Identification and validation of a five apoptosis-related genes signature for prediction of disease-free survival for testicular germ cell tumors
Introduction
Testicular cancer is a relatively rare cancer, but it is the most common solid malignancy of young men aged 15–34 years old, accounting for about 1–1.5% of male tumors (1). Its incidence has shown an upward trend in the past 20 years (2). It mainly includes three categories: germ cell tumors, sex cord/gonadal stromal tumors, and other non-specific stromal tumors. Testicular germ cell tumors (TGCT) account for 90–95% of all testicular cancers, and histologically they are divided into seminoma and non-seminoma germ cell tumors (NSGCT) (3). The cure rate of low- and medium-risk TGCT is very good, which mainly depends on early diagnosis, correct clinical judgment, early treatment, and tumor sensitivity to radiotherapy and chemotherapy (4). Currently, treatment decisions for TGCT patients are still based on traditional serum tumor marker levels and TNM classification (5). At present, serum tumor markers used for the diagnosis and stratification of TGCT include alpha-fetoprotein (AFP), human chorionic gonadotropin (HCG), and lactate dehydrogenase (LDH). During the course of the disease, approximately 90% of NSGCT show an increase in one or two serum markers, but only 30% of seminoma show an increase in HCG (6). Moreover, these markers have poor specificity for the follow-up and monitoring of advanced TGCT, and cannot accurately reflect the progression of the disease (7). Therefore, we believe that in the treatment of TGCT, clinicians need better biomarkers.
Apoptosis is a form of programmed cell death, which is regulated by genes. Studies have shown that apoptosis plays an important role in the occurrence and development of various tumors. In the process of cancer, the balance between cell division and cell death is lost, and abnormal cells that should have died do not receive the signal of apoptosis (8). In the process of apoptosis, caspases are activated, leading to the destruction of DNA and proteins in the cell, and changes in the cell membrane are recognized and swallowed by phagocytes (9). DNA damage-induced apoptosis can eliminate harmful cells and hinder the growth of tumors, and the release of this process can lead to an uninhibited proliferation of cells, which is related to the development of cancer and the resistance of cancer to drug treatment (10). Relief of apoptosis is considered to be one of the characteristics of cancer and can become one of the targets of tumor treatment (11). Selective induction of cancer cell death is one of the most effective methods to treat cancer (12,13). Apoptosis is the most common selective induction of cell death and plays a key role in tumorigenesis. Testicular cancer cells usually have a low apoptosis threshold and are easily induced by treatment including chemotherapy to cause apoptosis.
In this study, we first downloaded the mRNA expression profile and corresponding clinical data of TCGT patients from public databases. Then, we constructed a prognostic model based on ARGs in the TCGT of the TCGA data set and validated it in the GEO cohort. Finally, we constructed a nomogram to predict the prognosis of TCGT patients. We present the following article in accordance with the TRIPOD reporting checklist (available at http://dx.doi.org/10.21037/tau-20-1247).
Methods
Public data source
The RNA sequence data of 156 patients with TGCT were retrieved from the TCGA database (https://portal.gdc.cancer.gov/). The data of 165 normal tissue samples from healthy individuals came from the GTEx database (https://gtexportal.org/home/datasets). All the raw data were preprocessed using the “Limma” software package of the R software, using |log2 fold change (FC)|>1 and false discovery rate (FDR) less than 0.05 as the criteria for screening differentially expressed ARG. Also, two gene expression profiles (GSE3218 and GSE10783) were downloaded from the gene expression Omnibus (GEO) database (https://www.ncbi.nlm.nih.gov/geo/) were used as an external verification cohort. The GSE3218 data set includes 99 TCGT samples, and the GSE10783 data set includes 34 TCGT samples. After excluding samples with incomplete clinical information, 108 samples from GSE3218 and GSE10783 were used as an independent external validation cohort. “Limma” package was used for screening the differentially expressed genes (DEGs) between cancer samples and normal control samples.
Construction and verification of the prognostic model
The 132 eligible TCGT patients with clinical survival time in the TCGA database were randomly divided into two groups at a ratio of 7:3, which were divided into a training group and test group. The data of the training group was used to construct the Cox regression model of DFS, and the test group was used to verify the accuracy. First, univariate Cox regression analysis was used to select potential genes related to prognosis. Use Lasso regression analysis to avoid overfitting. Finally, multi-factor Cox proportional hazards regression was used to constructing a DFS prognostic risk model, and the regression coefficient of a single gene and the expression value of the selected gene was calculated to calculate the risk score of each patient. The formula for estimating the risk score (RS) is as follows:
Using the median of the risk score of the TCGA training group as the threshold, eligible patients were divided into high- and low-risk groups, and Kaplan-Meier survival curves were drawn to compare the prognostic differences between the two groups. In addition, we carefully evaluated the signatures we built in 3 verification sets, including the external verification data set (GEO), the TCGA internal verification data set, and the whole TCGA data set. The “glmnet”, “survival” and “survminer” package in R was used to construct a prognostic prediction model. The “survival”, “survminer” and “survivalROC” package in R was used to carry out Kaplan-Meier and ROC curve.
Construction of a prognostic nomogram
Several clinicopathological parameters and the risk score were used to construct the prognostic nomogram, which was used to evaluate the 1-, 3-, and 5-year DFS of TGCT. The accuracy of the nomogram was quantitatively evaluated with the area under curve (AUC) of the receiver operating characteristic curve (ROC). Calibration charts are also used to intuitively assess the prognostic ability of nomograms. The “rms”, “foreign” and “survival” package in R was used to establish and validate a nomogram.
Functional analysis
The biological functions of DEGs were comprehensively analyzed through GO enrichment and Kyoto encyclopedia of genes and genome (KEGG) pathway analysis. Gene ontology analysis includes cellular components (CC), molecular functions (MF), and biological processes (BP). FDR <0.05 and |log2FC|>1 was used as the threshold. We also conducted the Gene Set Enrichment Analysis (GSEA) to study the related functions of TGCT. It had been considered to be significantly enriched that normalized P value less than 0.05 and FDR less than 0.25.
RNA extraction and qRT-PCR
The study was conducted in accordance with the Declaration of Helsinki (as revised in 2013). The study was approved by ethics committee of First Affiliated Hospital of Nanjing Medical University (NO. 2021-SR-071) and informed consent was taken from all the patients. In our study, five patients with TGCT were enrolled, and their adjacent normal tissues was considered as normal tissue to be used as control. Their tumor tissues were used for RNA isolation. Ethical approval was obtained from the ethics committee of the cancer hospital and informed written consent was obtained from all of subjects. The total RNA from TGCT tissues and adjacent normal tissues was acquired using TRIzol Reagent (Invitrogen, Carlsbad, CA, USA) on the basis of a standard extraction protocol. The cDNA was synthesized by HiScript II (Vazyme, China), and qPCR analysis was performed on StepOne Plus Real-Time PCR system (Applied Biosystems, USA). The initial reaction was incubated at 95 °C for 10 min, followed by 40 cycles of 95 °C for 15 s and 60 °C for 1 min in accordance with the SYBR green method. The relative expression levels were analyzed by comparing 2−ΔΔCT values. All reactions were carried out in triplicate. Primers were synthesized by TSINGKE (Beijing, China). Quantitative realtime PCR (qRT-PCR) was performed to amplify cDNA with specific primers (CHGA: 5'-TAAAGGGGATACCGAGGTGATG-3' and 5'-TCGGAGTGTCTCAAAACATTCC-3', LPCAT1: 5'-CGCCTCACTCGTCCTACTTC-3' and 5'-TTCCCCAGATCGGGATGTCTC-3', PPP1CA: 5'-ACTACGACCTTCTGCGACTAT-3' and 5'-AGTTCTCGGGGTACTTGATCTT-3', PSMB5: 5'-AGGAACGCATCTCTGTAGCAG-3' and 5'-AGGGCCTCTCTTATCCCAGC-3', UBR2: 5'-GTACCAGCATTTAGCCCACTATG-3' and 5'-TGCAAGAATATGTAGGCTCTCCT-3') and the data were normalized to GAPDH (5'-TGTGGGCATCAATGGATTTGG-3' and 5'-ACACCATGTATTCCGGGTCAAT-3').
Statistical analysis
All statistical analyses were performed using R software 3.6.3, and P<0.05 was considered statistically significant. Categorical variables use the chi-square test or Fisher’s exact test to evaluate variables. Kaplan-Meier survival curve was used to analyze DFS. Use the Cox proportional hazards regression model for univariate and multivariate analysis. Time-dependent ROC analysis is used to evaluate the accuracy of models that predict prognosis. ROC curve analysis is also used to estimate the diagnostic value of gene expression.
Results
Identification of differently expressed ARGs
The RNA sequencing data of 156 TCGT tumor samples from The Cancer Genome Atlas (TCGA) database and 165 normal testicular tissues from the Genotype-Tissue Expression (GTEx) were included in the analysis. According to the “Limma” package, set the FDR to <0.05 and |log2(FC)|>1 as the cut-off criterion. From the 1,528 ARG list, 723 ARGs with different expressions were screened out, including 223 down-regulated ARGs and 500 up-regulated ARG. Figure 1A,B show their expression heat map and volcano map.
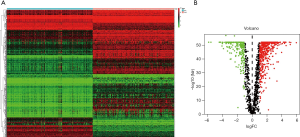
Construction of prognosis-related genetic risk score model in the TCGA training cohort and validation of the risk score in the TCGA testing cohort
The entire TCGA TGCT patients were randomly divided into two groups. Ninety six of 132 patients were selected as a training cohort and 36 cases were classified into a testing cohort. Univariate Cox regression analysis was used to evaluate the relationship between apoptosis-related genes (ARGs) and DFS in the TCGA training cohort, and 14 candidate genes were screened (Figure 2A). To further refine the model to avoid model overfitting, we performed a least absolute shrinkage and selection operator (LASSO) regression analysis on 14 prognostic-related genes and obtained ten genes (Figure 2B,C). Finally, we performed multivariate Cox regression analysis on the remaining ten genes, and finally obtained five prognostic-related genes, including CHGA, LPCAT1, PPP1CA, PSMB5, and UBR2 (Figure 2D). CHGA, PPP1CA, and PSMB5 with HR values greater than 1 are considered risk genes LPCAT1 and UBR2 with HR values less than 1 are thought to be protective genes. Based on the expression levels of these five genes, we constructed a risk score, which is calculated by the partial regression coefficient multiplied by the expression of each gene, as follows:
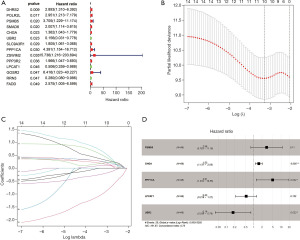
To verify the predictive ability of this risk score on the prognosis of TCGT patients, patients in the TCGA training group were divided into high-risk and low-risk groups according to the median value of the risk score. The distribution of the recurrence status of patients showed that the proportion of tumor recurrence in the high-risk group was higher than that in the low-risk group (Figure 3B,C). The results of Kaplan-Meier survival analysis also proved this point, that the high-risk group has poor DFS (P=0.02268, Figure 3D). The time-dependent ROC curve was used to estimate the sensitivity and specificity of the prediction results. The results showed that the AUC values of the 1-, 2-, 3-, and 5-year DFS were 0.780, 0.767, 0.767, and 0.731, respectively (Figure 3E,F,G). It demonstrated that the prediction effect was good. To verify this result, we conducted verification in the TCGA testing cohort. Based on the prognostic model we made, the survival status of TGCT patients revealed that as the risk score increased, the number of relapsing patients increased, which meant a worse prognosis (Figure 4A,B,C). Kaplan-Meier survival analysis showed that the DFS of the high-risk group was shorter than the low-risk group (P=0.0293e, Figure 4D). The 1-year (AUC =0.727, Figure 4E), 2-year (AUC =0.657, Figure 4F), 3-year (AUC =0.680, Figure 4G), and 5-year (AUC =0.680, Figure 4H) values of the TCGA testing cohort also indicate that the model has the better predictive ability.
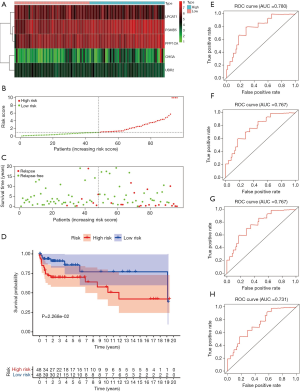
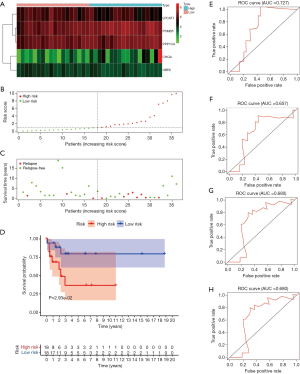
Validation of the risk scoring model in the entire TCGA cohort
We have proved that the risk score was valuable to predict the prognosis of TGCT in both the training group and testing group. Next, the validation of the model in the entire TCGA cohort was carried out. Heatmap of five key genes showing that expression of PPP1CA and PSMB5 was higher in the high-risk group and low-risk group, while CHGA, LPCAT1, and UBR2 seemed low-expressed in two groups (Figure 5A). The survival status of TGCT patients from the entire TCGA cohort indicated that as the risk score increased, the number of relapsing patients increased, which implied a worse outcome (Figure 5B,C). The Kaplan-Meier plots revealed that patients assigned to high-risk groups exhibited worse prognosis than in the low-risk group (P=0.002529, Figure 5D). Regarding gene IFIH1, we found that it had relatively lower expression in the high-risk group than in the low-risk group. A time-dependent ROC curve was constructed and AUC was 0.768 at 1 year, 0.733 at 2 years, 0.716 at 3 years, and 0.697 at 5 years (Figure 5E,F,G,H).
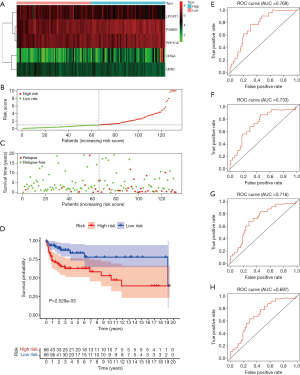
External validations of the six-gene risk score using the GEO database
From the GEO database, we downloaded the GSE3218 and GSE10783 datasets for validating the risk assessment formula once again. The risk score applied was consistent with the prognostic models we constructed previously. Next, survival status turned out that patients in high-risk cohorts had poorer OS than those in the low-risk cohort (Figure 6A,B,C). Meanwhile, the Kaplan-Meier curve testifying the predictive value of the prognostic model (P=1.794e-07, Figure 6D). The five-ARG risk score also showed great accuracy in predicting 1-year DFS (AUC =0.622, Figure 6E), 2-year DFS (AUC =0.682, Figure 6F), 3-year DFS (AUC =0.726, Figure 6G), and 5-year DFS (AUC =0.707, Figure 6H).
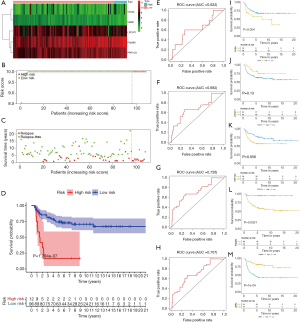
The Kaplan-Meier plots of the 5 Independent Prognostic ARGs were exhibited in Figure 6I,M. TGCT patients with high expression of LPCAT1, UBR2, and low expression of CHGA, PPP1CA, PSMB5 presented better prognosis, which was all statistically significant, indicating that these 5 genes could play a prognostic role in TGCT prognosis prediction.
Independent prognostic factor analysis
To find out whether several main clinicopathological characteristics (including age, lymphovascular invasion, serum tumor marker levels, stage, T stage, M stage, N stage, pathological type) and risk characteristics are independent predictors, we used univariate COX and multiple COX regression analysis (Figure 7A,B). The results showed that serum tumor marker levels (P<0.001) and risk scores (P<0.001) were significantly correlated with DFS (Figure 7B). The heat map reflecting expression levels of the five ARGs in the high- and low-risk subgroup patients in the TCGA cohort was presented in Figure 7C. Differences were observed for various clinicopathological parameters such as different pathological types (P<0.001) and serum tumor marker levels (P<0.01) between high and low-risk groups (Figure 7C). The ROC curve shows that the risk score predicts 1-year (AUC =0.759, Figure 7D), 3-year (AUC =0.712, Figure 7E) and5-year (AUC =0.712, Figure 7F) DFS survival rates are better than serum tumor marker levels, grades, age, tumor size, distant tumor metastasis, lymphovascular invasion, pathological type (Figure 7D,E,F,G). It is suggested that the risk score is one of the risk factors for patients with TGCT, and it can independently predict the prognosis of patients with TGCT.
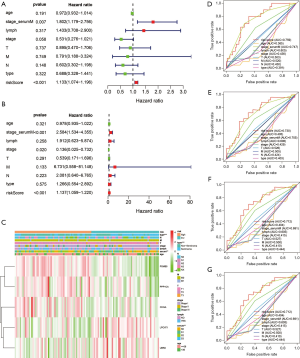
Clinicopathological parameters stratified by our established risk score
Based on our established signature, seven clinicopathological parameters containing age, Lymphovascular invasion, stage, T stage, M stage, N stage, pathological type were divided into high and low-risk subgroups. Our results indicated that our established risk score was capable of predicting DFS in age <35 (P=0.003), lymphovascular invasion negative (P=0.003), M0 (P<0.001), N0 (P=0.007), Serum S1-S3 (P=0.044), Stage I (P=0.003), T1 (P=0.004), Seminoma (P=0.007). On account of the P value being greater than 0.05, age >35 (P=0.739), lymphovascular invasion positive (P=0.288), M1 (P=0.275), N1-3 (P=0.420), SerumM S0 (P=0.352), Stage II-III (P=0.468) and non-Seminoma (P=0.295) had no significant difference in statistics. This may be caused by the too-small sample size (Figure 8).
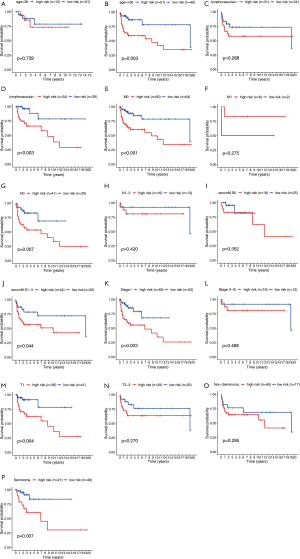
The box plot shows that compared with normal tissue samples, the expression of CHGA in tumor tissue samples is down-regulated, while the expression of LPCAT1, PPP1CA, PSMB5, and UBR2 is up-regulated (Figure 9A,B,C,D,E). Kaplan-Meier survival analysis separately assessed the relationship between the expression of 5 ARGs and the clinical outcome of patients with TCGT. The results showed that CHGA (P=0.0046), PPP1CA (P=0.0088), PSMB5 (P=0.0032) had poorer outcomes in the high expression group, while LPCAT1 (P=0.027), UBR2 (P=0.038) had poorer outcomes in the low expression group (Figure 9F,G,H,I,J). These results indicate that the expression of the five genes that constitute the risk score are all related to the prognosis of patients with TCGT.
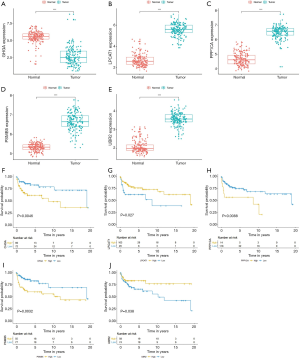
Construction of the nomogram based on the established signature and clinical characteristics
To establish a clinically applicable method for predicting the prognosis of TCGT patients, we established a prognostic nomogram using TCGA data to predict the 1-, 3-, and 5-year survival rates of TCGT patients (Figure 10A). Four prognostic parameters were included in the prediction model, including the risk score, age, pathological type, and tumor lymph node metastasis. The calibration chart (Figure 10B,C,D) shows that the 1-, 3-, and 5-year survival rates of the TCGA cohort predicted by the nomogram are in good agreement with the actual observations. The ROC curve was used to estimate the sensitivity and specificity of the nomogram, and the results showed that the AUC values of 1-, 3-, and 5-year DFS were 0.90, 0.806, and 0.806, respectively (Figure 10E,F,G).
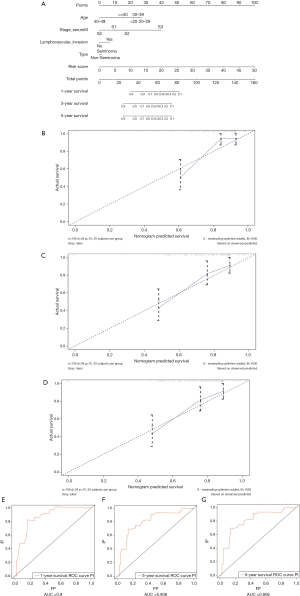
GO, KEGG and GSEA analysis of the differently expressed DEGs
Based on five ARGs risk-score, we divided 132 patients into high- and low-risk groups. 425 DEGs were identified based on the criteria that |log2FC|>1 along with FDR <0.05 (Figure 11A,B). We aimed to illuminate the biological functions and pathways related to DEGs between high- and low-risk score groups by performing GO and KEGG pathway enrichment analyses. As is illustrated in Figure 11C, GO enrichment analysis showed that in terms of BP, DEGs are mainly enriched in extracellular matrix organization, extracellular structure organization, ossification, gland development, transmembrane receptor protein serine/threonine kinase signaling pathway, gastrulation, response to BMP, Cellular response to BMP stimulus, regulation of humoral immune response, regulation of complement activation. In terms of cell components (CC), DEGs are mainly enriched in the collagen−containing extracellular matrix, endoplasmic reticulum lumen, immunoglobulin complex, blood microparticle, collagen trimer, Golgi lumen, basement membrane, fibrillar collagen trimer, banded collagen fibril, the complex of collagen trimers. In terms of MF, DEGs are mainly enriched in receptor-ligand activity, signaling receptor activator activity, extracellular matrix structural constituent, growth factor activity, glycosaminoglycan binding, antigen binding, heparin-binding, integrin binding, growth factor binding, platelet−derived growth factor binding. KEGG pathway analysis showed that DEGs were significantly enriched in the PI3K-Akt signaling pathway, proteoglycans in cancer, focal adhesion, TGF-β signaling pathway, Wnt signaling pathway, and other signaling pathways (Figure 11D).
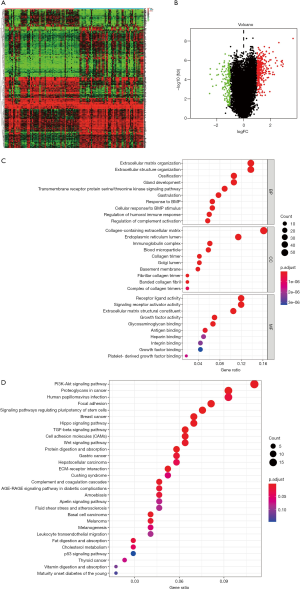
To further investigate the possible mechanisms leading to different outcomes in the high- and the low-risk group, we did GSEA based on the patients in two groups (Figure 12A,B,C,D,E,F,G). “adipogenesis”, “angiogenesis”, “cholesterol homeostasis”, “glycolysis”, “estrogen response late”, “hypoxia”, and “xenobiotic metabolism” were enriched pathways in the high-risk group.
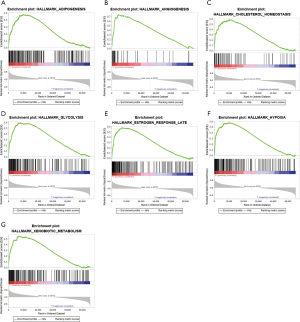
qRT-PCR assays
We collected the TCGT tissues and adjacent normal tissues from 5 tumor patients, and qRT-PCR was used subsequently to verify the expression patterns of these five genes. The results showed that the expression of CHGA in tumor tissues decreased, and the expression of PSMB5 and UBR2 increased. The difference was statistically significant (Figure 13, P<0.05). The mRNA expression of LPCAT1 in the tumor was higher than that in the adjacent tissues, but the difference was not statistically significant. There was no significant difference in the expression of PPP1CA between the two groups. It may be necessary to collect more samples for verification.
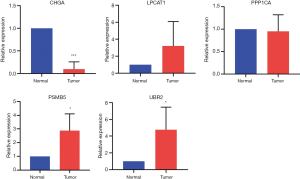
Discussion
During the occurrence of cancer, normal cells are transformed into malignant cells, and avoidance of cell death is one of the important changes in cells that cause this malignant transformation. In the 1970s, Kerr et al. linked apoptosis to the elimination, proliferation, and tumor progression of potentially malignant cells (14). There are many ways for malignant cells to escape apoptosis, including the balance between pro-apoptosis and anti-apoptotic proteins are disrupted, the function of caspase is reduced, and the death receptor signaling pathway is damaged. Chemotherapeutics play a key role in the treatment of testicular cancer. A large amount of evidence shows that there is a correlation between the sensitivity to apoptosis and chemotherapy response, and the sensitivity of different cell types to induce apoptosis varies greatly (12).
In this study, we combined the TCGA and GTEx databases to screen for differentially expressed ARG between tumor and normal tissues, and performed univariate Cox regression analysis, LASSO regression analysis, and multiple Cox regression analysis on DE-ARG, and screened five ARGs related to prognosis (CHGA, LPCAT1, PPP1CA, PSMB5, UBR2). Finally, based on five ARGs related to prognosis, we constructed a risk model to predict the prognosis of testicular cancer patients. Some studies have shown that these ARGs play an important role in tumorigenesis. Studies have shown that CHGA can be used as a potential biomarker for a variety of tumors including prostate cancer, small cell lung cancer, and colon cancer (15-17). According to reports, lysophosphatidylcholine acyltransferase 1 (LPCAT1) is related to the progression, metastasis, and recurrence of a variety of malignant tumors. LPCAT1 is a key membrane lipid remodeling enzyme. LPCAT1 is an important link between signal transduction and tumor growth, and lipid remodeling is one of the therapeutic targets of cancer (18). Studies by Du et al. showed that LPCAT1 expression in clear cell renal cell carcinoma (ccRCC) tissue is up-regulated, and is associated with unfavorable pathological features (higher tumor grade, higher TNM stage, and larger tumor size) and overall survival rate (19). LPCAT1 knockdown can inhibit the proliferation, migration, and invasion of kidney cancer cells, and induce cell cycle arrest in the G0/G1 phase. Overexpression of LPCAT1 may promote the development and progress of ccRCC by converting lysophosphatidylcholine (LPC) to phosphatidylcholine (PC) (19). Sun et al. found that PPP1CA can promote tumor growth and metastasis in colorectal cancer CRC through the ERK/MAPK pathway (20). Another study pointed out that the increased expression of PPP1CA in metastatic prostate cancer can activate the S6K/PP1α/B-Raf signaling pathway, thereby further activating MAPK signaling, which is antagonized by PML tumor inhibitors (21). The proteasome β subunit (PSMB) family is a component of the ubiquitin-proteasome system and has been demonstrated to play an important role in tumor cells and immune cells. High expression of PSMB5 was observed in breast cancer tissues, and high expression of PSMB5 predicted a poor survival rate. Knockdown of the PSMB5 gene can inhibit tumor cell growth and migration and can activate defensive M1 macrophages (22). Mo et al. found that PSMB5 knockdown can increase the sensitivity of multiple myeloma (MM) cells to bortezomib by activating apoptosis signals (23). PSMB5 may be a potential therapeutic target for MM. UBR2 is the only known E3 ubiquitin ligase in the N-end rule pathway, which can be upregulated by cachexia stimuli such as pro-inflammatory cytokines and tumors. The p38βMAPK-C/EBPβ signaling pathway can mediate the up-regulation of UBR2, which plays an important role in mediating muscle protein degradation in cancer cachexia (24). Studies have shown that UBR2 can promote the growth and metastasis of gastric cancer through the Wnt/β-Catenin pathway (25).
The current TNM staging and serum tumor markers are not sufficient to accurately predict the prognosis of TCGT patients. Studies have demonstrated that the number of embryonic carcinomas in tumors and lymphatic vascular invasion can be used as risk stratification factors for the prognosis of TGCT patients (26). The presence of non-pulmonary metastases (NPVM) and higher marker levels may represent tumor aggressiveness and tumor burden (27). In recent years, people have been devoted to identifying and verifying new markers to better predict TGCTs. Placental alkaline phosphatase (PLAP) is a membrane-bound protein expressed in fetal germ cells. In some studies, PLAP showed better sensitivity, specificity, and accuracy than AFP, hCG, and LDH (28). Fifty percent of seminoma have elevated PLAP levels and are an optional biomarker for monitoring patients with seminoma (29). TRA-1-60 is a cell surface antigen that expresses cancer and carcinoma in situ in the testis through embryos. In the research of Lajer and colleagues, about 80% of patients with advanced embryonic cancer had an increased expression level of TRA-1-60, and this level reduced by chemotherapy (30). Studies have shown that neuro-specific enolase (NSE), an isoenzyme of glycolytic enzyme 2-phospho-D-glycerate-hydrolase, is significantly elevated in patients with seminoma (especially in the metastatic stage), and it has been proposed as a potential new marker in this situation (31,32). A statistical model composed of multiple related genes is more accurate than using a single biomarker in assessing the prognosis of tumor patients, so it has been extensively used in research. There is not any relevant research on ARGs predicting the prognosis of testicular tumors. We developed a new prognostic signature based on the expression of five ARGs. The KM and ROC curves show that the risk score can easily distinguish different DFS. Besides, the results were verified in the GEO external verification data set. According to this risk scoring model, patients with testicular tumors are divided into high- and low-risk groups. The DFS of patients with a high-risk score was significantly lower than that of patients with a low-risk score. Besides, we combined the risk score and a variety of clinical factors to construct a nomogram to predict the DFS of patients with testicular cancer at 1, 3, and 5 years. The correction chart based on the TCGA database shows that the predicted value is very close to the observed value, indicating that the prediction performance of the nomogram is very good. Therefore, our new prognostic nomogram may be better than the original clinical parameters to help clinicians predict the survival status of TGCTs patients and provide specific individualized treatment.
As far as we know, this is the first nomogram to predict DFS in testicular cancer patients based on apoptosis-related signals. We successfully established a risk signature based on ARG and verified it. The results showed that it remained stable internally and externally. Of course, this study also has certain limitations. First, the clinical information downloaded from the TCGA and GEO database is incomplete. Secondly, our research is retrospective, and the predictive model has not been validated in large-scale clinical trials and prospective studies.
Conclusions
A total of 5 ARGs were screened, including CHGA, LPCAT1, PPP1CA, PSMB5, and UBR2, which were used to establish a new signature and successfully verified the signature internally and externally to predict the DFS of testicular tumor patients. It can help clinicians better and more intuitively predict the survival of patients. As an independent prognostic factor, the signatures we determined show a good predictive effect on DFS in patients with testes, and further studies are needed to verify our findings.
Acknowledgments
We would like to thank the researchers and study participants for their contributions.
Funding: This article was funded by the National Natural Science Foundation of China [grant number: 81871151; 81801438] and Medical key talent of Jiangsu Province: ZDRCA2016009.
Footnote
Reporting Checklist: The authors have completed the TRIPOD reporting checklist. Available at http://dx.doi.org/10.21037/tau-20-1247
Data Sharing Statement: Available at http://dx.doi.org/10.21037/tau-20-1247
Peer Review File: Available at http://dx.doi.org/10.21037/tau-20-1247
Conflicts of Interest: All authors have completed the ICMJE uniform disclosure form (available at http://dx.doi.org/10.21037/tau-20-1247). The authors have no conflicts of interest to declare.
Ethical Statement: The authors are accountable for all aspects of the work in ensuring that questions related to the accuracy or integrity of any part of the work are appropriately investigated and resolved. The study was conducted in accordance with the Declaration of Helsinki (as revised in 2013). The study was approved by ethics committee of First Affiliated Hospital of Nanjing Medical University (NO. 2021-SR-071) and informed consent was taken from all the patients.
Open Access Statement: This is an Open Access article distributed in accordance with the Creative Commons Attribution-NonCommercial-NoDerivs 4.0 International License (CC BY-NC-ND 4.0), which permits the non-commercial replication and distribution of the article with the strict proviso that no changes or edits are made and the original work is properly cited (including links to both the formal publication through the relevant DOI and the license). See: https://creativecommons.org/licenses/by-nc-nd/4.0/.
References
- Albers P, Albrecht W, Algaba F, et al. Guidelines on Testicular Cancer: 2015 Update. Eur Urol 2015;68:1054-68. [Crossref] [PubMed]
- Siegel RL, Miller KD, Jemal A. Cancer statistics, 2018. CA Cancer J Clin 2018;68:7-30. [Crossref] [PubMed]
- Thong AE, Lichtensztajn DY, Almario L, et al. Stage I testicular seminoma: a SEER analysis of contemporary adjuvant radiotherapy trends. J Urol 2013;190:1240-4. [Crossref] [PubMed]
- Boyle P. Testicular cancer: the challenge for cancer control. Lancet Oncol 2004;5:56-61. [Crossref] [PubMed]
- O'Sullivan B, Brierley J, Byrd D, et al. The TNM classification of malignant tumours-towards common understanding and reasonable expectations. Lancet Oncol 2017;18:849-51. [Crossref] [PubMed]
- Gilligan TD, Seidenfeld J, Basch EM, et al. American Society of Clinical Oncology Clinical Practice Guideline on uses of serum tumor markers in adult males with germ cell tumors. J Clin Oncol 2010;28:3388-404. [Crossref] [PubMed]
- Angulo JC, González J, Rodríguez N, et al. Clinicopathological study of regressed testicular tumors (apparent extragonadal germ cell neoplasms). J Urol 2009;182:2303-10. [Crossref] [PubMed]
- Wong RS. Apoptosis in cancer: from pathogenesis to treatment. J Exp Clin Cancer Res 2011;30:87. [Crossref] [PubMed]
- Kawai Y, Shibata K, Sakata J, et al. KIF20A expression as a prognostic indicator and its possible involvement in the proliferation of ovarian clear-cell carcinoma cells. Oncol Rep 2018;40:195-205. [Crossref] [PubMed]
- Fulda S. Tumor resistance to apoptosis. Int J Cancer 2009;124:511-5. [Crossref] [PubMed]
- Plati J, Bucur O, Khosravi-Far R. Dysregulation of apoptotic signaling in cancer: molecular mechanisms and therapeutic opportunities. J Cell Biochem 2008;104:1124-49. [Crossref] [PubMed]
- Fisher DE. Apoptosis in cancer therapy: crossing the threshold. Cell 1994;78:539-42. [Crossref] [PubMed]
- Huddart RA, Titley J, Robertson D, et al. Programmed cell death in response to chemotherapeutic agents in human germ cell tumour lines. Eur J Cancer 1995;31A:739-46. [Crossref] [PubMed]
- Kerr JF, Wyllie AH, Currie AR. Apoptosis: a basic biological phenomenon with wide-ranging implications in tissue kinetics. Br J Cancer 1972;26:239-57. [Crossref] [PubMed]
- Giridhar KV, Sanhueza C, Hillman DW, et al. Serum chromogranin-A-based prognosis in metastatic castration-resistant prostate cancer. Prostate Cancer Prostatic Dis 2018;21:431-7. [Crossref] [PubMed]
- Karlsson A, Cirenajwis H, Ericson-Lindquist K, et al. A combined gene expression tool for parallel histological prediction and gene fusion detection in non-small cell lung cancer. Sci Rep 2019;9:5207. [Crossref] [PubMed]
- Zhang X, Zhang H, Shen B, et al. Chromogranin-A Expression as a Novel Biomarker for Early Diagnosis of Colon Cancer Patients. Int J Mol Sci 2019;20:2919. [Crossref] [PubMed]
- Swinnen JV, Dehairs J, Talebi A. Membrane Lipid Remodeling Takes Center Stage in Growth Factor Receptor-Driven Cancer Development. Cell Metab 2019;30:407-8. [Crossref] [PubMed]
- Du Y, Wang Q, Zhang X, et al. Lysophosphatidylcholine acyltransferase 1 upregulation and concomitant phospholipid alterations in clear cell renal cell carcinoma. J Exp Clin Cancer Res 2017;36:66. [Crossref] [PubMed]
- Sun H, Ou B, Zhao S, et al. USP11 promotes growth and metastasis of colorectal cancer via PPP1CA-mediated activation of ERK/MAPK signaling pathway. EBioMedicine 2019;48:236-47. [Crossref] [PubMed]
- Chen M, Wan L, Zhang J, et al. Deregulated PP1α phosphatase activity towards MAPK activation is antagonized by a tumor suppressive failsafe mechanism. Nat Commun 2018;9:159. [Crossref] [PubMed]
- Wang CY, Li CY, Hsu HP, et al. PSMB5 plays a dual role in cancer development and immunosuppression. Am J Cancer Res 2017;7:2103-20. [PubMed]
- Mo HM, Wu QY, Han DY, et al. Effects of PSMB5 on proliferation and bortezomib chemo-resistance in human myeloma cells and its related molecular mechanisms. Zhonghua Xue Ye Xue Za Zhi 2017;38:1053-7. [PubMed]
- Zhang G, Lin RK, Kwon YT, et al. Signaling mechanism of tumor cell-induced up-regulation of E3 ubiquitin ligase UBR2. FASEB J 2013;27:2893-901. [Crossref] [PubMed]
- Mao J, Liang Z, Zhang B, et al. UBR2 Enriched in p53 Deficient Mouse Bone Marrow Mesenchymal Stem Cell-Exosome Promoted Gastric Cancer Progression via Wnt/β-Catenin Pathway. Stem Cells 2017;35:2267-79. [Crossref] [PubMed]
- Looijenga LH. Testicular cancer: risk stratification in adolescents with nonseminoma. Nat Rev Urol 2014;11:367-8. [Crossref] [PubMed]
- Kojima T, Kawai K, Tsuchiya K, et al. Identification of a subgroup with worse prognosis among patients with poor-risk testicular germ cell tumor. Int J Urol 2015;22:923-7. [Crossref] [PubMed]
- Neumann A, Keller T, Jocham D, et al. Human placental alkaline phosphatase (hPLAP) is the most frequently elevated serum marker in testicular cancer. Aktuelle Urol 2011;42:311-5. [Crossref] [PubMed]
- Koshida K, Uchibayashi T, Yamamoto H, et al. Significance of placental alkaline phosphatase (PLAP) in the monitoring of patients with seminoma. Br J Urol 1996;77:138-42. [Crossref] [PubMed]
- Lajer H, Daugaard G, Andersson AM, et al. Clinical use of serum TRA-1-60 as tumor marker in patients with germ cell cancer. Int J Cancer 2002;100:244-6. [Crossref] [PubMed]
- Fosså SD, Klepp O, Paus E. Neuron-specific enolase--a serum tumour marker in seminoma? Br J Cancer 1992;65:297-9. [Crossref] [PubMed]
- Kuzmits R, Schernthaner G, Krisch K. Serum neuron-specific enolase. A marker for responses to therapy in seminoma. Cancer 1987;60:1017-21. [Crossref] [PubMed]