Current applications of artificial intelligence combined with urine detection in disease diagnosis and treatment
Introduction
Artificial intelligence (AI) was proposed by John McCarthy at the Dartmouth conference held in 1956 in New Hampshire. It refers to a new technology science that researches and develops theories and methods (1). Generally speaking, AI is a kind of computer technology that can simulate human behavior and extend human ability beyond human instructions. In recent years, with the development of data analysis, image recognition, and other technologies, AI application in the medical field is gradually expanding and becoming more in-depth, especially in medical image recognition processing and clinical medical intelligent decision-making. AI provides new opportunities for early detection, accurate diagnosis, and the individualized treatment and management of diseases (2-4).
Urine is a kind of liquid excrement that is excreted from humans and vertebrates through the urinary system and urinary tract as a byproduct of metabolism. Urine contains many substances, such as proteins, electrolytes, sugars, and creatinine, amongst others, which can change under different physiological and pathological conditions (4-6). Therefore, AI combined with urine detection provides the possibility for the diagnosis of diseases. Based on the different urine components, this paper summarizes the research field and applications of AI combined with urine component detection and analysis in the diagnosis and treatment of urinary system diseases, cardiovascular diseases, digestive tract diseases, and other multisystem diseases, which are also summerized in Table 1 and Figure 1. We also explore its limitations and development prospects. We present the following article following the Narrative review checklist (available at http://dx.doi.org/10.21037/tau-20-1405).
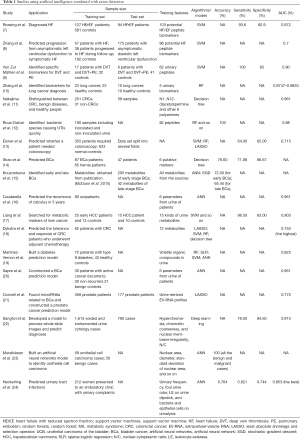
Full table
Methods
A systematic literature search was conducted in September 2020 based on computerized databases, including PubMed, EMBASE, the Cochrane Library, and 2 main Chinese databases (WANFANG and CNKI) without language restrictions. Search terms or keywords used included “artificial intelligence”, “machine learning”, “urine proteomic”, “urine metabolomic”, “urine RNomics”, and “urine cytopathology”. Moreover, we performed a search of the reference lists of the included studies or relevant reviews to ensure literature saturation.
AI and medicine
The core technologies of AI include computer vision, machine learning, natural language processing, robotics, and speech recognition (25,26). AI technology applications in the medical field are mainly computer vision and machine learning (27-34). Computer vision aims to replace the visual organ as the input means through the imaging system, then analyzes and processes the image through the computer. Therefore, computer vision is widely used in medical imaging to improve recognition and analysis ability to help predict and diagnose the disease (27-32). AI has been widely used in the image-based diagnosis and has shown strong perandom forestsormance. Kermany et al. (31) used the combination of deep learning and optical coherence tomography images to diagnose retinal-related lesions. This technology demonstrated good perandom forestsormance in diagnosing age-related macular degeneration and diabetic macular edema. Also, AI applications based on other data types, such as electronic health records, are developing rapidly. Liang et al. (32) developed a natural language processing system based on deep learning, which could effectively extract data from electronic health record and build a diagnostic system based on the data. The results showed that the system could accurately diagnose multiple pediatric diseases. Machine learning is multidisciplinary and interdisciplinary, covering probability theory knowledge, statistics knowledge, approximate theoretical knowledge, and complex algorithm knowledge. It uses the computer to simulate the real-time human learning process and divides existing content into knowledge structures to effectively improve learning efficiency (30-33). With the popularization of computer network technology and the rise of big data, massive medical data holds great value. Machine learning technology can effectively collect and process this data, and improve the level of disease diagnosis or provide the possibility for devising personalized treatment plans. According to different learning methods, AI can be divided into supervised learning, unsupervised learning, and reinforcement learning. Its common algorithms mainly include decision trees, support vector machines, random forests, artificial neural networks, and deep learning, amongst others. Of these algorithms, support vector machines and random forests are widely used in clinical medicine. These algorithms have different characteristics and advantages. Support vector machines can transform the problem of nonlinear separability into a linearly separable problem. A decision tree is a tree-like decision-making model in which each internal node represents a judgment on an attribute, each branch represents the output of a judgment result, and finally, each leaf node represents a classification result. Random forest is a classifier that contains multiple decision trees, randomizes the use of variables (columns) and data (rows), generates many classification trees, and summarizes the classification trees’ results. Deep learning is also gradually being utilized in medicine. Through its multilayer structure, the machine can automatically find the features or laws in the data to improve perandom forestsormance to predict and classify the data. According to the different characteristics of the algorithms, they are applied to many aspects. For example, deep learning has obvious advantages in processing the increasing amount of big data in medicine and medical imaging diagnosis. support vector machines and random forests are used to establish AI-assisted diagnosis technologies. Applications of other medical field algorithms are also being continuously developed and evaluated (34-36).
AI and urine detection
AI combined with urine proteomics
Urine produced under normal conditions contains a small number of polypeptides and proteins. When the body undergoes physiological and pathological changes, the types and content of polypeptides and proteins in the urine may change. Therefore, it is possible to recognize the changes of related proteins in urine through AI and carry out disease diagnosis (37-40). In 2016, Rossing et al. (7) of the University of Copenhagen in Denmark obtained urine proteins by capillary electrophoresis combined with mass spectrometry. Based on this, a machine learning algorithm for heart failure with decreased ejection fraction was established. After testing, the area under the receiver operating characteristic (ROC) curve (AUC), sensitivity, and specificity of the model were 0.972, 93.6%, and 92.9%, respectively.
Similarly, Zhang et al. (8) constructed a machine learning model based on 96 potential heart failure-specific peptide biomarkers to predict sudden heart failure, and the results showed that the AUC of the model was 0.7. These results suggest that the technique may help understand the pathogenesis and diagnosis of heart failure with reduced ejection fraction. Von Zur Mühlen et al. (9) from the Department of Cardiology of Freiburg University, Germany, used a support vector machines model and urine proteins obtained from capillary electrophoresis coupled to mass spectrometry to construct a prediction model of deep vein thrombosis in a 2015 study. The model’s sensitivity and specificity were 100% and 83%, respectively, in an independent cohort of 6 cases and 41 controls. This technique may help find the specific proteins and peptide markers of lower extremity venous thrombosis and assist clinicians in predicting the occurrence of lower extremity venous thrombosis earlier and more accurately in future clinical practice. Zhang et al. (10) of the State Key Laboratory of proteomics, China National Center for protein science, combined proteomics technology with a random forests model. They built a lung cancer prediction model by using the random forests algorithm to search for possible lung cancer-specific diagnostic markers in urine, and the feature selection algorithm was used to screen out sensitive urinary protein markers. After testing, the model could correctly classify most lung cancer cases in the training group (n=46) and the test set (n = 14–47), and the AUC ranged from 0.8747 to 0.9853. Nakajima et al. (11) of Tokyo Medical University used polyamine biomarkers in the urine combined with machine learning to distinguish between colorectal cancer, benign diseases, and healthy people. They utilized liquid chromatography-mass spectrometry (LC-MS) to profile 7 kinds of polyamines. Based on machine learning algorithms such as decision trees, the classifier achieved an effective prediction of colorectal cancer, and the study found that N1, N12-diacetylspermine had the best AUC (0.794) among these polyamine species. Moreover, polyamine combination showed a higher AUC of 0.961. Similarly, combining LC-MS with machine learning techniques, Roux-Dalvai et al. (12) used data-independent acquisition and machine learning algorithms to define a peptide signature of bacterial species causing urinary tract infection and then investigated the unknown urine samples by targeted proteomics. This technology effectively shortened the detection time of pathogens and had 100% accuracy when looking at data above the clinical threshold of 1×105 CFU/mL (CFU: colony forming units). The studies described above show that AI provides possibilities for non-invasive and precise diagnosis of diseases based on urine proteomics information.
AI combined with urine metabolomics
Metabolomics is a research method that conducts a quantitative analysis of all metabolites in organisms’ bodies and seeks to determine the relative relationship between metabolites and physiological and pathological changes (41,42). Urine, as the excreta produced by human metabolism, contains much metabolomics information. However, due to the variety of metabolites, a mass of data, and the remaining uncertainties regarding research on diseases’ pathophysiological changes, using urinary metabolites for disease diagnosis and treatment in the past mostly remained theoretical. The development of AI technology makes it possible to process urinary metabonomics data, which contains much noise. Eisner et al. (13) used machine learning algorithms to establish a predictive model, which aimed to predict whether a patient needed a colonoscopy based on urine’s metabolic profile. The study used the urine metabolic profiles, colonoscopy results, and medical histories of 988 patients (633 normal and 355 who required colonoscopy) to build a predictive model through machine learning algorithms such as support vector machines. The predictor judged whether a new patient needed a colonoscopy by analyzing their urine profile and medical history. The results showed that the predictive model had a sensitivity of 64% and a specificity of 65%, and the experimenter could balance and adjust the two. Shao et al. (14) analyzed the metabolites in patients’ urine with bladder cancer and hernia by ultra-high perandom forestsormance liquid chromatography-mass spectrometry. They then established the prediction model of bladder cancer based on the urine metabolism spectrum and 6 candidate urine markers. The algorithm’s diagnostic accuracy, sensitivity, and specificity were 76.60%, 71.88%, and 86.67%, respectively.
Similarly, Kouznetsova et al. (15) combined urine metabolites and machine learning algorithms to identify early and late bladder cancer. The best performing model was able to predict metabolite class of different stage with an accuracy of 82.54% and the area under precision-recall curve of 0.84 on the training set. Caudarella et al. (16) utilized an artificial neural network to process 6 parameters, including serum Na and K, Na, P, Oxalate, and the AP (CaP) index (ion-activity products, AP; calcium phosphate, CaP) from the urine of patients with urinary calculi and the recurrence of calculus in 5 years. They found that these parameters had a significant nonlinear relationship with the recurrence of calculus. The results suggested that this model could partly predict the recurrence of calculus. Liang et al. (17) analyzed the urine metabolism spectrum using liquid chromatography-quadrupole time of flight-mass spectrometry and then searched for liver metabolic markers cancer through a machine learning algorithm. They found that 15 kinds of urine metabolites were helpful for the diagnosis of liver cancer. Among them, the sensitivity and specificity of palmitic acid, alpha-N-Phenylacetyl-L-glutamine, phytosphingosine, indoleacetyl glutamine, and glycocholic acid for liver cancer diagnosis were 96.5% and 83.0%, respectively. Such techniques can be used not only to diagnose diseases but also to predict prognosis. Dykstra et al. (18) established a machine learning algorithm by combining urine metabonomics with AI to predict the tolerance and response of colorectal cancer patients after adjuvant chemotherapy. In this study, 4 different machine learning algorithms, including the least absolute shrinkage and selection operator algorithm, support vector machines, decision tree, and random forests, were used to construct prediction models to predict the 5-year survival rate, tumor recurrence, chemotherapy dose reduction, and treatment cycle extension of colorectal cancer patients after adjuvant chemotherapy. For 5-year survival, the AUC of the optimal prediction model was 0.612, the AUC of the optimal prediction model of cancer recurrence was 0.650, chemotherapy dose reduction was 0.542, and treatment cycle extension was 0.750. Martinez-Vernon et al. (19) used field asymmetric ion mobility spectrometry to analyze and determine the volatile organic compounds in the urine. These data used a variety of machine learning algorithms to build a diabetes prediction model, including random forests, sparse logistic regression, support vector machines and so on. Among them, the best AUC of the model based on sparse logistic regression was 0.825 [95% confidence interval (CI): 0.747–0.9], which could effectively identify diabetes. These findings suggest that urine metabolomics is a promising additional tool for clinicians to use in disease diagnosis and prognosis assessments.
AI combined with urine RNomics
RNAs are key molecules that regulate protein synthesis and cell functions in the body. Urine generally contains a small amount of microRNAs, which regulate gene expression by binding to mRNA molecules and affect their stability or translation. Therefore, microRNAs are often specifically expressed during tumor occurrence. These abnormal microRNAs can act as molecular biomarkers to assist in tumor diagnosis, predict prognosis, and evaluate treatment responses, and can be detected and analyzed using AI (43,44). Sapre et al. (20) selected 12 microRNAs that may be related to the occurrence of bladder cancer through previous research and constructed a bladder cancer prediction model based on a machine learning algorithm. The study confirmed that the prediction model of bladder cancer was constructed by miR16, miR200c, miR205, miR21, and miR34a with 88% sensitivity and 48% specificity. The AUC of the best predictor to distinguish patients with bladder cancer from non-recurrers was 0.85, especially for stage T1 bladder cancer with an AUC of 0.92. The results suggest that this model can reduce the cystoscopy rate in the validation queue by 30%. Connell et al. (21) constructed a prostate cancer prediction model based on urine-derived extracellular vesicle RNA. Through verification, the AUC of the model in diagnosing clinically significant middle and high-risk prostate cancer was 0.77. It also had a better predictive ability for the prognosis of prostate cancer patients who underwent active monitoring, providing a new direction for the non-invasive diagnosis of prostate cancer. The above results suggest that AI technology has high accuracy in diagnosing bladder cancer, prostate cancer, and other diseases based on the type and content of RNA in the urine.
AI combined with urine cytopathology
Under both physiological and pathological conditions, a variety of cells can be observed in urine, and most of them are of great significance to the diagnosis of urinary system diseases. White blood cells and pus cells in urine often indicate urinary system infection. Urine red blood cell morphology can be used to distinguish glomerular diseases from other diseases. The urinary tract’s epithelial cells come from the entire urinary system, from the kidney to the urethra. When urinary tract tumors occur, they can provide pathological evidence for diagnosis (45,46). Qin et al. (47) used support vector machines to efficiently recognize urine cell images, combined with hue saturation intensity color parameters, spatial parameters, and grid search cross-validation optimization selection parameters. Sanghvi et al. (22) developed a deep learning computational pipeline with multiple tiers of convolutional neural network models to process whole slide images and predict diagnosis. The algorithm’s sensitivity was 79.5%, and the specificity was 84.5% for high-grade urothelial carcinoma. Similarly, Muralidaran et al. (23) built an artificial neural networks model to identify urothelial cell carcinoma based on the visual and morphometric data from urine cytology. This model diagnosed all the cases correctly in the test set, except a low-grade case, which was diagnosed as high grade.
AI combined with urinary function
Lower urinary tract symptoms (LUTS) are common symptoms of urinary system diseases and mainly include symptoms that impact urinary storage and micturition period (48). The former refers to frequent urination, urinary urgency, increased nocturia, while the latter mainly refers to dysuria, thin urine line, slow urine flow and so on. LUTS are of great significance in diagnosing benign prostatic hyperplasia, urinary tract infection, neurogenic bladder, and other diseases. Moreover, Gacci et al. (49) found that older men with moderate to severe LUTS had an increased risk of cardiovascular events. Thus, LUTS are also significant for the diagnosis of some non-urinary diseases. However, the diagnosis of LUTS mainly depends on the patient’s description, urination log, and other methods at present, which have shortcomings of subjectivity and inaccurate record keeping, which makes it difficult to judge symptoms diagnose diseases. Heckerling et al. (24) combined artificial neural networks and genetic algorithms to evaluate urine and predict urinary tract infections. Firstly, they used genetic algorithms to select the 5 best urine variables that could be used to build predictive models, including urinary frequency, foul urine odor, leukocyte esterase on urine dipstick, and bacteria epithelial cells on urinalysis. Then they used artificial neural networks to construct a predictive model. The ROC area of the model for identifying urinary tract infection and non-infection was 0.853, and when the network output threshold was 0.25, the sensitivity was 0.821, the specificity was 0.744, and the accuracy was 0.764. Yuan et al. (50) established a machine learning algorithm based on the data collected by a portable urination recorder, such as daily urination volume, times, night urination volume, and night urination times, to objectively evaluate the degree of patients’ LUTS and provide evidence for accurate diagnosis and individualized treatment of the disease.
Discussion
The application of AI in the medical field has a bright prospect. Taking China as an example, an average of 57 million cases are misdiagnosed each year in clinical care, and the total misdiagnosis rate is as high as 27.6% (51). Both disease diagnosis and medical imaging analysis depend on the personal experience and subjective judgment of clinicians and imaging physicians. Therefore, in areas with poor medical conditions and weak medical systems, the chance of misdiagnosis and missed diagnosis is even higher. AI-assisted diagnosis technology can undoubtedly help to reduce misdiagnosis and missed diagnosis. Also, the development of big medical data has brought many possibilities for basic medicine and clinical diagnosis and treatment; however, the huge amount of data and redundant medical data are accumulating over time. AI technology can efficiently and accurately process this data and improve itself in the continuous accumulation and processing of data. It is unrealistic and inefficient to rely on humans to deal with this data. The above advantages are also well reflected in AI combined with urine detection. Urine-related data has the characteristics of large volume, easy collection, and rich information. The effective use of the data obtained from urine can help us understand many diseases’ pathological mechanisms and assist clinicians in diagnosis and treatment. It is noteworthy that urine collection is non-invasive and simple, providing new diagnostic methods and ideas for many diseases that rely on invasive procedures for diagnosis.
Despite more and more research and AI achievements in medicine, there is still a long way to go before AI products are widely used in medical practice. Firstly, the lack of evaluation standards for AI in terms of effectiveness, clinical applicability, and safety is still a big problem for most countries. Taking the urinary proteome as an example, the type and content of protein in urine are closely related to gender, age, race, and laboratory platform level and are affected by the test’s storage temperature and whether protease inhibitors are added. Therefore, the internal scientificity and external applicability of disease evaluation models based on AI still need to be further verified. In 2016, the Food and Drug Administration issued the first guideline for evaluating AI systems, which provides preliminary specifications and standards for AI products’ clinical application (52,53). The guidelines and specifications are still in their infancy and need to be further established and improved.
Furthermore, as medicine-related AI products are for human application, this will raise many ethical issues, including medical safety and responsibility attribution. Misdiagnosis and missed diagnosis by doctors can cause damage and risks to patients. When AI products cause medical accidents, how will responsibility be divided? Will regional differences in the application of AI products lead to medical inequality? When the conclusion of AI is inconsistent with that of doctors, will it increase patients' distrust of doctors and hospitals and worsen the doctor-patient relationship? These problems need to be solved in different cultural and social environments (54-58).
At present, the development of most AI products relies on a large amount of medical data. Through the collection and analysis of more and more extensive data, AI’s perandom forestsormance will further improve. These medical data involve patients’ basic information, such as name, gender, and age, and often involve privacy-sensitive information, such as past medical history, history of present illness, and family history. If the server or cloud storing this information is invaded, it will lead to patient information leakage and privacy breaches. Therefore, there is an unavoidable problem in applying AI in the medical field, that is, the contradiction between the efficiency of AI products and the privacy information of patients. If we want to improve AI’s efficiency, we will inevitably need more patient information, including sensitive and private information. If we want to fully respect and protect patients’ privacy, limiting the collection of their data will limit the effectiveness of AI products. Achieving a balance between the two is an ideal goal for the development of medical-related AI. However, this is a big challenge and is affected by disease types, national laws and regulations, and social cognition (59-61).
Conclusions
In summary, AI is conducive to making full use of the increasing amount of big data in medicine. In this article, we have demonstrated the use of AI in processing and analyzing urine detection data, such as urine proteins, urine metabolites, and urine RNA, which not only contributes to the early and accurate diagnosis of diseases but also provides new ideas for the non-invasive and simplified diagnosis of diseases. Currently, AI is still in the early stages of development, and urine-related AI is mostly used in the auxiliary diagnosis of urinary system diseases. The accuracy and specificity of AI for diagnosis need to be further improved. However, with the rapid developments in computer technology and medicine, AI combined with urine detection is expected to become another important means of disease diagnosis and treatment and is also expected to be more widely used in the early diagnosis, treatment, and follow-up monitoring of various diseases.
Acknowledgments
Funding: This work was supported by the Sichuan Science and Technology Program (2018TJPT0018), Chengdu Science and Technology Program (2019-YFYF-00087-SN), and the 1-3-5 project for disciplines of excellence-Clinical Research Incubation Project, West China Hospital of Sichuan University (2019HXFH015).
Footnote
Reporting Checklist: The authors have completed the Narrative Review reporting checklist. Available at http://dx.doi.org/10.21037/tau-20-1405
Conflicts of Interest: All authors have completed the ICMJE uniform disclosure form (available at http://dx.doi.org/10.21037/tau-20-1405). FQ and JY have the patent Portable Urine Recorder issued. The other authors have no conflicts of interest to declare.
Ethical Statement: The authors are accountable for all aspects of the work in ensuring that questions related to the accuracy or integrity of any part of the work are appropriately investigated and resolved.
Open Access Statement: This is an Open Access article distributed in accordance with the Creative Commons Attribution-NonCommercial-NoDerivs 4.0 International License (CC BY-NC-ND 4.0), which permits the non-commercial replication and distribution of the article with the strict proviso that no changes or edits are made and the original work is properly cited (including links to both the formal publication through the relevant DOI and the license). See: https://creativecommons.org/licenses/by-nc-nd/4.0/.
References
- Miller DD, Brown EW. Artificial Intelligence in Medical Practice: The Question to the Answer? Am J Med 2018;131:129-33. [Crossref] [PubMed]
- Chen J, Remulla D, Nguyen JH, et al. Current status of artificial intelligence applications in urology and their potential to influence clinical practice. BJU Int 2019;126:647. [Crossref] [PubMed]
- Goldenberg SL, Nir G, Salcudean SE. A new era: artificial intelligence and machine learning in prostate cancer. Nat Rev Urol 2019;16:391-403. [Crossref] [PubMed]
- Qin F, Yuan J. Research status and trend of artificial intelligence in the diagnosis of urinary diseases. Sheng Wu Yi Xue Gong Cheng Xue Za Zhi 2020;37:230-5. [PubMed]
- Ngiam KY, Khor IW. Big data and machine learning algorithms for health-care delivery. Lancet Oncol 2019;20:e262-73. [Crossref] [PubMed]
- Echeverry G, Hortin GL, Rai AJ. Introduction to urinalysis: historical perspectives and clinical application. Methods Mol Biol 2010;641:1-12. [Crossref] [PubMed]
- Rossing K, Bosselmann HS, Gustafsson F, et al. Urinary Proteomics Pilot Study for Biomarker Discovery and Diagnosis in Heart Failure with Reduced Ejection Fraction. PLoS One 2016;11:e0157167 [Crossref] [PubMed]
- Zhang ZY, Ravassa S, Nkuipou-Kenfack E, et al. Novel Urinary Peptidomic Classifier Predicts Incident Heart Failure. J Am Heart Assoc 2017;6:e005432 [Crossref] [PubMed]
- von Zur Mühlen C, Koeck T, Schiffer E, et al. Urine proteome analysis as a discovery tool in patients with deep vein thrombosis and pulmonary embolism. Proteomics Clin Appl 2016;10:574-84. [Crossref] [PubMed]
- Zhang C, Leng W, Sun C, et al. Urine Proteome Profiling Predicts Lung Cancer from Control Cases and Other Tumors. EBioMedicine 2018;30:120-8. [Crossref] [PubMed]
- Nakajima T, Katsumata K, Kuwabara H, et al. Urinary Polyamine Biomarker Panels with Machine-Learning Differentiated Colorectal Cancers, Benign Disease, and Healthy Controls. Int J Mol Sci 2018;19:756. [Crossref] [PubMed]
- Roux-Dalvai F, Gotti C, Leclercq M, et al. Fast and Accurate Bacterial Species Identification in Urine Specimens Using LC-MS/MS Mass Spectrometry and Machine Learning. Mol Cell Proteomics 2019;18:2492-505. [Crossref] [PubMed]
- Eisner R, Greiner R, Tso V, et al. A machine-learned predictor of colonic polyps based on urinary metabolomics. Biomed Res Int 2013;2013:303982 [Crossref] [PubMed]
- Shao CH, Chen C, Lin J, et al. Metabolite marker discovery for the detection of bladder cancer by comparative metabolomics. Oncotarget 2017;8:38802-10. [Crossref] [PubMed]
- Kouznetsova VL, Kim E, Romm EL, et al. Recognition of early and late stages of bladder cancer using metabolites and machine learning. Metabolomics 2019;15:94. [Crossref] [PubMed]
- Caudarella R, Tonello L, Rizzoli E, et al. Predicting five-year recurrence rates of kidney stones: an artificial neural network model. Arch Ital Urol Androl 2011;83:14-9. [PubMed]
- Liang Q, Liu H, Wang C, et al. Phenotypic Characterization Analysis of Human Hepatocarcinoma by Urine Metabolomics Approach. Sci Rep 2016;6:19763. [Crossref] [PubMed]
- Dykstra MA, Switzer N, Eisner R, et al. Urine metabolomics as a predictor of patient tolerance and response to adjuvant chemotherapy in colorectal cancer. Mol Clin Oncol 2017;7:767-70. [Crossref] [PubMed]
- Martinez-Vernon AS, Covington JA, Arasaradnam RP, et al. An improved machine learning pipeline for urinary volatiles disease detection: Diagnosing diabetes. PLoS One 2018;13:e0204425 [Crossref] [PubMed]
- Sapre N, Macintyre G, Clarkson M, et al. A urinary microRNA signature can predict the presence of bladder urothelial carcinoma in patients undergoing surveillance. Br J Cancer 2016;114:454-62. [Crossref] [PubMed]
- Connell SP, Hanna M, McCarthy F, et al. A Four-Group Urine Risk Classifier for Predicting Outcome in Prostate Cancer Patients. BJU Int 2019;124:609-20. [Crossref] [PubMed]
- Sanghvi AB, Allen EZ, Callenberg KM, et al. Perandom forestsormance of an artificial intelligence algorithm for reporting urine cytopathology. Cancer Cytopathol 2019;127:658-66. [Crossref] [PubMed]
- Muralidaran C, Dey P, Nijhawan R, et al. Artificial neural network in diagnosis of urothelial cell carcinoma in urine cytology. Diagn Cytopathol 2015;43:443-9. [Crossref] [PubMed]
- Heckerling PS, Canaris GJ, Flach SD, et al. Predictors of urinary tract infection based on artificial neural networks and genetic algorithms. Int J Med Inform 2007;76:289-96. [Crossref] [PubMed]
- Jain S, Lin S, Song W, et al. Urine-Based Liquid Biopsy for Nonurological Cancers. Genet Test Mol Biomarkers 2019;23:277-83. [Crossref] [PubMed]
- Wu J, Gao Y. Physiological conditions can be reflected in human urine proteome and metabolome. Expert Rev Proteomics 2015;12:623-36. [Crossref] [PubMed]
- Bini SA. Artificial Intelligence, Machine Learning, Deep Learning, and Cognitive Computing: What Do These Terms Mean and How Will They Impact Health Care? J Arthroplasty 2018;33:2358-61. [Crossref] [PubMed]
- El-Hassoun O, Maruscakova L, Valaskova Z, et al. Artificial intelligence in service of medicine. Bratisl Lek Listy 2019;120:218-22. [PubMed]
- Popp KH, Kosilek RP, Frohner R, et al. Computer Vision Technology in the Differential Diagnosis of Cushing's Syndrome. Exp Clin Endocrinol Diabetes 2019;127:685-90. [Crossref] [PubMed]
- Zhou Y, Xu X, Song L, et al. The application of artificial intelligence and radiomics in lung cancer. Jin Zhun Lin Chuang Yi Xue 2020;3:214-27. </jrn>.
- Kermany DS, Goldbaum M, Cai W, et al. Identifying Medical Diagnoses and Treatable Diseases by Image-Based Deep Learning. Cell 2018;172:1122-31.e9. [Crossref] [PubMed]
- Liang H, Tsui BY, Ni H, et al. Evaluation and accurate diagnoses of pediatric diseases using artificial intelligence. Nat Med 2019;25:433-8. [Crossref] [PubMed]
- Handelman GS, Kok HK, Chandra RV, et al. eDoctor: machine learning and the future of medicine. J Intern Med 2018;284:603-19. [Crossref] [PubMed]
- Fralick M, Colak E, Mamdani M. Machine Learning in Medicine. N Engl J Med 2019;380:2588-9. [Crossref] [PubMed]
- Li X, Ding X. Survey on Five Tribes of Machine Learning and the Main Algorithms. Ruan Jian Dao Kan 2019;18:4-9.
- Bi Q, Goodman KE, Kaminsky J, et al. What is Machine Learning? A Primer for the Epidemiologist. Am J Epidemiol 2019;188:2222-39. [Crossref] [PubMed]
- Vaidyanathan K. Urinary proteomics and metabolomics in the diagnosis of pediatric disorders. Proteomics Clin Appl 2015;9:482-9. [Crossref] [PubMed]
- Di Meo A, Batruch I, Yousef AG, et al. An integrated proteomic and peptidomic assessment of the normal human urinome. Clin Chem Lab Med 2017;55:237-47. [Crossref] [PubMed]
- Fliser D, Novak J, Thongboonkerd V, et al. Advances in urinary proteome analysis and biomarker discovery. J Am Soc Nephrol 2007;18:1057-71. [Crossref] [PubMed]
- Wu J, Gao Y. Physiological conditions can be reflected in human urine proteome and metabolome. Expert Rev Proteomics 2015;12:623-36. [Crossref] [PubMed]
- Feng J. Recent advances of metabolomics techniques for diagnosis and treatment of kidney disease. Zhong Guo Jian Yan Yi Xue Za Zhi 2018;41:246-50.
- Chen P, Chen B. Application of Metabolomics Technology in Precision Medicine. Hang Tian Yi Xue Yu Yi Xue Gong Cheng 2016;29:144-9.
- Acunzo M, Romano G, Wernicke D, et al. MicroRNA and cancer--a brief overview. Adv Biol Regul 2015;57:1-9. [Crossref] [PubMed]
- Piletič K, Kunej T. MicroRNA epigenetic signatures in human disease. Arch Toxicol 2016;90:2405-19. [Crossref] [PubMed]
- Gaston KE, Pruthi RS. Value of urinary cytology in the diagnosis and management of urinary tract malignancies. Urology 2004;63:1009-16. [Crossref] [PubMed]
- Sternberg I, Rona R, Olsfanger S, et al. The clinical significance of class III (suspicious) urine cytology. Cytopathology 2011;22:329-33. [Crossref] [PubMed]
- Qin Y, Sun J. Chen pin. Urine cells image recognition research based on support vector machine. Ji Suan Ji Gong Cheng Yu She Ji 2013;34:2185-9.
- Xiong Y, Zhang Y, Li X, et al. The prevalence and associated factors of lower urinary tract symptoms suggestive of benign prostatic hyperplasia in aging males. Aging Male 2020;25:1-8. [Crossref] [PubMed]
- Gacci M, Corona G, Sebastianelli A, et al. Male Lower Urinary Tract Symptoms and Cardiovascular Events: A Systematic Review and Meta-analysis. Eur Urol 2016;70:788-96. [Crossref] [PubMed]
- Yuan J, Qin F. Portable urine recorder: China, 201610006224. 2. 2018.12.11.
- Qi R, Lv W. The role and challenges of artificial intelligence-assisted diagnosis technology in the medical field. Zhong Guo Yi Liao Qi Xie Xin Xi 2018;24:27-8.
- Graham J. Artificial intelligence, machine learning, and the FDA. Accessed 2019;10: 25.
- He J, Baxter SL, Xu J, et al. The practical implementation of artificial intelligence technologies in medicine. Nat Med 2019;25:30-6. [Crossref] [PubMed]
- Zhang R, Xu F. Research on the Ethical issues of Artificial Intelligence Medicine and Its Countermeasures. Yi Xue Yu Zhe Xue 2020;41:14-9.
- Wang S, Cui X. Ethical Issues of Applications of Artificial Intelligence in Medicine. Zhong Guo Yi Xue Lun Li Xue 2019;32:972-6.
- Li M, Li Y, Dai L, et al. Ethical Issues of Medical Artificial Intelligence. Yi Xue Yu Zhe Xue 2019;40:1-4.
- Keskinbora KH. Medical ethics considerations on artificial intelligence. J Clin Neurosci 2019;64:277-82. [Crossref] [PubMed]
- Song F. Problems and Countermeasures of Regional Medical Big Data Construction. Xin Xi Yu Dian Nao 2020;32:28-30.
- Kayaalp M. Patient Privacy in the Era of Big Data. Balkan Med J 2018;35:8-17. [Crossref] [PubMed]
- Price WN 2nd, Cohen IG. Privacy in the age of medical big data. Nat Med 2019;25:37-43. [Crossref] [PubMed]
- Véliz C. Medical privacy and big data: A further reason in favour of public universal healthcare coverage. Oxford (UK): Oxford University Press, 2019.