Digital diagnostics and artificial intelligence in prostate cancer treatment in 5 years from now
The range of novelties included in this special series on “Update on molecular classification and individualized treatments of genitourinary tumors” published in the journal Translational Andrology and Urology (1-8) has led us to considerations on diagnosing and treating patients with genitourinary tumors in 5 years from now.
Digital pathology (DP)
This digital-based approach to histological diagnosis, also called DP, is a new approach in the morphologic analyses of glass slides either with tissue sections obtained from formalin-fixed paraffin embedded tissue (FFPE) or with frozen sections. Slide scanners are designed to obtain virtual slides of entire tissue sections, i.e., whole slide image (WSI), including large format histology, from large glass slides. The whole tissue section is represented in the virtual slide (Figure 1). The advantage of the virtual slides, compared with still images, is that a the user can change magnification to zoom in and out, while looking for diagnostic features as with an optical microscope (9,10). WSI technology has attracted the greatest attention in the pathology community. DP will become commonplace in five years from now.
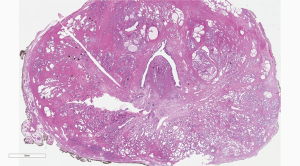
Artificial intelligence
AI is defined as “the ability of a machine to perform cognitive tasks to achieve a particular goal based on provided data” (11). The use of DP will allow the routine implementation of AI-based algorithms in the pathology (12,13). The most commonly used image-based AI algorithms are “convolutional neural networks” (14-16). These algorithms are based on WSI as learn and input associations and links between items such as a diagnosis made by a histopathologist, underlying molecular features and patients’ survival or response to adjuvant/neoadjuvant therapy, i.e., outcome measures (16). Such algorithms have the capability of going beyond the visual evaluation of morphologic features to identify tissue patterns that are not perceived by human recognition. This includes the tumor microenvironment. AI can combine together pieces of information derived from a host of features in order to make the overall final diagnosis. The features are then link to additional patients-related information in order to give data of potential value on the behavior of the disease, outcome of the patient and prediction of response to a certain therapeutic strategy (11).
Computational pathology and Gleason grading
Computer-based diagnosis of prostate cancer (PCa) on glass slides can be achieved by machine learning (ML) (Figure 2) to optimize reproducibility and accuracy as well as improve “health-care delivery by enabling the use of customized precision-care pathways” (11).

It is now feasible to grade PCa on virtual slides, thus improving reproducibility and accuracy by applying computer-based methods (17). Several investigators have dealt with PCa grading based on DP and AI (18). Lucas et al. (19) have attempted in a recent study to build an automatic classification of the Gleason pattern. This method can assist pathologists in the definition of the PCa Grade Groups (GG) in prostate biopsies. When distinguishing between Gleason patterns 4 or higher and Gleason pattern 3, accuracy was 90%, specificity and sensitivity were 94% and 77%, respectively. Concordance of their computer-based GG evaluation with the evaluation made by a pathologist was 65% (κ being 0.70). This indicates a substantial agreement.
AI can create algorithms that can allow a generalist to function as a specialist. Nagpal et al. (20) adopted “a supervised learning method to develop a deep learning system (DLS) for PCa grading” on radical prostatectomy specimens. Accuracy was assessed for the assignment of GG by generalists, in comparison to specialists. The DLS outperformed the generalist pathologists (accuracy of 0.70 vs. 0.64).
Digital microscopy of PCa with a fluorescence confocal microscope
Fluorescence confocal microscopy (FCM) is an optical imaging technique that provides digital microscopic images of fresh tissue in a real time fashion, without conventional processing (15). FCM has been widely applied in several fields including dermatopathology, for the detection of basal cell carcinoma and for the diagnosis of skin inflammatory diseases. In all studies, the acquisition of FCM images takes 2 to 5 minutes, without the need for conventional processing. There is no need for dedicated personnel and equipped laboratory (21). FCM digital images can be web-shared and interpreted by a remote pathologist in a real time, without the need for on-site personnel, i.e., a pathologist, other than the surgeon already involved in the surgical procedure. All studies showed the preservation of tissue integrity for further conventional tissue evaluation (Figure 3) as well as for immunohistochemistry after the initial FCM analysis.
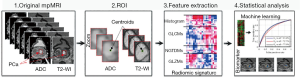
In a study from our group, detection of PCa with FCM was aligned with routine pathology reports in hematoxylin and eosin (HE) stained sections (95.1% of correct-diagnosis with FCM, κ being0.84). “Inter-rater agreement between pathologists was almost perfect for both HE and FCM for PCa detection (0.98 for HE, κ=0.95; 0.95 for FCM, κ=0.86); for cancer grade attribution only a moderate agreement was reached for both HE and FCM (HE κ=0.47; FCM κ=0.49)” (21).
PCa grade by radiomics
Radiomic analysis, “involving feature extraction from images with classifier techniques”, “can automatically predict the grade of cancer with a precision and speed beyond the scope of human visual analysis” (22).
Investigations have utilized radiomic features, obtained from magnetic resonance (MR) images, for computer-based diagnosis (23-25). In addition to basic diagnostic information, such analyses may detect subtle features and insights of the neoplasms, making additional evaluation into the “radiomic assessment of PCa as a priority” (Figure 4).
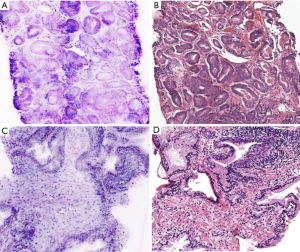
Radiomic features most important to predict the grade of PCa in order to estimate the aggressivity of the tumor under evaluation remain largely unexplored. Chaddad et al. (22) identified those radiomic features derived from both T2-WI and ADC images for the identification of three groups of Gleason scores (GS) and “classifying these groups using the RF classifier model”. Their evaluations found that three features, i.e., zone size non-uniformity, large zone size emphasis and zone size percentage, can discriminate groups of GS and correlate individual groups with aggressiveness Such items are of importance in predicting GS 6. The sum entropy is the most important to predict GS 4+3 (7). Radiomics, as a noninvasive test, has the potential, to predict the GS in men with PCa.
Conclusions: multi-criteria decision making and information fusion
Management of men with PCa is challenging due to the fact that there is biological “diversity between patients and histopathology alone cannot accurately predict PCa outcomes” (26).
In the last few years, utilization of genomic- based risk prediction models and molecular profiling has received a great interest. ML techniques are adopted in such evaluations in order to identify either the individual genes or groups of them for “which expression specificity to predict a certain clinical outcome is high” (27). Data can then be utilized to develop risk stratification and diagnostic tools, for producing targeted drug approaches. as well as for determining individualized treatment
ML algorithms are useful to improve treatment in patients with PCa by increasing the surgeon’s display with additional features including PCa location at the time of the robotic and other image-guided procedures. It can be used for the “autonomous manipulation of tools for assistance in the operating room” (11).
The process of merging data originating from multiple sources, including digital diagnostics, radiomics, diagnostic imaging and robotic surgery, “is defined as multi-criteria decision making and information fusion” (28). The resulting information, including the diagnostic and therapeutic decisions, when applied to men with PCa examined with large format histology and whole slide imaging (28), and with multiple biomarkers deriving from tissue, urine and blood samples, is far more accurate than when the various sources are evaluated separately and individually” (28). All this involves the utilization of AI.
Acknowledgments
Funding: None.
Footnote
Provenance and Peer Review: This article was commissioned by the editorial office, Translational Andrology and Urology for the series “Update on Molecular Classification and Individualized Treatments of Genitourinary Tumors”. The article did not undergo external peer review.
Conflicts of Interest: All authors have completed the ICMJE uniform disclosure form (available at http://dx.doi.org/10.21037/tau-2021-01). The series “Update on Molecular Classification and Individualized Treatments of Genitourinary Tumors” was commissioned by the editorial office without any funding or sponsorship. AC, LC, MS, ALB and RM served as the unpaid Guest Editors of the series. LC serves as an unpaid editorial board member of Translational Andrology and Urology from Dec 2018 to Nov 2022. The authors have no other conflicts of interest to declare.
Ethical Statement: The authors are accountable for all aspects of the work in ensuring that questions related to the accuracy or integrity of any part of the work are appropriately investigated and resolved.
Open Access Statement: This is an Open Access article distributed in accordance with the Creative Commons Attribution-NonCommercial-NoDerivs 4.0 International License (CC BY-NC-ND 4.0), which permits the non-commercial replication and distribution of the article with the strict proviso that no changes or edits are made and the original work is properly cited (including links to both the formal publication through the relevant DOI and the license). See: https://creativecommons.org/licenses/by-nc-nd/4.0/.
References
- Cimadamore A, Cheng L, Scarpelli M, et al. Towards a new WHO classification of renal cell tumor: what the clinician needs to know—a narrative review. Transl Androl Urol 2021;10:1506-20.
- Aurilio G, Cimadamore A, Lopez-Beltran A, et al. Narrative review: update on immunotherapy and pathological features in patients with bladder cancer. Transl Androl Urol 2021;10:1521-9.
- Montironi R, Cheng L, Cimadamore A, et al. Narrative review of prostate cancer grading systems: will the Gleason Scores be replaced by the Grade Groups? Transl Androl Urol 2021;10:1530-40.
- Rizzo A, Mollica V, Cimadamore A, et al. TNM staging towards a personalized approach in metastatic urothelial carcinoma: what will the future be like?—a narrative review. Transl Androl Urol 2021;10:1541-52.
- Giunchi F, Franceschini T, Fiorentino M. A narrative review of individualized treatments of genitourinary tumors: is the future brighter with molecular evaluations? Transl Androl Urol 2021;10:1553-61.
- Santoni M, Cimadamore A, Massari F, et al. Narrative review: predicting future molecular and clinical profiles of prostate cancer in the United States. Transl Androl Urol 2021;10:1562-8.
- Rocco B, Cimadamore A, Sarchi L, et al. Current and future perspectives of digital microscopy with fuorescence confocal microscope for prostate tissue interpretation: a narrative review. Transl Androl Urol 2021;10:1569-80.
- Mollica V, Rizzo A, Di Nunno V, et al. Adjuvant therapy in renal cell carcinoma: is it the right strategy to inhibit VEGF? Transl Androl Urol 2021;10:1581-7.
- Montironi R, Cheng L, Lopez-Beltran A, et al. Decision support systems for morphology-based diagnosis and prognosis of prostate neoplasms: a methodological approach. Cancer 2009;115:3068-77. [Crossref] [PubMed]
- Cimadamore A, Lopez-Beltran A, Scarpelli M, et al. Digital pathology and COVID-19 and future crises: pathologists can safely diagnose cases from home using a consumer monitor and a mini PC. J Clin Pathol 2020;73:695-6. [Crossref] [PubMed]
- Goldenberg SL, Nir G, Salcudean SE. A new era: artificial intelligence and machine learning in prostate cancer. Nat Rev Urol 2019;16:391-403. [Crossref] [PubMed]
- Rakha EA, Toss M, Shiino S, et al. Current and future applications of artificial intelligence in pathology: a clinical perspective. J Clin Pathol 2020; Epub ahead of print. [Crossref] [PubMed]
- Moradi M, Salcudean SE, Chang SD, et al. Multiparametric MRI maps for detection and grading of dominant prostate tumors. J Magn Reson Imaging 2012;35:1403-13. [Crossref] [PubMed]
- Krizhevsky A, Sutskever I, Hinton GE. ImageNet classification with deep convolutional neural networks. Commun ACM 2017;60:84-90. [Crossref]
- LeCun Y, Bengio Y, Hinton G. Deep learning. Nature 2015;521:436-44. [Crossref] [PubMed]
- Janowczyk A, Madabhushi A. Deep learning for digital pathology image analysis: A comprehensive tutorial with selected use cases. J Pathol Inform 2016;7:29. [Crossref] [PubMed]
- Epstein JI, Amin MB, Fine SW, et al. The 2019 Genitourinary Pathology Society (GUPS) White Paper on Contemporary Grading of Prostate Cancer. Arch Pathol Lab Med 2020. [Epub ahead of print]. doi:
10.5858/arpa.2020-0015-RA . - Montironi R, Cimadamore A. Considerations on current and future issues related to reproducibility and accuracy in prostate cancer grading. Virchows Arch 2021;478:375-7. [Crossref] [PubMed]
- Lucas M, Jansen I, Savci-Heijink CD, et al. Deep learning for automatic Gleason pattern classification for grade group determination of prostate biopsies. Virchows Arch 2019;475:77-83. [Crossref] [PubMed]
- Nagpal K, Foote D, Liu Y, et al. Development and validation of a deep learning algorithm for improving Gleason scoring of prostate cancer. NPJ Digit Med 2019;2:48. Erratum in: NPJ Digit Med 2019;2:113. [Crossref] [PubMed]
- Rocco B, Sighinolfi MC, Sandri M, et al. Digital Biopsy with Fluorescence Confocal Microscope for Effective Real-time Diagnosis of Prostate Cancer: A Prospective, Comparative Study. Eur Urol Oncol 2020; Epub ahead of print. [Crossref] [PubMed]
- Chaddad A, Niazi T, Probst S, et al. Predicting Gleason Score of Prostate Cancer Patients Using Radiomic Analysis. Front Oncol 2018;8:630. [Crossref] [PubMed]
- Peng Y, Jiang Y, Yang C, et al. Quantitative analysis of multiparametric prostate MR images: differentiation between prostate cancer and normal tissue and correlation with Gleason score--a computer-aided diagnosis development study. Radiology 2013;267:787-96. [Crossref] [PubMed]
- Stoyanova R, Takhar M, Tschudi Y, et al. Prostate cancer radiomics and the promise of radiogenomics. Transl Cancer Res 2016;5:432-47. [Crossref] [PubMed]
- Pathmanathan AU, van As NJ, Kerkmeijer LGW, et al. Magnetic Resonance Imaging-Guided Adaptive Radiation Therapy: A "Game Changer" for Prostate Treatment? Int J Radiat Oncol Biol Phys 2018;100:361-73. [Crossref] [PubMed]
- Seligson DB, Horvath S, Shi T, et al. Global histone modification patterns predict risk of prostate cancer recurrence. Nature 2005;435:1262-6. [Crossref] [PubMed]
- Libbrecht MW, Noble WS. Machine learning applications in genetics and genomics. Nat Rev Genet 2015;16:321-32. [Crossref] [PubMed]
- Montironi MA. From image analysis in pathology to robotics and artificial intelligence. Anal Quant Cytopathol Histol 2016;38:268-9.