Significance of TP53 and immune-related genes to prostate cancer
Introduction
Prostate cancer (PCa) is the most frequently diagnosed male cancer in 105 countries, ranking as the second most frequent cancer and the fifth leading cause of cancer death in men (1-3). Clinically, a great number of patients are diagnosed at an advanced stage, because there is no obvious symptom in the early stage. In recent years, prostate-specific antigen testing has increased the number of men diagnosed with and treated for PCa (2).
Genomic analysis is a commonly used method in cancer research worldwide, and PCa is no exception (4-7). A lot of research has been done on over-expressed genes and upregulated signal pathways in PCa, but the exact pathogenesis of the tumor is still unclear (4,5). However, some studies’ results have been translated into clinical treatments that have significantly prolonged the survival of PCa patients (3,6,8,9).
Although many factors are considered to influence prognosis, due to the current promotion of individualized treatments, but treatment plans are now more precise, and more and more patients who were considered to have a poor prognosis have benefited significantly (8). Thanks to The Cancer Genome Atlas (TCGA) and GEO databases, we can further understand the genetic differences in PCa patients, such as TP53 (4,7). Compared with the differences in clinical characteristics, genomics has shown a more accurate prediction of survival prognosis in many cancers (4-6), and A great many cancers have significantly improved prognosis since immunotherapy has been applied in clinics, revealing the giant potential of immune factors in the prognosis of cancer patients (3,6,8-10). In this study, we used a database of immune genetics, and Cox proportional hazard regression models to analyze the prognostic risk factors and establish a prognostic model.
We present the following article in accordance with the REMARK reporting checklist (available at http://dx.doi.org/10.21037/tau-21-179).
Methods
Data of PCa genes
We searched for PCa gene-expression data in TCGA using the UCSC Xena browser (https:xenabrowser.net) and downloaded both genetic and clinical information. The corresponding somatic mutation data was obtained through analysis of MuTect2. For research we also obtained the human HG38 v22 version of the gene annotation message in the GENCODE database. To test our results, we gathered a treatment-naive cohort of samples from patients with PCa from a GEO set (GSE116918).
Data of immune-related genes (IRGs)
IRGs were searched for in the Immunology Database and Analysis Portal (ImmPort) (https://www.immport.org/home) and a list of them was downloaded. In total, we had 1,811 IRGs.
Statistical analysis
Screening of differentially expressed (DE) IRGs
We first divided all samples into two groups based on the presence or absence of TP53 mutations. For further study, we used the R package edgeR to screen for DE genes between samples with and without TP53 mutations, requiring a fold-change (FC) >1.5 or FC <0.667, and requiring FDR (false discovery rate)-corrected P values <0.05. We excluded non-immune related genes using the list of IRGs.
Survival analysis
For convenience, we performed log2 (FPKM+1) conversion on the expression value of each gene. In order to select the genes tightly associated with survival prognosis, we used the R package coxph to analyze overall survival prognosis for all subjects, requiring P values <0.05.
Immune-related survival risk score
The immune genes related to survival prognosis were used to construct a prognosis score by weighting, and we divided the sample into high- and low-risk groups based on the median risk score, where Expi represents the expression value of the gene, and βi represents the weight of the gene expression value:
Immune cell ratio analysis
With the discovery of the tumor microenvironment, the cells surrounding the tumor have become a new research target (10-13). According to research, the infiltration of immune cells into tumors is closely related to the prognosis of patients and the efficacy of immunotherapy (11,13). To confirm the ratio of immune cells in PCa, we used CIBERSORT (https://cibersort.stanford.edu/). In order to make the results more credible, the expressed differences between high- and low-risk groups were determined by t-test, and the P values <0.05 were considered statistically significant.
Tumor mutational burden (TMB)
The TMB, which is regarded as an indicator for immunotherapy, is also used in PCa (14). According to the definition of TMB, we removed synonymous mutations and intron mutations from the PCa mutation data. We calculated the TMB score for both every single sample and the total samples (Table S1):
The study was conducted in accordance with the Declaration of Helsinki (as revised in 2013).
Results
Identification of DE immune genes
The mutation data downloaded from TCGA was first used to calculate the overall TMB score, which was 1.16. After analysis, we found a number of mutant genes, among which TP53 had the highest mutation frequency (Figure 1).
Using edgeR, a total of 3,101 DE genes (1,089 upregulated; 2,012 downregulated) were screened from samples with and without TP53 mutations (Figures 2,3).
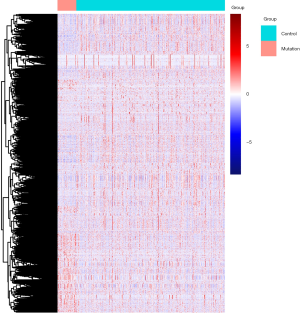
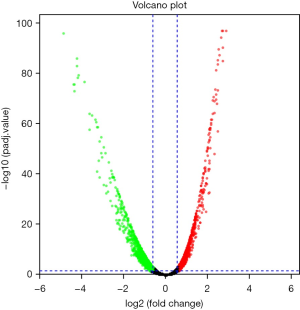
There were 1,811 IRGs downloaded from the ImmPort database, and we found 178 genes (84 upregulated; 94 downregulated) that simultaneously matched the differential expression in the TP53 mutant samples of PCa and immune correlation (Figures 4,5).
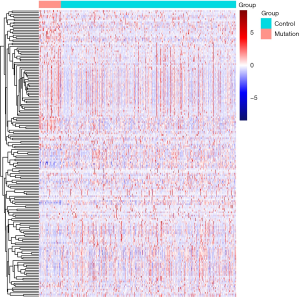
Enrichment analysis of DE IRGs
The GO(Gene Ontology) term ‘enrichment analysis’ showed that DE IRGs were mainly concentrated in immune response, complement activation, immunoglobulin etc. Moreover, they were also enriched in some pathways, such as neuroactive ligand-receptor interactions, cytokine ligand-receptor interactions, and chemokine signaling pathways (Figures 6,7, Figure S1).
Through previous analysis, we already had 178 target genes. Based on the patient’s clinical data, we performed a survival analysis. In the results, there were significant differences in the survival prognosis of six genes (P value <0.05).Three genes (DES, HBEGF and OPRK1) positively correlated with survival prognosis On the contrary, the other three genes (CALCB, OBP2A and UCN3) negatively affected prognosis (Table 1, Figure 8).
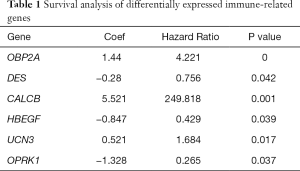
Full table
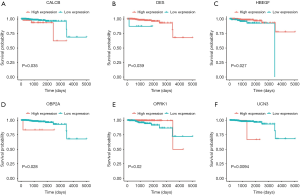
Risk score for patients with PCa
The six identified genes were used to calculate each patient’s risk score through weighting. Using these scores, we regrouped all patients as high risk or low risk. There was a significant difference in survival prognosis between groups (P value =0.0072). The high-risk group had a worse survival prognosis, and the low-risk group had a better survival prognosis (Figures 9,10).
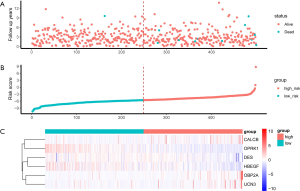
External dataset validation
The GSE116918 dataset was used to verify the accuracy of our risk score. Prior to validation, we used the optimal threshold (risk score =17.21523) to distinguish between the high and low risk groups. As a result, we found that the high-risk group had a significantly lower survival prognosis than the low-risk group in this dataset (P value =0.037) (Figure 11, Figure S2).
Prognostic performance of PCa risk score
In TCGA’s PCa data, the risk score made good predictions of patient’s 1-, 3-, and 5-year survival. The same conclusion was also obtained with the GSE116818 dataset (Figure 12, Figure S3).
Survival analysis of clinical factors of PCa
Afterwards, we classified all kinds of clinical factors, and found through survival analysis that each clinical factor has no significant difference in survival prognosis, but our risk score can have a good prediction of survival prognosis, which again proves that we have constructed The prognosis score was better than clinical factors. It is of great help to the clinic (Figures 13,14).
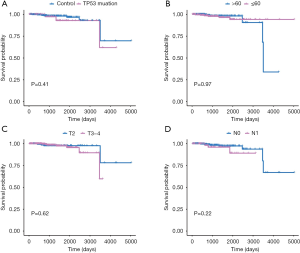
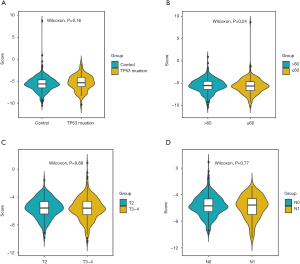
GSEA enrichment analysis of high and low risk groups
We performed Gene Set Enrichment Analysis (GSEA) to DE genes in high and low risk groups and found that both groups had some over-expressed genes, which were involved in many biochemical reaction. Genes that are highly expressed in the high-risk group are mainly involved in macrophage activation, epithelial cell apoptosis, and inflammatory response. In the low-risk group, the over-expressed genes mainly participate in nucleotide phosphorylation, tRNA metabolism, and mitochondrial gene expression (Figure 15).
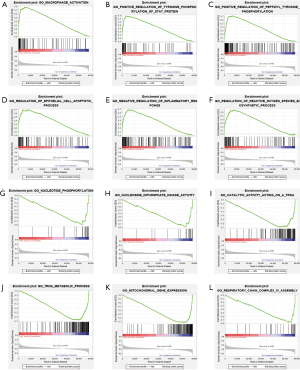
Comparison of the proportion of immune infiltrating cells
We analyzed in detail the difference in the proportion of immune cell infiltration between the two groups, but only found that the mast cell active had the significant differences. TIDE, a computational method to model two primary mechanisms of tumor immune evasion, predicted the outcome of melanoma patients treated with first-line anti-PD1 or anti-CTLA4 more accurately than other biomarkers such as PD-L1 level and mutation load (15). So we used the TIDE score (http://tide.dfci.harvard.edu/) to predict the efficacy of immunotherapy in high- and low-risk groups of PCa. However, there was no significant difference between the two groups (P value =0.7) (Figure 16, Figure S4).
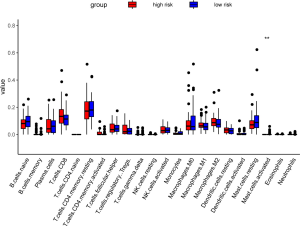
Nomogram of PCa
Through univariate Cox regression analysis for TP53 gene mutation, age, T stage, N stage, and high- and low-risk group in patients with PCa. Excitingly, requiring P value <0.05, we found that the risk group had more significant ability to predict survival prognosis (Table S2).
Finally, a nomogram of PCa was constructed, and the survival prognosis of patients was assisted by scoring their various factors (Figure 17).
Discussion
PCa is a high-profile cancer in man with an increasing morbidity and mortality. In this topic, we constructed a prognostic model through the DE immune related genes significantly associated with survival. If we regarded the median score as the demarcation between high and low risk groups, there was no significant difference in two groups from the GSE116918. So we reselected the optimal threshold (risk score =17.21523) to define high and low risk groups, and the result showed that the survival difference between high and low risk groups is significant. Judgment of prognosis is powerlessly only by clinical indicators, nevertheless our prognostic score can offer an excellent assessment for patients.
The results of this study have yet to be further confirmed by prospective studies or the inclusion of more experimental subjects. The occurrence and progression of cancer is the combined effect of a variety of gene abnormalities, including the loss or silencing of tumor suppressor genes, and the amplification or activation of proto-oncogenes. The regulation of gene expression in cancer cells is also significantly different from normal cells, such as miRNA and lncRNA. Prostate cancer is heterogeneous, which limits the efficacy of drugs. Therefore, the treatment of prostate should be precise and individualized. Adjuvant treatment plans should be formulated comprehensively based on the results of gene sequencing, combined with molecular subtypes.
Conclusions
The mutation of TP53 and the abnormal expression of some related immune genes have a significant impact on the prognosis of prostate cancer patients. DES, HBEGF, OPRK1, CACLB, OBP2A and UCN3 are prognostic-related genes, and these genes are closely related to the immune response. The comprehensive score of these six genes serves as the criterion for defining the high and low risk groups. The prognosis of the high-risk group is much worse than that of the low-risk group.
Acknowledgments
Funding: This research was supported by Zhejiang Provincial Natural Science Foundation of China under Grant No. LQ20H050001 and LY20H160013. This study was supported by Wenzhou Science and Technology Project (Y20190066).
Footnote
Reporting Checklist: The authors have completed the REMARK reporting checklist. Available at http://dx.doi.org/10.21037/tau-21-179
Conflicts of Interest: All authors have completed the ICMJE uniform disclosure form (available at http://dx.doi.org/10.21037/tau-21-179). The authors have no conflicts of interest to declare.
Ethical Statement: The authors are accountable for all aspects of the work in ensuring that questions related to the accuracy or integrity of any part of the work are appropriately investigated and resolved. The study was conducted in accordance with the Declaration of Helsinki (as revised in 2013).
Open Access Statement: This is an Open Access article distributed in accordance with the Creative Commons Attribution-NonCommercial-NoDerivs 4.0 International License (CC BY-NC-ND 4.0), which permits the non-commercial replication and distribution of the article with the strict proviso that no changes or edits are made and the original work is properly cited (including links to both the formal publication through the relevant DOI and the license). See: https://creativecommons.org/licenses/by-nc-nd/4.0/.
References
- Bray F, Ferlay J, Soerjomataram I, et al. Global cancer statistics 2018: GLOBOCAN estimates of incidence and mortality worldwide for 36 cancers in 185 countries. CA Cancer J Clin 2018;68:394-424. Erratum in: CA Cancer J Clin 2020;70:313. [Crossref] [PubMed]
- Falzarano SM, Ferro M, Bollito E, et al. Novel biomarkers and genomic tests in prostate cancer: a critical analysis. Minerva Urol Nefrol 2015;67:211-31. [PubMed]
- Schatten H. Immunodiagnostics and Immunotherapy Possibilities for Prostate Cancer. Adv Exp Med Biol 2018;1096:185-94. [Crossref] [PubMed]
- Spans L, Van den Broeck T, Smeets E, et al. Genomic and epigenomic analysis of high-risk prostate cancer reveals changes in hydroxymethylation and TET1. Oncotarget 2016;7:24326-38. [Crossref] [PubMed]
- Jividen K, Kedzierska KZ, Yang CS, et al. Genomic analysis of DNA repair genes and androgen signaling in prostate cancer. BMC Cancer 2018;18:960. [Crossref] [PubMed]
- Patel VG, Oh WK. The evolving landscape of immunotherapy in advanced prostate cancer. Immunotherapy 2019;11:903-12. [Crossref] [PubMed]
- Hamid AA, Gray KP, Shaw G, et al. Compound Genomic Alterations of TP53, PTEN, and RB1 Tumor Suppressors in Localized and Metastatic Prostate Cancer. Eur Urol 2019;76:89-97. [Crossref] [PubMed]
- Marshall CH, Antonarakis ES. Emerging treatments for metastatic castration-resistant prostate cancer: Immunotherapy, PARP inhibitors, and PSMA-targeted approaches. Cancer Treat Res Commun 2020;23:100164 [Crossref] [PubMed]
- Cha HR, Lee JH, Ponnazhagan S. Revisiting Immunotherapy: A Focus on Prostate Cancer. Cancer Res 2020;80:1615-23. [Crossref] [PubMed]
- Papanicolau-Sengos A, Yang Y, Pabla S, et al. Identification of targets for prostate cancer immunotherapy. Prostate 2019;79:498-505. [Crossref] [PubMed]
- Prokhnevska N, Emerson DA, Kissick HT, et al. Immunological Complexity of the Prostate Cancer Microenvironment Influences the Response to Immunotherapy. Adv Exp Med Biol 2019;1210:121-47. [Crossref] [PubMed]
- Venturini NJ, Drake CG. Immunotherapy for Prostate Cancer. Cold Spring Harb Perspect Med 2019;9:a030627 [Crossref] [PubMed]
- Vitkin N, Nersesian S, Siemens DR, et al. The Tumor Immune Contexture of Prostate Cancer. Front Immunol 2019;10:603. [Crossref] [PubMed]
- Ryan MJ, Bose R. Genomic Alteration Burden in Advanced Prostate Cancer and Therapeutic Implications. Front Oncol 2019;9:1287. [Crossref] [PubMed]
- Jiang P, Gu S, Pan D, et al. Signatures of T cell dysfunction and exclusion predict cancer immunotherapy response. Nat Med 2018;24:1550-8. [Crossref] [PubMed]
(English Language Editor: K. Brown)