Identification and validation of a six immune-related gene signature for prediction of biochemical recurrence in localized prostate cancer following radical prostatectomy
Introduction
In the light of the latest cancer statistics reported, Prostate cancer (PCa) is one of the most common aggressive malignant tumors and the second lethal heterogeneous cancer among males worldwide (1,2). Currently, the treatments of PCa include the surgical resection, chemoradiotherapy, endocrinotherapy and immunotherapy (3,4), and the radical prostatectomy (RP) is recommended as the primary therapy for localized PCa. However, approximately 20% of PCa patients following RP will undergo biochemical recurrence (BCR), and eventually progress into castration-resistant prostate cancer (CRPC) (5,6). In accordance with the definition of the European Association of Urology (EAU), BCR refers to consecutive rising prostate-specific antigen (PSA) values above 0.2 ng/mL twice (7). Clinicopathological parameters, including PSA, Gleason score (GS) and TNM stage, have been introduced to predict BCR in PCa patients following RP (8). Nevertheless, patients with the same clinicopathological characteristics may develop into opposite clinical outcomes eventually (9). Therefore, it’s of great importance to construct molecular markers to better stratify these patients into high- and low-risk groups.
Recently, increasing evidence illustrated that the immune system, such as immune microenvironment and immune cells (macrophages, neutrophils, T cells, NK cells, DCs, B cells, iNKT cells) establish a critical role in the tumorigenesis and progression (10). PCa can be capable of escaping the immune system through downregulating human leukocyte antigen class I, inducing T-cell apoptosis and secreting immunosuppressive cytokines (11,12). Immunotherapies, such as autologous cellular immunotherapy (Sipuleucel-T) and experimental PCa vaccines (PROSTVAC, DCVAC/PCa, ProstAtak), have made dramatical progress in clinical studies (13). Considering the critical impacts of the immune factors in PCa initiation and progression, immune-related risk signature may serve as the potential candidate complement to original risk stratification so as to improve the prediction of BCR.
In the current study, we concentrated our efforts on performing an immune-related gene prognostic signature based on the whole list of immune genes downloaded from the Molecular Signatures Database v4.0. The RNA sequencing (RNA-seq) patient data of the open-access databases, including the Cancer Genome Atlas (TCGA, https:/portal.gdc.cancer.gov/) and the Gene Expression Omnibus (GEO, https:/www.ncbi.nlm.gov/geo/) database were utilized for our analysis and prediction. Then, we employed the TCGA microarray as a discovery set and further constructed the immune-related gene signature for localized PCa patients undergoing RP, which was systematically identified in the validation set (GSE70770 microarray). Finally, we aimed to figure out the association between this signature and clinicopathological parameters in localized PCa patients. The study would help to provide a stable and eligible genomic prognostic signature, which might serve as a crucial supplement to traditional clinical prognostic features for BCR after RP. We present the following article in accordance with the TRIPOD reporting checklist (available at: http://dx.doi.org/10.21037/tau-20-1231).
Methods
Mining and processing of sample databases and immune-related genes
Expression profile of genes together with clinical follow-up parameters, including age, PSA, stage, GS and outcomes (BCR) for localized PCa patients were acquired from The Cancer Genome Atlas (TCGA) RNA-seq in our retrospective study. Moreover, the whole related candidate data sets were recognized and evaluated by thoroughly seeking another public cohort named Gene Expression Omnibus (GEO) data sets before April 10, 2020. Inclusion and exclusion criteria of data sets were as follows: The eligible data sets included: (I) biospecimens were concentrated from localized PCa patients following RP; (II) each data set contained more than 60 specimens; (III) both clinicopathological characteristics [GS, clinical stage (T) or PSA] and results (BCR) were contained. The exclusion criteria included: (I) databases lacking efficient and sufficient sample data; (II) duplicates of the previously qualified databases; (III) patients also undergoing radiotherapy or chemotherapy in addition to the surgical resection. The list of immune related-genes were extracted from the Molecular Signatures Database v4.0 (14) (Immune response M19817, Immune system process M13664, http://www.broadinstitute.org/gsea/msigdb/index.jsp).
Construction and validation of the risk score model
The analysis charts of our study were illustrated in Figure 1. To estimate the relationship between the recurrence-free survival (RFS) and the expression of immune-related genes in TCGA and GEO databases, the univariate Cox regression analysis was performed. P=0.05 was selected as a cut-off for the correlation analysis, and the eligible immune-related genes were identified for the construction of prognostic model. Only the immune-related genes which were common in both TCGA and GEO data sets were selected. Subsequently, each candidate gene was enrolled into the step-wise multivariate Cox’s regression model to evaluate the independent prognostic contribution to RFS in the discovery data set (TCGA), and only the eligible genes were selected for further construction of the prognostic risk score model. Then, according to the regression coefficients calculated by the multivariate Cox’ s model and the expression level of candidate immune-related genes, the following algorithm of a risk score predicting BCR in localized PCa patients undergoing RP was established as follows:
More specially, the risk score of each patient was figured out by a linear combination of the expression value of immune-related genes weighted by the regression coefficient (β). β i refers to the regression coefficient of gene i obtained from the discovery cohort, and expi is the expression value of prognostic immune-related genes. By employing the median risk score of the discovery database as the threshold, we separated localized PCa patients into high-risk and low-risk groups for the following analysis.
Establishment and verification of the immune-related gene nomogram
In accordance with the independent prognostic immune-related genes screened from the Cox proportional hazards regression analyses, a compound nomogram was established to forecast the probability of 1-, 3- and 5-year survival of localized PCa patients following RP in the TCGA set. Then, the time-dependent ROC curves were plotted, and the area under the ROC curve was calculated to verify the exactitude of the novel prognostic nomogram. Besides, we utilized the calibration curves to compare the predicted and observed outcomes of the immune-related gene nomogram, among which the 45° line represented the best prediction.
Statistical analysis
Statistical analyses were conducted utilizing SPSS Statistics 26.0 and R software 3.6.3. The Fisher’s exact test or Chi‐squared test was applied for categorical variables, and the Wilcoxon rank-sum test or Student t-test for continuous variables. The comparison of RFS between high‐ and low‐risk groups was evaluated by the Kaplan‐Meier curve utilizing the log-rank test. The univariate Cox regression analysis was performed to estimate the relationships between RFS and the risk score, and the multivariate Cox proportional regression analysis was utilized to evaluate whether the six immune‐related gene model was independent of other clinicopathological parameters. The hazard ratio and the regression coefficient were figured out by the Cox proportional hazards regression model. To further assess the predicted accuracy and sensitivity of the risk score, the time-dependent receiver operating characteristic (ROC) analysis was utilized, and the area under curve (AUC) was correspondingly acquired. Harrell’s index of concordance (C-index) was applied to calculate and estimate the prognostic ability of the risk score. Two-sided P values less than 0.05 were considered statistically significant for the whole statistical analyses.
Results
Acquisition of the eligible databases
The method of database selection was revealed in Figure 1, and eventually, the TCGA data set and the GSE70770 database from the GEO cohort were chosen in our study. The discovery set (TCGA) and the validation set (GSE70770) included 268 and 77 localized PCa patients undergoing RP, separately. The clinicopathological features of both databases were demonstrated in Table 1, and there were no obvious differences of clinical characteristics, such as age, clinical stage (T), Gleason score and PSA, between the high-risk and low-risk groups.
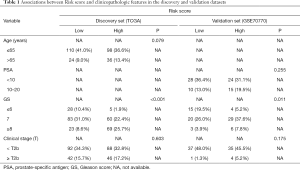
Full table
Identification and verification of the prognostic immune-related gene signature risk score
A total of 332 immune-related genes were extracted from the Molecular Signatures Database v4.0. Then, the Cox’s Analyses were performed in 332 immune-related genes for two data sets. After the univariate Cox’s analysis and log-rank test (P<0.05), we singled out 39 and 29 immune-related prognostic genes from the TCGA and the GSE70770 database, respectively, and only eleven common immune-related genes were found in both cohorts (Tables S1,S2 and Figure S1A). Further, to obtain the better prognostic genes, we applied the step-wise multivariate Cox’s analysis among eleven immune-related genes. In the end, six immune‐related genes comprising of SCIN, THY1, TBX1, NOTCH4, MAL and BNIP3L were identified, which were associated with RFS of localized PCa patients following RP.
In our study, we developed a six immune‐related gene prognostic signature by utilizing the risk score model. The risk score algorithm was calculated based on the regression coefficients and expression values of genes: Risk score = (−0.92247 × expression level of SCIN) + (2.54278 × expression level of THY1) + (1.13792 × expression level of TBX1) + (1.45694 × expression level of NOTCH4) + (−1.45727 × expression level of MAL) + (−3.29561 × expression level of BNIP3L) (Figure S1B and Table S3). Then, patients in the discovery set were divided into high- and low-risk groups and the median risk score was used as a cutoff value. Kaplan-Meier survival curves demonstrated that patients in the low-risk group had a significantly longer RFS compared to those in the high-risk one (P<0.0001) (Figure 2A). Those findings were subsequently verified in the validation data set (GSE70770) according to the same algorithm and regression coefficient (β), which displayed the similar results (P=0.0016) as expected (Figure 2B). In line with the coefficient value of the six immune‐related genes, the risk scores for samples were calculated and ranked in both cohorts. The survival condition of localized PCa patients following RP was signed as the dot plot. As shown in Figure 3A and B, with the increase of the risk scores, the localized PCa patients in the high-risk group increased, and the number of recurrent persons grew. The heatmap of six immune‐related genes expression profiles between high- and low-risk patients was also detailed in this figure.
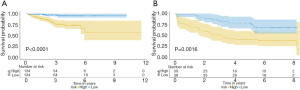
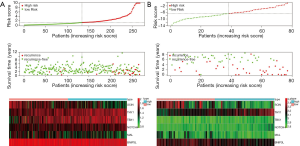
The immune-related gene signature is an independent prognostic factor of RFS in localized PCa patients
The Cox’s regression analyses were applied to assess whether the prognostic value of the six immune‐related gene signature was an independent risk factor in the discovery and validation sets compared to several clinicopathological factors. The results were shown in Tables 2 and 3. In the univariate Cox model of RFS, the high-risk group revealed a 7.453-fold increased and 2.973-fold increased risk of death compared with the low-risk one, respectively (discovery set: 95% CI: 2.613–21.261, P<0.001; validation set: 95% CI: 1.458–6.064, P=0.003) (Table 2). In the multivariate Cox regression model, after adjustment for age, PSA, Gleason score and clinical stage (T), the six immune‐related gene signature was an independent prognostic factor, which was significantly associated with RFS (discovery set: HR =5.232, 95% CI: 1.762–15.538, P=0.003; validation set: HR =2.158, 95% CI: 1.051–4.432, P=0.036) (Table 3).
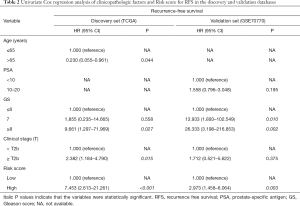
Full table
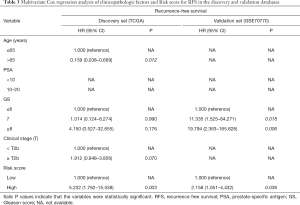
Full table
Supplementary to original clinical risk stratification with the risk score
To further evaluate the prognostic ability of the six immune‐related gene prognostic signature, the time-dependent ROC analyses were applied, and the AUC (area under curve) values were calculated and estimated. The risk score curve value for RFS was 0.834 in the discovery set, which was much higher compared with clinical stage (T) (AUC =0.610) and the Gleason score curve value (AUC =0.687). Similarly, the risk score AUC value in the validation set (AUC =0.798) was also remarkably higher than those connected with PSA (AUC =0.673), clinical stage (T) (AUC =0.553), and Gleason score (AUC =0.679) (Figure 4). The aforementioned results illustrated that the six immune‐related gene signature was a better prospective independent prognostic risk score of RFS in localized PCa patients than other clinical features.
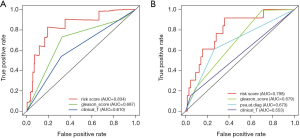
Additionally, to furnish a more accurate and valid prediction model for BCR in PCa patients after RP, the risk score was integrated with original clinical prognostic factors [clinical stage (T), PSA and Gleason score]. Hence, we constructed the multivariate prognostic modules, including GS + clinical stage (T) + risk score in the discovery set and PSA + GS + clinical stage (T) + risk score in the validation set. In Both data sets, the Concordance index (C-index) of the multivariate prognostic module was not only higher than that of the gene risk model but also was much higher than that of traditional clinical risk factors (Table 4). The results underscored that the risk score was a robust complement to clinical risk factors for BCR.
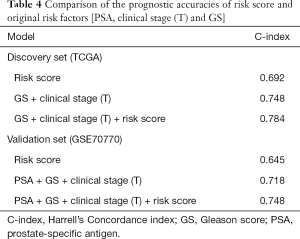
Full table
Establishment and verification of the prognostic nomogram based on six hub immune-related genes
In order to provide a clinically quantitative tool for prognosis of localized PCa patients, we integrated the six immune-related genes (SCIN, THY1, TBX1, NOTCH4, MAL, BNIP3L) signature to establish a nomogram plot (Figure 5). A horizontal line was drawn to determine the point of each variable. Then we calculated the total points for each patient by summing the points of all variables and normalize it to a distribution of 0 to 100. This allowed us to calculate the survival probabilities of PCa patients at 1-, 3- and 5-year by plotting a vertical line between the total point axis and each prognosis axis. The calibration curves of the nomogram indicated the strong consistency between the predicted survival and actual observed survival (Figure 6A). In addition, the time-dependent ROC analyses were utilized to further assess the power of our novel nomogram, which displayed the sensitivity and specificity of a clinical model. The X-axis meant false positive rate and the Y-axis meant sensitivity. The 1-, 3- and 5-year AUC values of our nomogram were 0.800, 0.828 and 0.815, respectively, suggesting an excellent prognostic ability (Figure 6B). The results illustrated that our prognostic nomogram could better predict patients’ survival status, which might assist relevant practitioners in developing clinical decision-making for localized PCa patients with BCR.
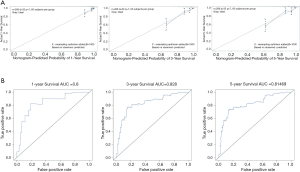
Discussion
Evidence illustrated that several localized PCa patients undergoing RP would eventually progress into BCR, and the study showed that 10-year survival probability of PCa patients BCR-free was 68% (15). Early BCR had significant association with PCa recurrence, metastasis, and mortality. Therefore, active surveillance of BCR for PCa patients following RP was quite meaningful for further therapies.
So far, surveillance of BCR in PCa patients has been based on clinicopathological characteristics with no strong integrity, so it would be beneficial and valuable to search for several new biomarkers, such as lncRNA, microRNA, mRNA for a better prediction of BCR. For instance, the long non-coding RNAs (lncRNAs) were of great importance for the molecular mechanism of PCa (16). Shao et al. (17) found that the five lncRNAs (CRNDE, PRKAG2-AS1, RP11-783K16.13, RP11-727F15.11 and AC013460.1) could serve as a novel prognostic gene signature for predicting BCR in PCa patients after RP, and these IncRNAs were likely to help PCa patients in targeted molecular therapies. The functions of microRNAs in PCa could not be denied. Zhao et al. (18) constructed the BCR scoring system based on the five-microRNA molecular biomarkers, and the combination of these microRNAs remarkably enhanced the ability of prediction for PCa patients experiencing BCR after RP. As for mRNA, Li et al. (19) identified that SAMD5 was overexpressed in PCa patients, which was feasible for BCR risk assessment, and the survival analysis gave a clear illustration that the increased expression of SAMD5 mRNA was related to a worse prognosis for PCa patients following RP. Nevertheless, few studies concentrated on the immune‐related genes and their effects on predicting BCR in localized PCa patients underwent RP.
In recent years, immune genes established a remarkable role in tumorigenesis, development and metastasis. Moreover, the immune response and immunotherapy showed great significance in tumor biological processes (10). With regard to PCa, the relationship between the immune system and its anti-tumor activity was quite complicated (20). For instance, mastocyte showed negative association with clinical features and BCR, while tumor-associated macrophage (TAM) played a positive role in cancer invasiveness and recurrence following RP (21,22). Referring to the immunotherapy, Sipuleucel-T was the first autologous cellular therapy for PCa, which was recommended by US Food and Drug Administration (FDA) (23).
In our study, we singled out six immune‐related genes (SCIN, THY1, TBX1, NOTCH4, MAL, BNIP3L), which was significantly connected with BCR in localized PCa patients undergoing RP. The regression coefficient of three genes (THY1, TBX1, NOTCH4) were additive, while the other three genes (SCIN, MAL, BNIP3L) were subtractive. According to the algorithm, the additive genes were positively corrected with the risk score. Our study showed that the higher the risk scores, the more the patients in high-risk group, and the higher the numbers of recurrent persons. Therefore, the additive genes (THY1, TBX1, NOTCH4) might contribute to the development and progression of PCa. On the contrary, SCIN, MAL, BNIP3L were negatively corrected with the risk score, which might play an antioncogenic role in PCa. Then, we established a six immune‐related gene signature that could distinguish samples into the high- and low-risk groups. Next, the survival curves demonstrated that high immune‐related genes risk scores were related to poor RFS in both the training cohort (TCGA) and the testing database (GSE70770). Based on the univariate and multivariate Cox regression models, the six immune‐related gene signature was considered as an independent prognostic parameter for BCR in localized PCa patients. The AUC (area under curve) values of the risk score for survival prediction were much higher than those of other clinicopathological prognostic factors, including PSA, clinical stage (T) and GS in both cohorts. Therefore, our risk score was superior to original clinical features and more sensitive and specific of predicting BCR in PCa. Furthermore, we combined the risk score with traditional clinicopathological parameters, and the C-index of the multivariate prognostic module got remarkably improved. The results illustrated that our risk score was supplementary to current clinical risk stratification, which enhanced the veracity of prognostic module for BCR. In addition, a novel nomogram was created to better predict the 1-year RFS, 3-year RFS and 5-year RFS of localized PCa patients after RP based on six immune-related gene signature. The presentation of calibration plot illustrated that there was great conformity between the predicted and observed results, and the time-dependent ROC analysis of our nomogram demonstrated the excellent prognostic power in predicting RFS.
Several studies indicated that these immune‐related genes played an important role in tumorigenesis, even in PCa. For example, silencing of SCIN reduced the expression of EGFR and slowed the growth of PCa cells (24). Furthermore, by inhibiting the expression of SCIN, the PCa cell proliferation was suppressed and cell cycle arrested in G0/G1 phase, which was likely to be a promising therapy for PCa patients in the future. As for THY1, it was reported that the overexpression of CD90/THY1 was linked to PCa stromal fibromuscular cells and the tumor microenvironment, which could serve as a tumor marker in the diagnosis and treatment of PCa (25,26). Originating from the T-box family, TBX1 served as a tumor inhibitor in thyroid cancer by regulating PI3K/AKT and MAPK/ERK pathways (27). As a biomarker in many tumors, NOTCH4 established significant roles in cell growth, invasion and apoptosis. Zhang et al. (28) revealed that suppressing the expression of NOTCH4 could inhibit the PCa progression and epithelial-mesenchymal transition by stimulating NF-κB. Regarding MAL (29), it was reported that low expression of MAL was associated with poor failure-free survival and it might serve as an independent prognostic signature when estimating the tumor immune infiltrate in classical Hodgkin lymphoma. Moreover, Cheng et al. (30) demonstrated that BNIP3Lwas significantly associated with PCa stage via the cell apoptosis way, and this made great contributions to the clarification of genomic alterations of PCa aggressiveness. In conclusion, studies as mentioned above revealed the reasonability and accuracy of our six immune‐related gene signature in cancer initiation and progression.
It is worth noting that several limitations should be mentioned in spite of our efforts to avert the defect in our study. First, this study was retrospective, thus the accuracy and effectiveness of our six immune‐related gene prognostic signature were required to be further verified in some other public cohorts, even in related clinical trials. Second, two databases were selected in our study with no complete clinicopathological parameters, such as PSA or age. Third, experimental validation was needed to pay attention to evaluate the biological functions. Therefore, multi-institutional and well-designed studies are required to verify this immune‐related gene signature in the future. Despite the mentioned deficiencies, the predictive value of the risk score for BCR in localized PCa patients following RP cannot be ignored.
Conclusions
In summary, we singled out six prognostic immune genes that could predict BCR in localized PCa patients following RP, namely SCIN, THY1, TBX1, NOTCH4, MAL, BNIP3L. Combining the immune-related gene signature with original clinicopathological parameters might further stratify these patients for diverse prognosis and treatment. However, further prospective studies are required to verify the efficiency and accuracy of this signature and to have a better understanding of the molecular mechanisms in PCa.
Acknowledgments
We would like to thank the researchers and study participants for their contributions.
Funding: The study was supported by the National Natural Science Foundation of China (81871151, 81971377).
Footnote
Reporting Checklist: The authors have completed the TRIPOD reporting checklist. Available at http://dx.doi.org/10.21037/tau-20-1231
Conflicts of Interest: All authors have completed the ICMJE uniform disclosure form (available at http://dx.doi.org/10.21037/tau-20-1231). The authors have no other conflicts of interest to declare.
Ethical Statement: The authors are accountable for all aspects of the work in ensuring that questions related to the accuracy or integrity of any part of the work are appropriately investigated and resolved. The study was conducted in accordance with the Declaration of Helsinki (as revised in 2013).
Open Access Statement: This is an Open Access article distributed in accordance with the Creative Commons Attribution-NonCommercial-NoDerivs 4.0 International License (CC BY-NC-ND 4.0), which permits the non-commercial replication and distribution of the article with the strict proviso that no changes or edits are made and the original work is properly cited (including links to both the formal publication through the relevant DOI and the license). See: https://creativecommons.org/licenses/by-nc-nd/4.0/.
References
- Bray F, Ferlay J, Soerjomataram I, et al. Global cancer statistics 2018: GLOBOCAN estimates of incidence and mortality worldwide for 36 cancers in 185 countries. CA Cancer J Clin 2018;68:394-424. [Crossref] [PubMed]
- Ferlay J, Colombet M, Soerjomataram I, et al. Estimating the global cancer incidence and mortality in 2018: GLOBOCAN sources and methods. Int J Cancer 2019;144:1941-53. [Crossref] [PubMed]
- Gunjur A. Personalising the treatment of prostate cancer. Lancet Oncol 2015;16:e592 [Crossref] [PubMed]
- Denmeade SR, Isaacs JT. A history of prostate cancer treatment. Nat Rev Cancer 2002;2:389-96. [Crossref] [PubMed]
- Luo HW, Chen QB, Wan YP, et al. Protein regulator of cytokinesis 1 overexpression predicts biochemical recurrence in men with prostate cancer. Biomed Pharmacother 2016;78:116-20. [Crossref] [PubMed]
- Simmons MN, Stephenson AJ, Klein EA. Natural history of biochemical recurrence after radical prostatectomy: risk assessment for secondary therapy. Eur Urol 2007;51:1175-84. [Crossref] [PubMed]
- Cornford P, Bellmunt J, Bolla M, et al. EAU-ESTRO-SIOG Guidelines on Prostate Cancer. Part II: Treatment of Relapsing, Metastatic, and Castration-Resistant Prostate Cancer. Eur Urol 2017;71:630-42. [Crossref] [PubMed]
- Mottet N, Bellmunt J, Bolla M, et al. EAU-ESTRO-SIOG Guidelines on Prostate Cancer. Part 1: Screening, Diagnosis, and Local Treatment with Curative Intent. Eur Urol 2017;71:618-29. [Crossref] [PubMed]
- Li D, Lv H, Hao X, et al. Prognostic value of serum alkaline phosphatase in the survival of prostate cancer: evidence from a meta-analysis. Cancer Manag Res 2018;10:3125-39. [Crossref] [PubMed]
- Gonzalez H, Hagerling C, Werb Z. Roles of the immune system in cancer: from tumor initiation to metastatic progression. Genes Dev 2018;32:1267-84. [Crossref] [PubMed]
- Lu X, Horner JW, Paul E, et al. Effective combinatorial immunotherapy for castration-resistant prostate cancer. Nature 2017;543:728-32. [Crossref] [PubMed]
- Drake CG, Jaffee E, Pardoll DM. Mechanisms of immune evasion by tumors. Adv Immunol 2006;90:51-81. [Crossref] [PubMed]
- Bilusic M, Madan RA, Gulley JL. Immunotherapy of Prostate Cancer: Facts and Hopes. Clin Cancer Res 2017;23:6764-70. [Crossref] [PubMed]
- Subramanian A, Tamayo P, Mootha VK, et al. Gene set enrichment analysis: a knowledge-based approach for interpreting genome-wide expression profiles. Proc Natl Acad Sci U S A 2005;102:15545-50. [Crossref] [PubMed]
- Roehl KA, Han M, Ramos CG, et al. Cancer progression and survival rates following anatomical radical retropubic prostatectomy in 3,478 consecutive patients: long-term results. J Urol 2004;172:910-4. [Crossref] [PubMed]
- Shao N, Zhu Y, Wan FN, et al. Identification of seven long noncoding RNAs signature for prediction of biochemical recurrence in prostate cancer. Asian J Androl 2019;21:618-22. [Crossref] [PubMed]
- Shao N, Tang H, Qu Y, et al. Development and validation of lncRNAs-based nomogram for prediction of biochemical recurrence in prostate cancer by bioinformatics analysis. J Cancer 2019;10:2927-34. [Crossref] [PubMed]
- Zhao Z, Weickmann S, Jung M, et al. A Novel Predictor Tool of Biochemical Recurrence after Radical Prostatectomy Based on a Five-MicroRNA Tissue Signature. Cancers 2019;11:1603. [Crossref] [PubMed]
- Li F, Xu Y, Liu RL. SAMD5 mRNA was overexpressed in prostate cancer and can predict biochemical recurrence after radical prostatectomy. Int Urol Nephrol 2019;51:443-51. [Crossref] [PubMed]
- Zhao SG, Lehrer J, Chang SL, et al. The Immune Landscape of Prostate Cancer and Nomination of PD-L2 as a Potential Therapeutic Target. J Natl Cancer Inst 2019;111:301-10. [Crossref] [PubMed]
- Strasner A, Karin M. Immune Infiltration and Prostate Cancer. Front Oncol 2015;5:128. [Crossref] [PubMed]
- Lanciotti M, Masieri L, Raspollini MR, et al. The role of M1 and M2 macrophages in prostate cancer in relation to extracapsular tumor extension and biochemical recurrence after radical prostatectomy. Biomed Res Int 2014;2014:486798 [Crossref] [PubMed]
- Handy CE, Antonarakis ES. Sipuleucel-T for the treatment of prostate cancer: novel insights and future directions. Future Oncol 2018;14:907-17. [Crossref] [PubMed]
- Wang D, Sun S Q, Yu YH, et al. Suppression of SCIN inhibits human prostate cancer cell proliferation and induces G0/G1 phase arrest. Int J Oncol 2014;44:161-6. [Crossref] [PubMed]
- Pascal LE, Goo YA, Vêncio RZ, et al. Gene expression down-regulation in CD90+ prostate tumor-associated stromal cells involves potential organ-specific genes. BMC Cancer 2009;9:317. [Crossref] [PubMed]
- True LD, Zhang H, Ye M, et al. CD90/THY1 is overexpressed in prostate cancer-associated fibroblasts and could serve as a cancer biomarker. Mod Pathol 2010;23:1346-56. [Crossref] [PubMed]
- Wang N, Li Y, Wei J, et al. TBX1 Functions as a Tumor Suppressor in Thyroid Cancer Through Inhibiting the Activities of the PI3K/AKT and MAPK/ERK Pathways. Thyroid 2019;29:378-94. [Crossref] [PubMed]
- Zhang Z, Bu X, Yang J, et al. NOTCH4 regulates colorectal cancer proliferation, invasiveness, and determines clinical outcome of patients. J Cell Physiol 2018;233:6975-85. [Crossref] [PubMed]
- Kelley TW, Pohlman B, Elson P, et al. The ratio of FOXP3+ regulatory T cells to granzyme B+ cytotoxic T/NK cells predicts prognosis in classical Hodgkin lymphoma and is independent of bcl-2 and MAL expression. Am J Clin Pathol 2007;128:958-65. [Crossref] [PubMed]
- Cheng I, Levin AM, Tai YC, et al. Copy number alterations in prostate tumors and disease aggressiveness. Genes Chromosomes Cancer 2012;51:66-76. [Crossref] [PubMed]