Comprehensive analysis of alternative splicing profiling reveals novel events associated with prognosis and the infiltration of immune cells in prostate cancer
Introduction
Prostate cancer (PCa) is the second leading cause of cancer death in American men and threatens the health of men worldwide (1). With the recent lifestyle changes in China, the incidence of PCa has also increased over time (2). Patients with non-metastatic PCa, who are fit enough to receive curative treatment, have a good prognosis. Yet, approximately 30% patients with biochemical recurrence (BCR) after primary radical surgery develop clinical recurrence, with a mortality rate of 16.4% (3). Owing to PCa screening, increasing numbers of PCa patients can be diagnosed at an early stage and achieve clinical cure. Even for those asymptomatic advanced patients, early treatment intervention can improve patient prognosis. However, the prognosis of advanced patients is still very poor (4). Moreover, prostate tumors have significant heterogeneity among different individuals. For metastatic castration-resistant PCa (mCRPC), genomic aberrations may be similar or different from primary prostate tumors that are androgen deprivation treatment naïve (5). Multiple diverse genomic explorations are required to unveil the malignant features of PCa. Alternative splicing (AS) is ubiquitous in the biological process of post-transcriptional regulation, which influences the translation of mRNA isoforms and the generation of protein diversities (6). With the advent of next-generation sequencing, landscapes of aberrant AS events were revealed by bioinformatics analysis and exhibited up to 30% more AS events in primary tumors than adjacent normal tissues (7). Under pathological conditions, different splicing isoforms could generate proteins with varied structures and functions, some of which play important roles in the process of oncogenesis and tumor progression (8). Increasing evidence has demonstrated that cancer-specific splicing events and abnormal spliceosomes could be used as prognostic factors and therapeutic targets in tumor treatment management (9). In hepatocellular carcinoma (HCC), networks between RNA-binding protein (RBP) genes and their corresponding AS events are enriched in a series of metabolism-related pathways, playing vital roles in the development of HCC (10). The upregulation of splicing factor (SF) 3b subunit 3 (SF3B3) expression parallels the increased inclusion of enhancer of zeste 2 polycomb repressive complex 2 subunit (EZH2) exon 14. Enforced expression of EZH2∆14 inhibits, and EZH2 promotes, cell growth, migration, proliferation, and tumorigenicity (11). Frequent overlap of mutations in isocitrate dehydrogenase [NADP (+)] 2 (IDH2) and serine and arginine rich splicing factor 2 (SRSF2) promotes the oncogenesis of leukemia through the synergistic effect of epigenome and RNA splicing. Although mutations in IDH2 or SRSF2 confer different splicing changes, the co-expression of mutant IDH2 upregulates the splicing effect of mutant SRSF2, resulting in fatal myelodysplastic abnormalities with new proliferative characteristics (12).
Numerous studies have implicated the importance of AS in the development and progression of cancers (13). In mCRPC patients, the presence of alternatively spliced androgen receptor variant 7 (AR-V7) has been suggested to be associated with a declined efficiency of androgen deprivation therapy (14). Recently, cancer-specific AS had been widely recognized as feasible markers for predicting treatment efficacy. In squamous carcinoma , long noncoding RNA epidermal growth factor receptor (EGFR)-AS1 mediates EGFR addiction. Reduced EGFR-AS1 levels shift splicing toward EGFR isoform D, leading to a high response rate to EGFR tyrosine kinase inhibitor (TKIs) regardless of the defect of focal amplification or activated mutation in EGFR (15). Moreover, growing evidence has demonstrated that AS is not only correlated with the malignant phenotype of tumors, but also plays an important role in the immune microenvironment formation, affecting immune cell infiltration and crosstalk between tumor and immune cells (16). Hence, an improved understanding of AS and its clinical significance in PCa is urgently required.
The Cancer Genome Atlas (TCGA) SpliceSeq is a web-based database for investigating the AS events of TCGA tumors. Based on this database, we highlighted a systematic profiling of genome-wide AS events, thus providing a better understanding of the potential biological function and underlying regulatory mechanisms of these events. We also clarified the relationship between prognosis and the tumor microenvironment in patients with PCa. The results of this study may provide research clues for the development of novel therapeutic targets of PCa. We present the following article in accordance with the REMARK reporting checklist (available at https://dx.doi.org/10.21037/tau-21-585).
Methods
Acquisition of AS events profiling
The corresponding RNA-Seq profiles of PCa were generated from the TCGA data portal (https://tcga-data.nci.nih.gov/tcga/). Patients with histologically confirmed prostate adenocarcinoma were included in TCGA PCa cohort, and detailed clinical and follow-up information was also collected. The study was conducted in accordance with the Declaration of Helsinki (as revised in 2013). To quantify AS events, we used the Percent Spliced In (PSI) value to evaluate each type of AS event. After implementing splice event filters (percentage of samples with PSI values ≥75), PSI values were obtained from the TCGA SpliceSeq (https://bioinformatics.mdanderson.org/TCGASpliceSeq/singlegene.jsp). Subsequently, an UpSet plot was constructed to quantitatively analyze the interactive sets among different AS patterns using UpSetR (version 1.3.3) (17). For visualized analysis of AS events and genes in chromosomes, Circos plots were constructed using Circlize (version 0.4.11) (18).
Explorations of prognosis-associated AS events
UpSet plots were constructed to display the intersection of genes that may have multiple splicing events significantly associated with progression free survival (PFS). The hazard ratios (HRs) and 95% confidence interval (95% CI) of each PFS-related event were calculated by univariate Cox regression analysis, and the prognostic AS events were shown by volcano plot. A Venn diagram was constructed to exhibit AS event-associated genes between the high and low PSI groups. Cytoscape (version 3.7.0) (19) was used to visually display the networks based on the PSI values of AS events and the expressions of SFs, and P-values were adjusted by Benjamini-Hochberg correction (|R|≥0.4, adjusted P<0.05).
Survival analysis
PCa cohorts were grouped according to the median PSI value of each AS event. Least absolute shrinkage and selection operator (LASSO) regression analysis was used to screen highly correlated genes to prevent overfitting of prognostic model. AS events with P values less than 0.05 in the LASSO regression analysis were brought into the multivariate Cox regression analysis to investigate the independent predictors, on which prognostic models of the PCa cohort were established. To estimate the efficiency of prognostic model, the area under the receiver operating characteristic (ROC) curve (AUC) with censored data were generated using the ROC package (version 1.0.3) (http://bioconductor.org/packages/release/bioc/html/ROC.html).
AS-based cluster analysis
To better classify TCGA PCa cohort, hierarchical consensus clustering was performed in an unbiased and unsupervised manner using the “ConsensusClusterPlus” Rpackage (version 3.12) (https://www.bioconductor.org/packages/release/bioc/html/ConsensusClusterPlus.html). In order to clarify the distribution of stromal and immune cells in tumor tissues, stromal and immune scores were calculated using the “ESTIMATE” Rpackage (https://bioinformatics.mdanderson.org/estimate/rpackage.html). TIMER (http://timer.cistrome.org) was conducted to visually assess the gene expression and immune infiltration.
Statistical analyses
Differences in continuous variables were assessed using the student’s t-test and analysis of variance (ANOVA) test. Spearman’s correlation coefficients were addressed for non-normally distributed data. All calculated P values were two-sided and P<0.05 was considered statistically significant. All statistical analyses were carried out using R 3.6.2 (https://www.r-project.org).
Results
The AS profiling of 489 PCa patients was obtained from the TCGA SpliceSeq database and survival data of 469 patients were collected. The median follow-up duration was 28.8 months (range, 1–167.5 months). Seventy (14.3%) patients were diagnosed with disease progression and 10 (2.0%) patients died of PCa. In this PCa cohort, the 5-year overall survival (OS) and PFS were 98.8% and 88.5%, respectively. In TCGA SpliceSeq database, we preliminarily explored 44,070 AS events from 10,381 genes in PCa. There were seven AS splicing modes included (Figure 1A): alternate acceptor site (AA), alternate donor site (AD), alternate promoter (AP), alternate terminator (AT), exon skipping (ES), mutually exclusive exons (ME), and retained intron (RI). Of these seven splicing modes, ES had the highest frequency (33.2%). The prevailing phenomenon was that most AS events were observed in only a small set of study cohorts. We further explored the cancer-specific AS alternations associated with PFS, and a total of 20,723 AS events from 10,381 genes were obtained for the following analysis. After screening, ES still had the highest percentage (31.7%) of all splicing events, following by AT (18.2%) and AP (17.4%). Furthermore, seven different splicing patterns could occur in one gene, and most genes had more than one splicing variant (Figure 1B). Circos plots were simultaneously generated to present an intuitive cognition of genome-wide alternations in PCa (Figure 1C).
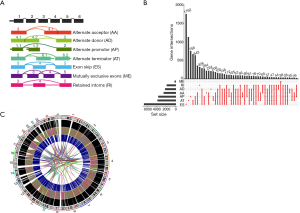
To investigate the prognostic value of AS events in PCa patients, univariate Cox regression analysis was performed to assess the prognostic efficacy of each AS event. In total, 2,805 AS signatures were found to be significantly related to PFS. A subset of overlapping AS events among the seven types of AS in PCa was illustrated using the UpSet plot diagram (Figure 2A). Prognosis-related AS events were exhibited as red dots in the volcano plot (Figure 2B). Obviously, one gene could have multiple corresponding PFS-related AS events in PCa. To further explore the gene atlas with variable AS types, a Venn diagram was generated to show the similarities and differences between the high and low PSI groups. There were 1,200 genes that had favorable AS events, 1,227 that had adverse AS events, with an intersection of 568 genes, which exhibited both (Figure 2C). The top 20 prognostic AS events of the seven patterns are shown in Figure 2D,E,F,G,H,I,J. Furthermore, SFs associated with these specific AS events were determined by survival analysis based on the gene expression profiles. In the correlation networks, there were 485 interactions between 65 PFS-associated SFs and 80 events, including 55 favorable and 35 unfavorable AS events (green dots) (Figure 3).
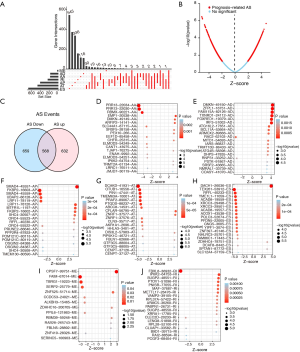
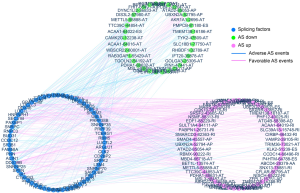
LASSO analysis was used to develop prognostic signatures based on AS events and prevent overfitting of the model, through which 12 AS events were screened out. The coefficient profile and partial likelihood deviance of the LASSO regression curve showed a strong potential to predict patient outcomes (Figure S1A and S1B). Based on the results of the LASSO regression model, multivariate Cox regression was addressed to further build the prognostic model and calculate the risk score of each cohort. Patients were divided into high- and low-risk groups according to the median risk score predicted by the final prognostic model. Survival analysis showed that the established model had a strong power to predict outcomes in PCa patients (P<0.001) (Figure 4A,B). Six AS events inferred were from the genes of acyl-CoA dehydrogenase family member 8 (ACAD8), dachshund family transcription factor 1 (DACH1), dermokine (DMKN), ring finger and FYVE like domain containing E3 ubiquitin protein ligase (RFFL), SMAD family member 4 (SMAD4), zinc finger protein like 1 (ZFPL1). The heatmap for each of the predictors (clustered by risk score) are shown in Figure 4C. The risk score curve and dot plot displayed a favorable efficiency to indicate outcomes in PCa patients (Figure 4D,E). The PFSs differed between the high- and low-risk groups (Figure S2). The validity of this prognostic model was represented by a ROC curve; the prognostic risk score system exhibited a high efficiency with an AUC of 0.793 for predicting the PFS of patients at 5 years (Figure 4F).
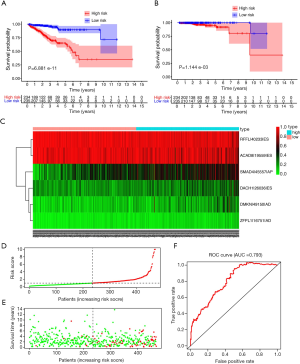
To better understand the molecular heterogeneity of PCa, unsupervised consensus analysis was conducted to investigate the intrinsic clusters of AS events and finally 487 cohorts were enrolled (Figure 5A): C1 (n=137, 38.0%), C2 (n=110, 22.5%), C3 (n=156, 31.9%), and C4 (n=84, 17.2%). Moreover, Kaplan-Meier analysis showed significant differences of OS and PFS among the different clusters (Figure 5B,C). The differences between each cluster of immune features (Immune Score, Stromal Score) were analyzed, and the result showed that different subgroups enjoyed distinct immune characteristics (Figure 5D,E). Furthermore, we evaluated the relationship between gene expression and immune status in TIMER. There was a positive correlation between ACAD8 expression and the infiltration of CD8+ T cells (Cor=0.327, P=8.12e-12) and macrophages (Cor=0.205, P=2.49e-05). The results also suggested that DACH1 expression was positively associated with the infiltration of B cells (Cor=0.345, P=5.81e-13), CD8+ T cells (Cor=0.445, P=1.22e-21), macrophages (Cor=0.359, P=4.49e-14), and neutrophils (Cor=0.319, P=3.04e-11). DMKN expression was positively related to the infiltration of CD4+ T cells (Cor=0.353, P=1.76e-13) and macrophages (Cor=0.325, P=1.02e-11). RFFL expression was negatively related to the infiltration of CD8+ T cells (Cor=0.54, P=6.28e-33). SMAD4 expression was positively related to the infiltration of B cells (Cor=0.206, P=2.21e-10), CD8+ T cells (Cor=0.598, P=9.68e-42), macrophages (Cor=0.395, P=5.83e-17), and dendritic cells (Cor=0.309, P=1.18e-10). ZFPL1 expression was negatively related to immune infiltration. The above results indicate that there were underlying links between the hub genes and immune infiltration, which may provide insight into the development of immunotherapy in PCa (Figure 6).
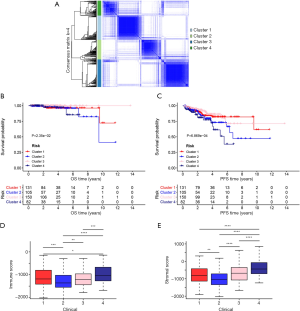
Discussion
PCa is one of the most common cancer threats to men. After radical treatment, a short interval to biochemical failure was suggested to be relevant with a high risk of tumor progression (20). An increasing number of studies have demonstrated that AS signatures play roles in the treatment resistance and progression of PCa. However, the function of these dysregulated AS events in PCa are still under investigation. With the development of high-throughput sequencing technology, genetic alterations could be detected constantly, thus providing new perspectives for exploring the prognostic markers of PCa. In our study, we systematically integrated the clinical and genomic data from TCGA and SpliceSeq to reveal the roles of AS in PCa. We used univariate analysis to identify seven subtypes of AS events associated with PFS. A predictive model was constructed, with a high efficiency of 0.792, and six hub genes were generated via multivariate analysis. Furthermore, biological heterogeneity was preliminarily revealed by a systematic analysis of AS-based clustering of PCa.
The existence of AS leads to the production of different mRNA isoforms and variant proteins with diverse physiological function from a single gene. The occurrence of splicing events is essential and important in eukaryotic gene expression, especially in hereditary cancer genes, which are susceptible to abnormal mutations in splicing sites (21). Large statistics from TCGA data across 32 cancer types provided substantial evidence that tumors have up to 30% more AS events than normal samples (7). Malignant cells undergo genomic dysregulation that leads to internal phenotype changes, enabling them to play a specific role in tumor development (22). Hence, understanding the regulation mechanism could help to provide deeper insights into the hallmarks of cancer. So far, a series of prognostic alternative transcripts in clinical applications have been confirmed, which predict the clinical outcomes and treatment response (23,24). In colorectal cancer (CRC), upregulation of Rac family small GTPase 1b (RAC1b) was believed to be a splicing biomarker with predictive potential. In particular, in CRC with BRAFV600E mutation, the high-frequency RAC1 splice variant is a widely accepted biomarker of poor prognosis in advanced tumors. In addition, RAC1b expression was reported to affect the prognosis of patients with metastatic CRC who received first-line FOLFOX/XELOX chemotherapy (25).
In PCa, upregulation of SFs has also been suggested with epithelial-mesenchymal transition (EMT) in the oncogenesis progress and disease development. After androgen deprivation, the expression of SF serine/arginine repetitive matrix protein 4 (SRRM4) is elevated and AS frequency of RE1-silencing transcription factor (REST) increased, leading to excessive production of REST truncations, which lack typical transcription repression domains. Consequently, these cancer cells turned into a new neuroendocrine phenotype that is less dependent on AR pathways (26). Mutation of Kruppel-like factor 6 (KLF6), which was well known as a tumor suppressor gene, was often observed in PCa. Germline single nucleotide polymorphisms (SNPs) in KLF6 can lead to increased generation of KLF6 splice variant 1 (KLF6- SV1), thereby influencing the ability of cell proliferation, as well as colony formation and invasion, indicating an inferior prognosis in PCa (27). In a recent study, heterogeneous nuclear ribonucleoprotein L (HNRNPL) was demonstrated to play important roles in PCa. It could directly regulate the AS behaviors of RNAs, including those genes that can play an important role in the development of PCa, such as androgen receptors. Moreover, HNRNPL could regulate the generation of circular RNA through reverse splicing. These studies provided new evidence to explore the mechanism of tumor progression and investigate potential therapeutic targets (28).
In our study, AS events of ACAD8 were shown to have a positive relationship with PFS, while SMAD4, DACH1, DMKN, and ZFPL1 variants were negatively correlated with PFS outcomes. Limited work had been done on ACAD8 in cancers previously. A previous study showed that AS in ACAD8, which encodes mitochondrial isobutyryl-CoA dehydrogenase, resulted in a mitochondrial defect and progressive hepatic steatosis in mice (29). In CRC development, alternative cleavage and polyadenylation (APA) of DMKN could alter the length of the 3'-untranslated region, which influences post-transcriptional regulation of gene expression. An APA shift towards a proximal polyA site in adenoma compared with normal tissue was observed, indicating that this event may occur even earlier in cancer progression (30). The loss of DMKN function in pancreatic ductal adenocarcinoma (PDAC) leads to decreased phosphorylation of signal transducer and activator of transcription 3, and increased activation of ERK1/2 and AKT serine/threonine kinases, thereby shrinking the proliferative ability of PDAC cells. In addition, DMKN knockdown reduced the invasion and migration behaviors of malignant cells, partially reversed the occurrence of EMT, slowed the tumor growth rate by reducing microvessel density, and prevent distant metastasis of tumors in xenograft models (31). The splicing variants of SMAD4 have been found in many cancers. In non-small cell lung cancer cells, recombinant expression of SMAD4 variants have significant effects on the proliferation and migration of cells, and also regulate cadherin 1 and vimentin protein expression (32). SMAD4 loss is correlated with worse clinical outcomes, resistance to chemotherapy, and decreased immune infiltrate, supporting its use as a prognostic marker in patients with CRC (33). In gastric cancer, knocking down of ZFPL1 can significantly increase cell mortality via autophagy, not apoptosis (34).
The construction of SF networks can help to further explore the potential mechanisms of splicing pathways. The analysis of AS events related to PFS may be of great significance in interpreting the mechanism of AS in tumor development. Considering that the regulation of key SFs may lead to widespread splicing abnormalities, we further conducted a systematic analysis of AS-based clustering. Under certain conditions, these variants could lead to changes in specific cell and tissue functions, providing tumor cells with a novel means to adapt the tumor microenvironment and escape from different immune barriers (35). Recent studies have shown that peptide-specific cytotoxic T lymphocyte responses could be elicited by peptides derived from BCR activator of RhoGEF and GTPase/ABL proto-oncogene splicing variants, providing a hint for exploring targets of cell-based immunotherapy (36).
In our primary investigation, expression of ACAD8, SMAD4, DACH1, DMKN, and RFFL were found to be moderately correlated with CD8+ T cells or macrophages. In PCa patients with programmed cell death-1 (PD-1) ligand (PD-L1) expression in ≥1% of tumor or stromal cells, we confirmed partial responses to pembrolizumab treatment with an impressive objective response rate of 17.4% (95% CI: 5.0–38.8%); 8 of 23 (34.8%) patients were assessed as stable (37). After ipilimumab therapy, the amount of CD4+ and CD8+ T cells increased, there was increased PD-1+, and ICOS+ subsets in blood samples were also observed. After treatment, PD-L1 expression in CD4+ T cells, CD8+ T cells, and CD68+ macrophages were higher than that in matched pretreatment PCa tissues (38). The results of this clinical trial provide further evidence for immune-checkpoint-blockade treatment as a promising approach for treating PCa. In addition, studies have shown that splicing-derived neoantigens may also be regarded as predictive biomarkers for evaluating the response efficiency of immune checkpoint blockade therapy. PD-1 induced cascade can suppress immune activation. Blocking these immune checkpoint cascades with monoclonal antibodies has subverted the treatment path of many cancers, leading to unprecedented long-term tumor response rate (39).
Conclusions
In this study, integration of splicing data from the TCGA SpliceSeq database with RNA sequencing data from TCGA for PCa were investigated. Important AS events and key hub genes associated with the progression of PCa were identified. In addition, survival analysis was performed and a Cox proportional hazard model was established to identify prognostic factors. A prognostic model composed of AS events was constructed, which exhibited favorable efficacy in predicting PFS. More importantly, a comprehensive clustering analysis revealed the inherent correlation between the molecular changes and immune characteristics in PCa. Nevertheless, this study was limited by lack of external experimental verification, and the predictors derived from the bioinformatics analysis require further confirmation by a series of laboratory experiments. Yet, this research could serve as reference for exploring novel clinical biomarkers and treatments to assist in the implementation of a new strategy for PCa.
Acknowledgments
Funding: Research in the authors’ laboratory was supported by the National Natural Funding of China (81402093, 81972744) and Anhui University Natural Science Research Project (No. KJ2019ZD13).
Footnote
Reporting Checklist: The authors have completed the REMARK reporting checklist. Available at https://dx.doi.org/10.21037/tau-21-585
Conflicts of Interest: All authors have completed the ICMJE uniform disclosure form (available at https://dx.doi.org/10.21037/tau-21-585). The authors have no conflicts of interest to declare.
Ethical Statement: The authors are accountable for all aspects of the work in ensuring that questions related to the accuracy or integrity of any part of the work are appropriately investigated and resolved. The study was conducted in accordance with the Declaration of Helsinki (as revised in 2013).
Open Access Statement: This is an Open Access article distributed in accordance with the Creative Commons Attribution-NonCommercial-NoDerivs 4.0 International License (CC BY-NC-ND 4.0), which permits the non-commercial replication and distribution of the article with the strict proviso that no changes or edits are made and the original work is properly cited (including links to both the formal publication through the relevant DOI and the license). See: https://creativecommons.org/licenses/by-nc-nd/4.0/.
References
- Siegel RL, Miller KD, Jemal A. Cancer statistics, 2020. CA Cancer J Clin 2020;70:7-30. [Crossref] [PubMed]
- Huang Y, Gu X, Wang Y, Hou J, Qi X. Prostate health index is useful for prostate cancer detecting in Chinese people. Transl Cancer Res 2019;8:836-9. [Crossref]
- Boorjian SA, Thompson RH, Tollefson MK, et al. Long-term risk of clinical progression after biochemical recurrence following radical prostatectomy: the impact of time from surgery to recurrence. Eur Urol 2011;59:893-9. [Crossref] [PubMed]
- Litwin MS, Tan HJ. The Diagnosis and Treatment of Prostate Cancer: A Review. JAMA 2017;317:2532-42. [Crossref] [PubMed]
- Robinson D, Van Allen EM, Wu YM, et al. Integrative Clinical Genomics of Advanced Prostate Cancer. Cell 2015;162:454. [Crossref] [PubMed]
- Paschalis A, Sharp A, Welti JC, et al. Alternative splicing in prostate cancer. Nat Rev Clin Oncol 2018;15:663-75. [Crossref] [PubMed]
- Kahles A, Lehmann KV, Toussaint NC, et al. Comprehensive Analysis of Alternative Splicing Across Tumors from 8,705 Patients. Cancer Cell 2018;34:211-24. [Crossref] [PubMed]
- Xiong HY, Alipanahi B, Lee LJ, et al. RNA splicing. The human splicing code reveals new insights into the genetic determinants of disease. Science 2015;347:1254806 [Crossref] [PubMed]
- Bonnal SC, López-Oreja I, Valcárcel J. Roles and mechanisms of alternative splicing in cancer - implications for care. Nat Rev Clin Oncol 2020;17:457-74. [Crossref] [PubMed]
- Li S, Hu Z, Zhao Y, et al. Transcriptome-Wide Analysis Reveals the Landscape of Aberrant Alternative Splicing Events in Liver Cancer. Hepatology 2019;69:359-75. [Crossref] [PubMed]
- Chen K, Xiao H, Zeng J, et al. Alternative Splicing of EZH2 pre-mRNA by SF3B3 Contributes to the Tumorigenic Potential of Renal Cancer. Clin Cancer Res 2017;23:3428-41. [Crossref] [PubMed]
- Yoshimi A, Lin KT, Wiseman DH, et al. Coordinated alterations in RNA splicing and epigenetic regulation drive leukaemogenesis. Nature 2019;574:273-7. [Crossref] [PubMed]
- Armenia J, Wankowicz SAM, Liu D, et al. The long tail of oncogenic drivers in prostate cancer. Nat Genet 2018;50:645-51. [Crossref] [PubMed]
- Zhang T, Karsh LI, Nissenblatt MJ, et al. Androgen Receptor Splice Variant, AR-V7, as a Biomarker of Resistance to Androgen Axis-Targeted Therapies in Advanced Prostate Cancer. Clin Genitourin Cancer 2020;18:1-10. [Crossref] [PubMed]
- Tan DSW, Chong FT, Leong HS, et al. Long noncoding RNA EGFR-AS1 mediates epidermal growth factor receptor addiction and modulates treatment response in squamous cell carcinoma. Nat Med 2017;23:1167-75. [Crossref] [PubMed]
- Yao J, Caballero OL, Huang Y, et al. Altered Expression and Splicing of ESRP1 in Malignant Melanoma Correlates with Epithelial-Mesenchymal Status and Tumor-Associated Immune Cytolytic Activity. Cancer Immunol Res 2016;4:552-61. [Crossref] [PubMed]
- Conway JR, Lex A, Gehlenborg N. UpSetR: an R package for the visualization of intersecting sets and their properties. Bioinformatics 2017;33:2938-40. [Crossref] [PubMed]
- Gu Z, Gu L, Eils R, et al. circlize Implements and enhances circular visualization in R. Bioinformatics 2014;30:2811-2. [Crossref] [PubMed]
- Shannon P, Markiel A, Ozier O, et al. Cytoscape: a software environment for integrated models of biomolecular interaction networks. Genome Res 2003;13:2498-504. [Crossref] [PubMed]
- Bandini M, Preisser F. UICC and AJCC 8th edition tumor-nodes-metastasis (TNM) classifications for patients treated with radical prostatectomy: reliable but not infallible prognostic tools. Ann Transl Med 2019;7:S41.
- Rhine CL, Cygan KJ, Soemedi R, et al. Hereditary cancer genes are highly susceptible to splicing mutations. PLoS Genet 2018;14:e1007231 [Crossref] [PubMed]
- Feinberg AP, Koldobskiy MA, Göndör A. Epigenetic modulators, modifiers and mediators in cancer aetiology and progression. Nat Rev Genet 2016;17:284-99. [Crossref] [PubMed]
- Calabretta S, Bielli P, Passacantilli I, et al. Modulation of PKM alternative splicing by PTBP1 promotes gemcitabine resistance in pancreatic cancer cells. Oncogene 2016;35:2031-9. [Crossref] [PubMed]
- Patel AP, Tirosh I, Trombetta JJ, et al. Single-cell RNA-seq highlights intratumoral heterogeneity in primary glioblastoma. Science 2014;344:1396-401. [Crossref] [PubMed]
- Alonso-Espinaco V, Cuatrecasas M, Alonso V, et al. RAC1b overexpression correlates with poor prognosis in KRAS/BRAF WT metastatic colorectal cancer patients treated with first-line FOLFOX/XELOX chemotherapy. Eur J Cancer 2014;50:1973-81. [Crossref] [PubMed]
- Li Y, Donmez N, Sahinalp C, et al. SRRM4 Drives Neuroendocrine Transdifferentiation of Prostate Adenocarcinoma Under Androgen Receptor Pathway Inhibition. Eur Urol 2017;71:68-78. [Crossref] [PubMed]
- Liu X, Gomez-Pinillos A, Loder C, et al. KLF6 loss of function in human prostate cancer progression is implicated in resistance to androgen deprivation. Am J Pathol 2012;181:1007-16. [Crossref] [PubMed]
- Fei T, Chen Y, Xiao T, et al. Genome-wide CRISPR screen identifies HNRNPL as a prostate cancer dependency regulating RNA splicing. Proc Natl Acad Sci U S A 2017;114:E5207-15. [Crossref] [PubMed]
- Sabbagha NG, Kao HJ, Yang CF, et al. Alternative splicing in Acad8 resulting a mitochondrial defect and progressive hepatic steatosis in mice. Pediatr Res 2011;70:31-6. [Crossref] [PubMed]
- Morris AR, Bos A, Diosdado B, et al. Alternative cleavage and polyadenylation during colorectal cancer development. Clin Cancer Res 2012;18:5256-66. [Crossref] [PubMed]
- Huang C, Xiang Y, Chen S, et al. Dermokine contributes to epithelial-mesenchymal transition through increased activation of signal transducer and activator of transcription 3 in pancreatic cancer. Cancer Sci 2017;108:2130-41. [Crossref] [PubMed]
- Wan R, Xu X, Ma L, et al. Novel Alternatively Spliced Variants of Smad4 Expressed in TGF-β-Induced EMT Regulating Proliferation and Migration of A549 Cells. Onco Targets Ther 2020;13:2203-13. [Crossref] [PubMed]
- Wasserman I, Lee LH, Ogino S, et al. SMAD4 Loss in Colorectal Cancer Patients Correlates with Recurrence, Loss of Immune Infiltrate, and Chemoresistance. Clin Cancer Res 2019;25:1948-56. [Crossref] [PubMed]
- Xie YZ, Ma WL, Meng JM, et al. Knockdown of ZFPL1 results in increased autophagy and autophagy-related cell death in NCI-N87 and BGC-823 human gastric carcinoma cell lines. Mol Med Rep 2017;15:2633-42. [Crossref] [PubMed]
- Marzese DM, Manughian-Peter AO, Orozco JIJ, et al. Alternative splicing and cancer metastasis: prognostic and therapeutic applications. Clin Exp Metastasis 2018;35:393-402. [Crossref] [PubMed]
- Volpe G, Cignetti A, Panuzzo C, et al. Alternative BCR/ABL splice variants in Philadelphia chromosome-positive leukemias result in novel tumor-specific fusion proteins that may represent potential targets for immunotherapy approaches. Cancer Res 2007;67:5300-7. [Crossref] [PubMed]
- Fay AP, Antonarakis ES. Blocking the PD-1/PD-L1 axis in advanced prostate cancer: are we moving in the right direction? Ann Transl Med 2019;7:S7. [Crossref] [PubMed]
- Gao J, Ward JF, Pettaway CA, et al. VISTA is an inhibitory immune checkpoint that is increased after ipilimumab therapy in patients with prostate cancer. Nat Med 2017;23:551-5. [Crossref] [PubMed]
- Ribas A, Wolchok JD. Cancer immunotherapyusing checkpoint blockade. Science 2018;359:1350-5. [Crossref] [PubMed]
(English Language Editor: A. Kassem)