumors (12), including KIRC. Furthermore, a great many of immune-related miRNAs have been identified as being associated with KIRC. Some studies have shown that certain miRNAs act as key regulators of the immune response in different tumors. In particular, recent studies have showed that miRNA-mediated mechanisms regulate the activation of special immune cells in the tumor microenvironment. In addition, several miRNAs have been revealed to affect important cancer-related immune pathways that mediate immune cells to secrete immunosuppressive or stimulatory factors (13). Qu et al. identified miR-497-5p to be a potential therapeutic target and biomarker of ccRCC, and elucidated a novel regulatory mechanism of programmed death-ligand 1 (PD-L1) expression (14). Gigante et al. reported that miR-29b and miR-198 are abnormally expressed in T cells of RCC patients, indicating that targeting these miRNAs is beneficial to the diagnosis and treatment of RCC patients (15). Meanwhile, Jasinski-Bergner et al. reported that overexpression of miR-548q and miR-628-5p could cause a downregulation of human leukocyte antigen (HLA-G) and enhance the natural killer (NK) cell-mediated HLA-G-dependent cytotoxicity, implying that the regulation of miRNAs can control the activity of immune cells. Therefore, miR-548q and miR-628-5p may be future therapeutic targets for RCC patients (16). Increasing evidence has shown that abnormal immuno-miRs are closely related to the occurrence and progression of ccRCC. There are some miRNA targeted therapy for ccRCC, for example, miR-144-3p and miR-21 expression can affect drug sensitivity of metformin, sunitinib and sorafenib. These drugs are common drugs used to treat RCC. Thus, miR-21 may be a potential therapeutic target. These data demonstrate that miR-21 may be the diagnosis, treatment, and prognosis biomarker candidate of ccRCC. Metformin can induce miR-21 expression (17). Gaudelot et al’s study showed that miR-21-silencing can increase drug sensitivity and decrease drug resistance in ccRCC (18). However, few, if any, definitive models currently exist that can systematically predict the tumor immune microenvironment and the overall prognosis of ccRCC patients based on immuno-miRs. Therefore, establishing a reliable and stable prognostic model has important clinical and therapeutic significance.
In this study, we thus obtained differentially expressed immune-related miRNAs (DEIMs) closely related to ccRCC through the “limma” package of The Cancer Genome Atlas (TCGA) and the Immune-miR database. Then, we further detected those immune-related miRNAs that were significantly related to prognosis. At last, we established an immune-related prognostic model by integrating immune-related miRNAs for ccRCC. We aimed to provide novel biomarkers that could effectively predict the prognosis of ccRCC patients.
Finally, we constructed a novel 9-immune-related-miRNA signature model based on TCGA database. The model can effectively predict the OS of ccRCC patients. Gene Ontology (GO) and Kyoto Encyclopedia of Genes and Genomes (KEGG) enrichment analysis revealed the biological function of miRNA-targeted genes and identified the significant signaling pathways related to ccRCC, which deepened our understanding of the pathogenesis of kidney cancer.
We present the following article in accordance with the TRIPOD reporting checklist (available at http://dx.doi.org/10.21037/tau-20-1495).
Methods
TCGA dataset collection
Level 3 miRNAs from 521 disease samples and 71 normal samples, mRNAs from 535 disease samples and 72 normal samples, and other information including clinical samples were downloaded from TCGA database (https://cancergenome.nih.gov/) (19). The detailed information of miRNA target genes was obtained from MiRTarBase (20). We acquired 245 immune-related miRNAs in online tools that contained specific miRNA-disease associations in the Immunology Database and Analysis Portal (Immune-miR; http://www.biominingbu.org/immunemir/index.html) database (21). The study was conducted in accordance with the Declaration of Helsinki (as revised in 2013).
Identification of differentially expressed miRNAs (DEMs) and mRNA in ccRCC
We obtained miRNA and mRNA expression data of ccRCC samples from TCGA dataset. We then used the R package “limma” to screen DEMs and mRNAs (DEmRNAs) between tumor samples and normal samples (22). DEMs and DEmRNAs were considered using log2 |fold change (FC)| >1 and adjusted P value <0.01 as the screening criteria.
The construction of the prognostic immune-related miRNA signature
First, we used the R package “caret” to randomly divide the sample into two categories (training set and testing set). We applied univariate analysis to select the independent risk miNRAs in the training samples. In addition, we used the multivariate Cox regression method (survival package) to identify corresponding coefficients of the ccRCC prognostic signature (23). The risk score of every tumor sample from TCGA cohorts was derived from the immune-related miRNA signature. All tumor samples were randomly divided into high- and low-risk groups with the median of the risk score. We used a Kaplan-Meier curve and log-rank test to evaluate survival analysis in the training, testing and whole set. The predictive performance of the immune-related miRNA signature was evaluated by the area under the curve (AUC) of 1-, 3-, and 5-year overall survival dependent on the receiver operating characteristic (ROC) curve according to the R software package, “survivalROC” (24).
Functional enrichment analysis of miRNA target genes
The relationship between miRNAs and target genes was obtained from miRTarBase (http://mirtarbase.mbc.nctu.edu.tw/). We applied the intersection between the differentially expressed genes (DEGs) and the miRNA target genes to clarify the molecular mechanism of the important genes in kidney cancer. Finally, we used the R package, “clusterProfiler”, (25) for functional enrichment analysis based on the intersection genes.
Statistical analysis
All statistical analysis were performed on R software (version 3.6.1). DEMs and mRNAs (DEmRNAs) were obtained by R package “limma”. Training set and testing set of tumor samples were divided by R package “caret”. Univariate Cox regression analysis were used to assess the relationship between miRNA and overall survival. The 9-miNRA model was established based on multivariate Cox proportional hazards regression model. Both the univariate and the multivariate Cox regression analysis were performed with the ‘survival’ package. The ROC curves and corresponding area under the curve (AUC) were drawn by the package of ‘survivalROC’ in R.
Results
Identification of differentially expressed immune-related miRNAs and mRNAs in ccRCC
We selected 59 DEIMs (|log FC| >1, P<0.01 after P value adjustment) between ccRCC tissues and normal tissues. Among the miNRAs, 26 miRNAs were upregulated and 33 were downregulated (Figure 1A). The cluster heatmap of 59 DEIMs is displayed in Figure 1B. In addition, there were a total of 6,204 DEGs, including 2,634 upregulated and 3,579 downregulated genes based on 535 tumor samples and 72 normal samples.
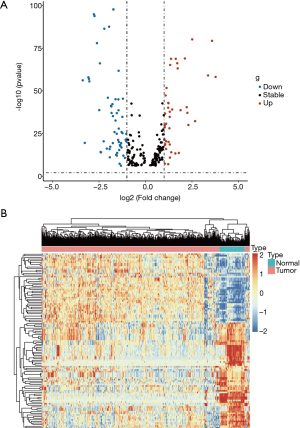
Screening of immune-related miRNAs with the prognostic value in ccRCC
All tumor samples were randomly divided (N=521) based on miRNA expression profiles into training samples (N=261) and testing samples (N=260). To identify DEIMs with prognostic characteristics, the expression of 59 miRNAs in the training group was evaluated by univariate Cox analysis in. Ultimately, we found that 18 immune-related miRNAs were closely related to OS. The details of immune-related miRNAs are shown in Table 1.
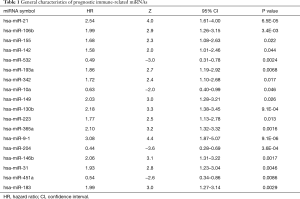
Full table
Construction of an immune-related prognostic model for ccRCC
We finally selected 9 miRNAs to construct a prognostic model in training samples based on the multivariate Cox analysis. The detailed formula of the model was as follows: risk score = (0.41 × expression level of hsa-miR-21) + (–0.06 × expression level of hsa-miR-342) + (0.02 × expression level of hsa-miR-149) + (0.47 × expression level of hsa-miR-130b) + (0.27 × expression level of hsa-miR-223) + (0.04 × expression level of hsa-miR-365a) + (–0.03 × expression level of hsa-miR-204) + (–0.12 × expression level of hsa-miR-14b) + (0.08 × expression level of hsa-miR-91). The risk scores were obtained by combining the expression level of miRNA with its corresponding regression coefficients. The AUC values of the ROC curve for 1-, 3-, and 5-year OS were respectively 0.714, 0.751, and 0.72 in the training set, 0.715, 0.667, and 0.680 in the testing set, and 0.715, 0.707, and 0.697 in the whole set. The results suggested that this prognostic model based on immune-related miRNAs demonstrated a good sensitivity and specificity (Figure 2A,B,C). We applied multivariate Cox regression to obtain regression coefficients. The risk scores were related to miRNA expression and regression coefficients. Based on the median risk score, we divided the KIRC patients into a high-risk group and low-risk group. Survival analysis revealed that the survival rate of patients in the high-risk group was lower than that in the low-risk group in each of the training, testing, and whole sets (P<0.0001; Figure 2D,E,F). In the training set, we applied a univariate and multivariate Cox regression model to characterize miRNAs associated with OS and tumorigenesis (Table 1). The Kaplan-Meier method identified hsa-miR-21, hsa-miR-342, hsa-miR-149, hsa-miR-130b, hsa-miR-223, hsa-miR-365a, hsa-miR-9-1, hsa-miR-204, and hsa-miR-146b as the 9 miRNAs that were closely related to patients’ OS (P value <0.05; Figure 3). Among these 9 miRNAs, 8 miRNAs [hsa-miR-21, hsa-miR-342, hsa-miR-149, hsa-miR-130b, hsa-miR-223, hsa-miR-365a, hsa-miR-9-1, and hsa-miR-146b; hazard ratio (HR) >1] were negatively associated with survival and 1 miRNA (hsa-miR-204, HR <1) was positively associated. This indicated that these 8 miRNAs had high-risk characteristics, because their high expression levels showed that patients had a shorter OS, but low expression levels of 1 miRNA indicated a shorter patient OS (Figure 3).
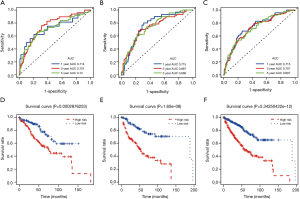
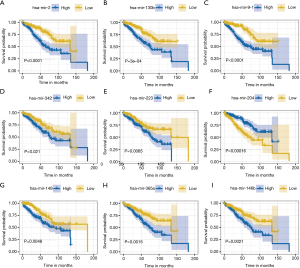
Independence between the immune-related prognostic signature and other clinical factors
The clinical information of ccRCC patients including gender, age, clinical stage and TNM stage was analyzed in depth in order to evaluate the independent predictive ability of the immune-related miRNA model based on univariate and multivariate Cox regression analyses. Univariate analysis showed that clinical stage (P<0.001), TNM classification (P<0.001), and the immune-related miRNA model (P<0.001) were significantly related to OS (Table 2).
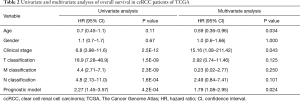
Full table
Functional enrichment analysis of miRNA-target genes associated with ccRCC
A total of 9 miRNA-target genes were validated by the miRTarBase dataset. According to the miRTarBase, there were 3,878 target genes for the 9 miRNAs. In addition, a total of 6,204 DEGs were obtained. There were 830 intersection genes between the 3,878 DEGs and the 6,204 validated target genes of the 9 miRNAs (Figure 4A). The molecular function (MF, Figure 4B), cellular component (CC, Figure 4C), and biological process in GO analysis (BP, Figure 4D) were depicted i n “dotplot” from “clusterProfile” packages. BP analysis mainly enriched kidney development, renal system development, and kidney epithelium development. CC functional analysis mainly included collagen-containing extracellular matrix, external side of plasma membrane, and cell-cell junction. MF analysis mainly contained coenzyme binding, growth factor binding, and virus receptor activity. The results of KEGG enrichment analysis showed that it identified about 830 intersection genes associated with ccRCC, of which counts >10 were mainly enriched in Epstein-Barr virus infection, cell adhesion molecules (CAMs), Hippo signaling pathway, AGE–RAGE signaling pathway in diabetic complications, antigen processing and presentation pathway, and intestinal immune network for immunoglobin A (IgA) production (P<0.01 after P value adjustment; Figure 4E). Additionally, in order to provide a detailed description of the complex relationship between KEGG pathway and target genes, the “pathway-gene” network was constructed, as shown in Figure 4F.
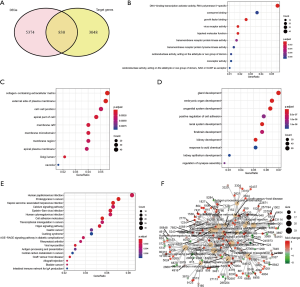
Discussion
KIRC is a highly malignant solid tumor that easily metastasizes to the rectum or lungs, and thus can seriously impinge on the survival of patients. Consequently, it has become increasing important to find novel prognostic markers that can aid in the treatment of patients. Therefore, understanding the pathogenesis of ccRCC is essential for the diagnosis and treatment of ccRCC. Assuming miRNA is the stabilizers of human tissues, miRNAs are considered to be novel biomarkers (26,27). Recently, a plethora of studies have indicated that specific miRNAs can play critical roles in the development of ccRCC (28-31). In this study, we systematically analyzed a miRNA dataset from TCGA database and screened DEMs using the “limma” package. We then identified those immune-related DEMs that are closely related to prognosis by using univariate and multivariate Cox regression analyses. Finally, a total of 59 immune-related DEMs were identified between 521 tumor samples and 71 normal samples. Some studies have shown that models based on multiple miRNAs have more stable performance than single miRNA models. Therefore, we established a 9-immune-related-miRNA signature (miR-21, miR-342, miR-149, miR-130b, miR-223, miR-365a, miR-9-1, miR-204, and miR-146b) that possessed a strong predictive diagnostic ability and prognostic significance for patients.
Several studies have shown that certain miRNAs are abnormally expressed during the occurrence and development of RCC (32-34). However, owing to the molecular heterogeneity of cancer, the reproducibility of the methods for identification of these miRNAs has limitations (35). In addition, the number of patient volunteers studied in real life is relatively small. TCGA database can provide gene expression data of solid tumor tissue and normal tissue. Making full use of these expression data is conducive to forming a better understanding of the underlying biological mechanism, which is necessary for improving the diagnosis of ccRCC and for creating a prognostic signature (36).
To identify an effective biomarker for cancer diagnosis and prognosis, we constructed a 9-immune-related miRNA prognostic signature from the ccRCC dataset. The 9-miRNA signature was an independent prognostic factor of ccRCC, with the survival rate of patients in the high-risk group being significantly lower than that of the low-risk group (Figure 2D,E,F). Out of the 9 miRNAs, miR-21 was regarded as an oncogene. Ample research indicates that miR-21 can promote cancer development by inhibiting tumor suppressor genes (32), and recently, Liang et al. reported that miRNA-21 could promote cell proliferation and differentiation, and prevent apoptosis by activating mTOR-STAT3 signaling pathway (37). Additionally, miR-21 was also found to be closely related to various tumors, including those of the breast (38), colon (39), bladder (40), liver (41), and kidney (42). Okato et al. revealed that the ectopic expression of miR-149 significantly inhibited cancer cell migration and invasion in ccRCC cells (43); meanwhile, Li et al. demonstrated that the downregulation of miR-130b inhibited cell migration, cell proliferation, and induced cell apoptosis of RCC, suggesting that miR-130b may be an effective biomarker for diagnosis and a therapeutic target for the treatment of RCC (44). Additionally, Xiao et al. discovered that the overexpression of miR-223-3p could enhance cell proliferation and metastasis in renal cancer cells (45), and Hildebrandt et al. showed that miR-9-1 may not only be involved in the occurrence and development of ccRCC but may also participate in the metastatic recurrence of KIRC (46). Moreover, Xiong et al. revealed that miR-204 can directly regulate the target gene, RAB22A, in inhibiting RCC proliferation and invasion (47). Finally, Yang’s findings suggested that HOXA11-AS could promote RCC growth and invasion by modulating the miR-146b-5p-MMP16 axis (48). Although miR-342 and miR-365a have not been reported in other studies, our results indicated that they showed significant differential expression in ccRCC samples, which suggests that these miRNAs may serve as significant biomarkers and therapeutic targets for treating the occurrence and development of ccRCC.
Although this study developed 9-miRNA immune-related prognostic model in ccRCC based on miRNA expression profiles in TCGA, there were still some limitations. First, the difference of the races, gender, age of ccRCC patient, tumor stages would lead to heterogeneity. Second, although our results were validated in testing set in TCGA dataset, the results were not verified by experiments such qPT-PCR in vitro. Further evidences are required to confirm our results by experiments on a great number of samples and clinical patients.
Conclusions
This study demonstrated that our 9-immune-related-miRNA signature could be a practical and reliable prognostic model for ccRCC. Furthermore, through evaluation using a testing set and a whole set of ccRCC patients, we showed that this prognostic model has the potential to be a valuable biomarker in ccRCC.
Acknowledgments
The authors would like to thank TCGA for sharing their ccRCC data.
Funding: This study was supported by funding from the Science and Technology Planning Project of Guangdong Province (no. 2015B020226002) and The Clinical Medicine Research Foundation of Guangdong Province (no. 2020ZJ03).
Footnote
Reporting Checklist: The authors have completed the TRIPOD reporting checklist. Available at http://dx.doi.org/10.21037/tau-20-1495
Conflicts of Interest: All authors have completed the ICMJE uniform disclosure form (available at http://dx.doi.org/10.21037/tau-20-1495). The authors have no conflicts of interest to declare.
Ethical Statement: The authors are accountable for all aspects of the work in ensuring that questions related to the accuracy or integrity of any part of the work are appropriately investigated and resolved. The study was conducted in accordance with the Declaration of Helsinki (as revised in 2013).
Open Access Statement: This is an Open Access article distributed in accordance with the Creative Commons Attribution-NonCommercial-NoDerivs 4.0 International License (CC BY-NC-ND 4.0), which permits the non-commercial replication and distribution of the article with the strict proviso that no changes or edits are made and the original work is properly cited (including links to both the formal publication through the relevant DOI and the license). See: https://creativecommons.org/licenses/by-nc-nd/4.0/.
References
- Shuch B, Amin A, Armstrong AJ, et al. Understanding pathologic variants of renal cell carcinoma: distilling therapeutic opportunities from biologic complexity. Eur Urol 2015;67:85-97. [Crossref] [PubMed]
- Gray RE, Harris GT. Renal Cell Carcinoma: Diagnosis and Management. Am Fam Physician 2019;99:179-84. [PubMed]
- Schmidt LS, Linehan WM. Genetic predisposition to kidney cancer. Semin Oncol 2016;43:566-74. [Crossref] [PubMed]
- Liao L, Testa JR, Yang H. The roles of chromatin-remodelers and epigenetic modifiers in kidney cancer. Cancer Genet 2015;208:206-14. [Crossref] [PubMed]
- Meister G, Tuschl T. Mechanisms of gene silencing by double-stranded RNA. Nature 2004;431:343-9. [Crossref] [PubMed]
- Bartel DP. MicroRNAs: genomics, biogenesis, mechanism, and function. Cell 2004;116:281-97. [Crossref] [PubMed]
- Chen J, Zhang D, Zhang W, et al. Clear cell renal cell carcinoma associated microRNA expression signatures identified by an integrated bioinformatics analysis. J Transl Med 2013;11:169. [Crossref] [PubMed]
- Heinzelmann J, Henning B, Sanjmyatav J, et al. Specific miRNA signatures are associated with metastasis and poor prognosis in clear cell renal cell carcinoma. World J Urol 2011;29:367-73. [Crossref] [PubMed]
- Wu X, Weng L, Li X, et al. Identification of a 4-microRNA signature for clear cell renal cell carcinoma metastasis and prognosis. PLoS One 2012;7:e35661. [Crossref] [PubMed]
- Ju X, Sun Y, Zhang F, et al. Long Non-Coding RNA LINC02747 Promotes the Proliferation of Clear Cell Renal Cell Carcinoma by Inhibiting miR-608 and Activating TFE3. 2020;10.
- Wang G, Xue W, Jian W, et al. The effect of Hsa_circ_0001451 in clear cell renal cell carcinoma cells and its relationship with clinicopathological features. J Cancer 2018;9:3269-77. [Crossref] [PubMed]
- Angell H, Galon J. From the immune contexture to the Immunoscore: the role of prognostic and predictive immune markers in cancer. Current opinion in immunology 2013;25:261-7. [Crossref] [PubMed]
- Paladini L, Fabris L, Bottai G, et al. Targeting microRNAs as key modulators of tumor immune response. J Exp Clin Cancer Res 2016;35:103. [Crossref] [PubMed]
- Qu F, Ye J, Pan X, et al. MicroRNA-497-5p down-regulation increases PD-L1 expression in clear cell renal cell carcinoma. J Drug Target 2019;27:67-74. [Crossref] [PubMed]
- Gigante M, Pontrelli P, Herr W, et al. miR-29b and miR-198 overexpression in CD8+ T cells of renal cell carcinoma patients down-modulates JAK3 and MCL-1 leading to immune dysfunction. J Transl Med 2016;14:84. [Crossref] [PubMed]
- Jasinski-Bergner S, Reches A, Stoehr C, et al. Identification of novel microRNAs regulating HLA-G expression and investigating their clinical relevance in renal cell carcinoma. Oncotarget 2016;7:26866-78. [Crossref] [PubMed]
- Kalogirou C, Schäfer D, Krebs M, et al. Metformin-Derived Growth Inhibition in Renal Cell Carcinoma Depends on miR-21-Mediated PTEN Expression. Urol Int 2016;96:106-15. [Crossref] [PubMed]
- Gaudelot K, Gibier JB, Pottier N, et al. Targeting miR-21 decreases expression of multi-drug resistant genes and promotes chemosensitivity of renal carcinoma. Tumour Biol 2017;39:1010428317707372. [Crossref] [PubMed]
- Cerami E, Gao J, Dogrusoz U, et al. The cBio cancer genomics portal: an open platform for exploring multidimensional cancer genomics data. Cancer Discov 2012;2:401-4. [Crossref] [PubMed]
- Huang HY, Lin YC, Li J, et al. miRTarBase 2020: updates to the experimentally validated microRNA-target interaction database. Nucleic Acids Res 2020;48:D148-d54. [PubMed]
- Prabahar A, Natarajan J., Immunemi R. A Database of Prioritized Immune miRNA Disease Associations and its Interactome. MicroRNA (Shariqah, United Arab Emirates) 2017;6:71-8. [Crossref] [PubMed]
- Ritchie ME, Phipson B, Wu D, et al. limma powers differential expression analyses for RNA-sequencing and microarray studies. Nucleic Acids Res 2015;43:e47. [Crossref] [PubMed]
- Stel VS, Dekker FW, Tripepi G, et al. Survival analysis II: Cox regression. Nephron Clin Pract 2011;119:c255-60. [Crossref] [PubMed]
- Heagerty PJ, Lumley T, Pepe MS. Time-dependent ROC curves for censored survival data and a diagnostic marker. Biometrics 2000;56:337-44. [Crossref] [PubMed]
- Yu G, Wang LG, Han Y, et al. clusterProfiler: an R package for comparing biological themes among gene clusters. Omics 2012;16:284-7. [Crossref] [PubMed]
- Mitchell PS, Parkin RK, Kroh EM, et al. Circulating microRNAs as stable blood-based markers for cancer detection. Proc Natl Acad Sci U S A 2008;105:10513-8. [Crossref] [PubMed]
- Sohn W, Kim J, Kang SH, et al. Serum exosomal microRNAs as novel biomarkers for hepatocellular carcinoma. Exp Mol Med 2015;47:e184. [Crossref] [PubMed]
- Shu X, Hildebrandt MA, Gu J, et al. MicroRNA profiling in clear cell renal cell carcinoma tissues potentially links tumorigenesis and recurrence with obesity. Br J Cancer 2017;116:77-84. [Crossref] [PubMed]
- Tusong H, Maolakuerban N, Guan J, et al. Functional analysis of serum microRNAs miR-21 and miR-106a in renal cell carcinoma. Cancer Biomark 2017;18:79-85. [Crossref] [PubMed]
- Zhu J, Ma X, Zhang Y, et al. Establishment of a miRNA-mRNA regulatory network in metastatic renal cell carcinoma and screening of potential therapeutic targets. Tumour Biol 2016. [Crossref] [PubMed]
- Trevisani F, Ghidini M, Larcher A, et al. MicroRNA 193b-3p as a predictive biomarker of chronic kidney disease in patients undergoing radical nephrectomy for renal cell carcinoma. Br J Cancer 2016;115:1343-50. [Crossref] [PubMed]
- Szabó Z, Szegedi K, Gombos K, et al. Expression of miRNA-21 and miRNA-221 in clear cell renal cell carcinoma (ccRCC) and their possible role in the development of ccRCC. Urol Oncol 2016;34:533.e21-.e27.
- Kurozumi A, Goto Y, Okato A, et al. Aberrantly expressed microRNAs in bladder cancer and renal cell carcinoma. J Hum Genet 2017;62:49-56. [Crossref] [PubMed]
- Liep J, Kilic E, Meyer HA, et al. Cooperative Effect of miR-141-3p and miR-145-5p in the Regulation of Targets in Clear Cell Renal Cell Carcinoma. PLoS One 2016;11:e0157801. [Crossref] [PubMed]
- Lánczky A, Nagy Á, Bottai G, et al. miRpower: a web-tool to validate survival-associated miRNAs utilizing expression data from 2178 breast cancer patients. Breast Cancer Res Treat 2016;160:439-46. [Crossref] [PubMed]
- Ramanathan R, Olex AL, Dozmorov M, et al. Angiopoietin pathway gene expression associated with poor breast cancer survival. Breast Cancer Res Treat 2017;162:191-8. [Crossref] [PubMed]
- Liang T, Hu XY, Li YH, et al. MicroRNA-21 Regulates the Proliferation, Differentiation, and Apoptosis of Human Renal Cell Carcinoma Cells by the mTOR-STAT3 Signaling Pathway. Oncol Res 2016;24:371-80. [Crossref] [PubMed]
- Kong W, He L, Coppola M, et al. MicroRNA-155 regulates cell survival, growth, and chemosensitivity by targeting FOXO3a in breast cancer. J Biol Chem 2016;291:22855. [Crossref] [PubMed]
- Velázquez KT, Enos RT, McClellan JL, et al. MicroRNA-155 deletion promotes tumorigenesis in the azoxymethane-dextran sulfate sodium model of colon cancer. Am J Physiol Gastrointest Liver Physiol 2016;310:G347-58. [Crossref] [PubMed]
- Wang H, Men CP. Correlation of Increased Expression of MicroRNA-155 in Bladder Cancer and Prognosis. Lab Med 2015;46:118-22. [Crossref] [PubMed]
- Han ZB, Chen HY, Fan JW, et al. Up-regulation of microRNA-155 promotes cancer cell invasion and predicts poor survival of hepatocellular carcinoma following liver transplantation. J Cancer Res Clin Oncol 2012;138:153-61. [Crossref] [PubMed]
- Li S, Chen T, Zhong Z, et al. microRNA-155 silencing inhibits proliferation and migration and induces apoptosis by upregulating BACH1 in renal cancer cells. Mol Med Rep 2012;5:949-54. [Crossref] [PubMed]
- Okato A, Arai T, Yamada Y, et al. Dual Strands of Pre-miR-149 Inhibit Cancer Cell Migration and Invasion through Targeting FOXM1 in Renal Cell Carcinoma. Int J Mol Sci 2017;18:1969. [Crossref] [PubMed]
- Li Y, Chen D, Li Y, et al. Identification of miR 130b as an oncogene in renal cell carcinoma. Mol Med Rep 2016;13:1902-8. [Crossref] [PubMed]
- Xiao W, Wang X, Wang T, et al. MiR-223-3p promotes cell proliferation and metastasis by downregulating SLC4A4 in clear cell renal cell carcinoma. Aging (Albany NY) 2019;11:615-33. [Crossref] [PubMed]
- Hildebrandt MA, Gu J, Lin J, et al. Hsa-miR-9 methylation status is associated with cancer development and metastatic recurrence in patients with clear cell renal cell carcinoma. Oncogene 2010;29:5724-8. [Crossref] [PubMed]
- Xiong F, Liu K, Zhang F, et al. MiR-204 inhibits the proliferation and invasion of renal cell carcinoma by inhibiting RAB22A expression. Oncol Rep 2016;35:3000-8. [Crossref] [PubMed]
- Yang FQ, Zhang JQ, Jin JJ, et al. HOXA11-AS promotes the growth and invasion of renal cancer by sponging miR-146b-5p to upregulate MMP16 expression. J Cell Physiol 2018;233:9611-9. [Crossref] [PubMed]
(English Language Editor: J. Gray)