Bioinformatics analysis reveals biomarkers with cancer stem cell characteristics in kidney renal clear cell carcinoma
Introduction
As a renal cortical tumor, the cytoplasmic growth pattern of Kidney renal clear cell carcinoma (KIRC) is similar to that of malignant epithelial cells, accounting for approximately 80–90% of renal cell carcinomas (1). Also, KIRC is usually resistant to radiotherapy and chemotherapy, such that surgery is the primary treatment. However, metastasis still occurs in 30% of patients undergoing surgery (2). Early detection of KIRC in patients contributes to more accurate clinical treatment. Therefore, there is an urgent need to identify new and reliable biomarkers to predict the prognosis of patients.
Many studies have shown that gene expression prediction models play a vital role in clinical prognosis. For example, Fatai et al. established a model to prove that the characteristics of 35 genes can distinguish fast-growing and slow-growing glioblastoma multiforme and predict the survival rate of known cancer subtypes (3). Han et al. analyzed reverse phase protein array (RPPA) data to understand the protein expression characteristics of KIRC survival time (4). Long et al. constructed a prognostic model for hepatocellular carcinoma (HCC) patients based on RNA sequencing data (5). Furthermore, Chen et al. and Wang et al. reported that potassium calcium-activated channel subfamily n member 4 (KCNN4) and aryl hydrocarbon receptor nuclear translocator like 2 (ARNTL2) affected the prognosis of renal clear cell carcinoma and the immune status of the tumor microenvironment, respectively (6,7). However, research on polygenic models for predicting the prognosis of KIRC patients is still limited. Here, we seek to use a range of methods to identify more potential KIRC-related genes.
Furthermore, on the basis of the theory of cancer stem cells (CSCs), some researchers have put forward a new concept, that is, stem index (8). The expression profile data of the sample mainly comes from The Cancer Genome Atlas (TCGA) and some other public databases. One-class logistic regression (OCLR), an innovative machine learning algorithm for logistic regression, has been used to extract the transcriptome and epigenetic feature sets of non-transformed pluripotent stem cells and their differentiated progeny (9). Two independent stem indexes are calculated: the messenger RNA expression-based stemness index (mRNAsi) and epigenetic regulation of mRNAsi (EGER-mRNAsi). The index range is 0-1. The closer to 1, the stronger are the stem cell characteristics of tumor cells.
Weighted gene co-expression network analysis (WGCNA) (10) is an effective and extensive tool for analyzing gene expression data and exploring network changes. In brief, after the expression profile data have been processed into weighted connections, WGCNA can be used to identify network topology and subnets called modules. Thus, only highly co-expressed genes can constitute a gene module (GM) that is linked through powerful connections in a network, and the corresponding modules and clinical features of interest can be associated (11).
This study identified key genes related to stemness by combining WGCNA and KIRC mRNAsi using the data of TCGA. Our findings establish a new method to identify stem cell-related genes and gain an in-depth understanding of the role of certain CSC-related genes.
We present the following article in accordance with the REMARK reporting checklist (available at https://dx.doi.org/10.21037/tau-21-647).
Methods
Data processing
The gene expression data of 50 normal samples and 588 human KIRC samples were obtained from the TCGA database. The merge script in Perl language was used to merge the RNA-sequencing (RNA-seq) results of 588 cancer samples and 50 normal samples into one matrix file. Then, we converted the relevant gene name from the ensemble ID no. into the corresponding gene symbol. The latest clinical data were downloaded from the database of TCGA, useful information was screened, including life status, staging, gender, age, and other information. The study was conducted in accordance with the Declaration of Helsinki (as revised in 2013).
mRNAsi in modules
mRNAsi can be described as the similarity between CSCs and cancer cells. The stemness index of the metastatic tumor was higher, and the stemness index was negatively correlated with the survival rate. As mentioned above, a comprehensive molecular characterization of KIRC samples from TCGA was performed to obtain the mRNAsi correlation index and molecular typing of each sample. The significant differences between the modules were verified using Kruskal-Wallis tests. The samples were divided into two groups by referring to this mRNAsi score. GraphPad Prism 8 (Graph Pad Software, San Diego, CA, USA) was used to analyze overall survival (OS) and the mRNAsi score to evaluate prognosis. The significance was calculated by the log-rank test and unpaired t-test.
Differentially expressed genes
The expression levels of normal tissues and tumor samples were compared using the ‘edge’ R package (The R Foundation for Statistical Computing). In addition, different expression genes (DEGs) were selected according to the following criteria: P value <0.05, fold change >2 and gene expression level >1.
WGCNA analysis
For the follow-up analysis the WGCNA R package (The R Foundation for Statistical Computing) was used. To ensure heterogeneity and accuracy of the bioinformatics analysis, we selected DEGs with the highest variance of 25%. First, we screened for the outliers in the RNA-seq data. Then, a Pearson correlation matrix was used to create the co-expression analysis of paired genes. Next, a weighted adjacency matrix was created using the power function amn = |cmn|b. An appropriate value of b was chosen to create a co-representation network. Then the adjacency matrix was further transformed into a topological overlap matrix (TOM) to detect the connectivity of the gene. Eventually, using the average linkage hierarchical clustering method, on the basis of the TOM dissimilarity, a gene tree greater than 30 was obtained, and the module tree was built for further analysis.
Search for significant modules
As the module for further analysis, the hierarchical clustering module most closely related to epigenetically regulated mRNAsi (EREG-mRNAsi) and mRNAsi was selected. Computational gene significance (GS) is the correlation between gene expression, EREG-mRNAsi, and mRNAsi and represents the correlation between each gene and feature in the module. In the module, modular meaning (MS) is the significant average of all genes. We used a threshold value of 0.55 to merge similar modules and then considered the module with the largest MS as the module with the highest correlation with the sample characteristics. In the principal component analysis of the gene expression matrix in each module, the first principal component obtained was defined as the module eigengenes (ME). In this study, the module with the highest MS value was considered to be related to EREG mRNAsi and mRNAsi. This module was selected for further the study.
Screening hub genes
After defining the important modules, the GS and module members of each gene were calculated module membership (MM), the correlation between the module’s genes and the gene expression profile). The correlation between genes and stemness index increased as the correlation between genes and important modules increased. Thus, the inclusion criteria of the hub gene were set as correlations for gene GS >0.4 and MM >0.6.
Interactions of hub genes
We used the Search Tool for the Retrieval of Interacting Genes/Proteins (STRING) (version 11.0) to visualize and study protein-protein interaction (PPI) networks. According to the gene expression level, the co-expression relationship between hub genes was calculated to determine their transcriptional strength. Pearson correlations between genes were calculated by using the R corrplot package 4 (The R Foundation for Statistical Computing). Then, we used the online GEPIA 5 database to verify whether the hub gene expression in tumor tissue was higher, and whether there were differences in the expression of hub genes for different tumor, node, and metastasis (TNM) stages.
Establishment of the prognostic signature
Univariate Cox regression analysis was used to evaluate the relationship between the expression level of each hub gene and OS. The Akaike information criterion (AIC) was used to input the hub genes with P values <0.05 into a multivariate Cox regression analysis. The corresponding data obtained by multivariate Cox proportional hazard regression analysis was used to create the risk scoring formula (P value <0.05). In formula 1, weight I is the coefficient of each important hub gene, GI is the expression value of the hub gene, and n is the number of hub genes with prognostic value. The patients were divided into a low-risk group (< medium risk score) and a high-risk group (> medium risk score), and then the Kaplan-Meier method and log-rank test were used to evaluate the differences in survival outcome and OS of high-risk and low-risk patients. Finally, the receiver operating characteristic (ROC) curve over time was used to verify the accuracy of the signature. The classical ROC curve analysis method assumes that the change of a single event and result over time is fixed, but in fact, the disease state and results change over time. A P value <0.05 was considered as indicating statistical significance.
Generating the nomogram and assessment
To minimize the influence of confounding factors, multivariate and univariate Cox regression analyses were used to assess the differences in risk scores and clinical features. A P value <0.05 was considered as indicating statistical significance. The nomogram of 1-, 3-, and 5-year survival rates was constructed using the RMS software package to visualize the prediction results.
Statistical analyses
Survival description was illustrated by the Kaplan-Meier curves with P value determined by a log-rank test. Continuous variables were compared by Mann-Whitney U test. We considered P<0.05 to indicate a statistically significant difference. Data analyses were performed using R program (R 4.3.1), using the survival and pROC packages (downloaded from Bio-conductor).
Results
Correlation between clinical features and mRNAsi in patients with KIRC
Twenty-six KIRC samples with insufficient clinical information and 11 KIRC samples that had no mRNAsi information were excluded from the TCGA dataset. We found that KIRC mRNAsi was significantly different from normal tissues. The level of mRNAsi in tumor tissues was significantly higher than that in normal tissues (Figure 1A). There were significant mRNAsi differences between different stages (Figure 1B,1C). The OS and progression-free survival (PFS) rates of high mRNAsi KIRC patients were significantly lower than those of low mRNAsi patients (Figure 1D,1E).
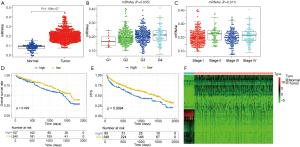
Identification of DEGs
Our aim was to compare the two groups to identify DEGs. In total, we found 7,369 DEGs, including 5,467 upregulated genes and 1,902 downregulated genes. The heat map of the DEGs is shown in Figure 1F.
WGCNA: the most important module and gene identification
After excluding 48 outlier samples, all DEGs were included in the co-expression network (Figure 2A), where B=3 satisfies the soft threshold parameter of the scale-free structure, and the curve reaches R2=0.935. Merging threshold was set to 0.55 to merge similar modules, resulting in 13 modules (Figure 2B). After evaluating the correlation between the module and patient characteristics, EREG-mRNA, and mRNAsi, we found the green module was negatively correlated with the mRNA expression of patients with KIRC (R2=−0.71, P=8e-77) (Figure 2C). In contrast, the yellow-brown module was positively correlated with mRNA expression in patients with KIRC (R2=0.42, P=1e-22) (Figure 2C). Moreover, genes in green modules (COR =0.78, P=3.8e-98) had high GS and MM characteristics (Figure 3A). Therefore, the green module was selected as the most important module in the follow-up study, because it showed the highest correlation. Finally, we obtained 37 genes based on thresholds of GS >0.4 and MM >0.6.
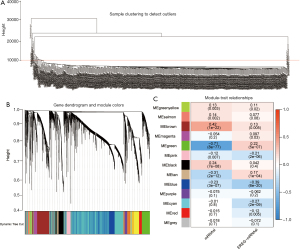
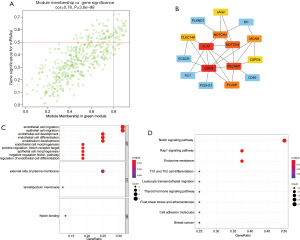
Function annotation
Using the STRING database, a PPI network composed of the first 16 central genes was constructed. The PPI network consisted of 16 nodes and 25 edges (Figure 3B), the average node degree was 6.8, and the correlation was strong. Because the green modules were the modules most closely related to mRNAsi, Gene Ontology (GO) and Kyoto Encyclopedia of Genes and Genomes (KEGG) analysis were performed for the genes included in the green module, and these were then introduced to enrich the results (Figure 3C,3D). The green module showed the strongest correlation with mRNAsi and was highly enriched in Notch binding, endothelial cell development, Notch signaling pathway, and Rap 1 signaling pathway.
Validated hub gene and construct PPI network
The expression level of the first 10 central genes in tumor tissues was higher than that in normal samples (Figure 4). Moreover, in different tumor (T), node (N), metastasis (M) stages, the 10 central genes were differentially expressed (Figure 5).
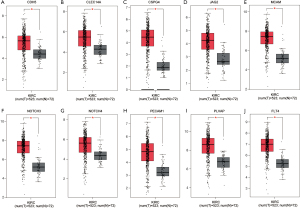
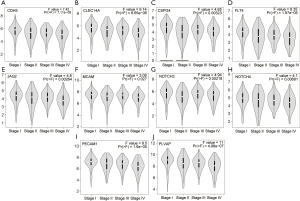
Prognostic signature establishment
To screen the predictive prognostic biomarker model, we used Cox regression analysis and finally screened out seven genes as biomarkers (Figure 6A). Prognostic markers included seven central genes, which were included in a multivariate Cox proportional hazard regression analysis, then the coefficients of the central genes were obtained by calculating the risk score in equation 1. The risk score for each patient was calculated as follows: Risk score = (−0.035× expression level of CDH5) + (−0.028× expression level of ECSCR) + (0.076× expression level of JAG2) + (0.005× expression level of MCAM) + (−0.012× expression level of PECAM1) + (−0.003× expression level of PLVAP) + (−0.021× expression level of CLEC14A).
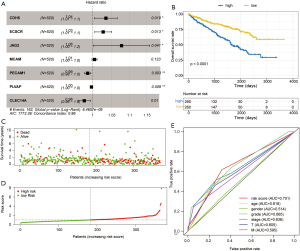
Compared with low-risk patients, high-risk patients had a significantly worse prognosis (Figure 6B). We counted the survival of all the samples (Figure 6C), then, take the median risk score of all samples as the threshold value to divide patients into a high-risk combination and a low-risk group (Figure 6D). A classical ROC curve analysis showed that the risk score biomarker had the highest accuracy and specificity and that the area under the curve (AUC) value was 0.701 (Figure 6E).
Generating the nomogram and assessment
After univariate and multivariate Cox regression analysis, the nomogram included the following independent risk factors (Table 1): gender, stage, metastasis stage (M0 vs. M1), and risk score (low vs. high). These risk factors made up the nomogram. The calibration curve had a good ability to predict 1-, 3-, and 5-year OS (Figure 7).
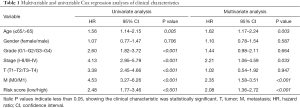
Full table
Discussion
KIRC is associated with high morbidity and mortality, but its pathogenesis remains unclear (12). Although several biomarkers for the prognosis and diagnosis of KIRC have been reported, their reliability and effectiveness still need clinical verification (13). Moreover, existing prognostic models ignored the correlation between mRNAsi and genes. The latest study by Malta et al. analyzed the correlations between mRNAsi-related genes and the survival and prognosis of cancer patients using TCGA tumors (8). However, there is no report on the molecular markers of KIRC mRNAsi. Therefore, we performed WGCNA analysis on TCGA data of KIRC patients and identified gene modules (GMs) related to mRNAsi. In this work, we used TCGA database data and the corresponding mRNAsi of each sample to determine the significance of mRNAsi related to the clinical characteristics of KIRC patients. Also, 10 key genes were identified by analyzing the genes in the first GMs, and then they were used to construct a new KIRC survival model. Our study shows that these 10 genes are potential prognostic and therapeutic targets for KIRC. The results also show that the hub gene is expressed more strongly in tumor tissues than in normal tissues, and that it has important prognostic significance for the progression of the disease. Finally, after correcting for confounding factors, a prognostic marker that contained seven prognostic genes with good predictive power was obtained. These seven central genes are highly expressed in KIRC and are also related to TNM staging and prognosis. These genes are: CDH5, ECSCR, JAG2, MCAM, PECAM1, PLVAP, and CLEC14A.
Cadherin 5 (Cadherin 5, CDH5), belongs to type II CDH, usually also called vascular endothelial CDH. Cadherin 5 plays an important role in contact inhibition, cell adhesion, endothelial cell migration, and apoptosis (14). However, abnormal expression of CDH5 was observed in various malignant tumor cells (15). Cadherin 5 plays a vital role in the angiogenesis simulation of melanoma cells or glioma. Cadherin 5 promotes tumor cell proliferation and invasion in breast cancer cells by stimulating transforming growth factor (TGF)-β signaling (16). In metastatic breast cancer, CDH5 expression level and CDH5 glycosylation represent biomarker tests that can distinguish patients with metastatic breast cancer from those without metastases (17).
Endothelial cell-specific chemotaxis regulator (ECSCR) is also synonymously named endothelial-cell-specific molecule 2 (ECSM2). ECSCR is an endothelial cell connexin protein, which is involved in the migration, apoptosis, and regulation of endothelial cells. The expression of ECSCR protein in endothelial cells is downregulated, indicating that the chemotaxis of matrix gel is reduced and angiogenesis is impaired. In multivariate analysis, ECSCR showed a tendency to become an independent prognostic marker for lung cancer in addition to the clinical parameter of age (18). Also, previous studies have found that higher levels of ECSCR expression are associated with longer OS in primary lung cancer samples (19).
Platelet and endothelial cell adhesion molecule-1 (PECAM1) is a common adhesion molecule in vascular endothelial cells (PECAM1, also known as CD31). It encodes a protein involved in the growth and proliferation of tumors, including extracellular circulation, vascular permeability, and angiogenesis (20). Many studies have shown that PECAM1 is involved in the progression of a variety of malignant tumors, including melanoma, lung cancer, and breast cancer (21,22). PECAM1 regulates tumor microenvironment (TME) and tumor cell proliferation, is related to the progress of tumor metastasis (23) and has significant differential expression in HCC (24). We need to further explore the expression of PECAM1 in KIRC and the molecular mechanism leading to tumorigenesis and discover potential therapeutic targets.
The JAG2 gene consists of 26 exons and is located on chromosome 14q32, encoding 5,825 BP and 5,721 BP mRNA variants (GenBank NM_002226.4 and NM_145159.2). The JAG2 protein contains an extracellular DSL region, a signal peptide region, 16 EGF-like repeat regions, a transmembrane region, a cysteine-rich region, and a short cytoplasmic region containing 132 amino acids (25). Many studies have shown that JAG2 promotes tumor cell metastasis in a variety of tumors (26-28).
PLVAP, CLEC14A, and MCAM also play an important role in the development of a tumor. MCAM is expressed in many tumors and is correlated with cancer progression and metastasis (29). PLVAP is the only known component of the diaphragm that is necessary for diaphragmatic muscle formation (30). PLVAP has been associated with cancer, traumatic spinal cord injury, acute ischemic encephalopathy, graft glomerulopathy, Norrie disease, and diabetic retinopathy (31). CLEC14A is a glycoprotein that is selectively overexpressed in the vascular system of many solid human tumors, and as such has attracted considerable attention as a target antigen (32). Earlier studies found that CLEC14A may be a tumor endothelial marker (33).
Therefore, all the genes included in the markers are significant to tumors, and they are significantly associated with KIRC survival. Our study indicates that CDH5, ECSCR, JAG2, MCAM, PECAM1, PLVAP, and CLEC14A play an essential role in KIRC. In the current treatment of KIRC, the US FDA has approved a variety of targeted drugs for the first-line treatment of advanced kidney cancer, including erlotinib, sunitinib, sorafenib, pazopanib, and axitinib, temsirolimus, everolimus, bevacizumab, cabozantinib and lenvatinib. These drugs are mainly anti-angiogenesis tyrosine kinase inhibitors and mTOR inhibitors (34). In addition, the tumor microenvironment is a key point that has to be considered in the treatment of KIRC. Angiogenesis and immunosuppression are the salient features of the tumor microenvironment of KIRC. Furthermore, the tumor stem cell microenvironment will bring new ideas for tumor treatment (35). More and more evidences show that CSCs are the key cell for tumorigenesis and metastasis. Our analysis found that CSCs showed activation of NOTCH signal in KIRC. Notch signaling is one of the most active pathways in tumor cells, and it plays a key role in angiogenesis and tumor stem cell self-renewal. Therefore, exploring the mechanism of Notch signaling pathway in CSCs may help to discover new treatment strategies for KIRC (36,37). In addition, Zhou et al. found that there is a closely correlation between the biological clock genes and immune infiltration: genes involved in the circadian rhythm in KIRC tissues are dysregulated, causing the changes of tumor microenvironment. Exploring the circadian clock genes and the tumor microenvironment of KIRC is conducive to a deeper understanding of KIRC (12).
Our study has several limitations. First of all, we used public data to verify our results and did not undertake additional experiments to confirm these results. Second, we cannot guarantee the quality of the data, because our research data come from online tools in public databases. Thus, further well-designed large-sample biological studies are needed. Despite these drawbacks, the significant and consistent correlations of our biomarker with OS with KIRC indicate that it is a potentially powerful prognostic marker for KIRC.
Acknowledgments
Funding: This work was supported by the National Science and Technology Major Project during the 13th Five-Year Plan Period (2019ZX09721001-007-002); Shenzhen Science and Technology Project (Project number: JCYJ20180507183842516).
Footnote
Reporting Checklist: The authors have completed the REMARK reporting checklist. Available at https://dx.doi.org/10.21037/tau-21-647
Conflicts of Interest: All authors have completed the ICMJE uniform disclosure form (available at https://dx.doi.org/10.21037/tau-21-647). All authors report that this work was supported by the National Science and Technology Major Project during the 13th Five-Year Plan Period (2019ZX09721001-007-002) and Shenzhen Science and Technology Project (Project number: JCYJ20180507183842516). The authors have no other conflicts of interest to declare.
Ethical Statement: The authors are accountable for all aspects of the work in ensuring that questions related to the accuracy or integrity of any part of the work are appropriately investigated and resolved. The study was conducted in accordance with the Declaration of Helsinki (as revised in 2013).
Open Access Statement: This is an Open Access article distributed in accordance with the Creative Commons Attribution-NonCommercial-NoDerivs 4.0 International License (CC BY-NC-ND 4.0), which permits the non-commercial replication and distribution of the article with the strict proviso that no changes or edits are made and the original work is properly cited (including links to both the formal publication through the relevant DOI and the license). See: https://creativecommons.org/licenses/by-nc-nd/4.0/.
References
- Hsieh JJ, Purdue MP, Signoretti S, et al. Renal cell carcinoma. Nat Rev Dis Primers 2017;3:17009. [Crossref] [PubMed]
- Zhu J, Liu Z, Zhang Z, et al. Development and internal validation of nomograms for the prediction of postoperative survival of patients with grade 4 renal cell carcinoma (RCC). Transl Androl Urol 2020;9:2629-39. [Crossref] [PubMed]
- Fatai AA, Gamieldien J. A 35-gene signature discriminates between rapidly- and slowly-progressing glioblastoma multiforme and predicts survival in known subtypes of the cancer. BMC Cancer 2018;18:377. [Crossref] [PubMed]
- Han G, Zhao W, Song X, et al. Unique protein expression signatures of survival time in kidney renal clear cell carcinoma through a pan-cancer screening. BMC Genomics 2017;18:678. [Crossref] [PubMed]
- Long J, Zhang L, Wan X, et al. A four-gene-based prognostic model predicts overall survival in patients with hepatocellular carcinoma. J Cell Mol Med 2018;22:5928-38. [Crossref] [PubMed]
- Chen S, Wang C, Su X, et al. KCNN4 is a potential prognostic marker and critical factor affecting the immune status of the tumor microenvironment in kidney renal clear cell carcinoma. Transl Androl Urol 2021;10:2454-70. [Crossref] [PubMed]
- Wang S, Ma X, Ying Y, et al. Upregulation of ARNTL2 is associated with poor survival and immune infiltration in clear cell renal cell carcinoma. Cancer Cell Int 2021;21:341. [Crossref] [PubMed]
- Chen M, Ye X, Wang R, et al. Research progress of cancer stem cells and IL-6/STAT3 signaling pathway in esophageal adenocarcinoma. Transl Cancer Res 2020;9:363-71. [Crossref]
- Sokolov A, Paull EO, Stuart JM. One-class detection of cell states in tumor subtypes. Pac Symp Biocomput 2016;21:405-16. [Crossref] [PubMed]
- Langfelder P, Horvath S. WGCNA: an R package for weighted correlation network analysis. BMC Bioinformatics 2008;9:559. [Crossref] [PubMed]
- Niemira M, Collin F, Szalkowska A, et al. Molecular Signature of Subtypes of Non-Small-Cell Lung Cancer by Large-Scale Transcriptional Profiling: Identification of Key Modules and Genes by Weighted Gene Co-Expression Network Analysis (WGCNA). Cancers (Basel) 2019;12:37. [Crossref] [PubMed]
- Zhou L, Luo Z, Li Z, et al. Circadian clock is associated with tumor microenvironment in kidney renal clear cell carcinoma. Aging (Albany NY) 2020;12:14620-32. [Crossref] [PubMed]
- Chen L, Xiang Z, Chen X, et al. A seven-gene signature model predicts overall survival in kidney renal clear cell carcinoma. Hereditas 2020;157:38. [Crossref] [PubMed]
- Cavallaro U, Liebner S, Dejana E. Endothelial cadherins and tumor angiogenesis. Exp Cell Res 2006;312:659-67. [Crossref] [PubMed]
- Mao XG, Xue XY, Wang L, et al. CDH5 is specifically activated in glioblastoma stemlike cells and contributes to vasculogenic mimicry induced by hypoxia. Neuro Oncol 2013;15:865-79. [Crossref] [PubMed]
- Haidari M, Zhang W, Caivano A, et al. Integrin α2β1 mediates tyrosine phosphorylation of vascular endothelial cadherin induced by invasive breast cancer cells. J Biol Chem 2012;287:32981-92. [Crossref] [PubMed]
- Fry SA, Robertson CE, Swann R, et al. Cadherin-5: a biomarker for metastatic breast cancer with optimum efficacy in oestrogen receptor-positive breast cancers with vascular invasion. Br J Cancer 2016;114:1019-26. [Crossref] [PubMed]
- Pircher A, Fiegl M, Untergasser G, et al. Favorable prognosis of operable non-small cell lung cancer (NSCLC) patients harboring an increased expression of tumor endothelial markers (TEMs). Lung Cancer 2013;81:252-8. [Crossref] [PubMed]
- Pircher A, Jöhrer K, Kocher F, et al. Biomarkers of evasive resistance predict disease progression in cancer patients treated with antiangiogenic therapies. Oncotarget 2016;7:20109-23. [Crossref] [PubMed]
- He Y, Liu Z, Qiao C, et al. Expression and significance of Wnt signaling components and their target genes in breast carcinoma. Mol Med Rep 2014;9:137-43. [Crossref] [PubMed]
- Piali L, Hammel P, Uherek C, et al. CD31/PECAM-1 is a ligand for alpha v beta 3 integrin involved in adhesion of leukocytes to endothelium. J Cell Biol 1995;130:451-60. [Crossref] [PubMed]
- Ilhan-Mutlu A, Siehs C, Berghoff AS, et al. Expression profiling of angiogenesis-related genes in brain metastases of lung cancer and melanoma. Tumour Biol 2016;37:1173-82. [Crossref] [PubMed]
- DeLisser H, Liu Y, Desprez PY, et al. Vascular endothelial platelet endothelial cell adhesion molecule 1 (PECAM-1) regulates advanced metastatic progression. Proc Natl Acad Sci U S A 2010;107:18616-21. [Crossref] [PubMed]
- Sarathi A, Palaniappan A. Novel significant stage-specific differentially expressed genes in hepatocellular carcinoma. BMC Cancer 2019;19:663. [Crossref] [PubMed]
- Shawber C, Boulter J, Lindsell CE, et al. Jagged2: a serrate-like gene expressed during rat embryogenesis. Dev Biol 1996;180:370-6. [Crossref] [PubMed]
- Hatano K, Saigo C, Kito Y, et al. Overexpression of JAG2 is related to poor outcomes in oral squamous cell carcinoma. Clin Exp Dent Res 2020;6:174-80. [Crossref] [PubMed]
- He W, Chan CM, Wong SC, et al. Jagged 2 silencing inhibits motility and invasiveness of colorectal cancer cell lines. Oncol Lett 2016;12:5193-8. [Crossref] [PubMed]
- Fiaschetti G, Schroeder C, Castelletti D, et al. NOTCH ligands JAG1 and JAG2 as critical pro-survival factors in childhood medulloblastoma. Acta Neuropathol Commun 2014;2:39. [Crossref] [PubMed]
- Stalin J, Traboulsi W, Vivancos-Stalin L, et al. Therapeutic targeting of soluble CD146/MCAM with the M2J-1 monoclonal antibody prevents metastasis development and procoagulant activity in CD146-positive invasive tumors. Int J Cancer 2020;147:1666-79. [Crossref] [PubMed]
- Hamilton BJ, Tse D, Stan RV. Phorbol esters induce PLVAP expression via VEGF and additional secreted molecules in MEK1-dependent and p38, JNK and PI3K/Akt-independent manner. J Cell Mol Med 2019;23:920-33. [Crossref] [PubMed]
- Guo L, Zhang H, Hou Y, et al. Plasmalemma vesicle-associated protein: A crucial component of vascular homeostasis. Exp Ther Med 2016;12:1639-44. [Crossref] [PubMed]
- Zhuang X, Maione F, Robinson J, et al. CAR T cells targeting tumor endothelial marker CLEC14A inhibit tumor growth. JCI Insight 2020;5:138808 [Crossref] [PubMed]
- Robinson J, Whitworth K, Jinks E, et al. An evaluation of the tumour endothelial marker CLEC14A as a therapeutic target in solid tumours. J Pathol Clin Res 2020;6:308-19. [Crossref] [PubMed]
- Barata PC, Rini BI. Treatment of renal cell carcinoma: Current status and future directions. CA Cancer J Clin 2017;67:507-24. [Crossref] [PubMed]
- Şenbabaoğlu Y, Gejman RS, Winer AG, et al. Tumor immune microenvironment characterization in clear cell renal cell carcinoma identifies prognostic and immunotherapeutically relevant messenger RNA signatures. Genome Biol 2016;17:231. Erratum in: Genome Biol 2017;18:46. [Crossref] [PubMed]
- Díaz-Montero CM, Rini BI, Finke JH. The immunology of renal cell carcinoma. Nat Rev Nephrol 2020;16:721-35. [Crossref] [PubMed]
- Venkatesh V, Nataraj R, Thangaraj GS, et al. Targeting Notch signalling pathway of cancer stem cells. Stem Cell Investig 2018;5:5. [Crossref] [PubMed]