A nomogram based on PI-RADS v2.1 and clinical indicators for predicting clinically significant prostate cancer in the transition zone
Introduction
Multiparametric magnetic resonance imaging (mp-MRI) is the most reliable noninvasive imaging technology for the diagnosis and evaluation of prostate cancer (PCa). The Prostate Imaging Reporting and Data System version 2 (PI-RADS v2) was released to improve the reporting standardization of mp-MRI of the prostate (1). In recent years, a simplified biparametric MRI (bp-MRI) comprising T2-weighted imaging (T2WI) and diffusion-weighted imaging (DWI) has been proposed (2), with the advantages of decreasing the examination time and avoiding potential adverse effects of gadolinium-based contrast agents (3,4). Thus, the bp-MRI protocol is increasingly being used for the detection of PCa, especially clinically significant prostate cancer (cs-PCa, defined as Gleason score ≥7) (5). Nevertheless, the moderate interreader agreement using PI-RADS v2 has hindered its widespread application (6).
The majority of PCa cases arise in the peripheral zone (PZ), and less than 30% of cases are located in the transition zone (TZ) (7). As the detection of PCa by MRI in the TZ is susceptible to being affected by benign prostatic hyperplasia (BPH) and other factors, the numbers of false-positive and false-negative cases are high, resulting in high tumor misdiagnosis rates in the TZ. Related literature has demonstrated that interreader agreement with PI-RADS v2 in the TZ is lower than that in the PZ (8,9). To address this limitation, PI-RADS version 2.1 was proposed, which made a few modifications for typical and atypical nodules in the TZ to improve the performance of TZ lesion categorization (10,11). A new study confirmed some improvements of PI-RADS v2.1 over PI-RADS v2 in diagnostic performance and interreader agreement. Nevertheless, the diagnostic sensitivity of using PI-RADS v2.1 alone at the best cutoff value of 4 for cs-PCa in the TZ was 63.0%, resulting in a poor diagnostic performance for TZ lesions (12). Hence, it is necessary to combine the PI-RADS v2.1 score with other clinical indicators [e.g., patient age, serum total prostate-specific antigen (t-PSA), percent free PSA, PSA density (PSAD) and prostate volume (PV)] to comprehensively diagnose TZ lesions. Previous studies have shown that the PI-RADS v2 score in combination with multiple clinical indicators can significantly improve the diagnostic efficacy of cs-PCa (13-15). To the best of our knowledge, few published studies have focused on a combination of the PI-RADS v2.1 score with clinical indicators to improve the diagnostic value of TZ cs-PCa.
Nomograms are useful computational tools for the visualization of models, the graphical assessment of variable importance and the calculation of predicted values, and they have been used in a variety of tumors, including PCa (16,17). The aim of this study was to establish a nomogram incorporating the PI-RADS v2.1 score based on bp-MRI and clinical factors that could be used to predict the probability of TZ cs-PCa.
We present the following article in accordance with the TRIPOD reporting checklist (available at http://dx.doi.org/10.21037/tau-21-49).
Methods
Study population
The study was conducted in accordance with the Declaration of Helsinki (as revised in 2013). The study was approved by institutional ethics board of our hospital (No.: JD-HG-2021-21) and individual consent for this retrospective analysis was waived. We retrospectively identified 988 consecutive patients who underwent biparametric MRI, and pathological results were obtained by systematic transrectal ultrasound (TRUS)-guided prostate biopsy and/or MRI-TRUS fusion targeted biopsy between January 2015 and March 2020 in our institution. All patients had either elevated PSA levels or abnormal findings on digital rectal examination (DRE). After excluding 414 patients who had a dominant lesion in the PZ, 574 eligible patients who had a dominant lesion in the TZ were included in this study. The selection of the dominant lesion was based on the highest PI-RADS v2 category according to the original MRI interpretation. In addition, 63 patients met the exclusion criteria, so the remaining 511 patients were enrolled and randomly divided into 383 patients in the training cohort and 128 patients in the validation cohort. The study population flowchart with the exclusion criteria is shown in Figure 1.
Bp-MRI acquisition protocol
All bp-MRI examinations were performed using a 32-channel body phased-array coil on a 3.0 T MRI scanner (Philips Ingenia, The Netherlands). According to the PI-RADS v2.1 criteria, the acquisition protocol included the following sequences: a high-resolution T2WI sequence acquired with axial (repetition time, 3,000 ms; echo time, 100 ms; field of view, 220 mm × 220 mm; slice thickness, 3 mm; no slice gap) and sagittal (repetition time, 4,978 ms; echo time, 100 ms; field of view, 240 mm × 180 mm; slice thickness, 1.5 mm; slice gap, 0.15 mm) orientations. DWI sequences with an axial orientation (repetition time, 6,000 ms; echo time, 77 ms; field of view, 260 mm × 260 mm; slice thickness, 3 mm; no slice gap) and multiple b values (b=0, 100, 1,000, 2,000 s/mm2) were acquired, and b=100 and b=2000 s/mm2 were used for the visual assessment and analysis of the apparent diffusion coefficient (ADC) map axial images. The MRI scanning range included the prostate gland and the bilateral seminal vesicles.
PI-RADS assessment category
According to the PI-RADS v2.1 protocol (11), each bp-MRI image was independently reviewed by two experienced radiologists (radiologist 1 with 4 years of experience; radiologist 2 with 8 years of experience) who were blinded to the pathological results and clinical information. For the evaluation of the TZ lesions, T2WI plays a dominant role, while DWI is only a secondary sequence.
Collection of clinical information
Clinical data, including patient age, t-PSA, percent free PSA, PV and PSAD, were collected and assessed. For the measurement of PV on MRI, PI-RADS v2.1 recommends that the maximum anteroposterior diameter (APD) and longitudinal diameter (LD) be measured on midsagittal T2WI while the maximum transverse diameter (TD) measured on the axial T2WI. PV was estimated as follows:
PV = (maximum APD) × (maximum TD) × (maximum LD) ×0.52 (11,18).
PSAD was calculated by the level of t-PSA divided by the PV (PSAD = t-PSA/PV) (18).
Pathology
All patients underwent a 10-core systematic TRUS-guided prostate biopsy. In addition, MRI-TRUS fusion targeted biopsy was used for suspicious PCa lesions on MRI and 2–3 targeted cores were added for these lesions. MRI-TRUS fusion targeted biopsy was performed using an ESAOTE Mylab Twice color Doppler ultrasound device equipped with a real-time virtual sonography (RVS) imaging fusion system. The ultrasonic probe model was an EC-123,7.5-MHz transrectal end-fire probe (EsaoteSpA, Genova, Italy). The prostate biopsy procedure was performed by a senior urologist with over 5 years of experience. The specimens were assessed by experienced pathologists in our institution according to the International Society of Urological Pathology (ISUP) 2014 updated Gleason score grading system (19,20).
Statistical analysis
Statistical Package for Social Science (SPSS) 22.0 and MedCalc version 15.2.2 were used for the statistical analysis. Continuous variables with normal distributions were analyzed by the independent samples t-test and are expressed as the mean and standard deviation. Categorical variables or continuous variables with nonnormal distributions were analyzed using a nonparametric test and are presented as the median (interquartile ranges). The interreader agreement of the PI-RADS v2.1 score was evaluated by weighted Cohen’s kappa statistic. Kappa values of 0–0.20 were regarded as slight, 0.21–0.40 as fair, 0.41–0.60 as moderate, 0.61–0.80 as substantial, and 0.81–1 as almost perfect agreement. Multivariable binary logistic regression analysis was performed to determine the significant independent predictors, and the areas under the receiver operating characteristic curve (AUCs) were calculated and compared to determine the diagnostic performance of the variables for cs-PCa in the TZ. The diagnostic sensitivity and specificity with corresponding 95% confidence intervals (CIs) were calculated. Using R software (version 3.6.2, http://www.r-project.org/), a nomogram was newly constructed based on the independent predictors in the training cohort. The nomogram was validated based on the discrimination and calibration using the AUC and the calibration curve in the training and validation cohorts. A higher AUC showed a better discriminatory capacity for prediction outcomes. The calibration curve was used to compare the nomogram-predicted and actual outcomes. A P value less than 0.05 was considered statistically significant.
Decision curve analysis was conducted to determine the clinical usefulness of the newly constructed nomogram by quantifying the clinical net benefits at different threshold probabilities in the training and validation cohorts. Decision curves were also plotted for the individual predictors and the prediction model, which were also performed using R software.
Results
Patient characteristics
The characteristics of all patients with TZ lesions in the training and validation cohorts are shown in Table 1. Overall, 511 patients were enrolled in this study (383 patients in the training cohort; 128 patients in the validation cohort). In the training cohort, 94 (24.5%) patients had a confirmed diagnosis of PCa, including 62 with cs-PCa and 32 with low-grade PCa (Gleason score 3+3). A total of 289 (75.5%) patients had noncancerous lesions (208 with BPH and 81 with acute or chronic prostatitis). In the validation cohort, there were 26 patients diagnosed with PCa (12 with cs-PCa and 14 with low-grade PCa) and 102 patients with noncancerous lesions (76 with BPH and 26 with acute or chronic prostatitis). In the training cohort, the P values of age, t-PSA, percent free PSA, PSAD, PV and PI-RADS v2.1 score between cs-PCa vs. low-grade PCa and noncancerous lesions were less than 0.001, indicating a significant difference between the two groups (P<0.05).
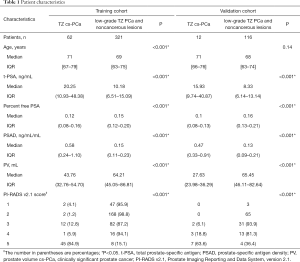
Full table
PI-RADS v2.1 score based on bp-MRI
The assessment of the PI-RADS v2.1 score between the two radiologists is shown in Table 2. The weighted kappa value of the interreader agreement for the PI-RADS v2.1 score was 0.648, which indicated that the consistency was substantial.
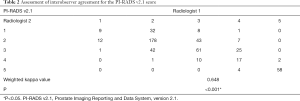
Full table
Multivariable logistic regression analysis of cs-PCa in the TZ
Although these univariate indicators were significantly different between patients with cs-PCa vs. low-grade PCa and noncancerous lesions, it was not appropriate to directly integrate them into the multivariable model due to the strong correlations between several variables (f/t-PSA was derived from t-PSA. PSAD was calculated by dividing t-PSA by PV). To avoid incorporating a confounding factor and to better handle strongly correlated variables, the multivariable model eliminated t-PSA and PV with a smaller AUC (AUCt-PSA =0.756, AUCPV =0.299) than PSAD (AUCPSAD =0.842). The final variables, including age, f/t-PSA, PSAD and PI-RADS v2.1 score, were incorporated into the multivariable logistic regression analysis, and then the independent predictors for cs-PCa in the TZ were determined. As shown in Table 3, the significant independent predictors for cs-PCa in the TZ were the PI-RADS v2.1 score and PSAD (P<0.05).
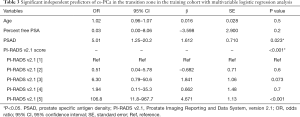
Full table
Construction and validation of a nomogram
Based on the PI-RADS v2.1 score and PSAD as independent predictors, a nomogram was constructed using the training cohort, and the risk for predicting cs-PCa in the TZ was calculated, as shown in Figure 2. The discrimination and calibration of the nomogram were validated in the training and validation cohorts. The AUCs of the nomogram were 0.936 in the training cohort (Figure 3A) and 0.963 in the validation cohort (Figure 3B), indicating that the model had good predictive accuracy. The calibration of the nomogram was internally performed by a calibration curve with bootstrap sampling (n=500). The calibration curve showed good consistency between the predicted probability of the model and the actual probability of TZ cs-PCa (Figure 3C). In addition, Figure 3D shows the comparison between the nomogram-predicted probability and the actual probability in the validation cohort, which indicated a good performance. The decision curve analysis is presented in Figure 3E,F and shows that the net benefits of the prediction model were higher than the individual predictors when the threshold probability exceeded 0.25 in the training cohort and 0.50 in the validation cohort.
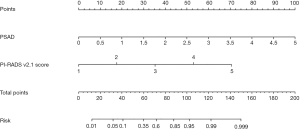
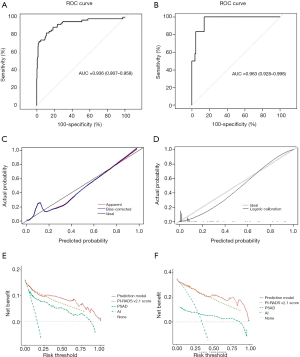
Figures 4 and 5 show two patients with a PI-RADS v2.1 score of 3. Based on the PI-RADS v2.1 score alone, their risk of clinically significant prostate cancer is considered to be relatively low. Combined with PSAD, according to the prediction model of the nomogram, the risk of cs-PCa in the patient in Figure 4 was calculated as 0.05, and the risk in the patient in Figure 5 was approximately 0.30. According to the prediction results, the risk of having cs-PCa in the patient in Figure 5 is 6 times that of the patient shown in Figure 4. The pathological results confirmed that the patient in Figure 5 had cs-PCa with a Gleason score of 4+3.
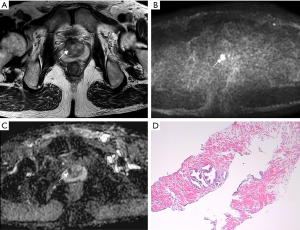
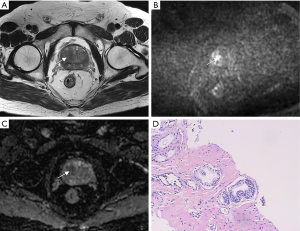
Diagnostic value of the prediction model for cs-PCa in the TZ
The prediction model (combining PI-RADS v2.1 score and PSAD) showed the highest AUC (AUC =0.936), which was significantly different compared with those of the PI-RADS v2.1 score (AUC =0.914, P=0.045) and PSAD (AUC =0.842, P<0.001). The diagnostic values of the independent indicators and prediction model for cs-PCa in the TZ were evaluated and compared (Table 4). At the best threshold of 4, the PI-RADS v2.1 score had 74.2% sensitivity and 92.5% specificity for diagnosing cs-PCa in the TZ. However, the prediction model (a combination of PI-RADS v2.1 score and PSAD) had 80.7% sensitivity and 93.8% specificity for diagnosing TZ cs-PCa, indicating that adding PSAD to the PI-RADS v2.1 score could improve the diagnostic performance for cs-PCa in the TZ.
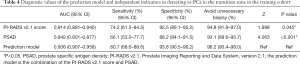
Full table
Discussion
The prostate Imaging Reporting and Data System (PI-RADS), as a structured reporting system for prostate MRI, has been widely used in clinical research. Although PI-RADS v2 had prominent advantages over PI-RADS v1 in reducing ambiguity for reporting interpretations and optimizing interdisciplinary communication, it still had some limitations and inconsistencies, particularly related to the ambiguous characterization for the evaluation of TZ lesions, making it necessary to develop the latest version (PI- RADS v2.1) in 2019 (11). As a small-step improvement from PI-RADS v2, one of the main purposes of PI-RADS v2.1 was to further reduce variability in reporting interpretations to improve interreader agreement and the diagnostic performance for TZ lesions. According to our results, the interreader agreement for TZ lesions using the PI-RADS v2.1 protocol was substantial (kappa value of 0.648) and was higher than that for PI-RADS v2, which had only moderate interreader agreement (all kappa values were less than 0.600) (6,9,12,21).
For the assessment of the diagnostic performance of PI-RADS v2.1, the results from Byun et al. demonstrated that the sensitivity and specificity of PI-RADS v2.1 category ≥4 were 63.0% and 96.9%, respectively, for diagnosing TZ cs-PCa (12). In this study, our results showed that the PI-RADS v2.1 score was one of the independent predictors for cs-PCa in the TZ, and the diagnostic sensitivity and specificity of using PI-RADS v2.1 category ≥4 to detect TZ cs-PCa were 74.2% and 92.5% respectively. Compared with Byun’s results, the discrepancy of the sensitivity and specificity may be related to the radiologists’ diagnostic experience and familiarity with the PI-RADS v2.1 scoring criteria for TZ lesions. These results indicated that using the PI-RADS v2.1 score alone for diagnosing cs-PCa in the TZ would inevitably result in false-positive and false-negative cases, largely because the results of the PI-RADS v2.1 score were closely associated with the reader’s diagnostic experience with MRI of the prostate. The subjective visual assessment of the images tended to increase the discrepancy between the readers. Hence, it was necessary to combine the PI-RADS v2.1 score with objective clinical indicators to improve the diagnostic value for cs-PCa in the TZ.
Our results also revealed that PSAD was another independent predictor of TZ cs-PCa. As a commonly used clinical indicator, previous studies have proven that PSAD plays a positive role in combined diagnosis of PCa or cs-PCa (22,23). We combined the PI-RADS v2.1 score and PSAD and constructed a new nomogram with a subjective bp-MRI visual category and an objective test indicator to predict the possibility of cs-PCa in the TZ. In the training cohort, by incorporating the PI-RADS v2.1 score and PSAD into a prediction model, the diagnostic performance for cs-PCa in the TZ could be improved with an AUC of 0.936, which was significantly higher than that of the PI-RADS v2.1 score (AUC =0.914) and PSAD (AUC =0.842). Furthermore, the prediction model could improve its diagnostic sensitivity from 74.2% to 80.7% and its specificity from 92.5% to 93.8% compared with the PI-RADS v2.1 score alone. Our results were similar to the study reported by Han et al. (24). They compared the performance of the PI-RADS v2.1 categories based on the mp-MRI or bp-MRI protocol in combination with PSAD in detecting cs-PCa in patients with PSA levels of 4–10 ng/mL and found that the combination of bp-MRI and PSAD showed the highest AUC (AUC =0.907) and an increased diagnostic sensitivity (89.2%) but a slightly decreased diagnostic specificity (87.2%) compared with bp-MRI alone (sensitivity, 81.1%; specificity, 88.4%).
The newly constructed nomogram incorporating the PI-RADS v2.1 score and PSAD was established and validated in the training and validation cohorts. The nomogram is a perfect example of integrating multiple related parameters to predict a specific end point by means of geometry graphs to visualize the results of the prediction model. With the nomogram, we could intuitively obtain a patient’s corresponding risk value for TZ cs-PCa on the prediction line at the bottom of the nomogram, which can then be used to guide urologists’ decision on whether to conduct a biopsy. The AUC of the nomogram was 0.963 for the validation cohort, indicating that the constructed model has satisfactory predictive accuracy for cs-PCa in the TZ. Furthermore, the calibration curve revealed good consistency between the actual outcomes and the predicted outcomes. In addition, for assessing the availability of the prediction model, we conducted the decision curve analysis. The results showed that using the prediction model to predict the risk of cs-PCa could obtain higher net benefit than treating all or no patient in most ranges of the threshold probabilities. The prediction model had more advantages in guiding physician decision-making.
However, there are several limitations in this study. First, although the present study included a relatively large number of patients with PCa in the TZ, it was a retrospective, single-center study, and patient selection bias may exist. Multicenter studies will be carried out to further validate these results. Second, MRI-TRUS fusion targeted biopsy was only performed for suspicious lesions on prostate MRI, which may miss potential lesions with a negative MRI but positive pathology. Third, the prediction model has not been validated by an independent external dataset and it requires further verification.
Conclusions
Our study constructed a novel internally validated nomogram to predict the probability of cs-PCa in the TZ based on the PI-RADS v2.1 score and PSAD. PI-RADS v2.1 based on bp-MRI is a strong predictor of TZ cs-PCa. In addition, adding PSAD to PI-RADS v2.1 could improve its diagnostic performance, thereby avoiding unnecessary biopsies.
Acknowledgments
We thank AJE Editing Service for editing this manuscript and Zhangyi Luo for his advice on R based data analysis.
Funding: This work was supported by financial grants from the National Natural Science Foundation of China (Grant No. 81801754); the Suzhou Science and Technology Development Plan (Grant No. SS2019012); the Project of State Key Laboratory of Radiation Medicine and Protection, Soochow University (Grant No. GZK1202023); and the Suzhou Science and Technology Bureau Development Plan (Grant No. SYS2020147).
Footnote
Reporting Checklist: The authors have completed the TRIPOD reporting checklist. Available at http://dx.doi.org/10.21037/tau-21-49
Data Sharing Statement: Available at http://dx.doi.org/10.21037/tau-21-49
Peer Review File: Available at http://dx.doi.org/10.21037/tau-21-49
Conflicts of Interest: All authors have completed the ICMJE uniform disclosure form (available at http://dx.doi.org/10.21037/tau-21-49). The authors have no conflicts of interest to declare.
Ethical Statement: The authors are accountable for all aspects of the work in ensuring that questions related to the accuracy or integrity of any part of the work are appropriately investigated and resolved. The study was conducted in accordance with the Declaration of Helsinki (as revised in 2013). The study was approved by institutional ethics board of our hospital (No.: JD-HG-2021-21) and individual consent for this retrospective analysis was waived.
Open Access Statement: This is an Open Access article distributed in accordance with the Creative Commons Attribution-NonCommercial-NoDerivs 4.0 International License (CC BY-NC-ND 4.0), which permits the non-commercial replication and distribution of the article with the strict proviso that no changes or edits are made and the original work is properly cited (including links to both the formal publication through the relevant DOI and the license). See: https://creativecommons.org/licenses/by-nc-nd/4.0/.
References
- Weinreb JC, Barentsz JO, Choyke PL, et al. PI-RADS Prostate Imaging - Reporting and Data System: 2015, Version 2. Eur Urol 2016;69:16-40. [Crossref] [PubMed]
- Kuhl CK, Bruhn R, Krämer N, et al. Abbreviated biparametric prostate MR imaging in men with elevated prostate-specific antigen. Radiology 2017;285:493-505. [Crossref] [PubMed]
- Stanzione A, Imbriaco M, Cocozza S, et al. Biparametric 3T magnetic resonance imaging for prostatic cancer detection in a biopsy naïve patient population: a further improvement of PI-RADS v2. Eur J Radiol 2016;85:2269-74. [Crossref] [PubMed]
- Junker D, Steinkohl F, Fritz V, et al. Comparison of multiparametric and biparametric MRI of the prostate: are gadolinium-based contrast agents needed for routine examinations? World J Urol 2019;37:691-9. [Crossref] [PubMed]
- Steiger P, Thoeny HC. Prostate MRI based on PI-RADS version 2: how we review and report. Cancer Imaging 2016;16:9. [Crossref] [PubMed]
- Rosenkrantz AB, Ginocchio LA, Cornfeld D, et al. Interobserver reproducibility of the PI-RADS version 2 lexicon: a multicenter study of six experienced prostate radiologists. Radiology 2016;280:793-804. [Crossref] [PubMed]
- McNeal JE, Redwine E, Freiha F, et al. Zonal distribution of prostatic adenocarcinoma: Correlation with histologic pattern and direction of spread. Am J Surg Pathol 1988;12:897-906. [Crossref] [PubMed]
- Mussi TC, Yamauchi FI, Tridente CF, et al. Interobserver agreement and positivity of PI-RADS version 2 among radiologists with different levels of experience. Acad Radiol 2019;26:1017-22. [Crossref] [PubMed]
- Muller BG, Shih JH, Sankineni S, et al. Prostate Cancer: Interobserver agreement and accuracy with the revised prostate imaging reporting and data system at multiparametric MR imaging. Radiology 2015;277:741-50. [Crossref] [PubMed]
- Barrett T, Rajesh A, Rosenkrantz AB, et al. PI-RADS version 2.1: one small step for prostate MRI. Clin Radiol 2019;74:841-52. [Crossref] [PubMed]
- Turkbey B, Rosenkrantz AB, Haider MA, et al. Prostate imaging reporting and data system version 2.1: 2019 update of prostate imaging reporting and data system version 2. Eur Urol 2019;76:340-51. [Crossref] [PubMed]
- Byun J, Park KJ, Kim MH, et al. Direct comparison of PI-RADS version 2 and 2.1 in transition zone lesions for detection of prostate cancer: preliminary experience. J Magn Reson Imaging 2020;52:577-86. [Crossref] [PubMed]
- Al-Azab R, Toi A, Lockwood G, et al. Prostate volume is strongest predictor of cancer diagnosis at transrectal ultrasound-guided prostate biopsy with prostate-specific antigen values between 2.0 and 9.0 ng/mL. Urology 2007;69:103-7. [Crossref] [PubMed]
- Distler FA, Radtke JP, Bonekamp D, et al. The value of PSA density in combination with PI-RADS™ for the accuracy of prostate cancer prediction. J Urol 2017;198:575-82. [Crossref] [PubMed]
- Polanec SH, Bickel H, Wengert GJ, et al. Can the addition of clinical information improve the accuracy of PI-RADS version 2 for the diagnosis of clinically significant prostate cancer in positive MRI? Clin Radiol 2020;75:157.e1-157.e7. [Crossref] [PubMed]
- Niu XK, Li J, Das SK, et al. Developing a nomogram based on multiparametric magnetic resonance imaging for forecasting high-grade prostate cancer to reduce unnecessary biopsies within the prostate-specific antigen gray zone. BMC Med Imaging 2017;17:11. [Crossref] [PubMed]
- Diao JD, Ma LX, Sun MY, et al. Construction and validation of a nomogram to predict overall survival in patients with inflammatory breast cancer. Cancer Med 2019;8:5600-8. [Crossref] [PubMed]
- Lee SJ, Oh YT, Jung DC, et al. Combined analysis of biparametric MRI and prostate-specific antigen density: role in the prebiopsy diagnosis of Gleason score 7 or greater prostate cancer. AJR Am J Roentgenol 2018;211:W166-W172. [Crossref] [PubMed]
- Schlenker B, Apfelbeck M, Buchner A, et al. MRI-TRUS fusion biopsy of the prostate: quality of image fusion in a clinical setting. Clin Hemorheol Microcirc 2018;70:433-40. [Crossref] [PubMed]
- Epstein JI, Egevad L, Amin MB, et al. The 2014 International Society of Urological Pathology (ISUP) Consensus Conference on Gleason Grading of Prostatic Carcinoma: Definition of Grading Patterns and Proposal for a New Grading System. Am J Surg Pathol 2016;40:244-52. [Crossref] [PubMed]
- Tamada T, Kido A, Takeuchi M, et al. Comparison of PI-RADS version 2 and PI-RADS version 2.1 for the detection of transition zone prostate cancer. Eur J Radiol 2019;121:108704 [Crossref] [PubMed]
- Kosaka T, Mizuno R, Shinojima T, et al. The implications of prostate-specific antigen density to predict clinically significant prostate cancer in men ≤ 50 years. Am J Clin Exp Urol 2014;2:332-6. [PubMed]
- Kubota Y, Kamei S, Nakano M, et al. The potential role of prebiopsy magnetic resonance imaging combined with prostate-specific antigen density in the detection of prostate cancer. Int J Urol 2008;15:322-6; discussion 327. [Crossref] [PubMed]
- Han C, Liu S, Qin XB, et al. MRI combined with PSA density in detecting clinically significant prostate cancer in patients with PSA serum levels of 4-10 ng/mL: Biparametric versus multiparametric MRI. Diagn Interv Imaging 2020;101:235. [Crossref] [PubMed]