The influence of lncRNAs on the prognosis of prostate cancer based on TCGA database
Introduction
Prostate cancer is a common cause of death in older men. In recent years, the incidence of prostate cancer has increased yearly, and the age of onset is younger, which has become a crucial factor affecting men’s quality of life. Many patients with prostate cancer cannot be diagnosed and are not treated early, making them progress to severe levels (1,2). LncRNA is a kind of non-coding RNA with a length more than 200 nucleotides. LncRNA plays an important role in many life activities such as dose compensation effect, epigenetic regulation, cell cycle regulation and cell differentiation regulation (3). In urinary system tumors, lncRNA has been found to participate in androgen receptor signalling in prostate cancer, hypoxia-inducible factor pathway activation in renal cell carcinoma and invasiveness in bladder cancer (3). Although the non-surgical treatment of prostate cancer is mainly based on the blocking of the androgen pathway, a large number of clinical outcomes show that once prostate cancer progresses to castration-resistant prostate cancer (CRPC), the tumor depends on other ways to maintain growth. The Cancer Genome Atlas (TCGA) is currently the largest cancer gene database. Research on the data in TCGA helps to discover the mechanism of cancer and explore treatment methods. Therefore, finding new therapeutic targets for tumors and better diagnosing them early and evaluating them better is the most urgent clinical problem. lncRNA, a new class of molecule, provides us with another choice (4).
We present the following article in accordance with the REMARK reporting checklist (available at http://dx.doi.org/10.21037/tau-21-154).
Methods
Data collection
Transcriptome data and clinical information from 499 cases cancer tissue and 25 cases normal tissues were retrieved from The Cancer Genome Atlas (TCGA) database (https://portal.gdc.cancer.gov/).
Statistical analysis
lncRNA differential expression profiles
LncRNA-seq data were retrieved using the TCGAbiolinks. 14083 LncRNAs were screened using the biomaRt package (5,6). The samples of normal tissue and cancer tissue were divided randomly into two groups, and DESeq2 was used for differential expression analysis. The screening criteria for up-regulated genes were P<0.05 and log2 fold change >1, and the criteria for down-regulated genes were P<0.05 and log2 fold change <−1. After retrieving the differentially expressed lncRNA in each group, use the Venn Diagram package to draw a Venn diagram to show the intersection (7).
Risk scoring model construction and evaluation
We used the survival package to perform a single-factor Cox analysis on the data downloaded through TCGAbiolinks. We screened out 13 LncRNAs related to overall survival (OS). The standard is P<0.001. Multivariate Cox regression analysis was carried out through the backward stepwise method to determine six independent prognostic factors LncRNA, and Lasso regression was implemented through glmnet for further verification. Finally, a risk-scoring model is established based on the results of multi-factor Cox regression.
According to the median of risk scores, patients were divided into high-risk groups and low-risk groups. The K-M survival curve was implemented with the survminer package. The time-dependent ROC curve was based on the risk score drawn by the survivalROC package.
Identification and functional analysis of co-expressed coding genes
We used WGCNA to screen the coding genes co-expressed with prognostic-independent lncRNA (the soft threshold was set to β=3, the adjacency threshold was set to 0.1; the Cytoscape software was used for visualization) (8,9). The functional enrichment analysis of GO and KEGG encoding genes is realized with the clusterProfiler package (10). Select “c2.all.v7.0.symbols.gmt” in the MSigDB gene set, and the screening criterion is P<0.05. GOplot visualized the results.
Screening of key coding genes and analysis of immune infiltration
We used the STRING database (https://string-db.org/) to retrieve the interactions of the genetically encoded proteins (score >0.4), and then used CytoHubba, a plug-in in the Cytoscape software, to identify 10 key coding genes (11,12). Immune infiltration was analyzed in TIMER2.0 (http://cistrome.dfci.harvard.edu/TIMER/) online tool (13).
The study was conducted in accordance with the Declaration of Helsinki (as revised in 2013).
Results
Abnormal expression of lncRNAs
We classified and grouped the transcriptome data of the PRAD patients (Table 1) in the TCGA database, which was N1 (26 normal tissues), N2 (26 normal tissues), T1 (250 cancerous tissues), and T2 (249 cancerous tissues). The difference analysis of 14,083 lncRNAs in the normal group and the cancerous group, the 461 lncRNAs that were significantly up-regulated in cancer tissues (Figure 1A), and 653 lncRNAs that were significantly down-regulated (Figure 1B) were finally retrieved. Compared with lncRNA, whose expression level has not changed significantly, we define that the expression level of up-regulated lncRNA is significantly increased in cancer tissue (T), while the expression level of down-regulated lncRNA is higher in normal tissue (N) (Figure 1C, https://cdn.amegroups.cn/static/public/tau-21-154-1.xlsx). The volcano map results also verified the correctness of the differentially expressed lncRNA we defined (Figure 1D).
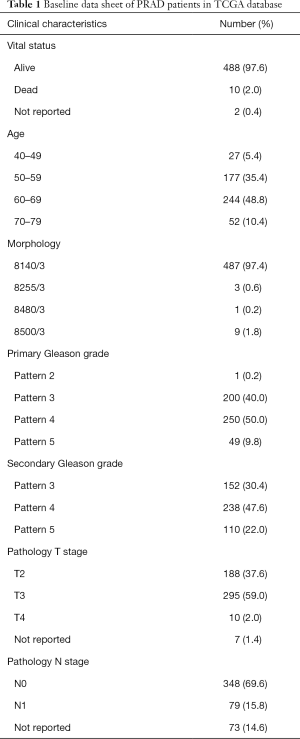
Full table
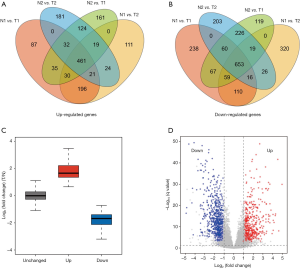
Construction of prognostic model
Combining the results of differential expression analysis and the clinical data of PRAD patients, through univariate Cox regression analysis, we retrieved 13 lncRNAs that were significantly related to overall survival. Further, through multivariate Cox regression analysis, 6 lncRNAs (AC245884.1, LINC01524, AL807752.4, AP000844.2, AC016590.1, LINC00641) were screened out as independent prognostic factors (Table 2). This result can be verified by Lasso regression (Figure 2A). The prognostic risk model based on these 6 lncRNAs (Figure 2B) is: risk score = 0.259 × AC245884.1 + 0.187 × LINC01524 -0.100 × AL807752.4 + 0.00256 × AP000844.2 + 0.0588 × AC016590.1 + 0.00557 × LINC00641. Then, we analyzed patients’ survival based on the risk score and found that more patients died in the high-risk group than in the low-risk group (Figure 2C), and the survival time decreased as the risk score increased (Figure 2D). Further analysis of K-M survival showed that the high-risk group’s prognosis was significantly worse than that of the low-risk group (Figure 2E). The risk model has good predictive power, with an AUC value of 0.778 (Figure 2F).
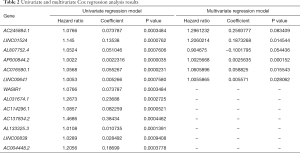
Full table
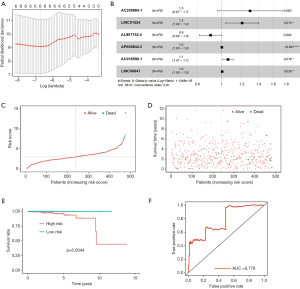
Relationship between 6 lncRNAs and clinical data
For these 6 lncRNAs, only LINC00641 was significantly downregulated in cancer tissues, and the expression of the remaining lncRNAs was significantly increased (Figure 3A). Simultaneously, we found that AC245884.1 was significantly correlated with the Gleason score, and the other 5 lncRNAs were not found to be significantly correlated (Figure 3B). We verified these results in the GSE115414 data set and found that the expression levels of AC245884.1, LINC01524, and AP000844.2 in PRAD tissues were significantly higher than those in normal tissues, while AC016590.1, although not significant, has the same trend, AL807752. 4 and LINC00641 have no obvious difference (Figure 3C). As for the relationship between gene expression and Gleason score, we can observe that the expression level of AC245884.1 may have a potential correlation with Gleason score (Figure 3D). Since the conclusions drawn by the two data sets of TCGA and GSE115414 are not completely consistent, we can explain that the number of lncRNA samples is smaller.
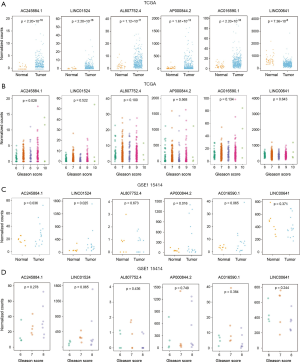
The biological functions of 6 lncRNAs
To further clarify the biological functions of these six lncRNAs, we used WGCNA to identify the coding genes co-expressed with them. To make the constructed network more in line with the scale-free network’s characteristics, we choose a soft threshold of 3 (Figure 4A,B). Among them, 14 coding genes were co-expressed with LINC01524 (Figure 4C), 35 were co-expressed with AL807752.4 (Figure 4D), and 555 were related to LINC00641 (Figure 4E). The remaining 3 lncRNAs were not found any co-expressed coding genes (https://cdn.amegroups.cn/static/public/tau-21-154-2.xlsx). Next, we performed GO analysis on these coding genes and found that the biological process of their significant enrichment is the sense of smell and taste (Figure 5A). The molecular function is the receptor activity of smell and taste, and alditol: NADP + 1-oxidation Reductase activity (Figure 5B). However, cell components were not significantly enriched. Enrichment analysis of the KEGG pathway also showed that these genes are related to smell and taste transmission (Figure 5C). We used the MSigDB gene set for enrichment analysis and found that these genes were significantly related to the C/3 metabotropic glutamate pheromone receptor (Figure 5D, https://cdn.amegroups.cn/static/public/tau-21-154-3.xlsx). We screened out 10 key genes among these genes, of which 7 are related to taste receptors (Figure 6A). Through immune infiltration analysis of PRAD, it was found that the expression levels of TAS2R4 (as a representative of taste receptor-related genes), P2RY14, GPR18 and CCR9 were related to immune cell (B cell, CD4+ T cells, CD8+ T cells, and macrophages) infiltration levels were significantly correlated (Figure 6B). Except that the expression level of TAS2R4 was not significantly correlated with tumor purity, the expression levels of P2RY14, GPR18 and CCR9 were negatively correlated with tumor purity.
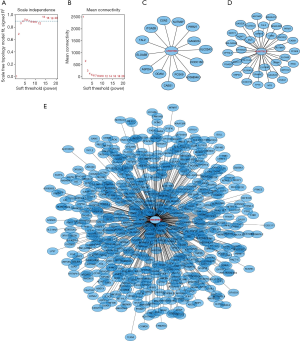
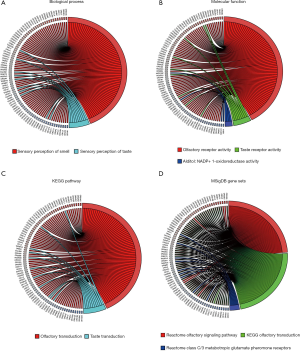
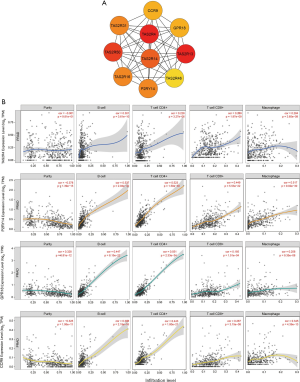
Discussion
Prostate cancer is one of the most common tumors in the male urinary system. The increasing incidence and poor prognosis have increasingly become a crucial factor limiting human life quality. LncRNA was originally thought to be a by-product of genome transcription and did not have biological functions. However, in later studies, it was found that it can interact with DNA and various RNAs, affecting the transcription and translation processes of cells. The function of most lncRNAs is still unclear, and many lncRNAs are still not taken seriously. Recent studies have shown that the amount of lncRNA in cancer differs significantly from that in normal tissues, and there are many differential expressions related to prognosis. Therefore, the study of lncRNA is helpful to improve the prognosis research of prostate cancer.
In this study, the differential expression analysis of lncRNA in PRAD patients in the TCGA database was performed. It was found that there are many differentially expressed lncRNAs in prostate cancer. Through COX regression analysis, we finally screened out 6 lncRNAs (AC245884.1, LINC01524, AL807752.4, AP000844.2, AC016590.1, LINC00641) as independent prognostic factors and successfully constructed a risk prognosis model. By calculating the risk score, the survival outcome of the patient can be effectively evaluated. Through further analysis, it was found that some of these lncRNAs were related to the Gleason score (AC245884.1), while others were not found to be related to common prognostic indicators. To understand the biological functions of these lncRNAs, WGCNA was used to determine the existence of a certain number of co-expressed genes in 3 lncRNAs. Through GO and KEGG enrichment analysis, it is found that the main enrichment is the sense and conduction of smell and taste. Also, enrichment analysis with MSigDB showed that these genes are related to C/3 metabotropic glutamate pheromone receptors. Through immune infiltration analysis, the expression levels of AS2R4, P2RY14, GPR18, and CCR9 are significantly related to the tumor’s purity and immune cell infiltration level.
There is a limitation in this article that the specific role of LncRNA in prostate cancer remains unclear. In existing studies, the overexpression of LINC00641 can inhibit the growth and invasion of prostate cancer cells (14). There is no relevant basic research on the other five genes in the results. Therefore, the lncRNA in the prediction, diagnosis, and treatment of prostate cancer has enormous potential.
Some calculation results were run than can be included in the article. Interested readers can find them in a supplementary appendix online: https://cdn.amegroups.cn/static/public/tau-21-154.zip.
Acknowledgments
Funding: This work was supported by the Zhejiang Provincial Natural Science Foundation of China under [Grant No. LQ20H050001 and LY20H160013] and Wenzhou Science and Technology Project [Y20190066].
Footnote
Reporting Checklist: The authors have completed the REMARK reporting checklist. Available at http://dx.doi.org/10.21037/tau-21-154
Conflicts of Interest: All authors have completed the ICMJE uniform disclosure form (available at http://dx.doi.org/10.21037/tau-21-154). The authors have no conflicts of interest to declare.
Ethical Statement: The authors are accountable for all aspects of the work in ensuring that questions related to the accuracy or integrity of any part of the work are appropriately investigated and resolved. Our study was following the publication guidelines provided by TCGA.The study was conducted in accordance with the Declaration of Helsinki (as revised in 2013).
Open Access Statement: This is an Open Access article distributed in accordance with the Creative Commons Attribution-NonCommercial-NoDerivs 4.0 International License (CC BY-NC-ND 4.0), which permits the non-commercial replication and distribution of the article with the strict proviso that no changes or edits are made and the original work is properly cited (including links to both the formal publication through the relevant DOI and the license). See: https://creativecommons.org/licenses/by-nc-nd/4.0/.
References
- US Preventive Services Task Force. Screening for Prostate Cancer: US Preventive Services Task Force Recommendation Statement. JAMA 2018;319:1901-13. Erratum in: JAMA 2018;319:2443. [Crossref] [PubMed]
- Kimura T, Sato S, Takahashi H, et al. Global Trends of Latent Prostate Cancer in Autopsy Studies. Cancers (Basel) 2021;13:359. [Crossref] [PubMed]
- Flippot R, Beinse G, Boilève A, et al. Long non-coding RNAs in genitourinary malignancies: a whole new world. Nat Rev Urol 2019;16:484-504. [Crossref] [PubMed]
- Gregg JR, Thompson TC. Considering the potential for gene-based therapy in prostate cancer. Nat Rev Urol 2021;18:170-84. [Crossref] [PubMed]
- Durinck S, Spellman PT, Birney E, et al. Mapping identifiers for the integration of genomic datasets with the R/Bioconductor package biomaRt. Nat Protoc 2009;4:1184-91. [Crossref] [PubMed]
- Colaprico A, Silva TC, Olsen C, et al. TCGAbiolinks: an R/Bioconductor package for integrative analysis of TCGA data. Nucleic Acids Res 2016;44:e71 [Crossref] [PubMed]
- Chen H, Boutros PC. VennDiagram: a package for the generation of highly-customizable Venn and Euler diagrams in R. BMC Bioinformatics 2011;12:35. [Crossref] [PubMed]
- Botía JA, Vandrovcova J, Forabosco P, et al. An additional k-means clustering step improves the biological features of WGCNA gene co-expression networks. BMC Syst Biol 2017;11:47. [Crossref] [PubMed]
- Smoot ME, Ono K, Ruscheinski J, et al. Cytoscape 2.8: new features for data integration and network visualization. Bioinformatics 2011;27:431-2. [Crossref] [PubMed]
- Yu G, Wang LG, Han Y, et al. clusterProfiler: an R package for comparing biological themes among gene clusters. OMICS 2012;16:284-7. [Crossref] [PubMed]
- Szklarczyk D, Franceschini A, Wyder S, et al. STRING v10: protein-protein interaction networks, integrated over the tree of life. Nucleic Acids Res 2015;43:D447-D452. [Crossref] [PubMed]
- Chin CH, Chen SH, Wu HH, et al. cytoHubba: identifying hub objects and sub-networks from complex interactome. BMC Syst Biol 2014;8:S11. [Crossref] [PubMed]
- Li B, Severson E, Pignon JC, et al. Comprehensive analyses of tumor immunity: implications for cancer immunotherapy. Genome Biol 2016;17:174. [Crossref] [PubMed]
- Liu WH, Lu JJ, Yu RK, et al. LINC00641 regulates prostate cancer cell growth and apoptosis via the miR-365a-3p/VGLL4 axis. Eur Rev Med Pharmacol Sci 2021;25:108-15. [PubMed]
(English Language Editor: J. Chapnick)