A novel genomic model for predicting the likelihood of delayed graft function in DCD kidney transplantation
Introduction
Kidney transplantation (KT) currently serves as the optimal treatment for patients with end-stage kidney disease, and a highly growing demand for organs has prompted the rising usage of donation after cardiac death (DCD) donors (1,2). However, due to its additional warm ischemia time (WIT), DCD kidneys are more vulnerable to ischemia-reperfusion injury (IRI) than kidneys from donation after brain death (DBD) donors or living donors. Delayed graft function (DGF), generally defined as the requirement for dialysis within the first week after KT, is one of the most common early complications following deceased-donor KT, particularly among those receiving DCD kidneys (3,4). DGF has attracted increasing attention because apart from its high incidence, it is closely related to poorer short-term outcomes (such as prolonged hospitalization, higher cost, and acute rejection) and long-term outcomes (such as graft failure), which pose great challenges to transplant clinicians (5,6). Thus, it is urgent and meaningful to deeply explore the mechanisms of DGF and precisely predict its occurrence pre- or early post-KT with DCD allografts (DCD KT) to prevent or minimize the impact of DGF.
In the last few years, several predictors associated with DGF occurrence have been identified, and numerous clinical or histologic models for DGF prediction have been proposed (7-11). However, considering the unique pathophysiological processes of DCD kidneys, the accuracy and applicability of these models for DGF prediction in DCD KT are limited, which indicates that the conventional clinicopathologic evaluations no longer meet the needs of clinical practice, and the transition from macroscopic assessment to molecular evaluation is necessary in the era of precision medicine (12,13). Fortunately, some efforts have been attempted to explore the molecular characteristics of DCD kidneys based on transcriptomic analyses of pre- or early postoperative biopsy (14,15). Nevertheless, DGF-related biomarkers for DCD KT remain to be investigated, and a gene-based predictive model specifically for predicting the likelihood of DGF in DCD KT has been rarely established to date.
By thoroughly searching the Gene Expression Omnibus (GEO), a reliable public database archiving massive genomic data and relevant clinical data in the biomedical field, we found a valuable dataset (GSE43974) that included the clinical and microarray data of kidney biopsies taken from human DBD or DCD allografts after cold storage and short-time reperfusion (timepoint 3, T3) (16). In this study, we aimed to identify DGF-related biomarkers for DCD kidneys based on the T3 kidney biopsies that could fully and timely reflect the whole IRI process of kidney allografts, and to further establish a genomic model specifically for DGF prediction in DCD KT. Of note, except for early posttransplant DGF prediction, the rise of normothermic machine perfusion (NMP) also promotes the feasibility and prospects of genomic models for accurate DGF prediction before DCD KT via ex vivo simulation of normal physiological status (17). We present the following article in accordance with the TRIPOD reporting checklist (available at http://dx.doi.org/10.21037/tau-20-1533).
Methods
Data preparation and DGF-related genes screening
The GSE43974 dataset, which includes the microarray data of kidney biopsies taken from three types of donations (living, DBD and DCD donors) at different time points, was downloaded from the GEO database (14). In the DCD (type III, awaiting cardiac arrest) cohort, kidney needle biopsies enrolled in this study were collected at three time points: the DCD-T1 group (after cardiac arrest and WIT, and before organ retrieval, n=38), the DCD-T2 group (after cold ischemia time (CIT), and before organ implantation, n=53) and the DCD-T3 group (at 45–60 min after reperfusion, n=64). In the DBD cohort, biopsies taken at 45–60 min after reperfusion (DBD-T3 group, n=105) were enrolled in this study. The key characteristics of the allografts in the DCD-T3 and DBD-T3 groups, which were both divided into two comparable subgroups (Cohort 1 and Cohort 2) in the raw data, are generally shown in Figure 1A and summarized in detail in Table S1. Moreover, the gene expression profile of 37 normal kidney tissues taken from living donor nephrectomy before clamping the renal artery was also enrolled in this study as normal controls. The R package “lumi” was used to preprocess and normalize the raw data, and the Z-score was further computed for each selected gene as its relative expression level during the modeling. The study was conducted in accordance with the Declaration of Helsinki (as revised in 2013) (18).
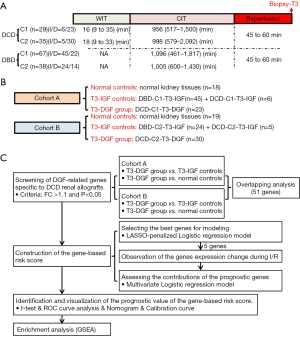
To reliably screen the DGF-related differentially expressed genes (DEGs) in T3 kidney biopsies specific to DCD KT, cohort A (DCD-T3-C1 plus DBD-T3-C1) and cohort B (DCD-T3-C2 plus DBD-T3-C2) were first set up, in which three subgroups (normal controls, T3-IGF controls and T3-DGF group) were then defined. In brief, the T3-DGF group comprised the patients requiring dialysis within the first week after DCD KT. T3-IGF controls were patients undergoing immediate graft function (IGF) after KT with DBD or DCD renal allografts. Normal controls were normal kidney biopsies, which were randomly assigned to the two cohorts. The details of the grouping information are shown in the Figure 1B. The DEGs of the T3-DGF group compared to T3-IGF controls and normal controls were screened out by R package “limma” using “fold change (FC) >1.1 with adjusted P<0.05” as the threshold in both the cohort A and the cohort B. Then, overlapping analyses between the two cohorts were further performed to identify the potential DGF-related DEGs specific to DCD renal allografts.
Gene selection and model construction
To select the optimal genes among DEGs for modeling, LASSO-penalized logistic regression analysis was performed in the DCD-T3 group (n=64) using the R package “glmnet”. The optimal gene set for modeling was determined on the basis of 10-fold cross-validation and lambda.min. After that, the selected genes were enrolled into multivariate logistic regression model to calculate their coefficients (β values). The gene-based risk score was constructed using the following formula: Risk score= (β1 * Z-score of gene1) + (β2* Z-score of gene2) +...+ (βn* Z-score of genen).
Gene set enrichment analysis (GSEA)
The 64 patients in the DCD-T3 group were segmented into two groups (low- or high risk of DGF) based on the optimal cutoff values of the risk score using receiver operating characteristic (ROC) curve analysis. Then, we conducted GSEA between the two groups to explore the underlying pathways significantly associated with high risk of DGF in DCD KT. “C2.cp.kegg.v7.0.symbols.gmt” was chosen as the reference gene set, and gene set with a normalized P<0.05 was deemed significantly enriched.
Statistical analysis
Continuous variables between the different groups were compared by Student’s t-test or one-way analysis of variance. ROC curves were utilized to evaluate the predictive effectiveness of the risk score for DGF. A predictive nomogram based on the risk score was established using the R package “rms”, and its performance was comprehensively evaluated by generating calibration plot and calculating the concordance index (C-index). Logistic regression model analysis was applied to assess the relevant factors in terms of DGF occurrence. R 3.4.2, SPSS 22.0 and GraphPad Prism 8.0.2 were used for statistical analysis and graphing. A P<0.05 was regarded as statistically significant.
Results
Screening of DGF-related DEGs in DCD kidney allografts
The flowchart describing the overall design of this study is shown in Figure 1C. The DEGs in the T3-DGF group compared to T3-IGF controls and normal controls were first recognized in both the cohort A and the cohort B (Figure 2A,B), and subsequent overlapping analysis further identified 30 upregulated genes and 21 downregulated genes significantly associated with DGF occurrence following DCD KT (Figure 2C). The relative expression levels of the 51 genes in different groups are briefly presented in the heat map (Figure 2D).
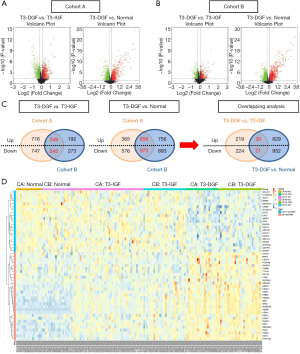
Construction of gene-based risk score
As shown in Figure S1, the optimal genes for modeling were identified by LASSO-penalized logistic regression analysis, including one upregulated gene (carbohydrate sulfotransferase 3, CHST3) and four downregulated genes (golgi phosphoprotein 3, GOLPH3; zinc finger BED-type containing 5, ZBED5; aldo-keto reductase family 1 member C4, AKR1C4; ERBB receptor feedback inhibitor 1, ERRFI1). The associations between the expression of the five genes in T3 biopsies and DGF occurrence after DCD KT were confirmed by univariate logistic regression model analyses (all P<0.05) (Table S2).
Using the microarray data of kidney biopsies taken from DCD allografts at three time points (T1, T2 and T3), we observed the expression changes of five genes during the processes of ischemia and reperfusion compared to normal controls and IGF controls. As shown in Figure 3, the expression levels of CHST3, GOLPH3 and ZBED5 were significantly changed in the DCD-T3-DGF group compared with the normal controls and DCD-T3-IGF controls (all P<0.05), while their expression levels in the other groups were not significantly different from the normal controls (all P>0.05), suggesting that the dysregulation of the three genes might mainly indicate injury of reperfusion. For AKR1C4, we found that its expression level was significantly downregulated after CI and reperfusion in the DGF groups (DCD-T2-DGF and DCD-T3-DGF), indicating that AKR1C4 might be a signature associated with injury of CI and reperfusion. Interestingly, the expression level of ERRFI1 began to dramatically decline after WI and was maintained until the end of CI both in the IGF groups (DCD-T1-IGF and DCD-T2-IGF) and DGF groups (DCD-T1-DGF and DCD-T2-DGF). After reperfusion, while ERRFI1 mRNA expression rose to relatively normal levels in the DCD-T3-IGF group, its level in the DCD-T3-DGF group remained low compared to the normal controls and IGF controls. This implied that ERRFI1 might be sensitive to renal ischemia and was a good signature reflecting the acute kidney injury (AKI) induced by IRI.
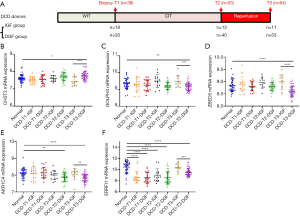
Next, the coefficients of five genes were further obtained by using a multivariate logistic regression model in the DCD-T3 group. The gene-based risk score was calculated using the following formula: Risk score = (1.685* Z-score of CHST3) + (−2.066* Z-score of GOLPH3) + (−1.023* Z-score of ZBED5) + (−3.831* Z-score of AKR1C4) + (−1.529* Z-score of ERRFI1) (Table S2).
Identification of the predictive value of gene-based risk score
In the DCD-T3 cohort, the risk score in the DGF group (n=53) was remarkably higher than that in the IGF group (n=11) (P<0.0001) (Figure 4A). ROC analyses further confirmed that the risk score had an excellent diagnostic value for DGF in DCD KT (AUC =0.9708, P<0.0001), which was obviously higher than that of the five genes alone (Figure 4B). In addition, to enhance its applicability, a convenient, visual and predictive nomogram based on the risk score was also established in this study (Figure 4C). The C-index of the nomogram was equivalent to the AUC, and the calibration plot displayed high consistency between the nomogram-predicted probability of DGF and the actual probability of DGF, suggesting that the nomogram was an ideal model for precise DGF prediction in DCD KT (Figure 4D).
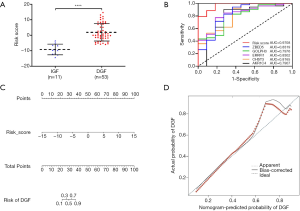
Enrichment analysis
To further explore the potential biological pathways related to DGF occurrence in DCD KT, GSEA was performed between the low or high-risk groups in terms of DGF defined by the risk score. As shown in Figure S2, a total of eight KEGG pathways were significantly enriched, including “drug metabolism cytochrome p450”, “arachidonic acid metabolism”, “glutathione metabolism”, “lysosome”, “proximal tubule bicarbonate reclamation”, “metabolism of xenobiotics by cytochrome p450”, “arginine and proline metabolism” and “retinol metabolism” (all P<0.05).
Discussion
Along with the increasing attention to the molecular mechanisms of DGF, several DGF-related biomarkers in varied sample sources taken at different timepoints, such as NGAL, HIF-1α, HSP72 and TIMP-1, have been identified (5,19-23). Nevertheless, their specificity and sensitivity are still insufficient for precise DGF prediction. This may attribute to the following reasons. First, the optimal sample types (kidney tissue, serum, urine, perfusate or preservation fluid) and sampling timepoint (before organ retrieval, pretransplant or postreperfusion) for DGF prediction are currently disputable (24). Given that DGF occurs in the context of IRI, the biomarkers or models identified based on the samples taken from donors or allografts before organ retrieval or pretransplant might not fully reflect the actual renal damage caused by ischemia or reperfusion (25,26). Consistent with this, no reliable DGF-related gene was found in the DCD kidney biopsies taken prior to transplantation (T1 and T2), as reported by Damman et al. (14). Of note, certain sample types (such as postoperative kidney needle biopsy) are either difficult to obtain in routine clinical practice or more invasive for recipients, and the predictive ability and timeliness of some sample types (such as first-day posttransplant blood) for DGF prediction are still inferior to those of kidney tissue biopsies (5). In addition, the different pathophysiological processes of varied donor types (living/DBD/DCD donors) should be taken into consideration, and unspecific research objects in most previous studies may diminish the predictive power of the existing biomarkers (19,24). Finally, the unsatisfactory predictive ability of the individual biomarkers alone may also underline the necessity of integrating multiple molecular signatures into a single model to enhance DGF predictive accuracy.
With these considerations in mind, DGF prediction based on transcriptomics analysis of kidney tissue biopsy taken after short-time reperfusion (such as 1-hour biopsy) undoubtedly has tremendous advantages over the other sample types from the perspective of timeliness, reliability, and feasibility. The identification of DGF-related biomarkers and establishment of a gene-based predictive model for DGF prediction specific to DCD KT are highly anticipated. In this study, thanks to the public clinical and microarray data provided by the GSE43974 dataset, the five key DGF-associated genes (CHST3, GOLPH3, ZBED5, AKR1C4, and ERRFI1) in T3 kidney biopsies were first identified by rigorous study design and reasonable statistical approaches, all of which exhibited good discrimination for DGF in DCD KT. In addition, for the purpose of strengthening their predictive accuracy, a risk score based on the above five genes was further established and displayed excellent predictive ability that was obviously higher than that of the five genes alone. Moreover, to enhance its clinical applicability, a convenient and visual nomogram based on the genomic risk score was also constructed. The high predictive accuracy of the genomic nomogram suggests that it may be an ideal model for precise DGF prediction that is specific to DCD KT.
The primary mechanism underlying DGF is thought to be associated with IRI, which is an inevitable and complex pathophysiological phenomenon (involving inflammatory and immune responses) in DCD KT leading to acute tubular necrosis (25). More insights gained in the molecular pathophysiology of IR in DCD renal allografts would open a door to the understanding and management of DGF. Consistent with the observed alterations of the five genes in DCD kidneys during IR in this study, previous studies have suggested that the above genes are closely involved in the pathogenic process of IRI to some extent (27-31). The general information of the five genes and the potential correlations between their dysregulation and renal IRI or DGF occurrence have been properly discussed in the Appendix 1. In addition, by conducting GSEA according to the genomic risk score, the underlying biological pathways associated with DGF occurrence specific to DCD kidneys, such as “arachidonic acid metabolism”, “lysosome”, “proximal tubule bicarbonate reclamation”, “glutathione metabolism”, were identified in this study, which might contribute to the development of mechanism research on DGF and its targeted therapies in DCD KT in the future.
In view of the good performance of the five-mRNA-based risk score for DGF prediction, this model may provide necessary guidance for early posttransplant or even pretransplant decision-making in DCD KT. On the one hand, transcription analysis of 1-hour renal biopsy by microarray or qPCR may provide timely warning of the risk of DGF immediately after DCD KT, which could promote timely therapeutic or preventive interventions to minimize the impact of DGF on KT recipients, including the close monitoring strategy, the adjustment of immunosuppressive regimen, the optimization of volume management and the administration of thymoglobulin (26,32). Meanwhile, patients with lower DGF risk may benefit from a shorter intensive monitoring period, therefore resulting in shorter overall hospital stays and lower medical costs (32). However, it is worth noting that the timeliness of gene expression quantification is key to the utility of the genomic model in clinical practice, which relies on the further development of rapid nucleic acid quantification technology and the optimization of whole processes of gene detection, including specimen collection and preparation, nucleic acid quantification and results return (33). On the other hand, given the limited recipient-based interventions for DGF, a more objective, reliable, and accurate quality assessment of DCD kidney grafts before KT may have greater practical significance (26). Fortunately, the ongoing improvement of NMP and rapid nucleic acid quantification undoubtedly enhances the feasibility and prospects of the genomic model in clinical practice for DGF prediction prior to DCD KT (17,33). The accurate risk stratification of DGF before transplantation based on the genomic model may not only guide comprehensive quality evaluation of DCD kidneys, reasonable allocation or discard of DCD kidneys and recipient-based preventive interventions (such as induction therapy by anti-thymocyte globulin), but also contribute to the speedy development of extracorporeal targeted therapies for DCD kidneys in the context of NMP, therefore preventing or minimizing the impact of DGF and effectively expanding the donor pool. Of note, ex vivo NMP is currently unable to perfectly simulate normal physiological status (including immune microenvironments), and the ideal and optimal NMP platform for donor kidney evaluation is still evolving. Thus, the feasibility of the genomic model for DGF prediction prior to DCD KT based on ex vivo NMP is worth further exploring in the future.
The excellent predictive ability and applicability of the genomic model for DGF prediction in DCD KT should be recognized, as well as the several limitations in our study. First, although the patients’ clinical baseline characteristics have been summarized in the original article of Damman et al. (14), these raw data have not been shared in detail in the GSE43974 dataset. Thus, the construction of a promising genomic-clinicopathologic nomogram integrating the genomic risk score and clinicopathological factors with independent predictive value for DGF could not be achieved in this study. In addition, due to the limited clinicopathological data in the GSE43974 dataset, the predictive ability of the genomic model cannot be compared with that of the existing clinical predictive models. Finally, unfortunately, except for the GSE43974 dataset, no other appropriate dataset was found after a thorough search in the GEO database. Therefore, the efficiency of the genomic model deserves more external validation using independent DCD KT cohorts with larger sample sizes in the future.
Conclusions
In conclusion, we established a novel and powerful genomic model based on the expression of five genes in kidney biopsies taken after short-time reperfusion for DGF prediction specific to DCD KT. This model may be a promising tool for risk stratification of DGF in DCD KT, which could provide necessary guidance for early posttransplant decision-making or even pretransplant decision-making in the context of NMP in the future.
Acknowledgments
Funding: This work was supported by the National Natural Science Foundation of China (grant number: 81570079).
Footnote
Reporting Checklist: The authors have completed the TRIPOD reporting checklist. Available at http://dx.doi.org/10.21037/tau-20-1533
Conflicts of Interest: All authors have completed the ICMJE uniform disclosure form (available at http://dx.doi.org/10.21037/tau-20-1533). The authors have no conflicts of interest to declare.
Ethical Statement: The authors are accountable for all aspects of the work in ensuring that questions related to the accuracy or integrity of any part of the work are appropriately investigated and resolved. The study was conducted in accordance with the Declaration of Helsinki (as revised in 2013).
Open Access Statement: This is an Open Access article distributed in accordance with the Creative Commons Attribution-NonCommercial-NoDerivs 4.0 International License (CC BY-NC-ND 4.0), which permits the non-commercial replication and distribution of the article with the strict proviso that no changes or edits are made and the original work is properly cited (including links to both the formal publication through the relevant DOI and the license). See: https://creativecommons.org/licenses/by-nc-nd/4.0/.
References
- Hart A, Smith JM, Skeans MA, et al. OPTN/SRTR 2017 Annual Data Report: Kidney. Am J Transplant 2019;19:19-123. [Crossref] [PubMed]
- Zhang L, Zeng L, Gao X, et al. Transformation of organ donation in China. Transpl Int 2015;28:410-5. [Crossref] [PubMed]
- Mallon DH, Summers DM, Bradley JA, et al. Defining delayed graft function after renal transplantation: simplest is best. Transplantation 2013;96:885-9. [Crossref] [PubMed]
- Mannon RB. Delayed Graft Function: The AKI of Kidney Transplantation. Nephron 2018;140:94-8. [Crossref] [PubMed]
- Mourao TB, Mine KL, Campos EF, et al. Predicting delayed kidney graft function with gene expression in preimplantation biopsies and first-day posttransplant blood. Hum Immunol 2016;77:353-7. [Crossref] [PubMed]
- Lim WH, McDonald SP, Russ GR, et al. Association Between Delayed Graft Function and Graft Loss in Donation After Cardiac Death Kidney Transplants-A Paired Kidney Registry Analysis. Transplantation 2017;101:1139-43. [Crossref] [PubMed]
- Irish WD, Ilsley JN, Schnitzler MA, et al. A risk prediction model for delayed graft function in the current era of deceased donor renal transplantation. Am J Transplant 2010;10:2279-86. [Crossref] [PubMed]
- Schold JD, Kaplan B, Baliga RS, et al. The broad spectrum of quality in deceased donor kidneys. Am J Transplant 2005;5:757-65. [Crossref] [PubMed]
- Jeldres C, Cardinal H, Duclos A, et al. Prediction of delayed graft function after renal transplantation. Can Urol Assoc J 2009;3:377-82. [Crossref] [PubMed]
- Rao PS, Schaubel DE, Guidinger MK, et al. A comprehensive risk quantification score for deceased donor kidneys: the kidney donor risk index. Transplantation 2009;88:231-6. [Crossref] [PubMed]
- Chapal M, Le Borgne F, Legendre C, et al. A useful scoring system for the prediction and management of delayed graft function following kidney transplantation from cadaveric donors. Kidney Int 2014;86:1130-9. [Crossref] [PubMed]
- Dare AJ, Pettigrew GJ, Saeb-Parsy K. Preoperative assessment of the deceased-donor kidney: from macroscopic appearance to molecular biomarkers. Transplantation 2014;97:797-807. [Crossref] [PubMed]
- Michalak M, Wouters K, Fransen E, et al. Prediction of delayed graft function using different scoring algorithms: A single-center experience. World J Transplant 2017;7:260-8. [Crossref] [PubMed]
- Damman J, Bloks VW, Daha MR, et al. Hypoxia and Complement-and-Coagulation Pathways in the Deceased Organ Donor as the Major Target for Intervention to Improve Renal Allograft Outcome. Transplantation 2015;99:1293-300. [Crossref] [PubMed]
- McGuinness D, Mohammed S, Monaghan L, et al. A molecular signature for delayed graft function. Aging Cell 2018;17:e12825 [Crossref] [PubMed]
- Barrett T, Wilhite SE, Ledoux P, et al. NCBI GEO: archive for functional genomics data sets--update. Nucleic Acids Res 2013;41:D991-5. [Crossref] [PubMed]
- DiRito JR, Hosgood SA, Tietjen GT, et al. The future of marginal kidney repair in the context of normothermic machine perfusion. Am J Transplant 2018;18:2400-8. [Crossref] [PubMed]
- World Medical Association. World Medical Association Declaration of Helsinki: ethical principles for medical research involving human subjects. JAMA 2013;310:2191-4. [Crossref] [PubMed]
- Parikh CR, Jani A, Mishra J, et al. Urine NGAL and IL-18 are predictive biomarkers for delayed graft function following kidney transplantation. Am J Transplant 2006;6:1639-45. [Crossref] [PubMed]
- Oda T, Ishimura T, Yokoyama N, et al. Hypoxia-Inducible Factor-1alpha Expression in Kidney Transplant Biopsy Specimens After Reperfusion Is Associated With Early Recovery of Graft Function After Cadaveric Kidney Transplantation. Transplant Proc 2017;49:68-72. [Crossref] [PubMed]
- van Balkom BWM, Gremmels H, Ooms LSS, et al. Proteins in Preservation Fluid as Predictors of Delayed Graft Function in Kidneys from Donors after Circulatory Death. Clin J Am Soc Nephrol 2017;12:817-24. [Crossref] [PubMed]
- Mueller T, Regele H, Posch M, et al. HSP-72 expression in pre-transplant donor kidney biopsies and post-transplant outcome. Transplantation 2004;78:292-5. [Crossref] [PubMed]
- Kusaka M, Kuroyanagi Y, Ichino M, et al. Serum tissue inhibitor of metalloproteinases 1 (TIMP-1) predicts organ recovery from delayed graft function after kidney transplantation from donors after cardiac death. Cell Transplant 2010;19:723-9. [Crossref] [PubMed]
- Quaglia M, Merlotti G, Guglielmetti G, et al. Recent Advances on Biomarkers of Early and Late Kidney Graft Dysfunction. Int J Mol Sci 2020;21:5404. [Crossref] [PubMed]
- Nieuwenhuijs-Moeke GJ, Pischke SE, Berger SP, et al. Ischemia and Reperfusion Injury in Kidney Transplantation: Relevant Mechanisms in Injury and Repair. J Clin Med 2020;9:253. [Crossref] [PubMed]
- Nashan B, Abbud-Filho M, Citterio F. Prediction, prevention, and management of delayed graft function: where are we now? Clin Transplant 2016;30:1198-208. [Crossref] [PubMed]
- Kai Y, Tomoda K, Yoneyama H, et al. RNA interference targeting carbohydrate sulfotransferase 3 diminishes macrophage accumulation, inhibits MMP-9 expression and promotes lung recovery in murine pulmonary emphysema. Respir Res 2015;16:146. [Crossref] [PubMed]
- You H, Li T, Zhang J, et al. Reduction in ischemic cerebral infarction is mediated through golgi phosphoprotein 3 and Akt/mTOR signaling following salvianolate administration. Curr Neurovasc Res 2014;11:107-13. [Crossref] [PubMed]
- Hayward A, Ghazal A, Andersson G, et al. ZBED evolution: repeated utilization of DNA transposons as regulators of diverse host functions. PLoS One 2013;8:e59940 [Crossref] [PubMed]
- Zeng CM, Chang LL, Ying MD, et al. Aldo-Keto Reductase AKR1C1-AKR1C4: Functions, Regulation, and Intervention for Anti-cancer Therapy. Front Pharmacol 2017;8:119. [Crossref] [PubMed]
- Ma P, Zhang C, Huo P, et al. A novel role of the miR-152-3p/ERRFI1/STAT3 pathway modulates the apoptosis and inflammatory response after acute kidney injury. J Biochem Mol Toxicol 2020; [Epub ahead of print]. [Crossref] [PubMed]
- Gerken ALH, Nowak K, Meyer A, et al. Quantitative Assessment of Intraoperative Laser Fluorescence Angiography with Indocyanine Green Predicts Early Graft Function after Kidney Transplantation. Ann Surg 2020; [Epub ahead of print]. [Crossref] [PubMed]
- Hu F, Li J, Zhang Z, et al. Smartphone-Based Droplet Digital LAMP Device with Rapid Nucleic Acid Isolation for Highly Sensitive Point-of-Care Detection. Anal Chem 2020;92:2258-65. [Crossref] [PubMed]